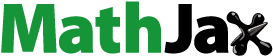
ABSTRACT
Disengagement from social media platforms encompasses various behaviours, such as reducing activity or abandoning them altogether. At the far end of this spectrum, permanently deleting user accounts holds substantial implications for individuals, the people they contact, and the platform itself. However, the motivations underlying this choice, which involves erasing all content and social connections rather than simply abandoning the platform, remain unclear. To address this research gap, we analysed 4.5 million active users on Tumblr, a microblogging service. We assessed the likelihood of users deleting their accounts using binary logistic regression in general and in specific clusters of users. Our findings reveal that users are more inclined to delete their accounts if they have previously removed content from their profiles, highlighting the role of account deletion in cleaning and controlling personal information. Additionally, we observed robust peer correlations, indicating that users are more likely to delete their accounts if those they follow have already taken this step. We discuss these findings in the context of non-use theories and propose the development of checking-out processes that can enhance the user experience associated with account deletion.
1. Introduction
As social media continues to encompass a significant portion of our lives, there is a growing interest in understanding the life cycle of users and patterns of non-use, which refer to the ways individuals disengage from these platforms (Satchell and Dourish Citation2009; Soliman and Rinta-Kahila Citation2020). Non-use is widespread phonomenon: a Pew Research Center study on teens, technology, and social media found that only 32% of U.S. teens aged 13–17 use Facebook at all, but in a previous survey from 2014–2015, that figure was 71% (Vogels, Gelles-Watnick, and Massarat Citation2022). Non-use can manifest as either a temporary break or a permanent departure. Rainie, Smith, and Duggan (Citation2013) found that 61% of Facebook users have taken breaks from the platform lasting several weeks, while 20% have chosen to delete their accounts. Similar rates of account deletion were also observed on Twitter (Mondal et al. Citation2016).
Account deletion can be understood as a behaviour within the broader context of social media non-use, also known as disengagement or discontinuation behaviours. As Baumer et al. (Citation2017) demonstrate, non-use covers a range of behaviours rather than a binary distinction between users and non-users. Users fall along a spectrum that ranges from active engagement to complete account deletion (Lampe, Vitak, and Ellison Citation2013; Saxena et al. Citation2020). The motives for reducing social media use vary and depend on personal factors such as dissatisfaction, fatigue, and privacy concerns (Grandhi, Plotnick, and Hiltz Citation2019). However, the specific reasons are also influenced by where users are on the non-use spectrum. For instance, fatigue is often mentioned as a reason for temporarily stepping away from social media (Schoenebeck Citation2014), whereas privacy concerns are frequently cited for permanently leaving a platform (Brown Citation2020).
Permanently deleting a social media account stands out as a particular type of non-use, yet it has not received the research attention it deserves. Systematic reviews tend to combine account deletion with other forms of non-use, overlooking the nuances between the two (see Farooq, Dahabiyeh, and Maier Citation2023; Nassen et al. Citation2023). Deleting an account should be examined closely because it can significantly affect the content available, relationships among users, and the overall structure of the network. Account deletion has the potential to notably alter the user network on a social media platform (Hesselberth Citation2018). While past research has largely concentrated on user abandonment to understand social media churn (D.-R. Liu et al. Citation2019; Dror et al. Citation2012; Lu et al. Citation2019; Ngonmang, Viennet, and Tchuente Citation2012; Óskarsdóttir et al. Citation2022; Volkova and Bell Citation2017; Ya, Yan-Bing, and Yun-Peng Citation2016), it is important to recognise that various disconnection behaviours stem from varied motivations (Baumer et al. Citation2019).
The primary objective of this study is to characterise account deletion within the broader spectrum of non-use behaviours in social media. We aim to provide a contextual framework for understanding it in relation to existing social media behaviours. Our work is motivated by several reasons. A better understanding of the factors that relate to account deletion can contribute to some concrete design implications. Firstly, identifying the factors contributing to account deletion can help improve the user experience by developing more user-friendly account deletion processes. Secondly, understanding the relationship between content deletion and account deletion is crucial for shaping data protection policies and technologies (Mayer-Schönberger Citation2011). This knowledge can inform the development of effective data deletion and minimisation practices. Lastly, gaining insights into the contextual factors surrounding account deletion can aid social media providers in retaining users and improving their overall experience on the platform. Providers can implement strategies to address concerns and enhance user satisfaction by understanding why users delete their accounts.
To address the existing research gap, we analyse approximately 4.5 million users' behaviour over four months to understand the relationship between users' behaviours before deleting their accounts. By using binary logistic regression, we assess likelihood of active users to delete their account in a four-month period from August 2018 to November 2018, considering several social media usage patterns, such as platform engagement, social connections, activity levels, and peer behaviours. To ensure a comprehensive analysis, we utilise prediction models tailored to different user segments based on their usage patterns. Based on our findings, we propose various strategies to improve the account deletion experience and address potential factors contributing to user disengagement from social media platforms.
2. Background
2.1. Non-use in social media
Contemporary social media scholarly have progressed beyond the binary classification of individuals as users or non-users, providing a more nuanced understanding of engagement and disengagement with these platforms (Breines, Madge, and Dalu Citation2020; Cherubini et al. Citation2021; Portwood-Stacer Citation2013; Radtke et al. Citation2022; Satchell and Dourish Citation2009; Wyatt Citation2003). Harmon and Mazmanian (Citation2013) argue that individuals engage in ongoing negotiations with technology, transitioning between intense engagement and intentional avoidance periods. Hargittai and Hsieh (Citation2010) have categorised users into four intensity levels, including non-users and omnivores who actively engage with a diverse range of social media platforms. Baumer et al. (Citation2019) classified Facebook users into three distinct groups, distinguishing between active users, those considering deactivation, and those who have already deactivated their accounts. Similarly, Ng (Citation2020) proposed an intermediate model for Twitter, differentiating between intermittent and permanent non-use. By exploring this spectrum, new research inquiries arise regarding the decision-making processes of individuals who opt for abrupt account deletion instead of gradually reducing their usage. In other words, if abandoning a platform is relatively straightforward, what factors lead some users to delete their accounts instead of simply abandoning them?
