ABSTRACT
Conversational technologies such as chatbots have shown to be promising in eliciting self-disclosure in several contexts. Implementing such a technology that fosters self-disclosure can help to assess sensitive topics such as behaviours that are perceived as unaccepted by others, i.e. the exposure to unaccepted (alternative) news sources. This study tests whether a conversational (chatbot) format, compared to a traditional web-based survey, can enhance self-disclosure in the political news context by implementing a two-week longitudinal, experimental research design (n = 193). Results show that users disclose unaccepted news exposure significantly more often to a chatbot, compared to a traditional web-based survey, providing evidence for a chatbots’ ability to foster the disclosure of sensitive behaviours. Unlike our hypotheses, our study also shows that social presence, intimacy, and enjoyment cannot explain self-disclosure in this context, and that self-disclosure generally decreases over time.
Conversational technologies (such as chatbots, animated virtual characters, or embodied conversational agents) are making their way into a wide variety of domains such as customer service, shopping, education, or the political news context by sending users personalised (news) recommendations (e.g. Maniou and Veglis Citation2020; Shin Citation2022; Yin Citation2019). Most notably, they are utilised in domains in which users are urged to reveal information about themselves, for example health-related, or other personal or emotional details (for an overview in the health domain, see Laranjo et al. Citation2018). Chatbots can be defined as programmes that use natural language to interact in a dialogical manner and simulate human conversations (Westerman, Cross, and Lindmark Citation2019). By doing so, they utilise the benefits of human-to-human communication: They can build rapport between user and chatbot and create a social environment for users to feel comfortable to reveal information about themselves (Pickard, Roster, and Chen Citation2016). At the same time, by having a non-human nature – they have technological benefits, such as perceivably being non-judgmental, objective, or free of bias (Pickard, Roster, and Chen Citation2016; Sundar and Kim Citation2019).
An especially important field of application is the potential of conversational technologies for self-disclosure in survey research. Respondents might avoid self-disclosing personal information in surveys because of fear of negative evaluation. While some studies explore the overall advantages of conversational technologies over traditional web-based surveys (Kim, Lee, and Gweon Citation2019; Xiao et al. Citation2020; Zarouali et al. Citation2023), self-disclosure of personal information to a conversational agent in comparison to other survey formats has not been extensively studied yet. We know, however, from related research in the health sector that conversational technologies are promising in eliciting self-disclosure (Crutzen et al. Citation2011; Lucas et al. Citation2014). Hence, we argue that implementing a chatbot could also be useful to enhance self-disclosure of sensitive topics such as behaviours that are deemed unacceptable.
People’s hesitancy to self-disclose unacceptable behaviours becomes especially prevalent in political news context. Assessing media usage behaviour that might be seen as unacceptable has been a major challenge for media exposure research due to the peculiarity of asking respondents about their use (Steppat, Herrero, and Esser Citation2020). A share of the population avoids legacy information sources intentionally when familiarising themselves with political issues (Bennett and Livingston Citation2018; Newman et al. Citation2021), and some turn to alternative online sources that report ‘a proclaimed and/or (self-) perceived corrective, opposing the overall tendency of public discourse’ (Holt, Ustad Figenschou, and Frischlich Citation2019, 862), including information of questionable accuracy (Bennett and Livingston Citation2018). These citizens, however, might not disclose exposure to such sources openly as they perceive this behaviour as unaccepted by the majority. Given its potential involvement in the rise of populism and fears of online ‘radicalisation’ (Holt, Ustad Figenschou, and Frischlich Citation2019), news exposure behaviour provides an important vantage point for studying self-disclosure of unaccepted behaviours.
Hence, this study tests whether a chatbot format (compared to a traditional web-based survey) can be a useful tool to enhance self-disclosure of perceived unaccepted news exposure. By doing so, we contribute to the growing body of research examining conversational technologies and self-disclosure. Furthermore, we investigate three mechanisms that have been shown to be related to self-disclosure in the context of conversational technologies and that can potentially explain self-disclosure towards a chatbot: Social presence (see Chaves and Gerosa Citation2021; Ciechanowski et al. Citation2019), perceived intimacy (see Croes and Antheunis Citation2021), and enjoyment (see Lee and Choi Citation2017). Lastly, we provide a methodological contribution to conversational technology research by assessing self-disclosure towards a chatbot with a longitudinal, diary-style research design.
1. Previous work on self-disclosure in conversational technology research
Self-disclosure – defined as the act of revealing personal information about oneself to someone else (Archer and Burleson Citation1980; Schmidt and Cornelius Citation1987) – is an important concept in the research field concerning conversational technologies. Research has established the benefits of self-disclosure towards conversational technologies; it showed for example that self-disclosure towards a chatbot has positive effects on user satisfaction and enhances relationship building between users and chatbots (S. Y. Lee and Choi Citation2017; Skjuve et al. Citation2022), or that disclosing more intimate information towards a chatbot can positively influence emotional, relational and psychological outcomes (Ho, Hancock, and Miner Citation2018; Y.-C. Lee et al. Citation2020).
Moreover, conversational technologies have been largely shown to be promising in eliciting self-disclosure. Research in the health sector demonstrated the potential of chatbots for delivering health-promotion initiatives to adolescents to create a comfortable environment for discussing sensitive topics (Crutzen et al. Citation2011), and to adults to reduce depression and anxiety (Fitzpatrick, Darcy, and Vierhile Citation2017). Previous work has shown chatbots to encourage self-disclosure when performing small talk (Y.-C. Lee et al. Citation2020), and most importantly for the current research, chatbots leading to more high-quality self-disclosure data in survey research than using a traditional web-based survey (Kim, Lee, and Gweon Citation2019; Xiao et al. Citation2020).
In the political news context, increasing polarisation in society and heated debates around political topics, such as climate change, election outcomes, or vaccination, spilled over to the sources of information itself. The news outlets someone visits have become an increasing issue for disagreement, as they give away a political stance, an elite-skepticism, the belief in conspiracy narratives, or populist attitudes (Bennett and Livingston Citation2018; Holt, Ustad Figenschou, and Frischlich Citation2019; Schulz Citation2019). Conversational agents survey techniques can potentially help to assess this exposure behaviour, as previous research in the era of news exposure has shown that when trusted, conversational interfaces can lead to reduced privacy concerns and increased self-disclosure (Shin et al. Citation2022).
