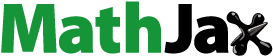
ABSTRACT
Online labor markets have gained significant importance in recent years, drawing considerable attention in academia and practice. These platforms enable workers worldwide to sell their labor services to a global pool of clients. However, the challenge lies in motivating workers effectively to enhance their productivity. To address this issue, we employ the self—determination theory and present a model that elucidates motivation's impact on productivity across various payment schemes. Additionally, we leverage the psychological trait theory and its suggested taxonomy to explore how compensation policies in online labor markets affect incentives differently based on individual differences. Our experiment tests predictions from a formal labor supply and productivity model for workers with varying compensation levels. The results indicate that intrinsic workers exhibit higher productivity when bonus rewards are introduced. Furthermore, our study confirms the presence of heterogeneous personality effects, emphasizing that increased worker productivity is primarily associated with conscientiousness and agreeableness traits. These findings illuminate the intricate mechanisms governing worker motivation and engagement in paid crowdsourcing environments. They provide valuable theoretical and managerial insights for researchers and crowdsourcing practitioners aiming to enhance worker productivity in online tasks.
1. Introduction
Online labour markets are rapidly growing means for companies and individuals to seek, find and hire workers with a particular skill set to complete a variety of online jobs (Randhawa, Wilden, and West Citation2019). These platforms that facilitate the connection between employers and workers to complete crowdsourcing tasks. Following this way, crowdsourcing communities can provide organisations with a cost-effective solution to exploit the ‘wisdom of crowds’ (Autor Citation2001; Howe Citation2008). This new concept of crowdsourcing, as an emerging IT innovation which promotes development more easily, efficiently and without boarder restrictions, attracts growing attention from scholars and practitioners (Mourelatos and Tzagarakis Citation2018; Ye and Kankanhalli Citation2015).
In fact, crowdsourcing workers possess the autonomy to choose tasks that are of interest to them and exert effort levels according to their discretion. Consequently, the efficacy of crowdsourcing is reliant upon optimal task design and high levels of public engagement to ensure quality outcomes (Li et al. Citation2022). As the online environment becomes increasingly saturated with a plethora of options for workers to allocate their limited resources, such as time, expertise, and skills, these resources are becoming scarcer for task requesters. Individuals’ involvement in crowdsourcing tasks is contingent and dynamic. Therefore, it is crucial to attract the participation of the crowd and incentivize their commitment to input additional resources (Chandler and Kapelner Citation2013). This requires a comprehensive comprehension of their motivation and an understanding of why people behave as they do, thus allowing organisations to create crowdsourcing tasks in a suitable and effective manner. This necessitates a holistic approach to designing crowdsourcing tasks that incorporates insights from motivational research to maximise engagement and output from participants. These factors have emerged as critical determinants of success in crowdsourcing endeavours.
Driven by that, our study focuses on online labour marketplaces, because they differ from their traditional counterparts in a few important aspects. A major difference is that the global reach of these platforms enables employers to access a larger pool of workers than before and enjoy a wider diversity of hiring choices (Brynjolfsson, Hu, and Smith Citation2003). Another notable difference lies in the offered wages. Because employers are uncertain ex ante whether the workers, they hire can offer high or low quality of work, economic theory predicts that employers are likely to offer wages between the wages of high-quality workers and those of low-quality workers. In turn, high quality workers are likely to exit the labour pool, causing wages and labour quality to spiral downwards (Farrell, Grenier, and Leiby Citation2017). In this rationale, the low compensation rates of some online labour markets reflect the low quality of work by the labour pool. Research has not yet addressed these fundamental issues and nowadays, there is a growing debate on the determinants of online productivity and its relationship with the offered reward (Horton and Chilton Citation2010; Hua et al. Citation2017; Syverson Citation2011). Also, purchasers of labour in these markets confront severe adverse selection issues – including uncertainty about workers’ effort and working behaviour. Thus, despite its success, this new form of ‘peer production’ faces several practical challenges in relation to workers behaviour (Beck et al. Citation2022).
To date, there are body of studies related to crowdsourcing workers’ incentives conceptually and empirically (Martinez Citation2017; Rogstadius et al. Citation2011), by analysing mainly survey data. These papers have primarily focused on examining the influence of perceived benefit on individuals’ motivation to participate and engage in crowdsourcing. Recent research, though, has also highlighted the interconnectedness of motivation and personality in influencing working behaviour. Pee, Koh, and Goh (Citation2018) and Badura et al. (Citation2020) have reported on findings that suggest a relationship between motivation and personality traits. Additionally, research by McIlroy et al. (Citation2015) and Donato et al. (Citation2017) have further emphasised the importance of personality in shaping working behaviour. Furthermore, Butschek et al. (Citation2022) have noted that the relationship between personality and motivation is well-established, with personality traits being a significant predictor of motivation. Yet there are few attempts that identify and experimentally link incentives with online productivity under difference payment schemes. This represents an important gap in the literature, as understanding the impact of incentives on productivity and how this may differ based on individual characteristics is critical for the effective management of crowdsourcing tasks.
Motivated by these knowledge gaps, this research aims, experimentally, to address the following questions: (i) How does the motivation of a worker drive online productivity? (ii) what are the differential effects of intrinsic and extrinsic incentives on online productivity under different payment schemes? (iii) To what extent does personality moderate the relationship between incentives and workers’ inputs in crowdsourcing tasks?
Drawing upon the self-determination theory, we put forth a conceptual framework to elucidate the impact of motivation on productivity under varying payment arrangements. Moreover, we leverage the psychological trait theory and its prescribed typology to infer whether remuneration policies in online labour markets differentially impact incentives based on individual differences. To directly examine these relationships, we used a pure productivity-computational task. Our paper is based on data drawn from an experiment designed in Amazon Mechanical Turk, which measures and assesses personality and motivation parameters to directly predict workers’ productivity under several performance-pay and fixed term wage scenarios (Goncalves et al. Citation2015; Rogstadius et al. Citation2011). We propose that online workers have different motivations than do other workers – including different concerns about wages and different degrees of intrinsic enjoyment of computer tasks. During the first experimental round, with fixed payments for all participants, intrinsic workers, were performing worse than extrinsic ones. After our bonus payment -per -correct -answer treatment took effect in the second round, the intrinsic workers increased their performance by approximately 3%. Also, when we took into account that, intrinsic motivation may be confounded by other personality traits, several effects of personality traits were revealed. Our attempt represents a significant contribution because employers-requesters are naturally interested in the extent to which the working behaviour of employees - workers change during an online job and how. Also, a better understanding of wage policies in online environments will enhance their ICT components, making them more efficient and providing a positive effect on online labour productivity (Relich Citation2017).
To the best of our knowledge, ours is one of the first studies directly aimed at unbundling, initially, the relationship between incentives and online working behaviour, under different payment conditions within an experimental framework, by exploring the self-determination theory as a basis for interpretation. A second objective is to reveal further evidence that personality traits are fundamental drivers of online working economic decisions. However, incentive-enhancing preferences are irreducibly heterogeneous and their link with non-cognitive behaviour needs further investigation (Bowles, Gintis, and Osborne Citation2001; Huffman and Bognanno Citation2018; Lindqvist and Vestman Citation2011). Hence, by taking all the above into consideration, our findings support Horton’s and Jain and Parkes’ arguments, that a further investigation in online labour markets is needed and advances in crowdsourcing as a methodology will require broadly applicable, performance predictive models, for revealing the key role of personality heterogeneity in explaining wage dispersion in online job environments.
2. Theoretical background
2.1 Compensation policy and worker performance
The role of compensation policy in influencing worker performance is still extensively under investigation by both economists and psychologists. Of particular interest in recent empirical research are the effects of payment schemes on worker productivity, often referred to as ‘incentive effects’. More specifically, there is a small body of empirical literature investigating whether paying workers on the basis of output induces them to produce more (i.e. performance pay policy) (Franceschelli, Galiani, and Gulmez Citation2010). Thus, the primary motivation behind introducing a piece rate scheme is, firstly, to further engage workers by increasing their efforts (Lazear Citation2000). Standard economic theories and empirical papers have pointed out that without adopting performance pay policies, extrinsic incentives erode intrinsic, and predict higher performance (Lazear Citation1992). However, recent research also revealed evidence that, under several working conditions, a link can establish between intrinsic motivation and performance (Cerasoli, Nicklin, and Ford Citation2014).
It is generally believed that an easy way to increase an individual’s productivity is to pay based on measured performance. Previous studies however have pointed out several difficulties with pay-for-performance wage structures in terms of measurement.Footnote1 The lack of data and the difficulty to find appropriate conditions in which some workers are in a performance-pay state, while others are paid a fixed amount regardless of productivity levelFootnote2 bring complex issues into the examination of the choice of payment scheme and its effect on individuals’ outputs in relation to cognitive, personality and demographic characteristics (Dohmen and Falk Citation2011; Franceschelli, Galiani, and Gulmez Citation2010; He, Qiu, and Cheng Citation2022; Lazear Citation2000). The analysis in this study does not suffer from this traditional problem. Our paper is based on data drawn from an experiment designed in an online labour market, which measures and assesses personality and motivation parameters to directly predict workers’ productivity under several performance-pay and fixed term wage scenarios (Goncalves et al. Citation2015; Rogstadius et al. Citation2011).
2.2 Motivation crowding out
Motivation crowding theory was developed to examine how worker utility functions are determined. This theory suggests that an external intervention via monetary incentives can undermine and -under different identifiable conditions strengthen- intrinsic motivation (Frey and Jegen Citation2001). Within this theoretical context, through a field experiment Kuvaas et al. Citation2017 showed that intrinsic motivation is positively related to affective commitment, resulting in positive employee outcomes. In the same direction, research studies investigating traditional labour markets confirmed the productivity crowding-out effects of intrinsic incentives and the role of workers’ self-selection mechanism in the labour markets (Barigozzi and Burani Citation2016; Barigozzi, Burani, and Raggi Citation2018; Cunyat Citation2019; Kim Citation2020). Lastly, Cassar and Meier Citation2018, have shown that nonmonetary intrinsic incentives and nonpecuniary aspects of one's job have substantial impacts on job satisfaction, productivity, and labour supply, meaning that, for many workers, work is a source of meaning. But do all the above findings also apply to online labour markets? Several papers have examined the link between incentives and compensation policies in online labour platforms from both economic and IT perspectives. For example, according to Faradani, Hartmann, and Ipeirotis Citation2011, just increasing the reward for a task decreases demand for it. High reward tasks are a signal for complex and involved tasks that decrease the utility of high rewards for the worker. Furthermore, overpriced tasks are also undesirable since requesters may invest excess capital qualifying a deluge of data from eager respondents (Gonen et al. Citation2014). More studies have also revealed that an unconditional reward policy can play an important role in productivity outcomes only for extrinsic driven participant workers (Dube et al. Citation2020; Liang et al. Citation2018). Improper pricing or scheduling often results in task starvation and loss of capital for these markets (Wu Citation2019). Consequently, the literature tries to explore the psychological determinantsFootnote3 of workers’ underlying incentive mechanism (Benabou and Tirole Citation2003; Kreps Citation1997), and how the crowding-out effect holds within a working environment, especially for intrinsic driven individuals, resulting in higher productivity and efficiency levels (Frey and Jegen Citation2001). While previous studies have examined the influence of motivation across different remuneration frameworks, such analyses have tended to adopt a relatively stable approach. When payment schemes are altered dynamically during a job task, individuals with different types of motivation may exhibit varying levels of adaptability to these changes. For example, individuals with intrinsic motivation while may demonstrate greater flexibility in their working behaviour, rewards can have a detrimental effect on their performance towards the task (Hendijani et al. Citation2016). On the other hand, those with extrinsic motivation may be more responsive to changes in compensation (Benabou and Tirole Citation2003). Understanding these dynamics is critical to developing compensation schemes that are aligned with individuals’ motivations, which can ultimately lead to improved task performance and job satisfaction. Motivation is a dynamic process. For that reason, our research utilises a dynamic approach in which compensation is adjusted during the crowdsourcing process over the course of two experimental rounds.