Systematic reviews analysed why users disengage from social media or stop using it altogether (Farooq, Dahabiyeh, and Maier Citation2023; Nassen et al. Citation2023). Studies indicate that user attitudes toward the platform, like finding Facebook not useful, can lead to reduced engagement (Dindar and Akbulut Citation2014; Slot and Opree Citation2021). Qualitative research has also identified that attitudes affecting social connectedness online play a significant role in the decreased use of Facebook (Aloudat, Al-Shamaileh, and Michael Citation2019). Additionally, negative experiences on the platform, such as regrettable user actions (Guha, Baumer, and Gay Citation2018), distressing social comparisons (Appel, Gerlach, and Crusius Citation2016), or exposure to disagreeable opinions (Y. Wang, Ahmed, and Bee Citation2023), contribute to non-use. Background factors, including personality traits (Hong and Oh Citation2020) and demographic variables like gender and age (Baumer Citation2018; Page et al. Citation2018), also influence a user's decision to disengage. Similar characteristics were found in diverse platforms, such as Wechat (Cao et al. Citation2020; J. Lin et al. Citation2020; J. Wang et al. Citation2021; Mahmud et al. Citation2020), Snapchat (Schmuck Citation2020), and LinkedIn (Grandhi, Plotnick, and Hiltz Citation2019). Moreover, the broader competitive landscape of social media platforms should be considered (Grandhi, Plotnick, and Hiltz Citation2019), as users often migrate among platforms based on their evolving preferences and needs.
Social media fatigue is a commonly reported reason for users stepping away from platforms, as shown in various papers (Han Citation2018; K.-M. Lin Citation2015; Kim Citation2022; Yoon and Rolland Citation2015; Zhang et al. Citation2016). Specifically, on Facebook, fatigue has been linked to privacy concerns and a lack of confidence in using the platform (Bright, Kleiser, and Grau Citation2015). Studies, including experiments with Facebook and Instagram users (Graham et al. Citation2021), have found that taking breaks from these platforms can slightly increase wellbeing. Against this backdrop, the concept of ‘digital detox’ –temporarily or permanently disconnecting from digital devices – has gained traction (Schmuck Citation2020; Sutton Citation2017). As highlighted by Schoenebeck (Citation2014), users increasingly consider quitting platforms like Twitter, either temporarily or for good. Similar temporary or permanent disengagement patterns have been observed with Instagram (Jorge Citation2019), Facebook users (Franks, Chenhall, and Keogh Citation2018), and Snapchat users (Hall et al. Citation2021; Schmuck Citation2020).
Privacy concerns and data security are increasingly central to individuals' choice to disengage from social media. In-depth surveys targeting Facebook users have consistently demonstrated that worries over personal data management are a significant driver for users to deactivate or delete their social media accounts (Aloudat, Al-Shamaileh, and Michael Citation2019; Baumer et al. Citation2019). Moreover, such disengagement is not purely for personal reasons; there are also political underpinnings. A segment of users has been documented as withdrawing from platforms in political protest, objecting to policies or ethical stances of the company (Li et al. Citation2019; Noman, Das, and Patil Citation2019). High-profile incidents have amplified these concerns. For instance, users cite the Cambridge Analytica data misuse scandal as a reason to leave Facebook (Brown Citation2020). Similarly, Twitter witnessed a shift in its user base following the takeover by Elon Musk, which brought changes that some users felt compromised the platform's integrity and their personal data security (He et al. Citation2023).
2.2. Churn analysis
Churn analysis, a concept commonly known in business administration (Karnstedt et al. Citation2011), focuses on understanding and predicting user discontinuation in the context of social media platforms. The objective of churn prediction is to identify users who are more likely to discontinue using a particular product or service, enabling the development of effective retention strategies to encourage them to stay (Neslin et al. Citation2006). Retaining existing users is often more cost-effective than acquiring new ones (Buttle Citation1996).
Extensive research has been conducted across various domains to comprehend churn dynamics. This research encompasses diverse industries such as mobile telecommunication networks (Dasgupta et al. Citation2008), online gaming (D.-R. Liu et al. Citation2019; Kawale, Pal, and Srivastava Citation2009; Óskarsdóttir et al. Citation2022), and social media platforms (Dror et al. Citation2012; Karnstedt et al. Citation2011; Lu et al. Citation2019; Ngonmang, Viennet, and Tchuente Citation2012; Ya, Yan-Bing, and Yun-Peng Citation2016). The primary aim of these studies is to gain insights into churn patterns and develop effective strategies for user retention.
Traditional churn models face limitations in the context of social media due to the unique challenges posed by the platform's business model, where users typically do not make direct payments for the service. To address this, many churn studies in social media have focussed on a concept called ‘partial churn’, which involves a significant decline in user activity. Various criteria have been used to define partial churn, such as activity dropping below a certain fraction of the average within a specific time window (Karnstedt et al. Citation2010), prolonged user inactivity exceeding a month (Ngonmang, Viennet, and Tchuente Citation2012), a substantial gap between the user's last and subsequent activities surpassing a specific threshold (D.-R. Liu et al. Citation2019), or the presence of certain words or images in their posts (Volkova and Bell Citation2017). However, these definitions often overlook the phenomenon of account deletion, which holds less economic significance for social media providers.
Existing models examining predictors for deleted accounts (Volkova and Bell Citation2017) or suspended accounts (Alorainy et al. Citation2018; Volkova and Bell Citation2017) have failed to consider former privacy behaviours, social effects, and social media behaviours. Consequently, there is a lack of comprehensive analysis regarding the factors associated with account deletion in social media. Account deletion remains largely unexplored in most churn analysis studies within the social media domain, highlighting the need for further investigation and understanding in this area.
2.3. Content deletion
Deleting individual posts is a common strategy employed by social media users to address potential negative consequences (Sleeper et al. Citation2013; Y. Wang et al. Citation2011; Yılmaz et al. Citation2021), particularly when sensitive information is involved (Almuhimedi et al. Citation2013) or when the content is deemed inappropriate (Gazizullina and Mazzara Citation2019; Zhou, Wang, and Chen Citation2016). Minaei, Mondal, and Kate (Citation2022) showed that users remove approximately 28% of tweets by their users utilising various mechanisms, such as deleting specific tweets or setting their profiles to private. On Facebook, users often delete posts due to privacy worries, the relevancy of the information, and whether it accurately reflects their current social connections (Ayalon and Toch Citation2013, Citation2017). Stieger et al. (Citation2013) has found that those who choose to delete their social media profiles are generally more privacy-conscious, score higher on scales measuring internet addiction, and display greater conscientiousness compared to those who keep their accounts active.
