ABSTRACT
The present study examined a potential mechanism underlying college students’ resisting in-class media multitasking under contextual control from a behavioural economic perspective. Using a hierarchical cluster analysis, we first identified subgroups of students who differed in frequencies of media multitasking across two contexts (preferred and non-preferred classes). We then compared a subgroup of students who could resist media multitasking in one context but not in another context with other subgroups of students in terms of rates of delay discounting and levels of impulse control. In the delay-discounting task, participants made a series of choices between smaller amounts of hypothetical monetary rewards available immediately and a larger amount of hypothetical monetary rewards available after a delay. Impulse control was measured by a self-reported scale. The primary finding was that the subgroup of students who could resist media multitasking in preferred but not non-preferred classes showed significantly lower rates of delay discounting than the subgroup of students who frequently engaged in media multitasking in both preferred and non-preferred classes. Implications for developing effective intervention strategies from a behavioural economic perspective are discussed.
Now more than ever before, our world has transitioned to a virtual format with the advancement of modern technologies. Media multitasking, defined as simultaneously engaging in multiple media activities or engaging in a media activity while engaging in a non-media activity (van der Schuur et al. Citation2015), has become the new norm for our almost constantly online, connected world. As smart devices connect us to the outside world via text messaging and social networking, we are exposed to more distraction as we spend more time on our screens, and multimedia is now being recognised as a distraction inhibiting students’ ability to focus in the classroom. According to a survey conducted in the United States, for example, college students reported that they spent an average of 20.9% of class time using their smart phone for non-educational purposes (McCoy Citation2016). A recent study revealed that each additional hour of phone use per day is associated with a 0.15 point reduction in students’ Grade Point Average (GPA) for the academic term (Sapci et al. Citation2021). Consistent with this finding, several experimental studies have demonstrated that students who engaged in texting during a class lecture scored significantly lower on quizzes assessing lecture content (e.g. Ellis, Daniels, and Jauregui Citation2010; Froese et al. Citation2012; Gingerich and Lineweaver Citation2014; Lee et al. Citation2017).
Since statistics demonstrate that media multitasking has negative academic outcomes, it is essential to analyse the potential predictors of problematic behaviours in the classroom. Previous research has identified various predictors for in-class media multitasking, which include but are not limited to: (a) variables associated with multimedia use outside of the classroom (e.g. Olmsted and Terry Citation2014; Wei and Wang Citation2010), (b) variables associated with professors’ behaviours and students’ attitudes in the classroom (e.g. Bolkan and Griffin Citation2017; Ledbetter and Finn Citation2016), (c) variables associated with students’ personality traits (e.g. Toyama and Hayashi Citation2022; Citation2023b), and (d) variables associated with students’ cognitive abilities (e.g. Hayashi and Nenstiel Citation2021; Wei, Wang, and Klausner Citation2012).
With respect to a potential mechanism underlying excessive in-class media multitasking, a series of previous studies have documented that some behavioural economic variables, such as delay discounting and demand for social interaction, play an important role in college students’ frequent in-class media multitasking. Behavioural economics, as defined by Bickel et al. (Citation2014), applies economic principles to understand individuals’ choices and decisions. From this perspective, in-class media multitasking can be viewed as a choice shaped by competing contingencies involving a trade-off between smaller-sooner rewards (e.g. social interaction from text messaging now) and larger-later rewards (e.g. a better exam grade), and it manifests behaviourally by preference towards the smaller-sooner rewards. This conceptualisation positions the impulsive nature of in-class media multitasking not just as a perspective, but as a crucial aspect in understanding and addressing the problem, which directs the primary focus of research to how to understand and ultimately influence the choice itself (cf. Lamb et al. Citation2016).
Previous research supports the behavioural economic conceptualisation of in-class media multitasking. Hayashi and Blessington (Citation2018) directly tested the validity of the conceptualisation and demonstrated that in-class media multitasking can be characterised as an impulsive choice for smaller-sooner rewards over larger-later rewards. Hayashi (Citation2021) showed that a hallmark of in-class media multitasking – discrepancies between students’ attitudes and behaviours (i.e. students perceive in-class media multitasking as inappropriate yet frequently engage in it anyway) – can be characterised by steeper rates of delay discounting of hypothetical monetary rewards. Finally, Toyama and Hayashi (Citation2023a) showed that excessive valuation of social rewards, as determined by a demand analysis, plays an important role in high frequencies of in-class media multitasking.
It is important to note that while these previous studies utilising behavioural economic approaches have documented some potential mechanisms underlying students’ frequent in-class media multitasking, it is still not clear under what mechanism students who do not frequently engage in in-class media multitasking can resist engaging in it. If such a mechanism is identified successfully, it should be of great use when developing intervention strategies. In addition, given a previous study demonstrating that the relationship between in-class media multitasking and its predictors is moderated by the urgency of the text message received as a contextual variable (Toyama and Hayashi Citation2023b), it is important to further examine how in-class media multitasking interacts with other contextual variables. For example, are there any students who frequently engage in media multitasking in one context but not in another context? If so, under what mechanism can such students resist engaging in media multitasking?
Taken together, the overarching goal of the present study was to examine (a) whether college students’ frequencies of in-class media multitasking differ across contexts, and if so, (b) under what mechanism such differences are generated. More specifically, using a cluster analysis, a statistical technique in which similar cases are grouped together based on selected variables, the present study determined whether there would be distinctive subgroups of college students who differed in terms of their frequencies of media multitasking under two different contexts – preferred and non-preferred classes based on students’ own evaluation (i.e. classes students report liking or not liking). We chose this contextual variable because we hypothesised that positive/negative attitudes towards classes would be associated with frequencies of in-class media multitasking. In addition, assuming that the value of long-term rewards resulting from in-class academic behaviours (e.g. a better exam grade) is higher in preferred than in non-preferred classes, as represented by lower frequencies media multitasking, we attempted to create two contexts that differed in the value of such rewards, hoping to reveal how a potential mechanism of resisting in-class media multitasking (described below) interacts with the contextual variable. Taken together, it was hypothesised that students would engage in media multitasking less frequently in preferred classes and that there would be several distinctive subgroups who differed in frequencies of media multitasking in preferred and non-preferred classes, but we had no specific hypothesis on what kind of subgroups would emerge.