2.3 Personality traits
In recent years, economists have been using psychological skills (personality traits and their relative aspects) to link personality to economic preference parameters which are fundamental drivers of decisions in economic theory (Borghans et al. Citation2008; Cadsby, Song, and Zubanov Citation2019; Heckman and Rubinstein Citation2001; Nyhus and Pons Citation2005). However, some of the measures used by researchers are likely to suffer from endogeneity issues and require a very careful approach (Drago Citation2011). For this reason, economists and psychologists have recently employed in their experiments the Five-Factor Model of Personality, which measures the so-called ‘Big Five’ personality traits (Costa and McCrae Citation1992). This model includes the traits of openness, conscientiousness, extraversion, agreeableness and neuroticism and is very efficient because these traits are unlikely to experience ordinal changes, particularly after school (Roberts and DelVecchio Citation2000). The stability and robustness of the Big Five Personality Test allowed several researchers to deepen into the study of the determinants of performance in labour markets (Cobb-Clark and Schurer Citation2012).
Thus, we utilise the psychological trait theory and its suggested taxonomy to draw conclusions on whether compensation policies in online labour markets affect, incentives based on individual differences differently. Personality is already linked with working behaviour in both offline (Cubel et al. Citation2016; Gensowski Citation2018; Heckman, Jagelka, and Kautz Citation2021) and online working environments (Kazai, Kamps, and Milic-Frayling Citation2011; Mourelatos and Tzagarakis Citation2016). Thus, we embed the Big Five personality traits measurements in our analysis. Robert’s, (Citation2009) and Roberts and DelVecchio (Citation2000), definitions of personality (i.e. non-cognitive skills) suggest that all psychological measurements are calibrated on measured behaviours or broadly defined ‘tasks’. Thus, in our case, to dynamically monitor participants’ real time response to monetary rewards, we adopted a task with several performance-related pay strategies. This technique is a standard element for behavioural economics researchers and business practitioners, who dynamically investigate and measure various productivity outcomes.
Already in the literature, within traditional (i.e. offline) markets and controlled laboratory environments, several attempts revealed relations between the ‘Big Five’ inventory and earning differences (Fletcher Citation2013; Nyhus and Pons Citation2005). For example, there is evidence that conscientiousness and emotional stability (the inverse of neuroticism) have, in general, a robust positive relationship with job performance (Burks et al. Citation2015; Donato et al. Citation2017; Hogan and Holland Citation2003; Witt et al. Citation2002). There are also some indications of a positive impact of extraversion and openness to experience on wages (Heckman, Jagelka, and Kautz Citation2021).
Regarding online labour markets, first attempts also give evidence that personality traits are key factors of workers’ behaviour. Mourelatos, Giannakopoulos, and Tzagarakis Citation2022, using a transcription task, showed that conscientiousness and agreeableness is associated with online job performance, while Kazai, Kamps, and Milic-Frayling (Citation2011; Citation2012), using a label task, showed a negative effect of extraversion and neuroticism in workers’ job accuracy. Shi et al. (Citation2022), linked conscientiousness, neuroticism and extraversion with solver online engagement. Nevertheless, there is a lack of research providing evidence from labour-oriented experiments on online labour markets (Kazai, Kamps, and Milic-Frayling Citation2012).
By taking all the above-mentioned into consideration, we, mainly, expect that our treatment will trigger the intrinsically- driven individuals to perform better than the extrinsic ones and personality traits will moderate this effect, especially for individuals who exert high levels of conscientiousness and agreeableness.
2.4 Self-Determination theory
At this point, a theoretical framework to collaborate all the mentioned theories is needed to our research questions to tie together. In this direction, self-determination theory (SDT hereafter) provides a framework for understanding the factors that promote motivation and psychological and behavioural functioning. SDT supports a basic distinction between intrinsic and extrinsic motivation and suggests a mechanism through which the treatment’s effect heterogeneity may have operated: by boosting the autonomous motivation of more intrinsically motivated workers (while reducing the controlled motivation of more extrinsically motivated individuals) (Ryan and Deci Citation2017). Autonomy involves acting with a sense of volition and having the experience of choice (Ryan et al. Citation2019). Intrinsic motivation is an example of autonomous motivation because when individuals engage an activity because they find it interesting, they are doing the activity wholly volitionally. Although the theory is psychological, research has also given attention to behavioural underpinnings of these processes and places them in an evolutionary perspective in various fields.
Many authors discuss SDT as a more global theory of motivation for the workplace (Baard, Deci, and Ryan Citation2004). Although is well established that the use of salient extrinsic rewards to motivate work behaviour can be deleterious to intrinsic motivation, Gagne, Deci, and Ryan (Citation2017), build on the SDT framework and they argue that also alternative ways exist, in which tangible rewards can be used so as not to be detrimental to intrinsic motivation. Employers should be careful not to overuse extrinsic rewards. Too many rewards can undercut intrinsic motivation (a phenomenon known as the over justification effect), yet too few can cause employees to feel unappreciated. Experimental laboratory studies in social psychology have already pointed out that when individuals are offered performance-contingent monetary rewards, it can make the external controlling aspect of the reward more prominent, which can undermine their intrinsic motivation. This occurs because the emphasis on the external reward can reduce individuals’ sense of autonomy and control over their work, which can diminish their intrinsic motivation (Hagger and Chatzisarantis Citation2011). In other words, the prominence of the performance-contingent monetary reward can crowd out the intrinsic motivation that individuals may have had for the task, leading to a decline in overall motivation and performance.
Recent experimental research on gig and online economies highlights the need for personalised payment schemes that consider individual differences in intrinsic motivation and task preferences, and suggests that the relationship between intrinsically motivated workers, extrinsic rewards, and productivity is complex and may vary depending on individual factors. Butschek et al. (Citation2022), found sizeable heterogeneity effects on online productivity with respect to intrinsic motivation and personality: Their proposed treatmentFootnote4 reduced performance for less intrinsically motivated workers and it increased performance among conscientious workers with a high task motivation. By drawing on this evidence and relating it to the literature in psychology, suggests that economic decisions are strongly influenced by a rich interplay of preferences, biases, and skills as well as the other factors.
3. Method
3.1 Amazon mechanical Turk
We recruited participants from the U.SA. through Amazon Mechanical Turk, an online labour market (AMT hereafter). AMT involves two major categories of participants. Employers, known as requesters, who post online jobsFootnote5 for potential individuals to do for pay, and individuals, known in bibliography as ‘Turkers’ who work in task-based labour in exchange for a wage set by the requesters. AMT’s price policy allows requesters either to set a flat rate wage strategy or a piece rate reward and hire Turkers using several criteria (i.e. Turkers who have previously worked in a number of HITs or have a general task completion approval rate over a set percentage). The general workflow of this crowdsourcing platform initially follows a requester, who posts the main task (i.e. which may consist of several smaller microtasks - HITs) under a set wage strategy. Depending on their work preferences, Turkers browsing offered jobs then choose the HITs they will take part in. After that, requesters review the work submitted by each participant, and accept or reject it, depending on whether its quality meets the job requirements set at the beginning of the task. In the end, AMT delivers the requester’s posted payment to the approved Turkers. The overall HITs workers complete over their working lifetime contributes to their approval rate. This index serves as a type of reputation score, offering requesters a proxy comprehension of Turkers’ work ethics (Kingsley, Gray, and Suri Citation2015). Thus, AMT’s design, which shapes market dynamics as an online labour market, was very compatible with our experiment’s workflow and characteristics, covering the basic components of job performance. Numerous studies have already shown that data gathered using AMT are no less quality than data collected on the standard physical lab (Arechar, Gächter, and Molleman Citation2018; Goodman and Paolacci Citation2017; Horton, Rand, and Zeckhauser Citation2011; Paolacci, Chandler, and Ipeirotis Citation2010).
3.2 General experimental design
The quasi-experimental sessions were divided into two rounds with an obligatory break in the middle. Each phase of the experiment had a duration of 150 s while the break had a duration from 120 to 150 s. In the instructions of the task, we informed potential participants that the reward was 1 $ for task completion (piece-rate pricing) and the total duration of the experiment, including the survey, was approximately ten minutes. To avoid self-selection bias, the offered wage was in line with AMT’s price policy. The real effort task uses the timed mathematical additions task of Niederle and Vesterlund and the first of which functioned as a demonstration and attention check.Footnote6 The task was programmed using zTree (Fischbacher Citation2007) and has characteristics that allow us to measure productivity as a function of cognitive abilities, soft skills and demographic characteristics, reflecting on an individual’s level of concentration, effort, stress management and adjustment. The numerical additions were designed to be reasonably simple, if repetitive. Earlier studies have deemed this a good measurement of intellectual effort and individual productivity (Niederle and Vesterlund Citation2007). The experiment conducted with a two-period design, to provide us with a baseline measure of online performance capturing task characteristics, intrinsic motivation to perform the task and with a measure of whether treatments affected intrinsic motivation (which can only be captured in a subsequent period without rewards) (Bradler, Neckermann, and Warnke Citation2019). To assure data quality, we set 2 default criteria for workers to have the opportunity to be hired in the experiment: 80%.Footnote7 success rate in their previous task completion activity and participation in at least 50 approved tasks in AMT (Peer, Vosgerau, and Acquisti Citation2014). We collected data from 465 workers only from the USA and 19,407 responds ().Footnote8
Table 1. Experimental design.
Lastly, we also run power calculations to overcome potential issues regarding the validity of our findings, which confirm that the sample size of the study is adequate to provide results with the desired power and significance.Footnote9 (See Appendix B for further discussion).