Account deletion encompasses more than simply removing the account; it involves deleting all associated content and restricting access to its information. Within this context, content deletion can be considered a step in an information control process that may eventually culminate in account deletion. Our objective is to examine whether content deletion behaviours can serve as indicators or predictors of account deletion. In particular, we are interested in investigating the connection between deleting individual posts and the subsequent deletion of the entire account. To address this inquiry, we formulate the following research question:
RQ1 : What is the relationship between deleting posts and account deletion?
To comprehensively examine content deletion, it is necessary to consider the overall activity levels on social media platforms. It is reasonable to hypothesise that users who are actively posting information are also more likely to engage in content deletion (Minaei, Mondal, and Kate Citation2022). Hence, ensuring that the act of deleting posts is not solely indicative of user activity is crucial.
2.4. Social effects
Social influence has a significant impact on various behaviours observed on social media platforms, encompassing privacy-related behaviours (Mendel and Toch Citation2017), security-based behaviours (Mendel and Toch Citation2023), or gaming behaviour (Óskarsdóttir et al. Citation2022). It has been observed that individuals tend to disengage from social media platforms collectively, for example on the forum website Skyrock (Ngonmang, Viennet, and Tchuente Citation2012) and the microblogging platform Tumblr (Edwards and Boellstorff Citation2021). On an internet forum site, users were more inclined to reduce their usage when their social connections also decreased their activity levels (Karnstedt et al. Citation2011). D.-R. Liu et al. (Citation2019) demonstrated, by analysing a large online community called Skyrock, that incorporating information about socially connected individuals who have reduced their activity enhances churn prediction models in virtual gaming contexts.
The phenomenon of account deletion introduces new considerations regarding potential social and community effects. While decreased activity due to the absence of content from social connections may not necessarily result in account deletion, it can impact user engagement. Given the social effects associated with account deletion, our aim is to identify factors that can shed light on and quantify such influences. Our research question is formulated within an asymmetric social network model, where user A may follow user B without a reciprocal connection. As account deletion is likely to impact activity within the local community, we explore the extent to which account deletion exhibits contagious behaviour. Hence, we propose the following research question:
RQ2 What is the relationship between account deletion and the presence of followers or followees (users the account holder follows) who have deleted their accounts?
Controlling for the number of social connections a user has is essential when examining social influence. Users with a larger number of social connections tend to exhibit higher activity levels (Xu et al. Citation2014), and isolating the effects of social influence requires accounting for variables such as the number of followers, followees, and activity levels.
Another complex factor to consider is social feedback. Recognition from others through social feedback plays a crucial role in driving information sharing (Vilnai-Yavetz and Levina Citation2018) and determining the extent of routine platform usage (Utz, Tanis, and Vermeulen Citation2012). For instance, by analysing behaviour on Facebook, Grinberg et al. (Citation2017) found that receiving more feedback from friends on a post is associated with a stronger sense of connectedness to one's social connections. Additionally, Lai and Teng highlighted that reciprocity between users, such as sharing each other's content, positively influences the activity level within online communities (Lai and Chen Citation2014). These observations motivate us to account for user reactions to social feedback and their sense of connectedness and social interaction when conducting our analysis.
2.5. Individual differences
Social media users exhibit notable variations in their activity levels, network sizes, and other characteristics (Roberts et al. Citation2008). These differences can stem from personality traits based on the Big Five measure (Błachnio and Przepiorka Citation2016; Ryan and Xenos Citation2011), motivations (Spiliotopoulos and Oakley Citation2020; Vilnai-Yavetz and Levina Citation2018), or objectives (Haimson Citation2018). For example, D. Liu and Campbell (Citation2017) demonstrate that the Big Five meta-trait Plasticity, which encompasses the personality factors of openness to experience and extraversion, positively predicts many social media activities. It is plausible that certain predictors may only be relevant to specific user segments. Utilizing the underlying statistical properties of social media platforms (Xu et al. Citation2014), we derive a set of user characteristics that can be inferred from the network structure and user behaviour. As a sensitivity analysis, we examine how account deletion models relate to essential characteristics such as the number of social connections, level of activity, tenure on the network, and the originality of content (e.g. sharing existing content versus creating new content). Hence, our objective is to assess the relationship between the aforementioned research questions and different segments within the research population.
RQ3 What is the sensitivity of account deletion prediction model to different population segments?
By addressing this research question, we aim to uncover how the models for account deletion vary across distinct population segments.
3. Method
3.1. Dataset
To address the research questions, we conducted our analysis using data from Tumblr, a well-studied microblogging platform (Attu and Terras Citation2017; Chang et al. Citation2014; Edwards and Boellstorff Citation2021; Encinas et al. Citation2018; Haimson Citation2018; Hillman, Procyk, and Neustaedter Citation2014; Ogden Citation2022; Pilipets and Paasonen Citation2022; Xu et al. Citation2014). Tumblr provides users with the ability to create multiple blogs using pseudonyms, allowing them to post original content or reblog posts from other blogs. Users can engage with posts by liking and replying to them, and they can follow other blogs without requiring mutual confirmation, similar to platforms like Twitter and Instagram. Consequently, the social ties network on Tumblr can be represented as a directed graph.
In the context of account deletion, Tumblr offers users a two-stage process to permanently delete their accounts, requiring the username and service password. Once an account is deleted, it cannot be recovered, and users lose all access to it. This distinctive account deletion mechanism sets Tumblr apart from other platforms and provides valuable insights into the dynamics of account deletion in social media settings.
Our study is based on a dataset comprising approximately 6 million blogs on Tumblr. This dataset was randomly sampled from the entire pool of blogs on the platform, using Tumblr's data analysis API and including only publicly available data. It is important to mention that demographic information such as gender or residence was not available to us due to the use of pseudonyms on Tumblr. Our analysis covered a four-month period from August 2018 to November 2018. This timeframe was chosen to ensure an ample sample of blogs and include data before Tumblr's announcement in December 2018 regarding the ban on all adult content.
3.2. Ethical considerations for data collection
We fully acknowledge the sensitivity associated with collecting data on content deletion and recognise the need to carefully consider the benefits and risks of utilising such a dataset (Fiesler and Proferes Citation2018). Understanding the phenomenon of account deletion is crucial for comprehending identity management and information control within the realm of social media. It is important to emphasise that detailed data regarding content deletion from commercial entities like Facebook is inaccessible (Leetaru Citation2021). In this context, obtaining a ground truth dataset becomes essential, particularly when large-scale verification of qualitative hypotheses is not feasible due to the lack of available datasets.