If any subgroups are identified, a next logical step is to examine a possible mechanism under which such subgroups emerged. Particularly, we were interested in whether there would be a subgroup of students who frequently engage in media multitasking in one context yet resist engaging in it in the other context, and if so, under what mechanism they could do so. Based on previous research (Hayashi and Blessington Citation2018; Hayashi and Nenstiel Citation2021) utilising the behavioural economic conceptualisation of in-class media multitasking as an impulsive choice, we explored two possible behavioural mechanisms underlying such a choice. The first one was lesser devaluation of future rewards or lower delay-discounting rates. Delay discounting refers to subjective devaluation of a reward as a function of time to its receipt (Green and Myerson Citation2004). If delay discounting underlies resisting in-class media multitasking, it should mean that students who resist in-class media multitasking can do so because the value of larger-later rewards (e.g. a better exam grade) is discounted less in these students, and goal-directed behaviours controlled by long-term consequences are more likely to occur. The second possible mechanism, on the other hand, is greater impulse (or effortful) control, which refers to the ability to inhibit a dominant response to perform a subdominant response (Rothbart and Bates Citation1988).Footnote1 If impulse control underlies resisting in-class media multitasking, it should mean that students are capable of suppressing the impulsive choice of in-class media multitasking while continuing an academic behaviour, independently of the value of the larger-later rewards associated with the academic behaviour. Because this is an exploratory investigation, we had no hypothesis on which of the two mechanisms is more dominant when college students could resist engaging in in-class media multitasking.
1. Method
1.1. Participants
One-hundred sixty-nine undergraduate students, enrolled in introductory psychology courses at a university in the northeastern United States and predominantly non-psychology majors, participated for course credit. The sample consisted of 97 female and 72 male students, and their mean age and years of higher education were 20.0 (SD = 4.1) and 1.7 (SD = 1.2), respectively. The sample size was determined based on the similar previous studies (Hayashi Citation2023; Hayashi et al. Citation2019).
1.2. Procedure
An online session was hosted by Qualtrics (Provo, UT). An email was sent to participants through the Qualtrics website including a link to the online questionnaire. After agreeing to participate, they completed questionnaires on their demographic information (age, gender, and years of higher education), in-class text-messaging behaviours, and levels of impulse control, as well as a delay-discounting task involving hypothetical monetary rewards. The present study is a part of a larger survey and portions of the present data were reported in different studies with different goals and analyses (e.g., Hayashi and Blessington, Citation2018, Citation2021; Hayashi and Glodowski, Citation2023). The Institutional Review Board at the first author’s affiliated university reviewed the study protocol and deemed the study exempt.
1.2.1. In-class media multitasking
The questionnaire included two questions on frequency of media multitasking in the classroom. One question assessed how often students engage in media multitasking in preferred classes. Another question assessed how often students engage in media multitasking in non-preferred classes. For both questions, a 5-point Likert scale, ranging from 1 (never) to 5 (always), was used (see Supplemental Material for all questionnaires used in this study). Higher scores indicate higher frequencies of in-class media multitasking. Two separate scores for both types of in-class media multitasking were analysed (see Bowling Citation2005, for advantages and disadvantages of a single-item question).
1.2.2. Impulse control
The levels of impulse control or response inhibition were assessed by the Impulse Control subscale of the Executive Function Index (EFI; Spinella Citation2005). The EFI is a self-reported measure of executive function developed with a non-clinical healthy adult population (Spinella Citation2005). The Impulse Control subscale of the EFI was employed in the present study because Hayashi and Nenstiel (Citation2021) demonstrated that only this subscale was a significant predictor of frequent in-class media multitasking. The subscale consists of five items with a 5-point Likert scale ranging from 1 (not at all) to 5 (very much). The scores of negatively worded items are reversed, and higher scores indicate higher levels of impulse control. The Cronbach’s alpha for the present sample was 0.65 (cf. 0.69 in Spinella Citation2005). Two participants did not answer all items in the questionnaire and their data were excluded from the analyses.
1.2.3. Delay-discounting task
The delay-discounting task was adopted from Jones and Rachlin (Citation2009) and modified for the present study. The participants made a series of choices between smaller hypothetical monetary rewards available immediately versus larger hypothetical monetary rewards available after a delay (1 week, 1 month, 6 months, 1 year, or 5 years presented in this order). On each screen, a single delay value was presented, and the participants made 11 choices between two types of rewards (see for a schematic presentation of the task). The smaller-immediate monetary rewards were listed in a column on the left of the screen in descending order ($99, $90, $80, $70, $60, $50, $40, $30, $20, $10, and $1). The larger-later monetary rewards were listed in a column on the right of the screen and always a fixed amount of $100 with one of the five delay periods. For each comparison (e.g. $99 immediately vs. $100 in 1 week), participants made a choice by clicking a radio button next to each reward value.
Indifference points, the points at which the smaller-immediate rewards and the larger-later rewards were equal in their subjective value, were calculated based on the method described in Rachlin, Raineri, and Cross (Citation1991). To evaluate the degree of delay discounting, the model-based area under the curve (AUC) was calculated for each participant based on the methods described in Gilroy and Hantula (Citation2018). In this process, we used Rachlin's (Citation2006) model because of its best fit to the groups’ median indifference points, as assessed by the discounting model selector (Gilroy, Franck, and Hantula Citation2017).Footnote2 Higher AUC values indicate lower rates of delay discounting.