3.3 Measures
Before the online task commenced, workers were asked to fill in a survey. Firstly, to see how personality traits affect their performance and adaptation to salary changes, we used the Big Five Personality test, a 44-item questionnaire (McCrae and Costa Citation1999) providing measures for each personality trait i.e. Openness, Conscientiousness, Extraversion, Agreeableness, Neuroticism (hereinafter OCEANFootnote10). The role of personality traits is well established in standard behavioural models on task performance and the adopted OCEAN taxonomy captures individual-specific differences in ways of thinking, feeling, and behaving (Filiz-Ozbay et al. Citation2018). In addition, this mid-sized test ensures an accurate and stable measurement of each personality facet (Cobb-Clark and Schurer Citation2012), without being excessively time-consuming, which could result in some measurement bias errors. The Big Five dimensions of personality were estimated on a scale of 1-5, where 1 = disagree, 2 = slightly disagree, 3 = neutral, 4 = slightly agree and 5 = agree. Afterwards, the OCEAN factors were constructed through a factor analysis, to render each trait orthogonal to the rest (McCrae and Costa Citation1999). To allow for an easier interpretation of our estimates, Big Five scores are standardised to have mean zero and standard deviation of one in all reported specifications.
We also ran a quick test through the survey, to measure participants’ level of computer competence.Footnote11 Given the rapid proliferation of computer technologies (ICT), computer skills in particular become even more important for predicting individual performance especially for older subjects, eliminating age-dependent differences.
Our computer competence questionnaire (CCQ), based on the INCOBI computer expertise inventory by Richter,Footnote12 contains 10 three-point Likert Scale items assessing theoretical and practical computer knowledge by allowing participants to express how much they agree or disagree with several computer-related aspects. Our aim was to create a measure of computer competence. The CCQ test demonstrated excellent reliability (Cronbach’s α = .873), with subscale reliabilities ranging from .852 to .870.
One last remark is that performance in the task chosen should also be influenced by the interaction of cognitive abilities with soft skills (Borghans et al. Citation2008). Therefore, our findings could be merely reflecting that workers with a higher level of cognitive skills also score higher in certain personality facets. For that reason, we include in our survey an IQ test, the Stanford-Binet IQ, which has been shown to include the result of cognitive and non-cognitive abilities. TermanFootnote13 also adapted the Stanford - Binet IQ test for use with American populations to predict academic performance. After taking into consideration an increasing body of literature that explores the relationship between productivity and a wide variety of economic and demographic outcomes, such as educational attainment and labour income, we also asked participants to report their age, gender, ethnicity, educational level, their mother’s and father’s educational level, their job status and average monthly family income.
3.4 Sample characteristics
presents the descriptive statistics for the explanatory variables, which are grouped in the individuals’ demographic characteristics, cognitive skills, socioeconomic characteristics and personality traits.
Regarding demographics, 52.5% of our sample is female subjects, with a mean age of 37 years. Concerning ethnicity, the workers were 83% Whites, 6.9% Black and 10.1% Asian. Regarding their cognitive abilities, 83.2% of our hired crowdsourcing workers have a high IQ level and 87.7% high computer competence. Subjects have a low educational profile (i.e. only 20% have completed college education), but 82% are working (i.e. active labour force status). Lastly, half of our participants have a high family income (i.e. at least 3,000 USD per month) and come from families with parents with at least tertiary education (i.e. 51.1%). Regarding incentives, we constructed a binary variable of intrinsic and extrinsic motivation, grouping workers who reported that they participate in our task for fun, or to contribute to the crowd, or as a way to develop their skills, as intrinsic ones, and workers who participate because it is their full- or part-time job, as extrinsic ones (Liang et al. Citation2018). Our sample consists of approximately 40% intrinsic and 60% extrinsic participants.
With respect to their personality profile, the five variables of the Big Five Personality test while are designed to give a mean of 50 with a standard deviation of 10 for each trait, we used the normalised version of OCEAN variables in a range of 1–5, where 1 denotes a very low level of the trait and 5 a very high level respectively.
According to the observed variation in personality traits, we see that the mean score for Openness is 3.62, suggesting a high tendency towards creativity and active imagination, for Conscientiousness 4.03, signifying high levels of thoroughness, for Extraversion 2.86, signifying a moderate level of worker energetic behaviour, for Agreeableness 3.84, indicating that our workers seem more empathetic and altruistic and for Neuroticism 2.80, suggesting that workers do not tend to experience negative emotions ().
Table 2. Sample characteristics.
Research assessing characteristics of the MTurk workers as social science research participants concludes that workers represent a large and diverse population, yet MTurk workers are a convenience sample (Paolacci and Chandler Citation2014). Research on online demographic characteristics has shown that, Amazon Mechanical Turk (MTurk) is a dynamic, ever-changing platform. Based on the latest evidence, approximately 57% of people on MTurk identify as female, 38% mostly 30–39 years old, 79% whites, with 23% at least college education (Coppock Citation2019; Litman and Robinson Citation2020). Hence, in relation with our study, we do not observe statistically significant differences between our sample and the general AMT population demographic attributes, confirming the internal validity and reliability of our results (Thomas and Clifford Citation2017) (See Appendix A for further discussion).
3.5 Empirical model
In this section, we utilise straightforward basic econometric methods to investigate whether the relationship between personality traits, motivation (intrinsic of extrinsic) and productivity is heterogeneous across subjects’ individual characteristics by following Heckman’s conceptualisation (Heckman, Jagelka, and Kautz Citation2021). We estimated the following baseline regression specification by ordinary least squares (OLS):
(1)
(1) where Yi is our productivity measurements (e.g. logarithmic values of productivity, payoffs and numbers of total correct answers and responses) by worker, NCi is a n-vector of non-cognitive skills of worker i (where k = 1, … ,5 corresponds to Openness, Conscientiousness, Extraversion, Agreeableness and Neuroticism, respectively), Mi is the motivation of worker i (i.e. whether worker i’s incentive is intrinsic or extrinsic), ECi is an m-vector of the experiment characteristics (i.e. where m = 1,2 denotes whether a worker belongs to the benchmark group or the treated group and the time period of the experimental phase, respectively), Xi are personal characteristics (i.e. demographic attributes, cognitive skills and socioeconomic background of worker i), îi is a vector of location fixed effects and ei an i.i.d. disturbance term.
Our experimental design assumes that the micro-task designer (requester) is willing to pay a bonus number of monetary values to a specific micro-worker to produce a correct outcome (Yi), i.e. the total amount of correct adding operations of two-digit numbers. The expected outcome (Yi) is assumed to be a stochastic function depending on observed productive characteristics (e.g. cognitive and non-cognitive skills), incentive variables (e.g. workers’ motivation in task participation) and other unobserved factors (e.g. innate ability, crowdsourcing experience).
4. Experiment
4.1 First experimental round
4.1.1 Procedure
Participants after accepting the HIT in Amazon Mechanical Turk were redirected through a web-link to the experiment, where they were asked to freely browse the detailed instructions. Once the navigation was completed, participants were asked comprehension questions as an attention check. All participants were asked to fill in the survey through a computer to prevent the use of smaller screen sizes (e.g. smartphones). Being in the actual experiments, in round 1 participants were asked to respond to as many additions of 2-digit random numbers as possible within the timeframe of 150 s.
4.1.2 Measures and task outcomes
The nature of our task allows us to investigate and measure individual productivity in several ways. Our primary outcome measure is the number of total correct answers provided, which is a direct measurement of the performance of a participant worker. Also, performance was measured as the division of total correct answers by the number of responses, effort as the total duration of task engagement in seconds, average payoffs as the mean value of earnings ($) and productivity index as the division of total payoffs by effort. Lastly, we calculated the cumulative number of responses as quantitative labour measurement. contains the summary statistics of the abovementioned task outcomes of the first experimental round (Column 2). For the whole sample, we observe an average of 20 correct additions, reflecting an average of 91.5% of task performance. Moreover, workers had an average of 51 s of pure effort on the additions of the two-digits and an average of 22 responses.
Table 3. Task outcomes summary statistics.
4.1.3 Results
present baseline estimates for the first experimental round. Model specifications control for several demographic, cognitive and social economic characteristics. It is noteworthy though, that following the recent literature on demographics and online productivity, we observe that female workers had statistically significant deteriorations in work performance and productivity than males, throughout all columns (Cantarella and Strozzi Citation2022; Straub et al. Citation2015). While this finding is in line with gender differences in traditional job performance studies, it is the first time that this negative relationship appears robust in the investigation of online labour markets. Regarding the estimated coefficients of personality traits, we show that extraversion is negatively associated with performance, while agreeableness is positively related to performance (Column 2). An increase of one unit in workers’ extraversion and agreeableness level is correlated with a decrease of 0.9% in performance and an increase of 1.9% in performance, respectively. Openness, conscientiousness, and neuroticism appear to be statistically insignificant.
Table 4. Determinants of outcome variables.
Finally, we do not observe incentive effects on labour outcomes coming from intrinsic motivated workers, on the first experimental round.
4.2 Second experimental round
4.1.4. Procedure
Between the two rounds, subjects had a two to two-and-a-half-minute break and were not allowed to leave the task environment. During the break, workers were informed that in the second round they could be given an additional bonus per correct answer, added to the initial payment of the one dollar in the first round. An algorithm randomly assigned all participants into five new groups of treatment. Each group had a different level of a bonus reward i.e. 1, 5 cents, 10 and 15 cents. Only one group of participants, ‘the benchmark group’, received no information on additional bonus rewards during the break. Until this time, no information about their actual performance was given and the workers were not informed of the randomisation process. While receiving the new instructions, workers could watch a song video. After the end of the song, the tab for the second phase was activated, and the subjects were able to proceed. During the second phase of the experiment, the workers could monitor their current performance through a counter measuring their bonuses each time they answered correctly.
4.1.5. Measures and task outcomes
also contains the summary statistics of the abovementioned task outcomes of the second experimental round (Column 3). For the whole sample, we observe an average of 23 correct additions, reflecting an average of 93.4% of task performance. Moreover, workers had an average of 57 s of pure effort on the additions of the two-digits and an average of 24 responses.
Column 4 includes the t-tests of the difference of the experimental rounds for each above variable. Regarding the whole sample, we observe that the increase of task labour outcomes in the second round are all 1% statistically significant, raising questions on the determinants of workers’ average improvement.
Analysing further, we divided workers into two major groups depending on whether they were assigned for treatment. The results show that average worker performance increased in round two (i.e. 2%), only for those treated with piece-rate scheme payments over basic-wage individuals. Furthermore, treated workers had a statistically significant increase in their average sum of correct answers, effort, the number of total responses, productivity and payoffs over workers who had no additional information about extra piece-rate payment in the second round. This initial finding, casts doubt on whether the magnitude of the treatment has a similarly positive effect on workers’ performance and productivity index. We thus grouped results regarding compensation schemes based on four random levels of extra bonuses.
Tellingly, participants assigned to the higher bonus group had a statistically significant improvement in performance (i.e. approximately 3%), while those in the lower extra bonus group had a statistically significant increase of 1.8% in round two.
On the other hand, the results concerning workers’ average effort and number of total responses coincide among the four categories of treatment.