To address potential risks and safeguard the ethical handling of public individual data, we adhered to best practices (Fiesler and Proferes Citation2018; Zimmer Citation2010). Our study was authorised by the institutional ethics committee at Tel Aviv University (ethics approval 1356). The committee had authorised that the consent given by Tumblr users in their terms of service to Tumble is a sufficient consent mechanism, as is common in many studies that were done on social media such as Tumblr and Twitter (Alrajebah, Carr, and Tiropanis Citation2018; Attu and Terras Citation2017; Chang et al. Citation2014; Nanath and Joy Citation2021; Volkova and Bell Citation2017; Xu et al. Citation2014). We ensured that we did not collect or analyse the actual content of blogs, refrained from collecting easily identifiable information (such as usernames), and made no attempts to collect or infer demographic information (such as location or gender) that could facilitate future re-identification. Furthermore, we did not retain the dataset beyond the analysis phase and did not report or share any results that could compromise the privacy of individual users. Keeping the data private may present challenges for further analysis and replication of our research. However, given the sensitive nature of the data, we believe that this decision aligns with ethical considerations.
3.3. Features
To explore the predictors of account deletion, we define a set of features that reflect measurable elements of the research questions.
3.3.1. Dependent variable
Our dependent variable is whether a blog was deleted or not. If a blog i was deleted during the data collection period, its value is 1, and the value is 0. We used a sliding window analysis and analysed the behaviour in the month before the deletion to identify behaviours that systems could continuously predict. To do this, we defined a label date for each blog by its use or non-use classification.
3.3.2. Independent variables
Our independent variables include several key features that represent user behaviour and blog characteristics (). The deleted posts feature measures the number of posts a blog i deleted during the one-month trial period. To assess potential social influence within close social network environments, we developed two measures: the proportion of deleted followees and the proportion of deleted followers. These measures capture the extent to which individuals within a user's social network have chosen to delete their own blogs during the trial period. In addition, we considered the concept of reciprocity by examining the number of followees a user has, denoted as , and the number of followers, denoted as
. By including these variables, we aimed to control for social influence correlations that may impact the likelihood of blog deletion.
Table 1. The independent variables for data analysis.
Drawing inspiration from the work of Grinberg et al. (Citation2016) on Facebook behaviour, we introduce the concept of social reactiveness, which captures variations in posting behaviour based on feedback received by the user. To measure this construct using objective behavioural variables rather than relying on self-reported scales, we devised a novel metric that reflects the user's motivations. Specifically, we calculated the ratio of the average number of posts made by a blog i on days when it received reblogs to the average number of posts made on days when it received no reblogs. A higher value indicates a greater disparity in the number of posts between reblog-receiving days and non-reblog-receiving days, thereby signifying the blog's heightened motivation to post in response to receiving reblogs. Since receiving reblogs is indicative of the popularity of the blog's posts, this measure offers insights into the extent to which social reactiveness drives the blog's posting behaviour.
3.3.3. Control variables
We incorporated several well-established control variables to control for the structural and behavioural aspects of users' social networks. These are based on metrics from social media research (Chang et al. Citation2014; Xu et al. Citation2014). The first metric, activity, quantifies the average number of posts published or shared by a user within the trial period. To capture the number of social connections, we considered two variables: the average number of blogs the user follows (as depicted in (a)) and the average number of blogs that follow the user. We calculated their averages since these numbers can vary throughout the trial period. As illustrated in (b), the distribution of social connections aligns with a Power Law distribution.
Figure 1. Distribution of in-degree and out-degree of blogs. (a) The distribution of the number of followees (out-degree) per account and (b) The distribution of the number of followers (in-degree) per account.
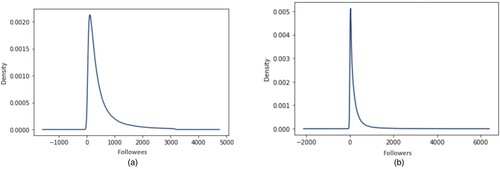
Given that Tumblr enables blogs to share original content and posts from other blogs through the ‘reblogging’ feature, we can assess the degree of originality for each blog. The originality metric captures the average proportion of original posts made by blog i out of all the posts it shared during its trial period. This metric serves as an indicator of the user's production of unique and original content.
An additional control variable we considered is the creation year of the blog, represented in (a). Most evaluated blogs were created between 2013 and 2018, exhibiting relatively consistent variability. During our data collection period, Tumblr categorised blogs with explicit or sensitive content as NSFW (Not Safe For Work). To account for the influence of this category on overall churn patterns, we included a binary variable as a control based on the blog's classification on the label date. This control variable helps ensure that NSFW blogs do not solely drive observed differences in complete churn.
3.4. Definition of active users
In our study, which centres on active account deletions rather than mere abandonment, we employed specific criteria to identify account deletions. We included accounts that made at least one post during our four-month study period and blogs active for more than three months. After refining our data, we ended up with 4,480,853 blogs. Our definition of active users adheres to established benchmarks used in similar research (Ahn et al. Citation2020; Baumer et al. Citation2015). We have chosen the criteria for defining active users by following best practices in studies that used similar methods (Ahn et al. Citation2020; Baumer et al. Citation2015).
This approach ensured that we examined active accounts recently, ruling out inactive or abandoned ones before our data collection. Our intent, similar to the focus in studies by Oentaryo et al. (Citation2012) and Ngonmang, Viennet, and Tchuente (Citation2012), was to understand the behaviour and choices of users who interact with the platform, as inactive or seldom-used accounts could skew our findings. These accounts often represent an atypical user experience and do not accurately reflect the platform's active user dynamics. Furthermore, we wanted to avoid biases introduced by new accounts, which, as Olteanu et al. (Citation2019) notes, may not exhibit established user patterns due to the experimental nature of their creation.
To evaluate how the definition of active users influences our dataset, presents the number of blogs categorised by activity levels. In line with literature conventions (Ngonmang, Viennet, and Tchuente Citation2012; Oentaryo et al. Citation2012), ‘Less Active’ users had at least one inactive month within the study's timeframe. In contrast, ‘More Active’ users posted at least once monthly. The data reveals that ‘Less Active’ users deleted their accounts at a rate of 0.3%, while ‘More Active’ users did so at a rate of 0.5%. Our analysis incorporates these activity variations within the ‘activity’ variable and accounts for the account age with the ‘created date’ variable.
Table 2. Proportion of deleted accounts in different levels of activity.