Consistent with previous studies (Smith, Lawyer, and Swift Citation2018), we identified the participants who showed nonsystematic patterns of responding based on the algorithm developed by Johnson and Bickel (Citation2008). Individuals’ data were excluded from analyses if an indifference point was greater than the preceding indifference point by more than 20%. We employed only Criterion 1 of their algorithm because the present study employed 5 years as the largest delay value (as opposed to 25 years in Johnson and Bickel Citation2008). The application of this algorithm identified eight participants (4.7%), and their data were excluded from analyses. This exclusion rate is lower than what is typically observed with college students (see Smith, Lawyer, and Swift Citation2018, for review).
1.3. Data analysis
To determine if there would be distinctive subgroups of students that differed in terms of frequencies of media multitasking in preferred and non-preferred classes, we conducted a hierarchical cluster analysis, which involves a series of sequential steps in which individual cases are merged together one at a time based on their similarities (Greenacre and Primicerio Citation2013). Frequencies of in-class media multitasking in preferred and non-preferred classes were entered into the analysis, with Ward's method as the method of clustering, squared Euclidian distances as the measure of the distance, and z-score conversion as the method of standardisation (Clatworthy et al. Citation2005; Yim and Ramdeen Citation2015). The number of clusters was determined based on the inverse screen technique (Yim and Ramdeen Citation2015).
Once distinct subgroups of students who differed in terms of their frequencies of media multitasking in preferred and non-preferred classes were identified through cluster analysis, the subgroups were then compared on the demographic variables, frequencies of in-class media multitasking, levels of impulse control, and rates of delay discounting. Gender was analysed by a chi-square test. Continuous variables were analysed by a one-way analysis of variable (ANOVA) or by a Welch ANOVA if the assumption of homogeneity of variances, as assessed by Levene's test for equality of variances, was violated. An exception was the ANOVA with frequencies of media multitasking in preferred classes, for which a regular ANOVA was performed because a Welch ANOVA could not be performed due to 0 variance in one subgroup. The post-hoc pairwise comparisons were performed by the Tukey–Kramer test or by the Games-Howell test if the assumption of homogeneity of variances was violated. Lastly, the frequencies of media multitasking between preferred and non-preferred classes were compared for each cluster by the paired-samples t-test. All statistical analyses were performed with SPSS Version 28. The statistical significance level was set at .05.
2. Results
shows the coefficient values of the cluster analysis with frequencies of media multitasking in preferred and non-preferred classes. Based on the visual inspection of the figure, there was a robust increase in coefficient values when transitioning from a four-cluster to a three-cluster model. This pattern indicates that the four-cluster model most accurately represents the data. shows the demographic characteristics of the four clusters. No statistically significant differences across clusters were found for gender, χ2(3) = 1.96, p = .580; age, F(3, 165) = 1.26, p = .291, partial η2 = .022; and years of higher education, F(3, 165) = 0.25, p = .864, partial η2 = .004.
Table 1. Demographic characteristics for all clusters.
shows mean frequencies of media multitasking in preferred and non-preferred classes for all clusters. First, the results of the paired-samples t-test conducted for each cluster revealed that frequencies of media multitasking were significantly higher in non-preferred classes than in preferred classes (p < .001; ), suggesting that the two contexts created here (preferred vs. non-preferred classes) differed in a meaningful manner. Second, the results of the ANOVA revealed the clusters differed significantly on frequencies of media multitasking in preferred classes, F(3, 165) = 209.58, p < .001, partial η2 = .79, as well as in non-preferred classes, F(3, 87.01) = 178.88, p < .001, partial η2 = .77. shows the results of the post-hoc comparisons. For both types of media multitasking, clusters differed significantly at all comparisons (p < .001), except for the one between the High-High and Low-High clusters in non-preferred classes (p = .692). Overall, the results of the cluster analysis revealed clear qualitative differences among the four clusters. Mean frequencies below 2, between 2 and 3, and above 3 in are categorised as low, moderate, and high frequencies, respectively. Using this categorisation, Cluster 1 was characterised by low frequencies of media multitasking in both preferred and non-preferred classes (hereafter referred to as the Low-Low subgroup). Clusters 2 and 3 were characterised by moderate and high frequencies of media multitasking in both types of classes and are referred to as the Moderate-Moderate and High-High subgroups, respectively. Cluster 4 was characterised by low frequencies of media multitasking in preferred classes but high frequencies in non-preferred classes and is referred to as the Low-High subgroup.
Figure 3. Mean frequencies of media multitasking in preferred and non-preferred classes.
Notes: Error bars indicate 95% confidence intervals. C1 = Low-Low subgroup. Cluster 2 = Moderate-Moderate subgroup. Cluster 3 = High-High subgroup. Cluster 4 = Low-High subgroup.
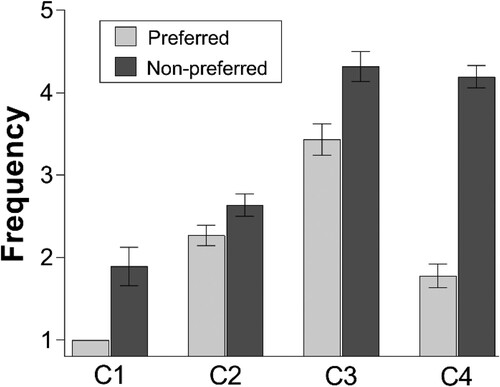
Table 2. Results of the paired-samples t-tests comparing frequencies of media multitasking between preferred and non-preferred classes.