4.1.6. Results.
Regarding, the findings of the second experimental round and throughout the entire experiment, includes the coefficient estimations for equation (1).
As we expected, given the repetitive nature of the online job, we find that regarding worker performance, in general, openness has a statistically significant negative effect on performance. An increase of one unit in worker openness is correlated with a decrease of 1.1% in performance, of 4.4% in the sum of total correct answers and of 3.3% in the sum of responses, with the magnitude of the effect being higher in the second round of the experiment, when the different payment schemes were used. Moreover, the coefficient for agreeableness seems to have a positive, high statistically significant effect (1% level of significance) on performance, confirming the findings by Gill and Prowse Citation2016. Concerning the level of workers’ conscientiousness, our finding also supports the existing literature. Our analysis also revealed the positive and significant effect of this trait on performance and payoffs (Cubel et al. Citation2016; Fletcher Citation2013). The coefficient for conscientiousness is, albeit insignificantly, also positive and of sizeable magnitude in relation to the average total correct answers of individuals. Furthermore, in respect to neuroticism and based on literature, we expected a negative effect on performance. Nonetheless, we failed to confirm such a correlation. Our results revealed that an increase of one unit in neuroticism is correlated with an increase of 14.4% in payoffs, 2.9% in the sum of responses and 10.8% in the productivity index. Finally, we find a negative correlation between the level of worker extraversion and performance; this effect is triggered in the first experimental round but disappears in the second round (Columns 2 & 3). Taking into consideration that extraversion is related in literature to ‘explicitly rewarded’ schemes (Stewart Citation1996) and given the wage working condition of our task, seems that the negative impact of extraversion on performance is to be expected.
The inspection of and , which follow participants’ average total correct answers over the time of the experiment, with the vertical line separating the two rounds of the experiment, suggests that even though we have an overall improvement of performance over time, this is independent and uncorrelated with the different payment schemes groups the workers were randomly assigned to.
Figure 2. Average total correct answers between the ‘Control’ and ‘Treated’ group of workers over time.
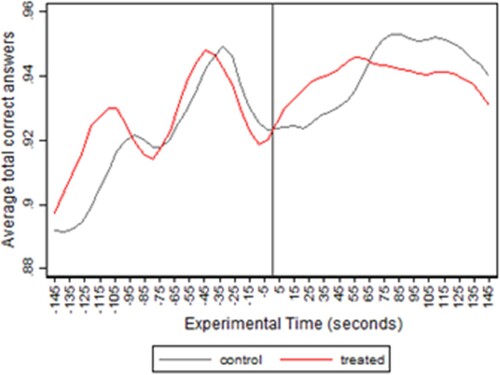
Figure 3. Average total correct answers between the ‘Control’ and the ‘Bonus’ groups of workers over time.
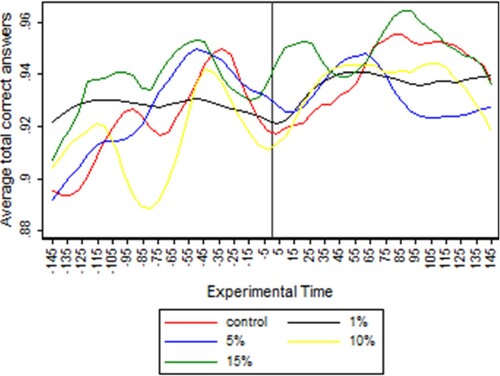
Finally, as known, the relationship between rewards and workers’ performance under intrinsic motivation is already established in literature. Bonus rewards could have negative effects on performance quality in a labour activity, once a part of the reward is received (Sansone and Harackiewicz Citation2000) for participants with intrinsic incentives, which are also related to the degree of their task enjoyment (Tauer and Harackiewicz Citation2004). By taking into consideration the ‘monotonous’ nature of the task and the experimental design, which includes two rounds, the first with a fixed payment and the second with performance-payments, a strongly negative relationship between several task outcome measurements and intrinsic motivation is expected. Furthermore, as regards performance and total correct answers, the effect of intrinsic motivation seems to exist only in the second round, in which performance-payments are applied. In total specifications, the subjects with intrinsic incentives performed on average 1.2% worse, having 5.8% fewer total correct answers, 12.9% fewer total payoffs and 4.5% fewer sum of responses, at 1%, 5% and 10% levels of statistical significance.
5. Heterogeneous effects
5.1 Incentive effects
Workers can use intermediate performance information to determine how much additional performance is necessary to obtain a bonus. This creates dynamic incentive effects, where the incentive effect of the pay-for-performance plan at each point in time depends on realised performance up to then (Benabou and Tirole Citation2003). Incentive plans based on relative performance, where prizes are awarded for sufficiently outperforming other competitors, are particularly prone to dynamic incentive effects (Baker Citation1992; Delfgaauw et al. Citation2014; Fama Citation1991). To investigate these different incentive effects deeper, we analyse the response level over the experiment’s period, on our database of 465 participants in two rounds, who submitted 19,407 responses in total.
shows the average treatment effect on three outcome variables (i.e. productivity, total correct answers, and effort- the seconds that, each worker did in each addition of two digits’ numbers) by motivation, during the experiment. Given the experimental design, it is obvious that as regards the ‘productivity index’ the treated subjects were more statistically significantly productive than the benchmark group. However, we included this outcome variable to investigate the motivation effect on response levels during the experiment. Although in baseline specifications intrinsic motivation did not influence productivity in round 2 (, Column 9), when we look at response levels, we observe a negative statistically significant effect on the productivity index (, Column 1). Concerning the sum of total correct answers, our analysis shows that there is no statistically significant average treatment effect on worker performance. However, the lack of an average treatment effect masks considerable effect heterogeneity by intrinsic motivation (, Column 2). In line with other studies on treatment effects by motivation, the coefficient on the interaction between treatment and the intrinsic incentive dummy is positive and statistically significant at 1% level of significance, although intrinsic subjects perform worse than extrinsic ones in the beginning of the task (i.e. round 1) (Butschek et al. Citation2022; Chen & Horton, Citation2016). Our regression estimates imply that during the experiment intrinsic subjects had −0.032 fewer total correct answers with bigger effort per response (0.071 s). A possible reason for this is that intrinsically motivated online workers might be less keen to maximise their performance due to differences in degrees of risk tolerance because of personality heterogeneity (Cubel et al. Citation2016; Müller and Schwieren Citation2012).
Table 5. Treatment effect on outcome variables by motivation during the experiment.
5.2 Personality effects
We now turn our attention on the moderating role of personality traits. This section focuses on how individual differences may interact with responses to incentives under our performance-pay intervention (i.e. treatment).
shows the estimates of our regression using the participants’ sum of total correct answers, which is the pure performance measurement in our experiment, as an outcome variable. Firstly, we observe that the coefficient on effect heterogeneity by intrinsic motivation remains similar in size and significance at 1% level of significance, throughout this exercise, even when controlling for all traits and interactions simultaneously. Including such a range of relevant personality characteristics gives us confidence that the heterogeneity by intrinsic motivation we observe is not just the effect of an omitted personality trait we do not observe. Hence, the heterogeneous treatment effect with respect to intrinsic motivation seems very robust. Furthermore, interestingly, conscientiousness and agreeableness lead to an independent heterogeneous effect in the same direction, while neurotic and extravert treated participants seem to have a loss in their online performance per response, during the experiment (, Column 7).
Table 6. Personality-dependent treatment effects on total correct answers outcome during the experiment.
In our formal model, conscientiousness can be viewed as a trait that similarly affects work performance: a more conscientious person exerts a specific service level driven by a feeling of obligation, which would also lead to a lower elasticity of sales and to a reduction in marginal monetary incentives (Barrick and Mount Citation1991; Salgado Citation1997; Tett, Jackson, and Rothstein Citation1991), while recent studies also point out that more agreeable subjects perform better (Gill and Prowse Citation2016). On the other hand, the negative heterogeneous treatment effects of neurotic subjects (i.e. subjects with lack of emotional stability and predictability and with anxiety and insecurity) appears to be in line with the existing literature (Nyhus and Pons Citation2005), showing that high degrees of worker neuroticism impair their ability to focus on a task, especially under time pressure (Borghans et al. Citation2008). Given that extraversion seems to positively correlate with tasks that involve social interaction, we assume that the negative impact on the performance of extraverts is driven by the monotonous task design and its characteristics.
6. Discussion
The main objective of this study was to examine the effect of intrinsic incentives on online labour productivity. Across a novel experimental procedure consisting of two rounds and several payment schemes, we provide evidence that immediate rewards increase intrinsic motivation by strengthening the activity-goal association. Intrinsically motivated workers achieve higher productivity outcomes as compared to less motivated ones. Following also previous studies on how workers with different personality traits respond to different payment schemes regarding their online performance and individual productivity, we observe that our results confirm several economics and psychology studies and provide good practice guidance for improving the effectiveness of online labour markets. Hence, this study also explores the underlying mechanisms and boundary conditions that explain why and under what circumstances personality traits (extraversion, neuroticism, and conscientiousness) moderate the effects of intrinsic motivation relate to productivity in online crowdsourcing communities. The results can be summarised as follows.
Initially, in line with other studies on treatment effects by motivation, we found that intrinsic individuals perform worse under a flat, no performance-based wage scheme (Kreps Citation1997; Larkin and Leider Citation2012). Surprisingly, our study reveals that when intrinsic individuals performed within piece-rate wage contexts, they changed their working behaviour and had significantly higher online productivity. We confirm the reverse payoff effect of performance-based payments by regressing performance on incentives, treatment and pay scheme (). By using self-determination theory, we confirm that an external wage intervention can crowd-out intrinsic motivation. Our results suggest that the compensation framework and the online working context may be more important than previously thought (Woolley and Fishbach Citation2018). The reasons why workers driven by intrinsic motivation increase their productivity after a change of the payment scheme from fixed payment to piece-rate payment remains to be further explored. In our case, we tried to interpret the results by embedding individuals’ personality traits in our analysis. Our experimental results demonstrate that personality skills are likely to be underlying factors associated with person-specific determinants of working behaviour (Heckman, Jagelka, and Kautz Citation2021). Thus, we further extend the findings of Cubel et al. Citation2016; Gill and Prowse Citation2016; Mourelatos, Giannakopoulos, and Tzagarakis Citation2022, revealing that agreeable and conscientious subjects perform even better when performance-based payments are applied to an online task ().
Intensified also by the COVID-19 pandemic, online labour markets are at the core of the economic and policy debate about the future of work and the conditions under which people will work mostly in online environments (Mueller-Langer and Gomez-Herrera Citation2022). Thus, our findings provide also key insights on online productivity determinants and contribute to this growing debate, aiming to improve several aspects of socioeconomic development.