3.5. Data analysis
We conducted two analyses to investigate the factors influencing how Tumblr users delete their accounts. Firstly, we examined the entire population and developed a unified model that links feature values to the deletion probability. Secondly, we conducted a sensitivity analysis of our model by segmenting the population into distinct user groups based on usage patterns and constructing separate models for each cluster. Segmenting the population is a well-established approach in churn analysis, as observed in previous studies (Baumer et al. Citation2015; Baumer et al. Citation2019; Bharat Citation2019; D.-R. Liu et al. Citation2019; De Caigny, Coussement, and De Bock Citation2018). This approach enables us to assess the robustness of the models across different user segments and determine if the effects remain consistent despite individual differences. Logistic regression also provides the probability of the occurrence of the account deletion. This is particularly useful in a social network context as it helps to understand whether a user might leave and how likely they are to do so. This probability can help prioritise retention efforts toward the most at-risk users.
Binary logistic regression was utilised to compare users who deleted their accounts with the entire sample. Logistic regression was preferred due to its robustness in churn analysis, surpassing alternative techniques such as survival analysis (Nie et al. Citation2011). This methodology is widely employed in the field, particularly in studies investigating event occurrence within a defined time frame (Asthana Citation2018; Vafeiadis et al. Citation2015). The primary objective of our research was to ascertain whether users would delete their accounts within a specific time frame rather than estimating the duration until deletion. Furthermore, Survival analysis models like the Cox model (Haenlein Citation2013) assume proportional hazards -- that the effect of the covariates is multiplicative with respect to the hazard function and constant over time. We could not assert that this assumption is true in the case of Tumblr.
The proportion of users who deleted their accounts during the study period is relatively low, as illustrated in . This creates an imbalance in the binary classification of the dependent variable. Approximately 20,000 instances of account deletions provide a sufficiently large dataset to train a robust machine learning model (Ling and Sheng Citation2008). While non-deleted blogs form most of our dataset, the significant count of deleted blogs still guarantees a substantial sample for analysis.
To assess the stability of the measured features over the trial period, we analysed their variability (refer to ). The results indicate relatively low variability, suggesting that the features remain stable over time. This stability enhances the reliability of using these variables to predict account deletion and analyse non-use behaviours. Furthermore, the features fulfill the assumptions of logistic regression analysis, including independence of errors and lack of strongly influential outliers. (Hair et al. Citation2010). Given the dataset's large scale, we have set the statistical significance level at 0.001. Consequently, we place greater emphasis on the sizes of the coefficients to infer correlations. Notably, in-degree, out-degree, and activity distribution align with previous analyses conducted on Tumblr (Chang et al. Citation2014).
4. Results
4.1. Account deletion behaviors
We conducted a binary logistic regression analysis to investigate the factors associated with account deletion among participants (refer to ). This analysis allows us to examine the relationship between the independent variables and the likelihood of users deleting their blogs. To assess the significance of our models, we calculated the deviance for both the full model and the constant-only model (‘0-Model’), as shown in . The results indicate that both models are statistically significant compared to the constant-only model, with the full model demonstrating greater predictive power. While the control variables also exhibit significance, their effects are generally weaker than those of the predictor variables. As shows, during the study period, 21,112 blogs were deleted, accounting for approximately 0.47%.
Table 3. Logistic regression models for account deletion.
4.2. Deleted posts
The number of deleted posts within a one-month period emerges as a robust and positive predictor of account deletion (). This effect is substantial, as deleting a post increases the likelihood of account deletion by approximately 65% (calculated using odds ratios). As depicted in (b), we have analysed the number of post deletions by users, resulting in a distribution of deleted posts characterised by long tail distribution. Notably, this relationship remains significant even after accounting for potential confounding factors associated with post deletion, including activity (
), number of followers (
), and number of followees (
). Consequently, we can confidently assert that the answer to Research Question 1 showcases a robust and positive association between the deletion of posts and subsequent account deletion.
4.3. Deleted followees and followers
The proportion of deleted followees emerges as the most influential predictor, demonstrating a positive association with the likelihood of blog deletion (). Based on the odds ratio, we can deduce that if half of the blogs a user follows are deleted within a month, the chances of deleting their own blog increase by approximately 86%. Notably, this relationship does not exhibit symmetrical effects with individuals who follow the user's blog.
The deletion of followers exhibits a minimal impact on the likelihood of blog deletion (). Remarkably, these results remain robust even after controlling for average follower count, average followee count, and the level of reciprocity. The weak correlation with the number of followers allows us to reject explanations linking account deletion to diminished popularity resulting from follower attrition. Hence, we can confidently assert that the answer to Research Question 2 unveils a robust association between the number of deleted followees and account deletion, while no significant relationship is observed with the number of followers who have deleted their accounts.
4.4. Social reactiveness
The impact of social reactiveness on account deletion behaviour within the context of our model is substantial. Users who exhibit higher levels of reactiveness tend to engage in increased posting immediately after being reblogged. Importantly, our findings reveal that individuals with greater social reactiveness are less susceptible to account deletion during the trial period (). Specifically, users with a reactiveness value of 0.25 demonstrate a 47% higher likelihood of deleting their accounts compared to those with a value of 0.75.
These outcomes hold significant implications, particularly when considering the influence of activity as a controlling factor, which encompasses the cumulative count of posts and reblogs. Notably, activity demonstrates a weak positive impact on blog deletion (), whereas reactiveness exerts a robust effect. Consequently, we can confidently conclude that the act of blogging and sharing content on its own does not strongly correlate with account deletion. In contrast, users' behavioural patterns serve as potent predictors for the occurrence of account deletion. Furthermore, this result withstands the inclusion of additional control variables in the model, such as the number of followers and followees. Thus, our comprehensive analysis underscores the importance of users' behaviour patterns, specifically social reactiveness, in discerning and forecasting account deletion tendencies.
4.5. Control variables
There is a weak correlation between social media activity on Tumblr and the probability of account deletion. More active individuals have a slightly higher chance of deleting their accounts than less active individuals (). Examining the overall statistics of account deletion provides a clearer picture. Only 13% of the deleted blogs had no activity in the month prior to deletion, indicating that the majority (87%) of the deleted blogs had at least one post or share. Notably, activity positively correlates with account deletion, suggesting that engaging in posting and reblogging activities increases the likelihood of users deleting their blogs. Although our research population was selected based on having at least one blog post during the study period, it is still interesting to observe that deletion is associated with increased activity rather than decreased activity.