Table 3. Post-hoc comparisons of frequencies of media multitasking in preferred and non-preferred classes among clusters.
shows mean AUC values and scores of impulse control for all clusters. The results of the ANOVA revealed the clusters differed significantly on AUC, F(3, 157) = 4.60, p = .004, partial η2 = .08, and impulse control, F(3, 163) = 9.98, p < .001, partial η2 = .16. shows the results of the post-hoc comparisons. With respect to the post-hoc comparisons on AUC, the Low-High subgroup, who appeared to demonstrate the highest values as determined by visual inspection, significantly differed from the Moderate-Moderate (p = .038) and High-High (p = .002) subgroups, but not from the Low-Low subgroup (p = .075). With respect to impulse control, the Low-Low subgroup, who appeared to demonstrate the highest scores by visual inspection, significantly differed from all other subgroups (p < .01).
Figure 4. Mean area under the curve and scores of impulse control.
Notes: Error bars indicate 95% confidence intervals. C1 = Low-Low subgroup. Cluster 2 = Moderate-Moderate subgroup. Cluster 3 = High-High subgroup. Cluster 4 = Low-High subgroup. *p < .05. **p < .01. ***p < .001.
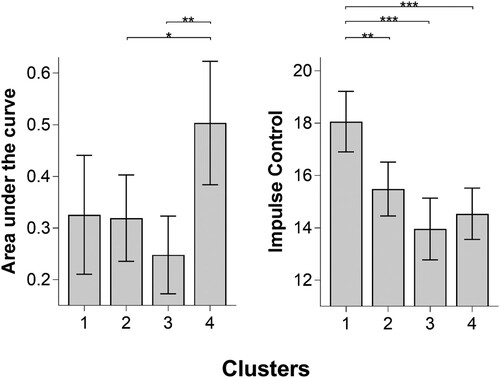
Table 4. Post-hoc comparisons of area under the curve (AUC) and impulse control among clusters.
3. Discussion
Using a hierarchical cluster analysis, the present study determined whether college students could be classified into distinct subgroups that differed in frequencies of media multitasking in preferred and non-preferred classes. The finding of particular interest is the identification of the Low-High subgroup, who infrequently engaged in media multitasking in preferred classes but frequently engaged in it in non-preferred classes. This finding is consistent with previous research demonstrating that the context matters for in-class media multitasking (e.g. Toyama and Hayashi Citation2023b), but it also extends previous research by demonstrating that some students who frequently engage in media multitasking in one context (non-preferred classes) can resist engaging in it in other context (preferred classes).
Subsequent analyses from a behavioural economic perspective revealed that the Low-High subgroup showed significantly lower rates of delay discounting than the Moderate-Moderate and High-High subgroups, but the rates of discounting did not differ significantly between the Low-High and Low-Low subgroups. In terms of identifying a potential underlying mechanism for resisting in-class media multitasking, a comparison of particular interest is between the Low-High and High-High subgroups, who could and could not resist media multitasking in preferred classes, respectively, while both subgroups frequently engaged in media multitasking in non-preferred classes. The fact that these two subgroups differed significantly in rates of delay discounting but not in levels of impulse control suggests that the degree of devaluation of future rewards, as determined by rates of delay discounting, plays an important role in resisting in-class media multitasking in one context but not in the other. More specifically, when the value of larger-later rewards from in-class academic behaviours (e.g. a better exam grade) is high in preferred classes, students with low discounting rates (i.e. greater future-orientation/goal-directedness; Hofmann, Schmeichel, and Baddeley Citation2012) can resist media multitasking because the larger-later rewards, although discounted to some extent, can still compete with the smaller-sooner rewards from media multitasking (e.g. social interaction from text messaging), resulting in the students’ not engaging in media multitasking. Students with high discounting rates (i.e. lesser future-orientation/goal-directedness), on the other hand, cannot resist media multitasking because the larger-later rewards, after discounted greatly, cannot compete with the smaller-sooner rewards, resulting in the students’ engaging in media multitasking. When the value of larger-later rewards from in-class academic behaviours was low in non-preferred classes, however, the lower-valued larger-later rewards, regardless of students’ discounting rates, cannot compete with the smaller-sooner rewards, resulting in both types of students’ engaging in media multitasking. Although previous research has shown that greater delay discounting has been associated with frequent in-class media multitasking in a general context (Hayashi Citation2021; Hayashi and Blessington Citation2018), this is the first study that proposed delay discounting as a potential mechanism of resisting in-class media multitasking under contextual control, and this has important implications for the development of effective intervention strategies (discussed below).
In addition to these primary findings, it is worth noting that the present study also demonstrated that the Low-Low subgroup showed significantly higher levels of impulse control than the other three subgroups. The finding that the AUC values for this subgroup did not differ significantly from other subgroups would suggest that greater impulse control is sufficient for the Low-Low subgroup not to engage in media multitasking all together in both preferred and non-preferred classes. Also, based on the finding that the Low-Low subgroup was the only subgroup who showed low frequencies of media multitasking in non-preferred classes, impulse control, not delay discounting, may be an important mechanism for not engaging in media multitasking in non-preferred classes. For these students, the context of preferred or non-preferred classes does not matter: They do not engage in in-class media multitasking regardless of the value of the larger-later rewards from in-class academic behaviours, presumably because future orientation/goal-directedness does not play an important role in their choice of engaging or not engaging in in-class media multitasking. This also has important implications for the development of effective intervention strategies, which will be discussed below.