7. Implications
7.1 Theoretical implications
Our study contributes to the literature on the relationship between incentives, personality traits, and online labour outcomes, providing insights on how personality-specific differences and incentives influence workers’ productivity in crowdsourcing. First, this study contributes to the deeper understanding of the underlying incentives mechanism of workers. Previous research has explored the impact of motivation under various payment structures; however, these analyses were conducted in a relatively static manner. In contrast, our experimental design leverages two rounds of experimentation to dynamically adjust compensation throughout the crowdsourcing process. This novel approach allows us to investigate the effects of motivation in a more nuanced and dynamic manner. Hence, the present study complements the existing literature by highlighting that the introduction of payment schemes based on real time performance does not have similar significant effects on working behaviour for all individuals. More specifically, while intrinsically motivated individuals, in general, seem to perform worse, their engagement with our wage intervention leads to a better labour outcome. Thus, our results contribute to the literature of self-determination and motivation crowding theory in online labour contexts, by providing insights on how personality-specific differences and incentives influence workers’ labour outcomes (Dube et al. Citation2020; Huffman and Bognanno Citation2018; Rustichini et al. Citation2016). A better understanding of wage policies in online environments will enhance their ICT components, making them more efficient and providing a positive effect on online labour productivity.
Second, we examined the moderating role of personality in relation between workers’ incentives and online outcomes, thus revealing the psychological mechanism of online working. Most of the previous studies have seen workers as a whole to explore the factors affecting their intention to participate, to engage and perform in crowdsourcing tasks. This study considers the influence of their personality a key determinant of their performance. Thus, we categorise personality into five traits (i.e. openness, conscientiousness, neuroticism, conscientiousness, agreeableness), and separately examine the disparity in its moderating effect between incentives and several productivity outcomes, which demonstrate the importance of individual’s personality in crowdsourcing procedure.
Third, while several previous studies highlight the importance of studying online workers’ behaviour in specific contexts and scenarios, this research contributes to existing literature by extending the current research on the development of the ICT aspects of crowdsourcing, by adopting a dynamic monitoring experimental procedure (Colman, Vineyard, and Letzring Citation2018; Mourelatos and Tzagarakis Citation2016). By investigating the workers’ response in depth within a pure productivity task, we highlight the need for further personalisation and task customisation strategies in online labour platforms (Ipeirotis and Horton Citation2011; Liang et al. Citation2018). From a macro perspective, our results inform policymakers on the regulatory treatment of online crowdsourcing workers, emphasising the need for more individual-specific and personalised approaches.
7.2 Managerial implications
Online labour markers are gradually emerging as a key driver of economic sustainability. Their rapid growth has focused attention on crowdsourcing and its contribution to social and economic development (Cui et al. Citation2021). Participants in these online platforms can obtain and access resources without boundaries or restrictions and have also the opportunity, though several ICT technologies, such as automatic matching and sorting technologies, to improve their efficiency and socioeconomic development (Harvey, Smith, and Golightly Citation2017). However, even though online labour markets and crowdsourcing are focal points of current research, the existing studies lack a comprehensive investigation covering various factors from social and economic perspectives, which may lead to higher efficiency and efficacy.
Within this context, the managerial implications of this study are manifold. Incentive issues have become an important challenge for the development and progression of the evolution of crowdsourcing. Many studies, especially economic, have focused on the incentive aspects of online labour platforms, examining motivations from a game theory perspective (Faberman and Kudlyak Citation2016 and Banfi and Villena-Roldan Citation2019) while IT scientists investigate incentives in terms of quality assurance and participation (Gong Citation2017). The workers’ behaviour in crowdsourcing is an important research topic because it shows the crowd’s effort and quantity of contribution. Our study is one of the first attempts to include individuals’ personality differences under different payment scenarios in the incentive mechanisms. The personality insights give a better understanding of individuals’ effort and online productivity. Firstly, our experimental study confirms the existing literature by revealing that intrinsically motivated participants perform worse in online labour markets. But when bonus payments are introduced to the experiments, intrinsically motivated participants seem to respond more efficiently in several working outcomes. We extended our analysis by embedding interaction terms with personality traits in the predication models. The findings show that, intrinsic individuals, having high levels of conscientiousness and agreeableness and being within the performance-pay condition (i.e. treatment group), appear to improve their online working behaviour.
Hence, we believe that our findings are crucial inputs to the growing debate on the role of ICT through crowdsourcing technologies in socio-economic development (Ipeirotis and Horton Citation2011; Palvia, Baqir, and Nemati Citation2018). Hence, we need an in-depth understanding of the underlying motivation mechanisms of participating and working in online environments. Moreover, the aim of this article is also to lay a conceptual foundation for future research on the design of personalised task recommendation matching mechanisms that assist individuals (i.e. workers) in finding suitable tasks and thus create benefits for both contributors and requesters and algorithms that will monitor and accommodate the online market needs (Möhlmann et al. Citation2021). A more efficient crowdsourcing adoption from an organisation’s perspective, in terms of i.e. quality of output, reducing risk, problem solving and organisational core competences, will elaborate on the strength of adopting crowdsourcing as a business strategy and will make it a useful innovation partner (Boudreau and Lakhani Citation2013; Jespersen Citation2018). Lastly, following the unique challenges of our time, online labour markets can be seen as a way of minimising socio-economic inequities within populations, by offering the opportunity to economies to reopen. Several crowdsourcing ideas from developing nations could point us towards simple, low-tech, inexpensive solutions that might be just what the future needs.
7.3 Limitations and future research
We have attempted to understand how individuals’ psychological mechanisms can develop further aspects of labour crowdsourcing, by conducting an experiment on an ICT platform. Despite the contributions of this study, some limitations need to be acknowledged. Firstly, while experimental studies in AMT crowdsourcing platform have high internal and external validity, we cannot extend our findings to the generalised real labour market. Our data was from US citizens only with particular cognitive characteristics (Ipeirotis Citation2010; Ross et al. Citation2009). This means that the study offers quite a narrow perspective on the global phenomenon of OLMs as it lacks, for instance, international, cross-cultural, and global viewpoints. All these limits the global generalizability of the results. Secondly, although the Big Five personality traits are stable indexes for adolescents and working-age adults, they do not provide compelling causal explanations for human behaviour (Cobb-Clark and Schurer Citation2012; Rantanen et al. Citation2007). Hence, the Big Five may be viewed as one important model in personality studies but not the integrative model of personality.
Hence, to achieve generalizability in terms of the effects of worker psychology on their online productivity and understand their contextual behaviour in depth, it still needs to be tested by analysing other psychological aspects (e.g. self-esteem, mood, emotions, etc.), under various crowdsourcing initiatives in a similar setting (Goodman and Paolacci Citation2017). A second issue is further studying the nature of online labour markets in future studies aiming to dynamically conceptualise the behavioural tension and psychological dimensions of online workers along with the ICT aspects of crowdsourcing, for personalised recommendation ICT agents to further develop online labour process (Sutherland and Jarrahi Citation2018; Zhao and Zhu Citation2014). For example, an automated recruitment match with worker-job recommendations for crowdsourcing online jobs, based on the correlations between task characteristics and personality traits of potential workers (Geiger and Schader Citation2014; Moayedikia, Ghaderi, and Yeoh Citation2020).
8. Conclusions
With the rise and proliferation of online labour markets, newly emerging business models, such as crowdsourcing has greatly shaped the way people work, by offering the ability to co-create via an increasingly connected world. However, attracting and retaining a necessary number of high-quality workers is often regarded as the first and the most important prerequisite for the success of the crowdsourcing procedure. By integrating self-determination and psychological trait theory, this study presents an initial attempt to investigate and understand deeper the incentive mechanisms and personality traits that trigger people to produce high-quality of work. The results revealed that, workers being driven by intrinsic incentives, have higher productivity outcomes, when bonus rewards are adopted. Another interesting finding is that, mainly, the personality-orientated factors of conscientiousness and agreeableness can also partially explain the increase of productivity levels. This study also provides some major implications. First and foremost, it shows that the effect of incentive schemes hinges on specific traits and preferences of the workforce. In this case stronger investments in the screening of online workers with respect to their motivation for the respective task should become more important. Future research should continue to enrich this line of research by considering other forms of crowdsourcing communities and personality-targeted experimental design approaches.
Disclosure statement
No potential conflict of interest was reported by the author(s).
Notes
1 For example, Fama (Citation1991) discusses payment based on measured time interval, while Baker (Citation1992) and Lazear (Citation1995) have a detailed investigation on defining the measurement of productivity outcomes.
2 Coupled with the condition that the comparisons do not involve several other heterogeneity factors that vary across groups.
3 A respectable number of social researchers suggest that a theoretical possibility exists, in which the motivation may be affected when a previously non-monetary relationship is transformed into an explicitly monetary one.
4 In their study, the treatment was a proposed alternative payment scheme consisting of a combination of a fixed and commission payment.
5 On Amazon Mechanical Turk, the posted jobs are known as Human Intelligent Tasks (HITs).
6 Overall, 25 participants across all conditions failed the main attention check. We dropped them from the final data set and excluded them from the analysis.
7 At the end of an AMT experiment, the experimenter can either approve or reject a participant. Rejection generally happens when a participant tries to submit the survey using a fake completion code. This could happen, for example, because the participant has failed the comprehension questions and did not receive a completion code. However, AMT allows experimenters to decide the minimum approval rate of participants to be recruited.
8 Total cost of the experiment was 654 $ (i.e 514 $*(initial flat rate) + 31$(bonus piece rate) +109$ (ATM 20%) and the experiment ran for 3 h.
9 This was to account for eliminated participants across our five conditions based on the attention check.
10 Openness refers to the tendency to be creative and unconventional, Conscientiousness to the tendency to be organized and disciplined, Extraversion to the tendency to be sociable and active, Agreeableness to the tendency to be trusting and modest and Neuroticism to the tendency to experience negative emotions.
11 As we know, the power of cognitive ability in predicting economic success and productivity is well documented (Bowles, Gintis, and Osborne Citation2001a; Borghans et al. Citation2008)
12 Richter, T., Naumann, J., and Groeben, N., 2001. Das Inventar zur Computerbildung (INCOBI).
13 Terman, Lewis M. (1916). The Measurement of Intelligence: An Explanation of and a Complete Guide for the Use of the Stanford Revision and Extension of the Binet-Simon Intelligence Scale. Boston, Houghton Mifflin Co.
14 Ipeirotis found that Turkers are slightly younger and consist of females with a slightly lower income than the general USA population.
References
- Arechar, A. A., S. Gächter, and L. Molleman. 2018. “Conducting Interactive Experiments Online.” Experimental Economics 21 (1): 99–131. https://doi.org/10.1007/s10683-017-9527-2.
- Autor, H. D. 2001. “Wiring the Labour Market.” Journal of Economic Perspectives 15 (1): 25–40. https://doi.org/10.1257/jep.15.1.25.
- Baard, P. P., E. L. Deci, and R. M. Ryan. 2004. “Intrinsic Need Satisfaction: A Motivational Basis of Performance and Well-Being in two Work Settings 1.” Journal of Applied Social Psychology 34 (10): 2045–2068. https://doi.org/10.1111/j.1559-1816.2004.tb02690.x.