Research comparing conversational technologies with ‘real’ human interaction partners, provided evidence that the non-human nature of virtual humans led to higher willingness to disclose personal health-related information compared to a human conversation partner (Lucas et al. Citation2014). Even though some scholars did not find any differences in emotional disclosures when interacting with a chatbot or with a human (Ho, Hancock, and Miner Citation2018), research on embodied conversational agents as interviewers has shown respondents preference for non-human over human interaction partners when discussing sensitive topics (Pickard, Roster, and Chen Citation2016).
Given this considerable body of literature supporting the potential for conversational technologies to elicit self-disclosure, chatbots might also be able to create a comfortable environment for people to disclose other types of information – in particular, behaviours that might be deemed unacceptable. One important behaviour that people might not want to discuss openly is the use of perceived unaccepted news exposure.
2. The political news context: perceived unaccepted news exposure
The rise of digital media has opened up new possibilities for citizens to be informed about public affairs (Bennett and Livingston Citation2018), and at the same time has complicated the correct assessment of news exposure further. New online-only outlets, such as Breitbart in the United States, the German version of Epoch Times in Germany (Hettena Citation2019; Levy et al. Citation2018), De Andere Krant, Gezond Verstand and Café Weltschmerz in the Netherlands (Newman et al. Citation2021), or Uncut-News and conviva-plus in Switzerland (Fög - Forschungsinstitut Öffentlichkeit und Gesellschaft Citation2017), have established themselves as an ‘alternative’ to legacy news media, in which trust is declining in specific segments of the population (Fawzi Citation2019). Alternative media are found at both the left and right wing of the political spectrum (Atkinson and Kenix Citation2019; Haller, Holt, and de la Brosse Citation2019). Recently, a closer connection to populist movements that also present themselves as an alternative to established political parties was asserted (Fawzi Citation2019).
Assessing the extent of alternative news sources is difficult. Drawing the line on an outlet level has been shown to be problematic, as perceptions of what alternative news sources are, can differ strongly (Holt, Ustad Figenschou, and Frischlich Citation2019; Steppat, Herrero, and Esser Citation2020). Recent research has shown that when being asked what alternative news sources they follow, most respondents indeed mentioned non-mainstream but also mainstream sources, (Steppat, Castro, and Esser Citation2023). This shows that perceptions of societally accepted and unaccepted news sources have changed, with potentially problematic consequences. Hence, our study is interested in assessing the extent to which citizens perceive news exposure to be not accepted by others. Studying the frequency of behaviour that is perceived as unaccepted gives important insights into how much media use has become a divisive topic in society: The perception of being outside the mainstream news cycle can potentially have negative consequences on trust, press, and politics as attention shifts to news sources that publish heavily disputed content itself.
Media use is closely connected to an individual’s social identity (see Chan Citation2017) and citizens can gather strength from being part of an in-group that consumes different news sources than an out-group (Tajfel and Turner Citation1986). In a survey response situation, however, citizens potentially understand that their media use would be ‘unaccepted’ by most citizens (the out-group) and subsequently do not report openly about their sources. Compared to other types of news exposures, we can expect that asking citizens to report exposure behaviour to alternative media is subject to stronger social desirability biases (see Prior Citation2009) regardless of the survey mode. Hence, citizens who are aware that the outlets from which they consume news diverge from the majority’s opinion would not speak freely about this behaviour, making it an important context in which to study self-disclosure.
3. Hypotheses development
3.1. Chatbots enhancing self-disclosure of perceived unaccepted news exposure
The current study compares a chatbot to a traditional web-based survey due to the relative proximity as opposed to other assessment modes. Web-based formats are generally suited to foster self-disclosure and assess sensitive information, and are shown to be superior to face-to-face, or telephone methods because social desirability can be reduced (e.g. Henderson et al. Citation2012; Zhang et al., Citation2017). While these beneficial effects of an online environment are present for both, a chatbot, and traditional web-based survey, we argue that chatbots can enhance self-disclosure even further.
The non-human nature of a chatbot as a communication partner might be perceived as non-judgmental if recognised as a machine, referred to in the literature as a ‘machine-heuristic’ (Sundar and Kim Citation2019). Beliefs in a machine heuristic have been shown to significantly predict attitudes and behavioural intentions to use conversational technologies in domains that involve the discussion of sensitive topics (i.e. health; Gambino, Sundar, and Kim Citation2019). This can also explain previous findings of conversational technologies reducing psychological barriers to self-disclosure by providing a ‘safe’ environment for users to reveal personal information (Lucas et al. Citation2014).
In addition to exploiting the benefits of having a non-human appeal, a chatbot can show social characteristics that can help foster self-disclosure by providing comfort in the interaction (Pickard, Roster, and Chen Citation2016). Several scholars could show that the conversational and social characteristics of a chatbot can facilitate user engagement, favourable assessments (Crutzen et al. Citation2011; Fitzpatrick, Darcy, and Vierhile Citation2017), and self-disclosure of personal information (Ischen et al. Citation2020b; Sah and Peng Citation2015). Since the potential of chatbots for self-disclosure has been shown in several domains (mainly in the health context), we hypothesise that this effect also holds in a political news context, i.e. the self-disclosure of unaccepted news exposure behaviour, and propose the following:
H1. Citizens self-disclose perceived unaccepted news exposure behavior more often to a chatbot compared with a traditional, web-based survey.
3.2. Underlying mechanism explaining enhanced self-disclosure towards a chatbot
In addition to studying the overall potential of chatbots for self-disclosure in a political news context, the current research seeks to understand why people might be more inclined to self-disclose information to a chatbot. Including concepts related to the social characteristics of a chatbot, we argue for three underlying mechanisms explaining why users are more likely to self-disclose sensitive information to a chatbot than to a traditional, web-based survey. Previous research has shown that social presence (Chaves and Gerosa Citation2021; Ciechanowski et al. Citation2019), perceived intimacy (Croes and Antheunis Citation2021), and enjoyment (Lee and Choi Citation2017) are related to self-disclosure in the context of chatbots.
3.2.1. Social presence
Social presence has initially been conceptualised as a psychological variable that reflects closeness in a mediated communication interaction. As a property of a medium it describes the ‘degree of salience of the other people in the interaction’ (Short, Williams, and Christie Citation1976, 65). For this research, we define social presence, in reference to Lee (Citation2004) and prevalent in human-machine communication, as a psychological state in which chatbots are ‘experienced as actual social actors’ (45) and in which users fail to notice the role of technology. In other words, users feel together with a chatbot in the communication environment (Lee Citation2004; Xu and Lombard Citation2017).