3.1. Implications for interventions
The present finding that students who could resist media multitasking in preferred but not non-preferred classes showed lower rates of delay discounting but not higher levels of impulse control indicates that such students’ media multitasking in non-preferred classes may not stem from their lack of impulse control. Rather, it may be under their volitional control, meaning that they may choose to engage in media multitasking in non-preferred classes because the (discounted) value of the larger-later reward is subjectively smaller than the value of the smaller-sooner reward. If this is the case, one intervention strategy may be to reduce the rates of delay discounting of the larger-later reward (i.e. convincing students of the long-term importance of the class). As an example, episodic future thinking, which extends one’s temporal window over which the value of a lager-later outcomes is integrated and thus the salience of such outcomes is enhanced (Stein et al. Citation2016), may be effective. Episodic future thinking can assist students in making future-oriented decisions by allowing the benefits of larger-later outcomes to be felt in the present (Boyer Citation2008). Previous research has documented that engaging in episodic future thinking to pre-experience future events can result in greater choices of larger-later outcomes (e.g. Dassen et al. Citation2016; Sasse et al. Citation2015), suggesting that episodic future thinking, which helps students (re)appreciate the value of their long-term goals (e.g. good GPA), may be a promising approach to reduce media multitasking in non-preferred classes.
It is important to note, however, that episodic future thinking may not be the only strategy for reducing in-class media multitasking. The present finding that students with high levels of impulse control can resist media multitasking in both preferred and non-preferred classes suggests that any strategies to improve students’ impulse control may be promising, particularly to students with high delay-discounting rates (or lesser future-orientation/goal-directedness). A recent meta-analysis revealed that inhibitory control training, in which participants learn to inhibit their urge to engage in an impulsive behaviour (presumably by strengthening rule-governance of the incompatible behaviour; Hayes, Gifford, and Ruckstuhl Citation1996), is effective for controlling various impulse behaviours (Allom, Mullan, and Hagger Citation2016). For example, a previous study reported a computer-based inhibitory control training, which required college students to withhold prepotent responses towards alcohol-related cues, reduced alcohol consumption (Houben et al. Citation2011). Perhaps, similar training may be effective for reducing in-class media multitasking in college students. At any rate, research on intervention strategies for in-class media multitasking is lacking. Additional studies are needed to evaluate the effectiveness of the intervention strategies discussed above and provide more conclusive explanations on how such interventions work to reduce in-class media multitasking.
3.2. Limitations
Although the present study yielded some unique findings, several limitations should be considered. First, the present study was cross-sectional in nature, which does not allow for drawing a causal conclusion on the role of delay discounting and impulse control in in-class media multitasking. We hypothesise that both delay discounting and impulse control play an etiological role in in-class media multitasking, but the opposite direction of causality is at least possible. In addition, because there were no measures of academic performance included, the relationship between such performance and delay discounting could not be examined in this study. Future research should aim to incorporate various academic performance indicators, such as GPA and exam scores, to gain a deeper understanding of the exact role of delay discounting in potentially influencing academic success.
Second, levels of impulse control were assessed by a self-reported questionnaire, and the value of Cronbach alpha was lower than what is typically recommended (Tavakol and Dennick Citation2011). While self-reported measures have high ecological validity (i.e. they can inform levels of impulse control in more typical everyday situations; Spinella Citation2005), the results with this scale need to be interpreted with caution given the low alpha value. Future research may supplement self-reported measures with performance-based assessments of impulse control, which may yield more reliable evaluation and potentially reveal different aspects of individuals’ levels of impulse control (Toplak, West, and Stanovich Citation2013).
Third, frequencies of in-class media multitasking were also based on self-reported data. It is at least possible that participants under-reported the inappropriate behaviours (Wentland Citation1993). Although the self-reported data were used only for group assignment purposes, it is advisable for future research to supplement self-reported data with observational data. Additionally, the questionnaire may contain some more issues that warrant attention: (a) the questions may not be relevant to students who uniformly dislike or like all of their courses; (b) the questionnaire did not differentiate between in-person and online courses; (c) the complexity of the courses was not taken into account. Given these limitations, it is recommended that future research take these factors into consideration to provide a more comprehensive understanding.
Fourth, some situations preset a choice between smaller-sooner and larger-later rewards where the alternatives may not appear to be mutually exclusive (e.g. sending a text message to a friend while listening to a lecture). However, it is important to note that while multitasking is often perceived as the simultaneous engagement in multiple activities, it is more precisely characterised as a rapid alternation between them (Monsell Citation2003), which is known to deteriorate the performance due to inherent capacity limitations in information processing by the human brain (Marois and Ivanoff Citation2005; cf. Rubinstein, Meyer, and Evans Citation2001). In this context, the choice alternatives are understood as mutually exclusive, both at the moment when the first choice is made and when subsequent task switching takes place. The behavioural economic framework of the present study effectively captures this mutual exclusivity. However, exploring how students themselves perceive these choices – whether as mutually exclusive or otherwise – and how this perception relates to the frequency of in-class media multitasking could be an interesting avenue for future research (cf. Sanbonmatsu et al. Citation2013).
Lastly, the present sample solely consisted of undergraduate students at one university in the Northeastern United States, and the present findings may not generalise to a larger population. Future research should include a larger, more diverse sample to evaluate the external validity of the present findings.
4. Conclusion
Using a hierarchical cluster analysis, we identified subgroups of students who differed in frequencies of media multitasking in their preferred and non-preferred classes. The primary finding of the present study was that the subgroup of students who could resist media multitasking in preferred but not non-preferred classes showed significantly lower rates of delay discounting than the subgroup of students who frequently engaged in media multitasking in both preferred and non-preferred classes. From a behavioural economic perspective, this finding is important as it suggests that the degree of devaluation of future rewards plays an important role in resisting in-class media multitasking under contextual control and that episodic future thinking may be effective in reducing the behaviour. It would be interesting for future research to extend the present study design to media multitasking in other contexts, such as work settings or outside of the classroom. Future research may also evaluate what exact role delay discounting would play in resisting in-class media multitasking by, for example, employing a longitudinal research design. These new insights would ultimately result in maximising the productivity in our society by minimising counterproductive forms of media multitasking.