The impact of the other control variables on account deletion is generally weak. The age of the account shows a slight positive correlation with deletion (), indicating that older blogs have a slightly higher likelihood of being deleted. However, it's worth noting that we have already excluded inactive blogs from our analysis. The number of followees also exhibits a weak positive correlation with deletion (
), suggesting that having more followees is associated with a slightly higher probability of account deletion. On the other hand, the correlation with the number of followers is not statistically significant, indicating that the number of followers does not significantly impact account deletion. The variables NSFW (Not Safe for Work) and originality show very weak correlations with account deletion (
and
respectively). This implies that these factors have minimal influence on the likelihood of account deletion.
4.6. Account deletion in user segments
Our last goal is to model the relationship between individual differences and account deletion. We carry out this type of sensitivity analysis by segmenting users into groups and fitting models to each group individually. This analysis helps us control our research questions for different usage patterns in the network, determining whether we can generalise our results to all of Tumblr's users.
To categorise each account, we considered five variables: activity, created date, in-degree, out-degree, and originality. These dimensions were selected based on their high variability observed in previous studies of Tumblr (Xu et al. Citation2014). Our clustering approach began by identifying blogs with no followers, labelled as ‘Never followed’ blogs. Since these blogs lack followers, analysing them using network and social measures would be irrelevant.
For the remaining blogs, we created 5-dimensional vectors representing their normalised network and behavioural characteristics. We then applied K-means clustering to segment the blogs based on these vectors. We employed the elbow method to determine the optimal number of clusters, plotting the explained variation using the Sum of Squares Error (SSE) as a function of the number of clusters. We have picked up the elbow of the curve as the number of clusters to use. The analysis, shown in , suggests that four clusters best capture the data's underlying patterns.
We obtained a total of five clusters, including four clusters generated by the algorithm and an additional cluster consisting of ‘Never followed blogs’. To label each cluster, we considered the relative centroid values and drew inspiration from user types mentioned in the relevant literature, such as long-term users, recently registered users, and active users (Agarwal et al. Citation2008). Summary statistics for each cluster can be found in and . The first cluster, ‘Long term followed’, represents users who have been on the platform for an extended period and have amassed significant followers (high In-degree). The second cluster, ‘Recently registered’, includes users who joined the site recently and have limited posting activity. The third cluster, ‘Active most-followed’, comprises users who frequently post and are followed by numerous other blogs. Lastly, the fourth cluster, ‘Original’, consists of blogs that exhibit relatively high levels of originality.
Figure 3. The four segments, each with its centroid value of the five variables. The ‘never followed’ users are not presented because they do not have centroid values.
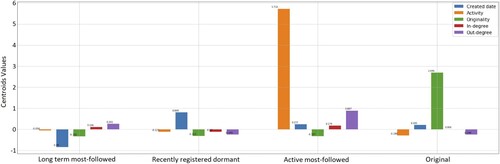
The proportions of blog deleters in each segment significantly differ, providing insights into RQ3 and highlighting the distinct characteristics among user segments. A contingency table was constructed to examine the frequency of deleters within each group (). Pearson's chi-squared test results indicate a significant variation in the proportion of deleters across the groups (test statistic = 4247.198, p<0.001, df = 4). This suggests that the prevalence of complete account deletion varies depending on the user segment. To further investigate the significance of the higher proportion of deleters in the ‘Original users’ group, a post-hoc hypothesis test was conducted using an adjusted Pearson residual. The test results reveal an adjusted Pearson residual of 22.73, indicating significance at the 0.05 level. Therefore, we can conclude that ‘Original’ blogs exhibit a higher likelihood of account deletion compared to other blog types.
Table 4. The contingency of the number of blog deleters categorised by segment, along with the results of post-hoc hypothesis tests.
We conducted separate logistic regression analyses for each segment to examine the predictors of account deletion. The predictor variables included in each model were the factors analysed in the full model, and the response variable was the label indicating whether the blog's account was deleted. The results of these analyses are presented in . The significance of each model was assessed by comparing it to the constant-only model (‘0-Model’) using a chi-square test with 5 degrees of freedom. All models were statistically significant, although the ‘Never followed blogs’ model had a borderline p-value of 0.046.
Table 5. Logistic regression models for each cluster.
Analysing each segment individually reveals differences in deletion patterns among different types of blogs. Across all segments of active blogs, the deletion of posts is a significant predictor of blog deletion. However, this predictor has the strongest effect on ‘original users’ compared to the intercept and other predictors. This finding highlights the significance that highly original users place on their content, which may contain personal and potentially sensitive information. The strong correlation between post-deletion and account deletion among users with more original content further supports the observation that previous deletion behaviour is linked to privacy concerns. In contrast, there are no significant predictors for deletion in the ‘Never followed’ segment, indicating that blog deletions in this group are essentially random with respect to our independent variables.
Social correlations exhibit significant variations among different user segments. The predictor ‘deleted followees’ shows significance only in the ‘long-term followed’ and ‘recently registered’ segments. This indicates that users managing these blogs are more susceptible to social influence than the ‘active most-followed’ and ‘original’ segments, which are driven by different motivations. On the other hand, the predictor ‘deleted followers’ remains significant in all models except for the ‘never followed’ segment. However, it is a particularly strong predictor in the ‘original’ segment (in comparison to the intercept) and exhibits a weaker influence in the other segments.
Social reactiveness is a significant predictor only for the ‘active most-followed’ users. This finding highlights the importance placed by these users on receiving reactions to their posts, and within this segment, users who are more reactive exhibit a lower likelihood of deleting their accounts. This connection suggests that social reactiveness is closely tied to distinct social media usage patterns, particularly in retaining users with an active and extensive social network.
5. Discussion
This section presents our findings within the broader context of existing literature on non-use. We also propose the design of checking-out mechanisms to facilitate a seamless and organised departure from social media networks.
5.1. Characterizing account deletion
Our findings point to the place of account deletion within the broader spectrum of non-use behaviours in social media. Firstly, we argue that studies focussed on churn in social media, which primarily investigate abandonment (D.-R. Liu et al. Citation2019; Dror et al. Citation2012; Hamilton et al. Citation2017; Karnstedt et al. Citation2011; Lu et al. Citation2019; Ngonmang, Viennet, and Tchuente Citation2012; Sadeque and Bethard Citation2019; Ya, Yan-Bing, and Yun-Peng Citation2016, should expand their analysis to include a more nuanced exploration of non-use behaviours. While some recent literature has acknowledged the importance of distinguishing between the various forms of non-use (Soliman and Rinta-Kahila Citation2020), most research works remain insensitive to these distinctions.