Previous research has shown that social presence is an important variable in the context of chatbots, demonstrating that chatbots imbuing social or human-like cues are perceived as higher in social presence than chatbots not presenting these cues (Araujo Citation2018; Go and Sundar Citation2019). Recent research comparing different survey chatbots shows that a human-like chatbot leads to higher social presence, and higher self-disclosure compared to a baseline chatbot (Rhim et al. Citation2022). We argue that social presence is also higher for a chatbot than for a web-based survey, since the chatbot can act as a communication partner and deliver social interactions through its dialogic properties (Guzman Citation2019).
Research on source orientation has demonstrated that users orient towards conversational technology as a communication partner, instead of thinking they communicate with a human through the technology (Guzman Citation2019). If users perceive a chatbot as their communication partner (and perceive it as socially present), this might in turn foster self-disclosure. By being socially present, chatbots can provide a comfortable environment for users to reveal information about themselves similar to interpersonal communication, at the same time emphasising their non-human nature as the technology users communicate with it (Pickard, Roster, and Chen Citation2016).
3.2.2. Perceived intimacy
Intimacy can be described as an affective reaction towards a communication partner (Edinger and Patterson Citation1983). Previous research has suggested that perceived intimacy may play a significant role in the development of the relationship with a chatbot as a communication partner (Croes and Antheunis Citation2021). We argue that by showing a dialogical character and displaying social or human-like cues, intimacy in an interaction can be enhanced. Chatbots as social actors allow for a more intimate relationship development and can take for example the role of a companion (Gronau et al. Citation2020), thus feeling more personal than filling in a traditional survey. Initial qualitative results by Kim and colleagues (Citation2019) show that respondents indeed perceived intimacy with a chatbot using a more casual conversational style.
Previous research from computer-mediated communication showed perceptions of intimacy leading users to reveal more intimate details about themselves (Jiang, Bazarova, and Hancock Citation2013). Furthermore, by seeing a chatbot as a communication partner, we can utilise theories from interpersonal communication to explain the underlying mechanism. An important theory from human-to-human relationships is that of social penetration by Altman and Taylor (Citation1973), which states that relationships do not remain the same throughout a timespan but rather have distinctive phases. When a relationship moves from a shallow level to a more personal one, it is also more likely that intimate thoughts and details about one’s life are revealed to the interaction partner, both in breadth (the variety of topics that are addressed) and depth (the amount of information provided; Derlega and Chaikin Citation1977). Thus, the more intimate someone feels with another social actor, the more likely the person is to self-disclose.
3.2.3. Enjoyment
Lastly, we examine enjoyment as an underlying mechanism, defined as perceiving an interaction with a medium or communication partner as enjoyable in its own right (Carrol and Thoma Citation1988; Hassanein and Head Citation2007). Several studies could show that interacting with a chatbot can be an enjoyable experience (Kim, Lee, and Gweon Citation2019; Lee et al. Citation2020; Skjuve et al. Citation2021), and leads to more enjoyment than receiving information from a website (Ischen et al. Citation2020a). The dialogical interaction provided by a chatbot can lead to higher engagement that can in turn encourage participants to self-disclose personal information (Xiao et al. Citation2020).
In sum, we propose social presence, intimacy, and enjoyment as being underlying mechanisms that explain self-disclosure towards a chatbot and hypothesise the following:
H2. Chatbot modality leads to higher citizens’ self-disclosure of perceived unaccepted news exposure behavior compared to web-based survey modality, positively mediated by (a) perceptions of social presence, (b) perceived intimacy, and (c) enjoyment.
3.3. Longitudinal dynamics of self-disclosure towards a chatbot
Self-disclosure is an important variable in relationship building over time (Ho, Hancock, and Miner Citation2018). Skjuve and colleagues (Citation2021), for example, found that a human-to-chatbot relationship to be developed over time where self-disclosure increased as time progressed and trust in the chatbot as a conversational partner increased. Through the establishment of a trusting relationship over time, conversations deepened and people self-disclosed more, irrespective of whether the chatbot self-disclosed itself. To extend research conducted on the longitudinal dynamics of the relationship-building with chatbots (Croes and Antheunis Citation2021; Lee et al. Citation2020), we are interested in the trajectories of self-disclosure over time, and pose the following research question:
RQ1. Does the self-disclosure of perceived unaccepted news exposure behavior to a chatbot compared to a traditional, web-based survey show different trajectories over time?
4. Method
4.1. Experimental design of the study
This study is part of a larger data collection that aims to answer substantial and methodological questions, implementing a longitudinal, diary-style split-method experimental research design with two experimental conditions. The study was approved by the Ethical Review Board of the University of Amsterdam and was pre-registered with the open science framework.Footnote1 Data was collected between November and December 2020 via a professional polling company (Panel Inzicht), where all participants were part of a research panel. Criteria for a participation invitation were being adult (i.e. over 18 years), owning a smartphone, and having (or being willing to set up) a Skype account. To reflect in part the online population of the Netherlands, light quotas were added ensuring variance in terms of gender and age, thus participants were not specifically sought to be consumers of unaccepted (alternative) media.
In both conditions, participants were invited to answer daily questionnaires over a period of fourteen days. In the chatbot-based questionnaire, participants were invited to answer daily questionnaires through conversations with a chatbot via Skype. The second condition involved a traditional, web-based survey, in which participants were asked to respond to daily questionnaires using the Qualtrics platform.
Before being randomly assigned to one of the two conditions, participants completed a recruitment questionnaire in Qualtrics including demographical and control variables (for the overall project). Afterwards, participants received invitations to daily surveys, based on their individual response pattern. These daily questionnaires were completed either via Skype (in the chatbot condition) or via Qualtrics (as part of the web-based survey condition).
While simultaneously ensuring ecological validity of both conditions, the design of the chatbot and the web survey strove to keep conditions as similar as possible. Therefore, participants only interacted with the chatbot via text in the standard Skype instant messenger chat window with a rule-based set-up (see ). The questions were asked in different three configurations: (1) open-ended questions, where participants answered in text, (2) closed-ended questions with text options, where participants answered in text using one of the pre-defined options, and (3) closed-ended questions with numerical options, where the answer provided by participants was a number. Participants could miss two subsequent daily surveys if they responded on the third subsequent day. If they however did not respond to the invitations of three subsequent daily surveys, they were no longer invited and received a debrief survey, being dismissed from sample early. Hence, participants could respond to a maximum of fourteen, and a minimum of five daily surveys, without being dismissed from the sample early. After fourteen days, all remaining participants received a debrief survey.
4.2. Sample
Of 304 participants who completed the recruitment survey, 236 (77%) completed at least one daily survey. The average retention rate across field time was 68%, varying from 93% to 25% per day. On average, the 304 participants answered 7.6 daily surveys; 16 participants participated only once, while 58 participants participated on all fourteen days. For this study, only participants with at least two measurement points for unaccepted news exposure were included in the further assessment, resulting in 193 participants with a mean age of 47 (SD = 16.44) of which 46% reported to be female.