Ethics approval
The institutional review board at the Pennsylvania State University reviewed the study protocol and deemed the study exempt.
Informed consent
Informed consent was obtained from all individual participants included in the study.
Supplemental Material
Download MS Word (27.1 KB)Disclosure statement
No potential conflict of interest was reported by the authors.
Data availability statement
The datasets generated and analysed during the current study are available from the corresponding author on reasonable request.
Notes
1 From a behaviour analytic perspective, impulse control is essentially prevention or disruption of a well-established behaviour (e.g. contingency-shaped behaviour) through rule-governance (Hayes, Gifford, and Ruckstuhl Citation1996). In the present case, in-class media multitasking maintained by social interaction is prevented/disrupted by the continuation/occurrence of an academic behaviour maintained by a rule (e.g. ‘If I study hard, I can get an A in this class’) that is incompatible with media multitasking.
2 Consistent with previous studies (e.g. McKerchar et al. Citation2009), we compared four models: Mazur's (Citation1987) hyperbolic model, the exponential model (cf. Samuelson Citation1937), Myerson and Green's (Citation1995) hyperbola-like model, and Rachlin's (Citation2006) power-function model. The mean AIC values of these four models were: –8.50 (Mazur’s), –3.18 (exponential), –13.69 (Myerson and Green’s), and –13.87 (Rachlin’s).
References
- Allom, V., B. Mullan, and M. Hagger. 2016. “Does Inhibitory Control Training Improve Health Behaviour? A Meta-Analysis.” Health Psychology Review 10 (2): 168–186. https://doi.org/10.1080/17437199.2015.1051078.
- Bickel, W. K., M. W. Johnson, M. N. Koffarnus, J. MacKillop, and J. G. Murphy. 2014. “The Behavioral Economics of Substance Use Disorders: Reinforcement Pathologies and Their Repair.” Annual Review of Clinical Psychology 10 (1): 641–677. https://doi.org/10.1146/annurev-clinpsy-032813-153724.
- Bolkan, S., and D. J. Griffin. 2017. “Students’ Use of Cell Phones in Class for Off-Task Behaviors: The Indirect Impact of Instructors’ Teaching Behaviors Through Boredom and Students’ Attitudes.” Communication Education 66 (3): 313–329. https://doi.org/10.1080/03634523.2016.1241888.
- Bowling, A. 2005. “Just One Question: If One Question Works, Why Ask Several?” Journal of Epidemiology & Community Health 59 (5): 342–345. https://doi.org/10.1136/jech.2004.021204.
- Boyer, P. 2008. “Evolutionary Economics of Mental Time Travel?” Trends in Cognitive Sciences 12 (6): 219–224. https://doi.org/10.1016/j.tics.2008.03.003.
- Clatworthy, J., D. Buick, M. Hankins, J. Weinman, and R. Horne. 2005. “The Use and Reporting of Cluster Analysis in Health Psychology: A Review.” British Journal of Health Psychology 10 (3): 329–358. https://doi.org/10.1348/135910705X25697.
- Dassen, F. C. M., A. Jansen, C. Nederkoorn, and K. Houben. 2016. “Focus on the Future: Episodic Future Thinking Reduces Discount Rate and Snacking.” Appetite 96:327–332. https://doi.org/10.1016/j.appet.2015.09.032.
- Ellis, Y., B. W. Daniels, and A. Jauregui. 2010. “The Effect of Multitasking on the Grade Performance of Business Students.” Research in Higher Education Journal 8 (1): 1–10. http://aabri.com/rhej.html.
- Froese, A. D., C. N. Carpenter, D. A. Inman, J. R. Schooley, R. B. Barnes, P. W. Brecht, and J. D. Chacon. 2012. “Effects of Classroom Cell Phone Use on Expected and Actual Learning.” College Student Journal 46:323–333. https://www.projectinnovation.com/college-student-journal.html.
- Gilroy, S. P., C. T. Franck, and D. A. Hantula. 2017. “The Discounting Model Selector: Statistical Software for Delay Discounting Applications.” Journal of the Experimental Analysis of Behavior 107 (3): 388–401. https://doi.org/10.1002/jeab.257.
- Gilroy, S. P., and D. A. Hantula. 2018. “Discounting Model Selection with Area-Based Measures: A Case for Numerical Integration.” Journal of the Experimental Analysis of Behavior 109 (2): 433–449. https://doi.org/10.1002/jeab.318.
- Gingerich, A. C., and T. T. Lineweaver. 2014. “OMG! Texting in Class = u Fail :(Empirical Evidence That Text Messaging During Class Disrupts Comprehension.” Teaching of Psychology 41 (1): 44–51. https://doi.org/10.1177/0098628313514177.
- Green, L., and J. Myerson. 2004. “A Discounting Framework for Choice with Delayed and Probabilistic Rewards.” Psychological Bulletin 130:769–792. https://doi.org/10.1037/0033-2909.130.5.769.
- Greenacre, M., and R. Primicerio. 2013. Multivariate Analysis of Ecological Data. Bilbao: Fundación BBVA.
- Hayashi, Y. 2021. “Attitude Moderates the Relation Between Frequency of Media Multitasking in the Classroom and Delay Discounting.” The Psychological Record 71 (2): 211–218. https://doi.org/10.1007/s40732-020-00443-w.
- Hayashi, Y. 2023. “Problematic Mobile Phone Use as Impulsive Choice: Development and Empirical Verification of a Reinforcer Pathology Model.” Journal of the Experimental Analysis of Behavior Advance online publication. https://doi.org/10.1002/jeab.900.