- Badura, K. L., E. Grijalva, B. M. Galvin, B. P. Owens, and D. L. Joseph. 2020. “Motivation to Lead: A Meta-Analysis and Distal-Proximal Model of Motivation and Leadership.” Journal of Applied Psychology 105 (4): 331. https://doi.org/10.1037/apl0000439.
- Baker, George. 1992. “Incentive Contracts and Performance Measurement.” Journal of Political Economy 100 (3): 598–614. https://doi.org/10.1086/261831.
- Banfi, S., and B. Villena-Roldan. 2019. “Do High-Wage Jobs Attract More Applicants? Directed Search Evidence from the Online Labor Market.” Journal of Labor Economics 37 (3): 715–746. https://doi.org/10.1086/702627.
- Barigozzi, F., and N. Burani. 2016. “Screening Workers for Ability and Motivation.” Oxford Economic Papers 68 (2): 627–650. https://doi.org/10.1093/oep/gpw005.
- Barigozzi, F., N. Burani, and D. Raggi. 2018. “Productivity Crowding-out in Labor Markets with Motivated Workers.” Journal of Economic Behavior & Organization 151: 199–218. https://doi.org/10.1016/j.jebo.2018.03.018.
- Barrick, Murray R, and Michael K Mount. 1991. “The Big Five Personality Dimensions and Job Performance: A Meta-Analysis.” Personnel Psychology 44 (1): 1–26. https://doi.org/10.1111/j.1744-6570.1991.tb00688.x.
- Beck, S., T. M. Brasseur, M. Poetz, and H. Sauermann. 2022. “Crowdsourcing Research Questions in Science.” Research Policy 51 (4): 104491. https://doi.org/10.1016/j.respol.2022.104491.
- Benabou, R., and J. Tirole. 2003. “Intrinsic and Extrinsic Motivation.” The Review of Economic Studies 70 (3): 489–520. https://doi.org/10.1111/1467-937X.00253.
- Borghans, Lex, Angela L. Duckworth, James J. Heckman, and Bas ter Weel. 2008. “The Economics and Psychology of Personality Traits.” Journal of Human Resources 43 (4): 972–1059. https://doi.org/10.1353/jhr.2008.0017.
- Boudreau, K. J., and K. R. Lakhani. 2013. “Using the Crowd as an Innovation Partner.” Harvard Business Review 91 (4): 60–69.
- Bowles, Samuel, Herbert Gintis, and Melissa Osborne. 2001. “Incentive-Enhancing Preferences: Personality, Behavior, and Earnings.” American Economic Review 91 (2): 155–158. https://doi.org/10.1257/aer.91.2.155.
- Bradler, C., S. Neckermann, and A. J. Warnke. 2019. “Incentivizing Creativity: A Large-Scale Experiment with Performance Bonuses and Gifts.” Journal of Labor Economics 37 (3): 793–851. https://doi.org/10.1086/702649.
- Brynjolfsson, E., Y. Hu, and M. D. Smith. 2003. “Consumer Surplus in the Digital Economy: Estimating the Value of Increased Product Variety at Online Booksellers.” Management Science 49 (11): 1580–1596. https://doi.org/10.1287/mnsc.49.11.1580.20580.
- Burks, S. V., C. Lewis, P. A. Kivi, A. Wiener, J. E. Anderson, L. Götte, and A. Rustichini. 2015. “Cognitive Skills, Personality, and Economic Preferences in Collegiate Success.” Journal of Economic Behavior & Organization 115: 30–44. https://doi.org/10.1016/j.jebo.2015.01.007.
- Butschek, S., R. G. Amor, P. Kampkötter, and D. Sliwka. 2022. “Motivating gig Workers–Evidence from a Field Experiment.” Labour Economics 75: 102105. https://doi.org/10.1016/j.labeco.2021.102105.
- Cadsby, C. B., F. Song, and N. Zubanov. 2019. “The “Sales Agent” Problem: Effort/Leisure Allocation Under Performance pay as Behavior Towards Risk.” Economic Inquiry 57 (4): 1997–2016. https://doi.org/10.1111/ecin.12821.
- Cantarella, M., and C. Strozzi. 2022. “Workers in the Crowd: The Labor Market Impact of the Online Platform Economy.” Industrial and Corporate Change 30 (6): 1429–1458. https://doi.org/10.1093/icc/dtab022.
- Cassar, L., and S. Meier. 2018. “Nonmonetary Incentives and the Implications of Work as a Source of Meaning.” Journal of Economic Perspectives 32 (3): 215–238. https://doi.org/10.1257/jep.32.3.215.
- Cerasoli, C. P., J. M. Nicklin, and M. T. Ford. 2014. “Intrinsic Motivation and Extrinsic Incentives Jointly Predict Performance: A 40-Year Meta-Analysis.” Psychological Bulletin 140 (4): 980. https://doi.org/10.1037/a0035661.
- Chandler, D., and A. Kapelner. 2013. “Breaking Monotony with Meaning: Motivation in Crowdsourcing Markets.” Journal of Economic Behavior & Organization 90: 123–133. https://doi.org/10.1016/j.jebo.2013.03.003.
- Chen, D. L., and J. J. Horton. 2016. “Research Note—Are Online Labor Markets Spot Markets for Tasks? A Field Experiment on the Behavioral Response to Wage Cuts.” Information Systems Research 27 (2): 403–423.
- Cobb-Clark, D. A., and S. Schurer. 2012. “The Stability of big-Five Personality Traits.” Economics Letters 115 (1): 11–15. https://doi.org/10.1016/j.econlet.2011.11.015.
- Colman, D. E., J. Vineyard, and T. D. Letzring. 2018. “Exploring Beyond Simple Demographic Variables: Differences Between Traditional Laboratory Samples and Crowdsourced Online Samples on the Big Five Personality Traits.” Personality and Individual Differences 133: 41–46. https://doi.org/10.1016/j.paid.2017.06.023.
- Coppock, A. 2019. “Generalizing from Survey Experiments Conducted on Mechanical Turk: A Replication Approach.” Political Science Research and Methods 7 (3): 613–628. https://doi.org/10.1017/psrm.2018.10.
- Costa, P. T., and R. R. McCrae. 1992. “Four Ways Five Factors are Basic.” Personality and Individual Differences 13 (6): 653–665. https://doi.org/10.1016/0191-8869(92)90236-I.
- Cubel, M., A. Nuevo-Chiquero, S. Sanchez-Pages, and M. Vidal-Fernandez. 2016. “Do Personality Traits Affect Productivity? Evidence from the Laboratory.” The Economic Journal 126 (592): 654–681. https://doi.org/10.1111/ecoj.12373.
- Cui, L., Y. Hou, Y. Liu, and L. Zhang. 2021. “Text Mining to Explore the Influencing Factors of Sharing Economy Driven Digital Platforms to Promote Social and Economic Development.” Information Technology for Development 27 (4): 779–801.
- Cunyat, A. 2019. “Crowding-out Effect and Sorting in Competitive Labour Markets with Motivated Workers.” Applied Economics Letters 26 (4): 326–330. https://doi.org/10.1080/13504851.2018.1468550.
- Delfgaauw, J., R. Dur, A. Non, and W. Verbeke. 2014. “Dynamic Incentive Effects of Relative Performance pay: A Field Experiment.” Labour Economics 28: 1–13. https://doi.org/10.1016/j.labeco.2014.02.003.
- Difallah, D. E., M. Catasta, G. Demartini, P. G. Ipeirotis, and P. Cudré-Mauroux. 2015. “The Dynamics of Micro-Task Crowdsourcing: The Case of Amazon Mturk.” In Proceedings of the 24th International Conference on World Wide Web, 238–247. https://doi.org/10.1145/2736277.2741685
- Dohmen, T., and A. Falk. 2011. “Performance pay and Multidimensional Sorting: Productivity, Preferences, and Gender.” American Economic Review 101 (2): 556–590. https://doi.org/10.1257/aer.101.2.556.
- Donato, K., G. Miller, M. Mohanan, Y. Truskinovsky, and M. Vera-Hernandez. 2017. “Personality Traits and Performance Contracts: Evidence from a Field Experiment among Maternity Care Providers in India.” American Economic Review 107 (5): 506–510. https://doi.org/10.1257/aer.p20171105.
- Drago, F. 2011. “Self-esteem and Earnings.” Journal of Economic Psychology 32 (3): 480–488. https://doi.org/10.1016/j.joep.2011.03.015.
- Dube, A., J. Jacobs, S. Naidu, and S. Suri. 2020. “Monopsony in Online Labor Markets.” American Economic Review: Insights 2 (1): 33–46. https://doi.org/10.1257/aeri.20180150.
- Faberman, J., and M. Kudlyak. 2016. “What Does Online job Search Tell us About the Labor Market?” Economic Perspectives 40 (1): 1–15.
- Falk, A., and J. J. Heckman. 2009. “Lab Experiments are a Major Source of Knowledge in the Social Sciences.” Science 326 (5952): 535–538.
- Fama, Eugene F. 1991. “Time, Salary, and Incentive Payoffs in Labor Contracts.” Journal of Labor Economics 9 (1): 25–44. https://doi.org/10.1086/298257.
- Faradani, S., B. Hartmann, and P. G. Ipeirotis. 2011. “What’s the Right Price? Pricing Tasks for Finishing on Time.” In Workshops at the Twenty-Fifth AAAI Conference on Artificial Intelligence.
- Farrell, A. M., J. H. Grenier, and J. Leiby. 2017. “Scoundrels or Stars? Theory and Evidence on the Quality of Workers in Online Labor Markets.” The Accounting Review 92 (1): 93–114. https://doi.org/10.2308/accr-51447.
- Filiz-Ozbay, Emel., John C. Ham, John H. Kagel, and Erkut Y. Ozbay. 2018. “The Role of Cognitive Ability, Personality Traits and Gender in Gift Exchange Outcomes.” Experimental Economics 21 (3): 650–672. https://doi.org/10.1007/s10683-016-9503-2.
- Fischbacher, U. 2007. “z-Tree: Zurich Toolbox for Ready-Made Economic Experiments.” Experimental Economics 10 (2): 171–178. https://doi.org/10.1007/s10683-006-9159-4.
- Fletcher, J. M. 2013. “The Effects of Personality Traits on Adult Labor Market Outcomes: Evidence from Siblings.” Journal of Economic Behavior & Organization 89: 122–135. https://doi.org/10.1016/j.jebo.2013.02.004.
- Franceschelli, I., S. Galiani, and E. Gulmez. 2010. “Performance pay and Productivity of low-and High-Ability Workers.” Labour Economics 17 (2): 317–322. https://doi.org/10.1016/j.labeco.2009.04.006.
- Frey, B. S., and R. Jegen. 2001. “Motivation Crowding Theory.” Journal of Economic Surveys 15 (5): 589–611. https://doi.org/10.1111/1467-6419.00150.