4.3. Measurements
The dependent variable in this study was perceived unaccepted news exposure (PUNE), which was used as a proxy for self-disclosure. This variable was measured every second day of the fourteen-day survey period in total seven times (day 2, 4, 6, 8, 10, 12 and 14). The variable was assessed through a single-item question asking: ‘People can have different tastes in news. Did you consume information from news sources today that you wouldn’t tell everybody about?’. A summative index of daily responses was calculated, which was divided by the days of responses.
Social presence, perceived intimacy and enjoyment were measured once in the debrief survey. All three mediators asked the participant if they agreed or disagreed with a series of statements. All the items were assessed on a seven-point Likert-scale with 1 being ‘strongly disagree’ and 7 being ‘strongly agree’. Social presence, adapted from Gefen and Straub (Citation2004), was measured with five items, e.g. ‘There was a sense of human contact in the chatbot [online] surveys’. Perceived intimacy was adapted from Croes and Antheunis (Citation2021) with three items, e.g. ‘I experienced the interaction with the chatbot [online] surveys as intimate’. Enjoyment was adapted from Bosnjak, Metzger, and Gräf (Citation2010) with three items, e.g. ‘It was fun for me to fill out the questions in the chatbot [online] surveys’.
5. Results
5.1. Self-disclose of unaccepted news exposure behaviour
To test the first hypothesis of whether citizens self-disclose unaccepted news exposure behaviour more often to a conversational chatbot compared to a traditional, web-based survey, we first calculated a relative measurement of unaccepted news exposure on an individual data level. Participants were asked every second day whether they were exposed to news sources that they would not tell anyone about, resulting in seven measurements. However, not all participants answered the question on all the days they were asked this question. To receive a reliable estimate, we excluded participants who answered this question less than twice. To account for this variation in participation and to make data comparable, we calculated a relative exposure measurement on an individual data level. The number of unaccepted news exposures reported was divided by the number of days each participant had answered the survey. The relative measure ranged from 0 to 1 on a continuous level, with 1 indicating unaccepted news exposure reported on all days of participation.
We conducted an independent sample t-test with this unaccepted news exposure measurement as the dependent variable and condition (chatbot vs. web-based survey) as the independent variable. Participants in the chatbot condition (M = 0.36, SD = 0.32) indicated significantly higher levels of perceived unaccepted news exposure than participants in the web-based survey condition (M = 0.15, SD = 0.27), t(384) = −5.75, p < .001. This supports H1, participants self-disclosed unaccepted news exposure behaviour more often to a conversational chatbot compared to a traditional, web-based survey.
5.2. Mediation
To test the mediation effects of social presence, perceived intimacy, and enjoyment on perceived unaccepted news exposure, we used the mediation package in R (Tingeley et al. Citation2019). We calculated three different models with unaccepted news exposure as the dependent, condition (chatbot vs. web-based survey) as the independent and (a) social presence, (b) perceived intimacy, and (c) enjoyment as the mediator. Means and standard deviations are shown in and the indirect effect (average causal mediation effect i.e. ACME), direct effect (average direct effects i.e. ADE) and total effect are depicted in .
Figure 2. Mediation effects of social presence, perceived intimacy, and enjoyment. Notes: ACME = Average causal mediation effect (indirect effect); ADE = average direct effect.
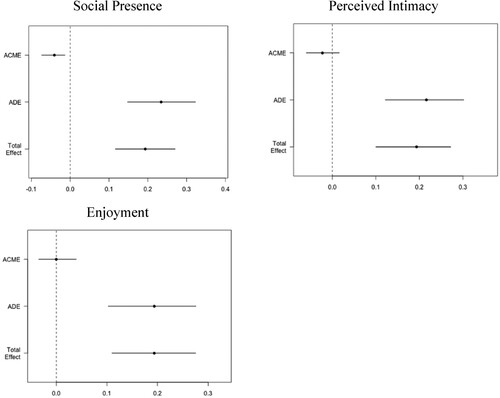
Table 1. Overall means and standard deviations of the variables of interest.
5.2.1. Social presence
The total effect of condition on perceived unaccepted news exposure was significant (B = 0.21, SE = 0.04, t(191) = 4.94, p < .001), and so was the direct effect (B = 0.23, SE = 0.05, t(183) = 5.20, p < .001). The direct effect of condition on the mediator social presence was also significant (B = −1.30, SE = 0.23, t(184) = −5.55, p < .001), and so was the direct effect of the mediator social presence on unaccepted news exposure (B = 0.03, SE = 0.01, t(183) = 1.39, p = .018). We calculated the unstandardised indirect effects for each of 1000 bootstrapped samples, and the 95% confidence interval by determining the indirect effects at the 2.5th and 97.5th percentiles. The effect of condition on unaccepted news exposure was mediated by social presence, the indirect effect was significant (B = −.04, CI = [−0.07, −0.01], p = .004). This means that taking the survey in the chatbot condition resulted in lower social presence compared to the traditional web-based survey, which negatively affected reported unaccepted news exposure. In other words, although unaccepted news exposure is higher in the chatbot condition (vs. web-based survey), lower social presence tempered this difference. H2a is therefore not supported.
5.2.2. Perceived intimacy
The total effect of condition on perceived unaccepted news exposure was significant (B = 0.21, SE = 0.04, t(191) = 4.94, p < .001), and so was the direct effect (B = 0.22, SE = 0.05, t(183) = 4.55, p < .001). The direct effect of condition on the mediator perceived intimacy was also significant (B = −1.33, SE = 0.19, t(184) = −6.98, p < .001), but the direct effect of the mediator perceived intimacy on unaccepted news exposure was not significant (B = 0.02, SE = 0.02, t(183) = 1.04, p = .200). We again calculated the unstandardised indirect effects for each of 1000 bootstrapped samples, and the 95% confidence interval. The effect of condition on unaccepted news exposure was not mediated by perceived intimacy, the indirect effect was not significant (B = -.12, CI = [−0.38, 0.09], p = .230). H2b is not supported.