- Hayashi, Y., and G. P. Blessington. 2018. “A Behavioral Economic Analysis of Media Multitasking: Delay Discounting as an Underlying Process of Texting in the Classroom.” Computers in Human Behavior 86:245–255. https://doi.org/10.1016/j.chb.2018.04.049.
- Hayashi, Yusuke, and Glenn P Blessington. 2021. “Excessive Valuation of Social Interaction in Text-Message Dependency: A Behavioral Economic Demand Analysis.” The Psychological Record 71 (2): 237–245. https://doi.org/10.1007/s40732-020-00418-x.
- Hayashi, Y., J. E. Friedel, A. M. Foreman, and O. Wirth. 2019. “A Cluster Analysis of Text Message Users Based on Their Demand for Text Messaging: A Behavioral Economic Approach.” Journal of the Experimental Analysis of Behavior 112 (3): 273–289. https://doi.org/10.1002/jeab.554.
- Hayashi, Yusuke, and Kathryn R Glodowski. 2023. “Males (but not females) who use social networking sites excessively make more impulsive decisions than those who do not: Role of gender and delay discounting.” Behaviour & Information Technology 42 (1): 77–88. https://doi.org/10.1080/0144929X.2021.2015441.
- Hayashi, Y., and J. N. Nenstiel. 2021. “Media Multitasking in the Classroom: Problematic Mobile Phone Use and Impulse Control as Predictors of Texting in the Classroom.” Current Psychology 40 (9): 4500–4506. https://doi.org/10.1007/s12144-019-00395-7.
- Hayes, S. C., E. V. Gifford, and L. E. Ruckstuhl, Jr. 1996. “Relational Frame Theory and Executive Function: A Behavioral Approach.” In Attention, Memory, and Executive Function, edited by G. R. Lyon and N. A. Krasnegor, 279–305. Baltimore: Paul H Brookes Publishing Co. https://doi.org/10.1097/00004703-199608000-00014.
- Hofmann, W., B. J. Schmeichel, and A. D. Baddeley. 2012. “Executive Functions and Self-Regulation.” Trends in Cognitive Sciences 16 (3): 174–180. https://doi.org/10.1016/j.tics.2012.01.006.
- Houben, K., C. Nederkoorn, R. W. Wiers, and A. Jansen. 2011. “Resisting Temptation: Decreasing Alcohol-Related Affect and Drinking Behavior by Training Response Inhibition.” Drug and Alcohol Dependence 116 (1–3): 132–136. https://doi.org/10.1016/j.drugalcdep.2010.12.011.
- Johnson, M. W., and W. K. Bickel. 2008. “An Algorithm for Identifying Nonsystematic Delay-Discounting Data.” Experimental and Clinical Psychopharmacology 16 (3): 264–274. https://doi.org/10.1037/1064-1297.16.3.264.
- Jones, B. A., and H. Rachlin. 2009. “Delay, Probability, and Social Discounting in a Public Goods Game.” Journal of the Experimental Analysis of Behavior 91 (1): 61–73. https://doi.org/10.1901/jeab.2009.91-61.
- Lamb, R. J., D. R. Maguire, B. C. Ginsburg, J. W. Pinkston, and C. P. France. 2016. “Determinants of Choice, and Vulnerability and Recovery in Addiction.” Behavioural Processes 127:35–42. https://doi.org/10.1016/j.beproc.2016.04.001.
- Ledbetter, A. M., and A. N. Finn. 2016. “Why Do Students Use Mobile Technology for Social Purposes During Class? Modeling Teacher Credibility, Learner Empowerment, and Online Communication Attitude as Predictors.” Communication Education 65 (1): 1–23. https://doi.org/10.1080/03634523.2015.1064145.
- Lee, S., M. W. Kim, I. M. McDonough, J. S. Mendoza, and M. S. Kim. 2017. “The Effects of Cell Phone Use and Emotion-Regulation Style on College Students’ Learning.” Applied Cognitive Psychology 31 (3): 360–366. https://doi.org/10.1002/acp.3323.
- Marois, R., and J. Ivanoff. 2005. “Capacity Limits of Information Processing in the Brain.” Trends in Cognitive Sciences 9 (6): 296–305. https://doi.org/10.1016/j.tics.2005.04.010.
- Mazur, J. E. 1987. “An Adjusting Procedure for Studying Delayed Reinforcement.” In Quantitative Analyses of Behavior: The Effect of Delay and of Intervening Events on Reinforcement Value, Vol. 5, edited by M. L. Commons, J. E. Mazur, J. A. Nevin, and H. Rachlin, 55–73. Hillsdale, NJ: Lawrence Erlbaum Associates.
- McCoy, B. 2016. “Digital Distractions in the Classroom Phase II: Student Classroom Use of Digital Devices for Non-Class Related Purposes.” Journal of Media Education 7 (1): 5–32.
- McKerchar, T. L., L. Green, J. Myerson, T. S. Pickford, J. C. Hill, and S. C. Stout. 2009. “A Comparison of Four Models of Delay Discounting in Humans.” Behavioural Processes 81 (2): 256–259. https://doi.org/10.1016/j.beproc.2008.12.017.
- Monsell, S. 2003. “Task Switching.” Trends in Cognitive Sciences 7 (3): 134–140. https://doi.org/10.1016/S1364-6613(03)00028-7.
- Myerson, J., and L. Green. 1995. “Discounting of Delayed Rewards: Models of Individual Choice.” Journal of the Experimental Analysis of Behavior 64 (3): 263–276. https://doi.org/10.1901/jeab.1995.64-263.