- Gagne, M., E. L. Deci, and R. M. Ryan. 2017. “Self-determination Theory Applied to Work Motivation and Organizational Behavior.” In Handbook of Industrial, Work & Organizational Psychology. Volume 2: Organizational Psychology, Vol. 2, 97–121. SAGE.
- Geiger, D., and M. Schader. 2014. “Personalized Task Recommendation in Crowdsourcing Information Systems—Current State of the art.” Decision Support Systems 65: 3–16. https://doi.org/10.1016/j.dss.2014.05.007.
- Gensowski, M. 2018. “Personality, IQ, and Lifetime Earnings.” Labour Economics 51: 170–183. https://doi.org/10.1016/j.labeco.2017.12.004.
- Gill, D., and V. Prowse. 2016. “Cognitive Ability, Character Skills, and Learning to Play Equilibrium: A Level-k Analysis.” Journal of Political Economy 124 (6): 1619–1676. https://doi.org/10.1086/688849.
- Goncalves, J., S. Hosio, J. Rogstadius, E. Karapanos, and V. Kostakos. 2015. “Motivating Participation and Improving Quality of Contribution in Ubiquitous Crowdsourcing.” Computer Networks 90: 34–48. https://doi.org/10.1016/j.comnet.2015.07.002.
- Gonen, R., D. Raban, C. Brady, and M. Mazor. 2014. “Increased Efficiency Through Pricing in Online Labor Markets.” Journal of Electronic Commerce Research 15 (1): 58.
- Gong, Y. 2017. “Estimating Participants for Knowledge-Intensive Tasks in a Network of Crowdsourcing Marketplaces.” Information Systems Frontiers 19 (2): 301–319. https://doi.org/10.1007/s10796-016-9674-6.
- Goodman, J. K., and G. Paolacci. 2017. “Crowdsourcing Consumer Research.” Journal of Consumer Research 44 (1): 196–210. https://doi.org/10.1093/jcr/ucx047.
- Hagger, M. S., and N. L. Chatzisarantis. 2011. “Causality Orientations Moderate the Undermining Effect of Rewards on Intrinsic Motivation.” Journal of Experimental Social Psychology 47 (2): 485–489. https://doi.org/10.1016/j.jesp.2010.10.010.
- Harvey, J., A. Smith, and D. Golightly. 2017. “Giving and Sharing in the Computer-Mediated Economy.” Journal of Consumer Behaviour 16 (4): 363–371. https://doi.org/10.1002/cb.1499.
- He, S., L. Qiu, and X. Cheng. 2022. “Surge Pricing and Short-Term Wage Elasticity of Labor Supply in Real-Time Ridesharing Markets.” MIS Quarterly 46 (1).
- Heckman, J. J., T. Jagelka, and T. Kautz. 2021. Some Contributions of Economics to the Study of Personality. The Guilford Press.
- Heckman, J., and Yona Rubinstein. 2001. “The Importance of Noncognitive Skills: Lessons from the GED Testing Program.” American Economic Review, 145–149. https://doi.org/10.1257/aer.91.2.145.
- Hendijani, R., D. P. Bischak, J. Arvai, and S. Dugar. 2016. “Intrinsic Motivation, External Reward, and Their Effect on Overall Motivation and Performance.” Human Performance 29 (4): 251–274. https://doi.org/10.1080/08959285.2016.1157595.
- Hogan, J., and B. Holland. 2003. “Using Theory to Evaluate Personality and job-Performance Relations: A Socioanalytic Perspective.” Journal of Applied Psychology 88 (1): 100–112. https://doi.org/10.1037/0021-9010.88.1.100.
- Horton, J. J., and L. B. Chilton. 2010. “The Labor Economics of Paid Crowdsourcing.” In Proceedings of the 11th ACM Conference on Electronic Commerce, 209–218.
- Horton, J. J., D. G. Rand, and R. J. Zeckhauser. 2011. “The Online Laboratory: Conducting Experiments in a Real Labor Market.” Experimental Economics 14 (3): 399–425. https://doi.org/10.1007/s10683-011-9273-9.
- Howe, J. 2008. Crowdsourcing: How the Power of the Crowd Is Driving the Future of Business. New York: Random House.
- Hua, Y., S. Ma, Y. Wang, and Q. Wan. 2017. “To Reward or Develop Identification in Online Brand Communities: Evidence from Emerging Markets.” Information Technology for Development 23 (3): 579–596. https://doi.org/10.1080/02681102.2017.1311831.
- Huffman, D., and M. Bognanno. 2018. “High-powered Performance pay and Crowding out of Nonmonetary Motives.” Management Science 64 (10): 4669–4680. https://doi.org/10.1287/mnsc.2017.2846.
- Ipeirotis, P. G. 2010. Demographics of Mechanical Turk.
- Ipeirotis, P. G., and J. J. Horton. 2011. “The Need for Standardization in Crowdsourcing.” In Proceedings of the Workshop on Crowdsourcing and Human Computation at CHI.
- Jespersen, K. R. 2018. “Crowdsourcing Design Decisions for Optimal Integration Into the Company Innovation System.” Decision Support Systems 115: 52–63. https://doi.org/10.1016/j.dss.2018.09.005.
- Kazai, G., J. Kamps, and N. Milic-Frayling. 2011. “Worker Types and Personality Traits in Crowdsourcing Relevance Labels.” In Proceedings of the 20th ACM International Conference on Information and Knowledge Management, 1941–1944.
- Kazai, G., J. Kamps, and N. Milic-Frayling. 2012. “The Face of Quality in Crowdsourcing Relevance Labels: Demographics, Personality and Labeling Accuracy.” In Proceedings of the 21st ACM International Conference on Information and Knowledge Management, 2583-2586. ACM.
- Kim, J. Y. 2020. “Exploring Perceptional Typology of Social Media Quitters and Associations among Self-Esteem, Personality, and Motivation.” Behaviour & Information Technology, 1–14.
- Kingsley, S. C., M. L. Gray, and S. Suri. 2015. “Accounting for Market Frictions and Power Asymmetries in Online Labor Markets.” Policy & Internet 7 (4): 383–400. https://doi.org/10.1002/poi3.111.
- Kittur, A., E. H. Chi, and B. Suh. 2008, April. “Crowdsourcing User Studies with Mechanical Turk.” In Proceedings of the SIGCHI Conference on Human Factors in Computing Systems (pp. 453–456). ACM.
- Kreps, D. M. 1997. “Intrinsic Motivation and Extrinsic Incentives.” The American Economic Review 87 (2): 359–364.
- Kuvaas, B., R. Buch, A. Weibel, A. Dysvik, and C. G. Nerstad. 2017. “Do Intrinsic and Extrinsic Motivation Relate Differently to Employee Outcomes?” Journal of Economic Psychology 61: 244–258. https://doi.org/10.1016/j.joep.2017.05.004.
- Larkin, I., and S. Leider. 2012. “Incentive Schemes, Sorting, and Behavioral Biases of Employees: Experimental Evidence.” American Economic Journal: Microeconomics 4 (2): 184–214. https://doi.org/10.1257/mic.4.2.184.
- Lazear, E. P. 1992. “Compensation, Productivity, and the new Economics of Personnel.” Research Frontiers in Industrial Relations and Human Resources, 341–380.
- Lazear, E. P. 1995. Personnel Economics. Cambridge, MA: MIT Press.
- Lazear, E. P. 2000. “Performance pay and Productivity.” American Economic Review 90 (5): 1346–1361. https://doi.org/10.1257/aer.90.5.1346.
- Li, J., Y. Wang, D. Yu, and C. Liu. 2022. “Solvers’ Committed Resources in Crowdsourcing Marketplace: Do Task Design Characteristics Matter?” Behaviour & Information Technology 41 (8): 1689–1708. https://doi.org/10.1080/0144929X.2021.1895320.
- Liang, H., M. M. Wang, J. J. Wang, and Y. Xue. 2018. “How Intrinsic Motivation and Extrinsic Incentives Affect Task Effort in Crowdsourcing Contests: A Mediated Moderation Model.” Computers in Human Behavior 81: 168–176. https://doi.org/10.1016/j.chb.2017.11.040.
- Lindqvist, E., and R. Vestman. 2011. “The Labor Market Returns to Cognitive and non-Cognitive Ability: Evidence from the Swedish Enlistment.” American Economic Journal: Applied Economics 3 (1): 101–128. https://doi.org/10.1257/app.3.1.101.
- Litman, L., and J. Robinson. 2020. Conducting Online Research on Amazon Mechanical Turk and Beyond. Sage Publications.
- Martinez, M. G. 2017. “Inspiring Crowdsourcing Communities to Create Novel Solutions: Competition Design and the Mediating Role of Trust.” Technological Forecasting and Social Change 117: 296–304. https://doi.org/10.1016/j.techfore.2016.11.015.
- McCrae, R. R., and P. T. Costa Jr. 1999. “A Five-Factor Theory of Personality.” Handbook of Personality: Theory and Research 2: 139–153.
- McIlroy, D., K. Poole, ÖF Ursavas, and A. Moriarty. 2015. “Distal and Proximal Associates of Academic Performance at Secondary Level: A Mediation Model of Personality and Self-Efficacy.” Learning and Individual Differences 38: 1–9. https://doi.org/10.1016/j.lindif.2015.01.004.
- Moayedikia, A., H. Ghaderi, and W. Yeoh. 2020. “Optimizing Microtask Assignment on Crowdsourcing Platforms Using Markov Chain Monte Carlo.” Decision Support Systems 139: 113404. https://doi.org/10.1016/j.dss.2020.113404.
- Mourelatos, E., N. Giannakopoulos, and M. Tzagarakis. 2022. “Personality Traits and Performance in Online Labour Markets.” Behaviour & Information Technology 41 (3): 468–484. https://doi.org/10.1080/0144929X.2020.1815840.
- Mourelatos, E., and M. Tzagarakis. 2016. “Worker’s Cognitive Abilities and Personality Traits as Predictors of Effective Task Performance in Crowdsourcing Tasks.” In Proceedings of 5th ISCA/DEGA Workshop on Perceptual Quality of Systems (PQS 2016), 112–116. https://doi.org/10.21437/PQS.2016-24
- Mourelatos, E., and M. Tzagarakis. 2018. “An Investigation of Factors Affecting the Visits of Online Crowdsourcing and Labor Platforms.” NETNOMICS: Economic Research and Electronic Networking 19 (3): 95–130. https://doi.org/10.1007/s11066-018-9128-z.
- Möhlmann, M., L. Zalmanson, O. Henfridsson, and R. W. Gregory. 2021. “algorithmic Management of Work on Online Labor Platforms: When Matching Meets Control.” MIS Quarterly 45(4).
- Mueller-Langer, F., and E. Gomez-Herrera. 2022. “Mobility Restrictions and the Substitution Between on-Site and Remote Work: Empirical Evidence from a European Online Labour Market.” Information Economics and Policy 58: 100951. https://doi.org/10.1016/j.infoecopol.2021.100951.