5.2.3. Enjoyment
The total effect of condition on perceived unaccepted news exposure was significant (B = 0.21, SE = 0.04, t(191) = 4.94, p < .001), and so was the direct effect (B = 0.19, SE = 0.05, t(183) = 4.24, p < .001). The direct effect of condition on the mediator perceived intimacy was also significant (B = −0.91, SE = 0.17, t(184) = −5.53, p < .001), but the direct effect of the mediator perceived intimacy on unaccepted news exposure was not significant (B = 0.00, SE = 0.02, t(183) = 0.01, p = .995). We again calculated the unstandardised indirect effects for each of 1000 bootstrapped samples, and the 95% confidence interval. The effect of condition on unaccepted news exposure was not mediated by enjoyment, the indirect effect was not significant (B = -.00, CI = [−0.21, 0.23], p = .970). H2c is not supported.
5.3. Trajectories over time
To test the research question of whether the self-disclosure of unaccepted news exposure behaviour to a conversational chatbot compared to a traditional, web-based survey shows different trajectories over time, we computed a linear mixed-effects model using the R package lme4 (Bates et al. Citation2015). We hereby treated unaccepted news exposure as nested per participant, instead of relying on one relative measurement on an individual level.
Mixed-effects models allow for dependencies in repeated measures data. While treating participants as nested random factors, condition was included as a fixed factor explaining differences in perceived unaccepted news exposure. In a first step (model 1), we specified a random intercept model, in which participants were entered as a random factor to control for their associated intraclass correlation. In a second step (model 2), we included the three predictor variables: condition, participation day, and since we expect a dependency among the unaccepted news exposure variable, we also include a lagged dependent variable as an additional predictor. In the last step (model 3), an interaction term of condition (web-based survey = 0, chatbot = 1) and participation day was added. The intraclass correlation coefficients (ICC) for both models were moderately high (ICCmodel1 = 0.38, ICCmodel2 = 0.36, ICCmodel3 = 0.37), indicating that a significant amount of variance can be explained within subjects, while a bigger part is left unexplained.
Regression results are presented in . Regression results of the full model (model 3) show that unaccepted news exposure at t-1 does not predict unaccepted news exposure at t. This means that previous unaccepted news exposure as such does not predict current unaccepted news exposure. Hence, unaccepted news exposure is not a constant occurrence among participants but most likely dependent on daily news experiences. Moreover, results show that condition is a significant predictor of perceptions of unaccepted news exposure, supporting our results found for H1. The chatbot condition (in comparison to the web-based survey condition) did in fact lead to a higher reporting of unaccepted news exposure.
Table 2. Mixed-effects regression of unaccepted news exposure (fixed effects).
Day of participation is a significant negative predictor of reporting of unaccepted news exposure, and the interaction effect is also significant. These results indicate that later in the study there were significantly less instances of unaccepted news exposure reported. This in turn points to a habituation effect. The significantly negative interaction effect of condition and day shows that the chatbot condition over the study period has a stronger impact on reported unaccepted news exposure than the web-based survey condition over the same period. It is thus stipulated that though over time the negative slope for reported unaccepted news exposure is bigger in the chatbot condition than in the web-based survey condition. This means that while unaccepted news exposure reports decrease in both conditions over time, this type of self-disclosure becomes even less likely in the chatbot condition. Hence, we cannot observe that continuous interaction with a chatbot increases chances of self-disclosure of behaviour that might be perceived as unaccepted by participants. The overall means of PUNE over time in the two conditions are presented in .
6. Discussion
Our work has three main implications which we will discuss in the following. First, we find that people disclose unaccepted news exposure significantly more often to a chatbot, compared to a traditional web-based survey. This adds to previous research (Y.-C. Lee et al. Citation2020; Lucas et al. Citation2014; Pickard, Roster, and Chen Citation2016) showing that the use of conversational technologies such as chatbots can increase self-disclosure in a political news context. Based on our data, we cannot say which mode – chatbot or web-based survey – is better for assessing actual unaccepted news exposure. Yet, given that such exposure is not willingly expressed by everyone, the fact that self-disclosure is higher in the chatbot condition means that chatbots should be considered helpful to encourage users to reveal information about more sensitive topics. Researchers and practitioners can benefit from this finding, as chatbots can overall be seen as having the potential (or at least not performing worse) than online surveys when it comes to self-disclosure.
Second, based on previous research, our study tests potential explanations for differences of self-disclosure. Interestingly, we do not find that social presence, intimacy, and enjoyment of taking the survey are perceived as higher in a chatbot interaction. While previous research has suggested that these mechanisms can be useful for self-disclosure (Jiang, Bazarova, and Hancock Citation2013; Pickard, Roster, and Chen Citation2016) we find no or even the opposite effect: the perceived lower social presence slightly but significantly inhibits self-disclosure towards a chatbot (in comparison to a web-based survey). Some previous research found similar seemingly counterintuitive results, for example, a chatbot being perceived as less human-like than a website (Ischen et al. Citation2020a). One explanation for these findings might be the level of social cues. Previous research has indeed shown that manipulating the interactive features such as the anthropomorphic design (i.e. the chatbot has a name and uses dialogue commonly used in human communication) can have effects on the emotional bond a respondent has with a chatbot (Skjuve et al. Citation2021) and that anthropomorphic linguistic cues (i.e. conversational language) can foster self-disclosure (Sah and Peng Citation2015). The chatbot as used in the current research did not have any particular anthropomorphic features. Using a relatively simply conversational interface might thus not be enough to evoke the social benefits that can enhance self-disclosure.
Third, we tested whether repeated interaction will likely build a relationship with a chatbot that makes it easier to disclose sensitive behaviour. While this is often assumed, this is one of the first studies that investigates effects of a chatbot on self-disclosure over time. Our results do not provide evidence that an extended duration of interaction, over several days or even weeks, increases the level of self-reported unaccepted news exposure behaviour. Furthermore, while the chatbot led to higher self-disclosure compared to the web-based survey at all time points, we do find that a longer duration of taking a survey with a chatbot decreased the chance of self-disclosure. Potential reasons for this are that the increased duration of engagement may showcase the repetitive nature of the chatbot conversation that becomes increasingly similar to answering a static web-based survey and thereby approach similar levels of self-disclosure over time. We could thus argue for a habituation effect in both modes.
7. Conclusion
Conversational technologies such as chatbots can be social communication partners, and at the same time have technological benefits such as being (perceived as) non-judgemental in computer-aided interactions. Hence, there is a growing interest in implementing chatbots in domains in which users are sharing personal information about themselves. This study investigated the potential of a chatbot (in comparison to a web-based survey) to elicit self-disclosure in a political news context, i.e. the exposure to news that might be perceived as unaccented by others. Furthermore, it investigated three underlying mechanisms (i.e. social presence, enjoyment, intimacy) that can explain differences in self-disclosure, and examined trajectories over time. In summary, we find that overall self-disclosure was higher for a chatbot compared to a web-based survey, however, social presence, enjoyment, and intimacy cannot explain this effect, and that self-disclosure generally decreases over time.