- Olmsted, N. M., and C. P. Terry. 2014. “Who’s Texting in Class? A Look at Behavioral and Psychological Predictors.” Psi Chi Journal of Psychological Research 19 (4): 183–190. https://doi.org/10.24839/2164-8204.JN19.4.183.
- Rachlin, H. 2006. “Notes on Discounting.” Journal of the Experimental Analysis of Behavior 85 (3): 425–435. https://doi.org/10.1901/jeab.2006.85-05.
- Rachlin, H., A. Raineri, and D. Cross. 1991. “Subjective Probability and Delay.” Journal of the Experimental Analysis of Behavior 55 (2): 233–244. https://doi.org/10.1901/jeab.1991.55-233.
- Rothbart, M. K., and J. E. Bates. 1988. “Temperament.” In Handbook of Child Psychology: Vol. 3. Social, Emotional, and Personality Development. 5th ed., edited by N. Eisenberg, 105–176. New York: Wiley.
- Rubinstein, J. S., D. E. Meyer, and J. E. Evans. 2001. “Executive Control of Cognitive Processes in Task Switching.” Journal of Experimental Psychology. Human Perception and Performance 27 (4): 763–797. https://doi.org/10.1037/0096-1523.27.4.763.
- Samuelson, P. A. 1937. “A Note on Measurement of Utility.” The Review of Economic Studies 4 (2): 155–161. https://doi.org/10.2307/2967612.
- Sanbonmatsu, D. M., D. L. Strayer, N. Medeiros-Ward, and J. M. Watson. 2013. “Who Multi-Tasks and Why? Multi-Tasking Ability, Perceived Multi-Tasking Ability, Impulsivity, and Sensation Seeking.” PLoS One 8 (1): e54402. https://doi.org/10.1371/journal.pone.0054402.
- Sapci, O., J. D. Elhai, A. Amialchuk, and C. Montag. 2021. “The Relationship Between Smartphone Use and Students` Academic Performance.” Learning and Individual Differences 89:102035. https://doi.org/10.1016/j.lindif.2021.102035.
- Sasse, L. K., J. Peters, C. Büchel, and S. Brassen. 2015. “Effects of Prospective Thinking on Intertemporal Choice: The Role of Familiarity.” Human Brain Mapping 36 (10): 4210–4221. https://doi.org/10.1002/hbm.22912.
- Smith, K. R., S. R. Lawyer, and J. K. Swift. 2018. “A Meta-Analysis of Nonsystematic Responding in Delay and Probability Reward Discounting.” Experimental and Clinical Psychopharmacology 26 (1): 94–107. https://doi.org/10.1037/pha0000167.
- Spinella, M. 2005. “Self-Rated Executive Function: Development of the Executive Function Index.” International Journal of Neuroscience 115 (5): 649–667. https://doi.org/10.1080/00207450590524304.
- Stein, J. S., A. G. Wilson, M. N. Koffarnus, T. O. Daniel, L. H. Epstein, and W. K. Bickel. 2016. “Unstuck in Time: Episodic Future Thinking Reduces Delay Discounting and Cigarette Smoking.” Psychopharmacology 233 (21–22): 3771–3778. https://doi.org/10.1007/s00213-016-4410-y.
- Tavakol, M., and R. Dennick. 2011. “Making Sense of Cronbach’s Alpha.” International Journal of Medical Education 2:53–55. https://doi.org/10.5116/ijme.4dfb.8dfd.
- Toplak, M. E., R. F. West, and K. E. Stanovich. 2013. “Practitioner Review: Do Performance-Based Measures and Ratings of Executive Function Assess the Same Construct?” Journal of Child Psychology and Psychiatry 54 (2): 131–143. https://doi.org/10.1111/jcpp.12001.
- Toyama, M., and Y. Hayashi. 2022. “Links of Personality Traits to Media Multitasking: Conscientiousness Predicts Mobile Phone Use in the College Classroom.” Current Psychology 41 (12): 8660–8667. https://doi.org/10.1007/s12144-020-01258-2.
- Toyama, M., and Y. Hayashi. 2023a. “A Behavioral Economic Demand Analysis of Media Multitasking in the College Classroom: A Cluster Analysis.” Current Psychology 42:27620–27629. https://doi.org/10.1007/s12144-022-03894-2.
- Toyama, M., and Y. Hayashi. 2023b. “Personality and Media Multitasking in the College Classroom: Context-Dependent Implications of Conscientiousness and Agreeableness.” Current Psychology 42:4391–4399. https://doi.org/10.1007/s12144-021-01793-6.
- van der Schuur, W. A., S. E. Baumgartner, S. R. Sumter, and P. M. Valkenburg. 2015. “The Consequences of Media Multitasking for Youth: A Review.” Computers in Human Behavior 53:204–215. https://doi.org/10.1016/j.chb.2015.06.035.
- Wei, F.-Y. F., and Y. K. Wang. 2010. “Students’ Silent Messages: Can Teacher Verbal and Nonverbal Immediacy Moderate Student Use of Text Messaging in Class?” Communication Education 59 (4): 475–496. https://doi.org/10.1080/03634523.2010.496092.
- Wei, F.-Y. F., Y. K. Wang, and M. Klausner. 2012. “Rethinking College Students’ Self-Regulation and Sustained Attention: Does Text Messaging During Class Influence Cognitive Learning?” Communication Education 61 (3): 185–204. https://doi.org/10.1080/03634523.2012.672755.
- Wentland, E. J. 1993. Survey Responses: An Evaluation of Their Validity. San Diego, CA: Academic Press.
- Yim, O., and K. T. Ramdeen. 2015. “Hierarchical Cluster Analysis: Comparison of Three Linkage Measures and Application to Psychological Data.” The Quantitative Methods for Psychology 11:8–21. https://doi.org/10.20982/tqmp.11.1.p008.