- Müller, J., and C. Schwieren. 2012. What can the Big Five Personality Factors contribute to explain Small-Scale Economic Behavior? (No. 12-028/1). Tinbergen Institute.
- Niederle, Muriel, and Lise Vesterlund. 2007. “Do Women shy Away from Competition? Do men Compete Too Much?” The Quarterly Journal of Economics 122 (3): 1067–1101. https://doi.org/10.1162/qjec.122.3.1067.
- Nyhus, E. K., and E. Pons. 2005. “The Effects of Personality on Earnings.” Journal of Economic Psychology 26 (3): 363–384. https://doi.org/10.1016/j.joep.2004.07.001.
- Palvia, P., N. Baqir, and H. Nemati. 2018. “ICT for Socio-Economic Development: A Citizens’ Perspective.” Information & Management 55 (2): 160–176. https://doi.org/10.1016/j.im.2017.05.003.
- Paolacci, G., and J. Chandler. 2014. “Inside the Turk: Understanding Mechanical Turk as a Participant Pool.” Current Directions in Psychological Science 23 (3): 184–188. https://doi.org/10.1177/0963721414531598.
- Paolacci, G., J. Chandler, and P. G. Ipeirotis. 2010. “Running Experiments on Amazon Mechanical Turk.” Judgment and Decision Making 5 (5): 411–419. https://doi.org/10.1017/S1930297500002205.
- Pee, L. G., E. Koh, and M. Goh. 2018. “Trait Motivations of Crowdsourcing and Task Choice: A Distal-Proximal Perspective.” International Journal of Information Management 40: 28–41. https://doi.org/10.1016/j.ijinfomgt.2018.01.008.
- Peer, E., J. Vosgerau, and A. Acquisti. 2014. “Reputation as a Sufficient Condition for Data Quality on Amazon Mechanical Turk.” Behavior Research Methods 46 (4): 1023–1031. https://doi.org/10.3758/s13428-013-0434-y.
- Randhawa, K., R. Wilden, and J. West. 2019. “Crowdsourcing Without Profit: The Role of the Seeker in Open Social Innovation.” R&d Management 49 (3): 298–317. https://doi.org/10.1111/radm.12357.
- Rantanen, J., R. L. Metsäpelto, T. Feldt, L. E. A. Pulkkinen, and K. Kokko. 2007. “Long-Term Stability in the Big Five Personality Traits in Adulthood.” Scandinavian Journal of Psychology 48 (6): 511–518. https://doi.org/10.1111/j.1467-9450.2007.00609.x.
- Relich, M. 2017. “The Impact of ICT on Labor Productivity in the EU.” Information Technology for Development 23 (4): 706–722. https://doi.org/10.1080/02681102.2017.1336071.
- Roberts, B. W. 2009. “Back to the Future: Personality and Assessment and Personality Development.” Journal of Research in Personality 43 (2): 137–145. https://doi.org/10.1016/j.jrp.2008.12.015.
- Roberts, B. W., and W. F. DelVecchio. 2000. “The Rank-Order Consistency of Personality Traits from Childhood to old age: A Quantitative Review of Longitudinal Studies.” Psychological Bulletin 126 (1): 3. https://doi.org/10.1037/0033-2909.126.1.3.
- Rogstadius, J., V. Kostakos, A. Kittur, B. Smus, J. Laredo, and M. Vukovic. 2011. “An Assessment of Intrinsic and Extrinsic Motivation on Task Performance in Crowdsourcing Markets.” In Proceedings of the International AAAI Conference on Web and Social Media, Vol. 5(1).
- Ross, J., A. Zaldivar, L. Irani, and B. Tomlinson. 2009. Who are the Turkers? Worker Demographics In Amazon Mechanical Turk. Department of Informatics, University of California, Irvine, USA, Tech. Rep.
- Rustichini, A., C. G. DeYoung, J. E. Anderson, and S. V. Burks. 2016. “Toward the Integration of Personality Theory and Decision Theory in Explaining Economic Behavior: An Experimental Investigation.” Journal of Behavioral and Experimental Economics 64: 122–137. https://doi.org/10.1016/j.socec.2016.04.019.
- Ryan, R. M., and E. L. Deci. 2017. Self-Determination Theory. Basic Psychological Needs in Motivation, Development, and Wellness.
- Ryan, R. M., W. S. Ryan, S. I. Di Domenico, and E. L. Deci. 2019. The Nature and the Conditions of Human Autonomy And Flourishing: Self-Determination Theory and Basic Psychological Needs.
- Salgado, Jesus F. 1997. “The Five Factor Model of Personality and job Performance in the European Community..” Journal of Applied Psychology 82 (1): 30–43. https://doi.org/10.1037/0021-9010.82.1.30.
- Sansone, C., and J. M. Harackiewicz, eds. 2000. Intrinsic and Extrinsic Motivation: The Search for Optimal Motivation and Performance. Elsevier.
- Shi, X., R. Evans, W. Pan, and W. Shan. 2022. “Understanding the Effects of Personality Traits on Solver Engagement in Crowd Sourcing Communities: a Moderated Mediation Investigation.” Information Technology & People 35 (2): 750–780.
- Stewart, G. L. 1996. “Reward Structure as a Moderator of the Relationship Between Extraversion and Sales Performance.” Journal of Applied Psychology 81 (6): 619–627. https://doi.org/10.1037/0021-9010.81.6.619.
- Straub, T., H. Gimpel, F. Teschner, and C. Weinhardt. 2015. “How (not) to Incent Crowd Workers.” Business & Information Systems Engineering 57 (3): 167–179. https://doi.org/10.1007/s12599-015-0384-2.
- Sutherland, W., and M. H. Jarrahi. 2018. “The Sharing Economy and Digital Platforms: A Review and Research Agenda.” International Journal of Information Management 43: 328–341. https://doi.org/10.1016/j.ijinfomgt.2018.07.004.
- Syverson, C. 2011. “What Determines Productivity?” Journal of Economic Literature 49 (2): 326–365. https://doi.org/10.1257/jel.49.2.326.
- Tauer, J. M., and J. M. Harackiewicz. 2004. “The Effects of Cooperation and Competition on Intrinsic Motivation and Performance.” Journal of Personality and Social Psychology 86 (6): 849–861. https://doi.org/10.1037/0022-3514.86.6.849.
- Tett, Robert P, Douglas N Jackson, and Mitchell Rothstein. 1991. “Personality Measures as Predictors of Job Performance: A Meta-Analytic Review.” Personnel Psychology 44 (4): 703–742. https://doi.org/10.1111/j.1744-6570.1991.tb00696.x.
- Thomas, K. A., and S. Clifford. 2017. “Validity and Mechanical Turk: An Assessment of Exclusion Methods and Interactive Experiments.” Computers in Human Behavior 77: 184–197. https://doi.org/10.1016/j.chb.2017.08.038.
- Witt, L. A., L. A. Burke, M. R. Barrick, and M. K. Mount. 2002. “The Interactive Effects of Conscientiousness and Agreeableness on job Performance.” Journal of Applied Psychology 87 (1): 164–169. https://doi.org/10.1037/0021-9010.87.1.164.
- Woolley, K., and A. Fishbach. 2018. “It’s About Time: Earlier Rewards Increase Intrinsic Motivation.” Journal of Personality and Social Psychology 114 (6): 877–890. https://doi.org/10.1037/pspa0000116.
- Wu, P. F. 2019. “Motivation Crowding in Online Product Reviewing: A Qualitative Study of Amazon Reviewers.” Information & Management 56 (8): 103163. https://doi.org/10.1016/j.im.2019.04.006.
- Ye, H., and A. Kankanhalli. 2015. “Investigating the Antecedents of Organizational Task Crowdsourcing.” Information & Management 52 (1): 98–110. https://doi.org/10.1016/j.im.2014.10.007.
- Zhao, Y., and Q. Zhu. 2014. “Evaluation on Crowdsourcing Research: Current Status and Future Direction.” Information Systems Frontiers 16 (3): 417–434. https://doi.org/10.1007/s10796-012-9350-4.
Appendices
Appendix A
A short note on external validity and generalizability of results
Many studies conduct experiments in Amazon Mechanical Turk, in order to reduce cost, time and inconvenience, as it is currently the most appropriate online labour market for experimentation. Horton, Rand, and Zeckhauser Citation2011 has shown that it is possible to quickly and inexpensively replicate findings from traditional, physical laboratory experiments in the online laboratory, by replicating several well-known experiments conducted in the context of online labour markets. The external validity of these online results relies on the representativeness and quantitative versus qualitative generalizability of the crowd (Kittur et al. 2008; Horton and Chilton Citation2010). It is already known that individuals who choose to participate in social sciences experiments represent a small segment of the population. The same is also true for subjects who work online. Concerning the laboratory experiments, university students, who make up the subjects in most these physical experiments, are highly selected compared to the U.S population, so too are subjects in online experiments.
Our experimental sample is in line with many surveys which have revealed that Amazon U.S-based workers are more likely to be young females with high computer competence (Ipeirotis Citation2010)Footnote14 and suggest that the profile of the typical Turker is not a person that participates in online tasks for a living in a developing country (Ross et al. Citation2009). Moreover, the online population of the AMT remains relatively stable over time and follows the economic circumstances of the real labour USA market (Difallah et al. Citation2015). However, even if the participants of our experiments ‘look like’ population, in terms of observable cognitive and demographic attributes, some degree of self-selection bias is unavoidable. In line with the physical laboratory experiments, issues of ‘who is willing to participate’ in an online task exist, but this matter does not determine the usefulness of such research studies (Falk and Heckman Citation2009).
Appendix B
Singh and Masuku (2014), indicate that the detection of sample size is a challenging procedure for each experimental study, and sufficient sample size is a crucial factor in drawing valid conclusions. Thus, to overcome potential issues regarding validity of our empirical study, we conduced power calculation to determine the sample size for the linear regression models (Dupont & Plummer, 1998). In the sample size calculations, we assume that the sample would have 95% reliability about population and a sampling error of 5%. The calculations show that a sample size of N = 283 participants is required for the exact approach to attain the target power 0.9, with the significance level of 95%. We confirm that the actual sample size of the study is adequate to achieve results with the desired power and significance. For the implementation, we use the new Stata module of samsi_reg (Mander 2019) and the powercal (Newson 2004).
Additional References
Dupont, W. D., & Plummer Jr, W. D. (1998). Power and sample size calculations for studies involving linear regression. Controlled clinical trials, 19(6), 589–601.
Mander, A. (2019). SAMPSI_REG: Stata module to calculate the sample size/power for linear regression.
Newson, R. (2004). Generalized power calculations for generalized linear models and more. The Stata Journal, 4(4), 379–401.
Singh, A. S., & Masuku, M. B. (2014). Sampling techniques & determination of sample size in applied statistics research: An overview. International Journal of economics, commerce and management, 2(11), 1–22.