We identify two limitations that can provide suggestions for future research. Firstly, our study only focuses on social perceptions as underlying mechanisms. The finding that self-disclosure in the chatbot condition is higher but cannot be explained by the factors (i.e. social presence, enjoyment, intimacy) tested leaves us with a puzzle: What is it that, despite random assignment, leads citizens to higher self-disclosure with a chatbot, especially at the beginning of the study? One possibility might be the difference in functionality between chatbot and web-based survey. An important feature of chatbots is the direct interaction with users, which may even be perceived as reciprocal by some. The mere fact that typing a response causes a reaction may indeed be enough for respondents to self-disclose to a greater extent, than if they were filling in a matrix grid question in an online survey. This, however, would mean that the social cues of a chatbot are much less important than the mere functionality of a chatbot, e.g. providing fast responses, or possibly the novelty of such an interaction. To address these and related questions, future research should further investigate the underlying mechanisms of self-disclosure in survey research, particularly focusing on the utilitarian perceptions of chatbots, e.g. perceived efficiency (which has, for example, been investigated in the context of online avatars; Etemad-Sajadi Citation2014).
A second limitation of this study is related to the longitudinal setup of this study. The design of our chatbot followed a pre-defined script, which could lead to perceptions of repetition over time. This can potentially explain the decrease in self-disclosure over time. Future research should take this possible explanation into account by having a specific focus on engaging users in chatbot interactions. Another reason for the decrease of self-disclosure towards chatbot as well as web-based survey might be that once disclosed, participants might have not deemed it necessary to repeatedly mention an additional exposure in the subsequent questionnaire. Future research should take these possible explanations into account by having a specific focus on engaging users. The methodological advancement of questionnaires used for repeated measurements in the context of chatbots would hence be a useful endeavour for future research.
Concluding, even though further research about the underlying mechanisms and trajectories over time is needed, we can show that implementing a chatbot can be a promising way to elicit self-disclosure of behaviours that might be perceived as unaccepted by others. This applies to media usage behaviours, such as the exposure to (alternative) media sources, but also has wider implications for the implementation of chatbots in domains that involve sensitive questions, not only regarding health (e.g. unsafe sexual behaviour, see Crutzen et al. Citation2011), but also the political (e.g. non-voting) context.
Disclosure statement
No potential conflict of interest was reported by the author(s).
Data availability statement:
The data that support the findings of this study are openly available in Chatbots as Data Collection Modes at https://osf.io/ws6ax/.
Additional information
Funding
Notes
1 https://osf.io/wcjgz?view_only=ff738bb2f35e47f0a73329f75d8827a6; for further details on the method see also (Zarouali et al. Citation2023)
References
- Altman, I., and D. A. Taylor. 1973. Social Penetration: The Development of Interpersonal Relationships. Holt: Rinehart & Winston.
- Araujo, T. 2018. “Living up to the Chatbot Hype: The Influence of Anthropomorphic Design Cues and Communicative Agency Framing on Conversational Agent and Company Perceptions.” Computers in Human Behavior 85: 183–189. https://doi.org/10.1016/j.chb.2018.03.051.
- Archer, R. L., and J. A. Burleson. 1980. “The Effects of Timing of Self-disclosure on Attraction and Reciprocity.” Journal of Personality and Social Psychology 38 (1): 120. https://doi.org/10.1037/0022-3514.38.1.120
- Atkinson, J. D., and L. J. Kenix, eds. 2019. Alternative Media Meets Mainstream Politics: Activist Nation Rising. London: Lexington Books.
- Bates, D., M. Mächler, B. M. Bolker, and S. C. Walker. 2015. “Fitting linear mixed-effects models using lme4.” Journal of Statistical Software 67 (1), https://doi.org/10.18637/jss.v067.i01.
- Bennett, W. L., and S. Livingston. 2018. “The Disinformation Order: Disruptive Communication and the Decline of Democratic Institutions.” European Journal of Communication 33 (2): 122–139. https://doi.org/10.1177/0267323118760317.
- Bosnjak, M., G. Metzger, and L. Gräf. 2010. “Understanding the Willingness to Participate in Mobile Surveys: Exploring the Role of Utilitarian, Affective, Hedonic, Social, Self-Expressive, and Trust-Related Factors.” Social Science Computer Review 28 (3): 350–370. https://doi.org/10.1177/0894439309353395.
- Carrol, J. N., and J. C. Thoma. 1988. “Fun.” ACM SIGCHI Bulletin 19 (3): 21–24. https://doi.org/10.1145/49108.1045604.
- Chan, M. 2017. “Media Use and the Social Identity Model of Collective Action: Examining the Roles of Online Alternative News and Social Media News.” Journalism & Mass Communication Quarterly 94 (3): 663–681. https://doi.org/10.1177/1077699016638837.
- Chaves, A. P., and M. A. Gerosa. 2021. “How Should My Chatbot Interact? A Survey on Social Characteristics in human–Chatbot Interaction Design.” International Journal of Human–Computer Interaction 37 (8): 729–758. https://doi.org/10.1080/10447318.2020.1841438.
- Ciechanowski, L., A. Przegalinska, M. Magnuski, and P. Gloor. 2019. “In the Shades of the Uncanny Valley: An Experimental Study of Human–Chatbot Interaction.” Future Generation Computer Systems 92: 539–548. https://doi.org/10.1016/j.future.2018.01.055.
- Croes, E. A. J., and M. L. Antheunis. 2021. “Can we be Friends with Mitsuku? A Longitudinal Study on the Process of Relationship Formation Between Humans and a Social Chatbot.” Journal of Social and Personal Relationships, 279–300. https://doi.org/10.1177/0265407520959463.
- Crutzen, R., G. J. Y. Peters, S. D. Portugal, E. M. Fisser, and J. J. Grolleman. 2011. “An Artificially Intelligent Chat Agent That Answers Adolescents’ Questions Related to Sex, Drugs, and Alcohol: An Exploratory Study.” Journal of Adolescent Health 48 (5): 514–519. https://doi.org/10.1016/j.jadohealth.2010.09.002.
- Derlega, V. J., and A. L. Chaikin. 1977. “Privacy and Self-Disclosure in Social Relationships.” Journal of Social Issues 33 (3). https://doi.org/10.1111/j.1540-4560.1977.tb01885.x
- Edinger, J. A., and M. L. Patterson. 1983. “Nonverbal Involvement and Social Control.” Psychological Bulletin 93 (1): 30–56. https://doi.org/10.1037/0033-2909.93.1.30.