The motivations behind account deletion and abandonment can differ significantly, and their impact on network structure and user attitudes may also vary greatly. Moreover, the processes leading to abandonment and deletion can exhibit distinct characteristics. Abandonment is typically characterised as a negative behaviour involving reducing or ceasing specific social media practices (Grandhi, Plotnick, and Hiltz Citation2019; Schoenebeck Citation2014). In contrast, account deletion represents a proactive and often final action, requiring users to take deliberate and challenging steps. To illustrate this contrast metaphorically through the words of Dylan Thomas, if account abandonment is akin to a gradual fading process, a ‘dying of the light', then account deletion can be seen as an active act of defiance, a symbolic ‘burn and rave at the close of day’.
5.2. Account deletion as a cleaning process
We found a strong and consistent association between post-deletion and account deletion across all segments of active users. Although we did not directly measure privacy concerns, our findings align with the theoretical link between content deletion on social networks (Ayalon and Toch Citation2017; Mondal et al. Citation2016). This connection, which was previously inconclusive in other studies (Baumer et al. Citation2019), is reinforced by our results in two significant ways. Our findings highlight the correlation between the established practice of deleting posts and the eventual deletion of the entire account. Secondly, our research sheds light on the deletion process itself, revealing that users often begin by removing specific posts before deciding to delete their entire account.
Examining different user segments based on their network and behavioural characteristics has allowed us to understand the relationship between deleting posts and deleting accounts. Specifically, we discovered a stronger association between deleting content and deleting their accounts when focussing on users who tend to publish more original content (referred to as ‘original users’). This finding suggests that original content, which is more personal and unique to the user, may play a role in their decision to delete their account. This result indirectly suggests that sensitive content could potentially increase the likelihood of account deletion. It is possible that users may be more inclined to abandon their accounts if the content they have created is reused by others rather than permanently deleting it.
5.3. Social effects
Previous studies have demonstrated the impact of social influence on non-use behaviour in social media (D.-R. Liu et al. Citation2019; Karnstedt et al. Citation2011; Ngonmang, Viennet, and Tchuente Citation2012). Our findings extend this correlation to the context of account deletion, revealing that social connections and user behaviour on the social network are influential factors. Specifically, following a user who deletes their account is a strong predictor of account deletion. This suggests that social influence plays a role, as users become aware of account deletion through the actions of those they follow and may be influenced by their decisions. Similar patterns have been observed in other social networking behaviours (Mendel and Toch Citation2017), indicating the applicability of this phenomenon to account deletion. Another explanation is that when followees quit, it reduces content and social connections, potentially resulting in a ‘community collapse’. Theoretical models support the plausibility of such a collapse occurring when central users depart from the network (Rehman et al. Citation2020).
We observe that social effects influence certain segments of users. This includes users who heavily rely on social interaction, whether they are relatively new (‘Recently registered dormant’ users) or more established (‘Long-term most-followed users’). Interestingly, we found that the impact of followers who delete their accounts is relatively weak. It appears that the decrease in followers, which can result in reduced social engagement, does not have a significant or noticeable effect on account deletion. Our work strengthens approaches that put an emphasis on collective activity rather than on reduced interests. Studies have pointed to collective quitting of social networks in cases where there are major changes to the network (Edwards and Boellstorff Citation2021), but our work shows that even if the network remains relatively stable, social processes have a significant impact.
Our analysis has showed that profile deletion is related to social interaction patterns. Previous studies have indicated the short-term effects of feedback from social connections (Grinberg et al. Citation2016) and the long-term effects of social capital on the usefulness and enjoyment of social networks (Lampe, Vitak, and Ellison Citation2013). Our findings bridge these strands of research by providing evidence of the relationship between social feedback and social media usage. By segmenting users into clusters, we identified two distinct motivations for account deletion among different user types. In the segment comprising the most socially active users, known as ‘active most-followed’ users, high social reactiveness showed a strong negative correlation with the likelihood of profile deletion. However, these results were not significant for other user types, suggesting that different types of social feedback may not retain users with different motivations. Reciprocity emerged as a significant factor in predicting profile deletion across all segments, although its effect size was relatively weak.
5.4. Retaining users
Our prediction models can be utilised in designing new user interactions centred around account deletion. First, we explore checking-out mechanisms to enhance the account deletion experience. Certain behavioural measures, such as deleting posts, can serve as indicators for future account deletion. Social media platforms can actively suggest that users consider deleting their accounts after removing significant content. While this may initially seem counter-intuitive for social media platforms, it is important to consider the benefits of implementing orderly account deletion procedures. Firstly, it can improve the user experience for individuals who wish to leave the service. Secondly, it addresses legal data retention and rights issues and offers enhanced data protection for all users. Lastly, it assists in cleaning up the service by removing old and irrelevant data.
Social media platforms can leverage indicators of account deletion to enhance user retention and improve overall user experiences. As suggested by Au, Ma, and Li (Citation2003), developing effective retention policies involves two steps: understanding the reasons behind account deletions and identifying users at high risk of deletion. Our findings highlight that certain aspects of the user experience can contribute to user retention. Specifically, the absence of feedback emerges as a strong predictor for users leaving the platform, particularly among active and social users. For instance, implementing measures to increase feedback, such as making user posts more visible, can be a robust retention strategy. However, it is essential for service providers to ensure that their retention policies are not misused by employing dark patterns that discourage users from deleting their accounts. Balancing retention efforts with user autonomy and control is crucial for maintaining a positive user experience.
Segmenting users based on behavioural characteristics reveals valuable insights for tailoring retention policies to specific user types. Our analysis indicates that ‘long-term most-followed’ users' behaviour is primarily related to their social environment. This suggests that an intervention strategy, such as suggesting replacements for deleted users, could effectively mitigate churn for this particular group. For instance, providing feedback from other users can enhance the productivity of these users, and utilising push notifications to amplify followee feedback can further improve retention. The intervention can be implemented more widely and effectively by focussing the treatment on this specific user segment. These nuanced findings highlight the importance of personalised approaches in increasing retention rates.