- Etemad-Sajadi, R. 2014. “The Influence of a Virtual Agent on web-Users' Desire to Visit the Company.” International Journal of Quality & Reliability Management 31 (4): 419–434. https://doi.org/10.1108/IJQRM-05-2013-0077.
- Fawzi, N. 2019. “Untrustworthy News and the Media as ‘Enemy of the People?’ How a Populist Worldview Shapes Recipients’ Attitudes Toward the Media.” The International Journal of Press/Politics 24 (2): 146–164. https://doi.org/10.1177/1940161218811981.
- Fög - Forschungsinstitut Öffentlichkeit und Gesellschaft. (2017). Jahrbuch 2017 Qualität der Medien F.-F. Ö. und Gesellschaft ed. Basel: Schwabe Verlag.
- Fitzpatrick, K. K., A. Darcy, and M. Vierhile. 2017. “Delivering Cognitive Behavior Therapy to Young Adults With Symptoms of Depression and Anxiety Using a Fully Automated Conversational Agent (Woebot): A Randomized Controlled Trial.” JMIR Mental Health 4 (2), https://doi.org/10.2196/mental.7785.
- Gambino, A., S. S. Sundar, and J. Kim. 2019. “Digital Doctors and Robot Receptionists.” In Conference on Human Factors in Computing Systems - Proceedings, 1–6.
- Gefen, D., and D. W. Straub. 2004. “Consumer Trust in B2C e-Commerce and the Importance of Social Presence: Experiments in e-Products and e-Services.” Omega 32 (6): 407–424. https://doi.org/10.1016/j.omega.2004.01.006.
- Go, E., and S. S. Sundar. 2019. “Humanizing Chatbots: The Effects of Visual, Identity and Conversational Cues on Humanness Perceptions.” Computers in Human Behavior, https://doi.org/10.1016/j.chb.2019.01.020.
- Gronau, N., M. Heine, K. Poustcchi, H. Krasnova, N. Zierau, C. Engel, M. Söllner, and J. M. Leimeister. 2020. “WI2020 Zentrale Tracks.” WI2020 Zentrale Tracks, 99–114. https://doi.org/10.30844/wi_2020_a7-zierau.
- Guzman, A. L. 2019. “Voices in and of the Machine: Source Orientation Toward Mobile Virtual Assistants.” Computers in Human Behavior 90: 343–350. https://doi.org/10.1016/j.chb.2018.08.009.
- Haller, A., K. Holt, and R. de la Brosse. 2019. “The ‘Other’ Alternatives: Political Right-Wing Alternative Media.” Journal of Alternative & Community Media 4 (1): 1–6. https://doi.org/10.1386/joacm_00039_2.
- Hassanein, K., and M. Head. 2007. “Manipulating Perceived Social Presence Through the Web Interface and its Impact on Attitude Towards Online Shopping.” International Journal of Human-Computer Studies 65 (8): 689–708. https://doi.org/10.1016/j.ijhcs.2006.11.018.
- Henderson, C., S. Evans-Lacko, C. Flach, and G. Thornicroft. 2012. “Responses to Mental Health Stigma Questions: The Importance of Social Desirability and Data Collection Method.” The Canadian Journal of Psychiatry 57 (3): 152–160. https://doi.org/10.1177/070674371205700304.
- Hettena, S. 2019. “The Obscure Newspaper Fueling the Far-Right in Europe.” The New Republic. https://newrepublic.com/article/155076/obscure-newspaper-fueling-far-right-europe.
- Ho, A., J. Hancock, and A. S. Miner. 2018. “Psychological, Relational, and Emotional Effects of Self-Disclosure After Conversations With a Chatbot.” Journal of Communication 68 (4): 712–733. https://doi.org/10.1093/joc/jqy026.
- Holt, K., T. Ustad Figenschou, and L. Frischlich. 2019. “Key Dimensions of Alternative News Media.” Digital Journalism 7 (7): 860–869. https://doi.org/10.1080/21670811.2019.1625715.
- Ischen, C., T. Araujo, G. van Noort, H. Voorveld, and E. Smit. 2020a. “‘I Am Here to Assist You Today’: The Role of Entity, Interactivity and Experiential Perceptions in Chatbot Persuasion.” Journal of Broadcasting & Electronic Media 64 (4): 615–639. https://doi.org/10.1080/08838151.2020.1834297.
- Ischen, C., T. Araujo, H. Voorveld, G. van Noort, and E. Smit. 2020b. “Privacy Concerns in Chatbot Interactions.” In Chatbot Research and Design: Third International Workshop, CONVERSATIONS 2019, Amsterdam, The Netherlands, November 19–20, 2019. Lecture Notes in Computer Science; Vol. 11970, edited by A. Følstad, T. Araujo, S. Papadopoulos, EL-C. Law, O-C. Granmo, E. Luger, and P. B. Brandtzaeg, 34–48. Springer. https://doi.org/10.1007/978-3-030-39540-7_3.
- Jiang, L. C., N. N. Bazarova, and J. T. Hancock. 2013. “From Perception to Behavior.” Communication Research 40 (1): 125–143. https://doi.org/10.1177/0093650211405313.
- Kim, S., J. Lee, and G. Gweon. 2019. “Comparing Data from Chatbot and Web Surveys.” In Conference on Human Factors in Computing Systems - Proceedings, 1–12.
- Laranjo, L., A. G. Dunn, H. L. Tong, A. B. Kocaballi, J. Chen, R. Bashir, D. Surian, et al. 2018. “Conversational Agents in Healthcare: A Systematic Review.” Journal of the American Medical Informatics Association 25 (9): 1248–1258. https://doi.org/10.1093/jamia/ocy072.
- Lee, K. M. 2004. “Presence, Explicated.” Communication Theory 14 (1): 27–50. https://doi.org/10.1111/j.1468-2885.2004.tb00302.x.
- Lee, S. Y., and J. Choi. 2017. “Enhancing User Experience with Conversational Agent for Movie Recommendation: Effects of Self-Disclosure and Reciprocity.” International Journal of Human-Computer Studies 103 (January): 95–105. https://doi.org/10.1016/j.ijhcs.2017.02.005.
- Lee, Y.-C., N. Yamashita, Y. Huang, and W. Fu. 2020. “‘I Hear You, I Feel You’: Encouraging Deep Self-Disclosure Through a Chatbot.” In Proceedings of the 2020 CHI Conference on Human Factors in Computing Systems, 1–12.
- Levy, D. A. L., N. Newman, R. Fletcher, A. Kalogeropoulos, and R. K. Nielsen. 2018. Reuters Institute Digital News Report 2018.
- Lucas, G. M., J. Gratch, A. King, and L. P. Morency. 2014. “It’s Only a Computer: Virtual Humans Increase Willingness to Disclose.” Computers in Human Behavior 37: 94–100. https://doi.org/10.1016/j.chb.2014.04.043.
- Maniou, T. A., and A. Veglis. 2020. “Employing a Chatbot for News Dissemination During Crisis: Design, Implementation and Evaluation.” Future Internet 12 (12): 109–114. https://doi.org/10.3390/fi12070109.
- Newman, N., R. Fletcher, A. Schulz, S. Andi, C. T. Robertson, and R. K. Nielsen. 2021. “Reuters Institute Digital News Report 2021.” https://www.digitalnewsreport.org/.
- Pickard, M. D., C. A. Roster, and Y. Chen. 2016. “Revealing Sensitive Information in Personal Interviews: Is Self-Disclosure Easier with Humans or Avatars and Under What Conditions?” Computers in Human Behavior 65: 23–30. https://doi.org/10.1016/j.chb.2016.08.004.
- Prior, M. 2009. “The Immensely Inflated News Audience: Assessing Bias in Self-Reported News Exposure.” Public Opinion Quarterly 73 (1): 130–143. https://doi.org/10.1093/poq/nfp002.
- Rhim, J., M. Kwak, Y. Gong, and G. Gweon. 2022. “Application of Humanization to Survey Chatbots: Change in Chatbot Perception, Interaction Experience, and Survey Data Quality.” Computers in Human Behavior 126: 107034. https://doi.org/10.1016/j.chb.2021.107034.
- Sah, Y. J., and W. Peng. 2015. “Effects of Visual and Linguistic Anthropomorphic Cues on Social Perception, Self-Awareness, and Information Disclosure in a Health Website.” Computers in Human Behavior 45: 392–401. https://doi.org/10.1016/j.chb.2014.12.055.
- Schmidt, T. O., and R. R. Cornelius. 1987. “Self-disclosure in Everyday Life.” Journal of Social and Personal Relationships 4 (3): 365–373. https://doi.org/10.1177/026540758700400307.
- Schulz, A. 2019. “Where Populist Citizens get the News: An Investigation of News Audience Polarization Along Populist Attitudes in 11 Countries.” Communication Monographs 86 (1): 88–111. https://doi.org/10.1080/03637751.2018.1508876.
- Shin, D. 2022. “The Perception of Humanness in Conversational Journalism: An Algorithmic Information-Processing Perspective.” New Media & Society 24 (12): 2680–2704. https://doi.org/10.1177/1461444821993801.
- Shin, D., B. Zaid, F. Biocca, and A. Rasul. 2022. “In Platforms We Trust?Unlocking the Black-Box of News Algorithms Through Interpretable AI.” Journal of Broadcasting & Electronic Media 66 (2): 235–256. https://doi.org/10.1080/08838151.2022.2057984.
- Short, John, E. Williams, and B. Christie. 1976. The Social Psychology of Telecommunications. New York: John Wiley & Sons.
- Skjuve, M., A. Følstad, K. I. Fostervold, and P. B. Brandtzaeg. 2021. “My Chatbot Companion – A Study of Human-Chatbot Relationships.” International Journal of Human-Computer Studies 149), https://doi.org/10.1016/j.ijhcs.2021.102601.
- Skjuve, M., A. Følstad, K. I. Fostervold, and P. B. Brandtzaeg. 2022. “A Longitudinal Study of Human-Chatbot Relationships.” International Journal of Human Computer Studies 168 (102903): 1–14. https://doi.org/10.1016/j.ijhcs.2022.102903.
- Steppat, D., L. Castro, and F. Esser. 2023. “What News Users Perceive as ‘Alternative Media’ Varies Between Countries: How Media Fragmentation and Polarization Matter.” Digital Journalism 11 (5): 741–761. https://doi.org/10.1080/21670811.2021.1939747.
- Steppat, D., L. C. Herrero, and F. Esser. 2020. “News Media Performance Evaluated by National Audiences: How Media Environments and User Preferences Matter.” Media and Communication 8 (3): 321–334. https://doi.org/10.17645/mac.v8i3.3091.
- Sundar, S. S., and J. Kim. 2019. “Machine Heuristic.” In Proceedings of the 2019 CHI Conference on Human Factors in Computing Systems - CHI ‘19, 1–9.
- Tajfel, H., and J. C. Turner. 1986. “The Social Identity Theory of Intergroup Behavior.” In Psychology of Intergroup Relations, edited by S. Worchel, and W. G. Austin, 276–293. Nashville: Nelson-Hall.
- Tingeley, D., T. Yamanoto, K. Hirose, L. Keele, K. Imai, M. Trinh, and W. Wong. 2019. “Mediation: Causal Mediation Analysis (4.5.0).” https://cran.r-project.org/package=mediation.
- Westerman, D., A. C. Cross, and P. G. Lindmark. 2019. “I Believe in a Thing Called bot: Perceptions of the Humanness of “Chatbots”.” Communication Studies 70 (3): 295–312. https://doi.org/10.1080/10510974.2018.1557233.
- Xiao, Z., M. X. Zhou, Q. V. Liao, G. Mark, C. Chi, W. Chen, and H. Yang. 2020. “Tell Me About Yourself: Using an AI-Powered Chatbot to Conduct Conversational Surveys with Open-ended Questions.” ACM Transactions on Computer-Human Interaction (TOCHI) 27 (3), https://doi.org/10.1145/3381804.
- Xu, K., and M. Lombard. 2017. “Persuasive Computing: Feeling Peer Pressure from Multiple Computer Agents.” Computers in Human Behavior 74: 152–162. https://doi.org/10.1016/j.chb.2017.04.043.
- Yin, S. 2019. “Where Chatbots are Headed in 2020.” https://chatbotsmagazine.com/where-chatbots-are-headed-in-2020-4e4cbf281fc9.
- Zarouali, B., T. Araujo, J. Ohme, and C. de Vreese. 2023. “Comparing Chatbots and Online Surveys for (Longitudinal).” Data Collection: An Investigation of Response Characteristics, Data Quality, and User Evaluation. Communication Methods and Measures, 1–20. https://doi.org/10.1080/19312458.2022.2156489.
- Zhang, X. C., Kuchinke, L., Woud, M. L., Velten, J., & Margraf, J. (2017). “Survey Method Matters: Online/Offline Questionnaires and Face-to-Face or Telephone Interviews Differ.” Computers in Human Behavior, 71, 172–180. https://doi.org/10.1016/j.chb.2017.02.006