5.5. Checking-out processes
Users often find the process of deleting their accounts to be unnecessarily complicated (Minaei, Mondal, and Kate Citation2022). To respect users' decisions to leave while maintaining a positive impression of the service, simplifying the account deletion process is crucial. This simplification involves providing straightforward navigation to the deletion feature, reducing the required steps, and offering clear information about the extent of data removal. Since deleting specific posts often precedes account deactivation, offering users tools to easily review and manage their content (such as posts and interactions) can empower them with greater control over their data. This enhanced control might lead users to reconsider completely deleting their accounts, as they feel more capable of managing their online presence.
Developers can leverage the insights from our research to identify several indicators that might initiate the account checking-out process. The link between content deletion and full account deactivation underscores the value users place on privacy management. Furthermore, designers can predict and respond to community changes by analysing network dynamics and identifying users potentially influenced by others leaving the platform. Recognizing signs of community collapse could prompt the checking-out process, helping platforms engage with users proactively and address their concerns before they choose to leave.
5.6. Limitations
It is essential for readers to consider the contextual nature of our findings. Our research was specifically conducted on the Tumblr platform, and it is important to exercise caution when generalising these findings to other systems. Each social media platform has unique protocols and procedures for account deactivation and departure (Grandhi, Plotnick, and Hiltz Citation2019), and these variations can significantly impact patterns of non-use. Furthermore, different platforms may have different definitions and criteria for categorising non-users (Saxena et al. Citation2020). For example, some platforms offer the option to temporarily deactivate accounts (Baumer et al. Citation2019), while others only allow permanent account deletion. Our findings are specifically based on the characteristics of Tumblr, where hibernation of accounts is not available, and account deletion is a final decision (see ). Therefore, it is crucial to recognise that the processes and mechanisms associated with account deletion in other systems may differ significantly.
In the Tumblr platform, users can create multiple accounts (referred to as blogs) and use pseudonyms. However, when users delete their account on Tumblr, they also remove all their followers, followees, content, and interactions associated with that account. Previous studies have highlighted the importance of these investments in the lives of Tumblr users (Bourlai and Herring Citation2014; Hillman, Procyk, and Neustaedter Citation2014), indicating that deleting an account represents a significant sacrifice for many users. As a result, our analysis may have greater relevance to platforms like Twitter, where users can have multiple accounts, compared to platforms like Facebook, which encourages users to have a single identified account and discourages multiple accounts.
It is important to stress that our findings should not be naively interpreted as indicating a causal relationship. We do not claim that changes in the independent variables directly cause or prevent the deletion of profiles. Instead, our analysis focuses on identifying correlations that can be used for prediction. Furthermore, it is worth mentioning that our study did not examine certain variables that could potentially mediate the effects of the independent variables. For example, we did not measure homophily, commonly associated with social influence (Noel and Nyhan Citation2011).
6. Conclusions
In this study, we explore the phenomenon of account deletion within the context of social media as a form of non-use. Our research focuses on Tumblr and utilises a comprehensive dataset of over 4.5 million active users. We examine the complex relationships between factors related to user accounts and their behaviours. By analysing events leading up to account deletion during a specific sampling period, we employ logistic regression models to identify key predictors of account deletion.
Our analysis shows that the deletion of specific posts is a reliable predictor of account deletion, indicating that account deletion is part of more comprehensive cleaning processes of personal data. We also document a correlation with peer behaviour: the number of users followed by an individual who has deleted their accounts strongly influences the likelihood of account deletion. This highlights the importance of peer influence in the decision to delete one's account, suggesting potential community-level effects in the dynamics of non-use. Furthermore, our study observes the impact of individual differences, such as social interaction patterns and responsiveness to social feedback, on the likelihood of account deletion. These factors contribute to a better understanding of why some individuals delete their accounts while others do not.
Future Research should allow us to gain deeper insights into the complex dynamics of account deletions. One aspect that should be further investigated is the motivations of users and the connection with specific behaviours of account deletion. This type of research can adopt a multi-method approach, combining our type of behavioural analysis with surveys and content analysis. This approach will provide a more comprehensive understanding of the underlying reasons behind account deletion and help uncover additional factors associated with non-use. Merging psychological surveys and constructs with actual user behaviour could lead to a more comprehensive understanding of why users decide to delete their accounts. Additionally, future studies should explore the effect of peer behaviour on account deletion in more detail. For example, employing network analysis techniques could effectively map and quantify how their peers influence an individual's decision to delete their account, offering valuable insights into the social mechanisms that drive account deletion.
Further future studies can use our metrics and see how various intervention methods could impact people's account deletion patterns, such as retention of check-out methods. As our research was specific to Tumblr, extending similar studies to various social media platforms could shed light on how unique features and community cultures impact account deletion. By comparing platforms with distinct policies and types of interaction (such as Facebook and Instagram), we could uncover broader trends and more universally applicable factors that influence account deletion.
Disclosure statement
No potential conflict of interest was reported by the author(s).
Additional information
Funding
References
- Agarwal, N., H. Liu, L. Tang, and P. S. Yu. 2008. “Identifying the Influential Bloggers in a Community.” In Proceedings of the 2008 International Conference on Web Search and Data Mining, 207–218.
- Ahn, J., J. Hwang, D. Kim, H. Choi, and S. Kang. 2020. “A Survey on Churn Analysis in Various Business Domains.” IEEE Access 8:220816–220839. https://doi.org/10.1109/Access.6287639.
- Almuhimedi, H., S. Wilson, B. Liu, N. Sadeh, and A. Acquisti. 2013. “Tweets are Forever: A Large-Scale Quantitative Analysis of Deleted Tweets.” In Proceedings of the 2013 Conference on Computer Supported Cooperative Work, 897–908.
- Alorainy, W., P. Burnap, H. Liu, A. Javed, and M. L. Williams. 2018. “Suspended Accounts: A Source of Tweets with Disgust and Anger Emotions for Augmenting Hate Speech Data Sample.” In 2018 International Conference on Machine Learning and Cybernetics (ICMLC), Vol. 2, 581–586. IEEE.
- Aloudat, A., O. Al-Shamaileh, and K. Michael. 2019. “Why Some People Do Not Use Facebook?.” Social Network Analysis and Mining 9 (1): 19. https://doi.org/10.1007/s13278-019-0564-z.
- Alrajebah, N., L. Carr, and T. Tiropanis. 2018. “The Platform Effect: Analysing User Activity on Tumblr.” In International Conference on Internet Science, 154–168. Springer.
- Appel, H., A. L. Gerlach, and J. Crusius. 2016. “The Interplay Between Facebook Use, Social Comparison, Envy, and Depression.” Current Opinion in Psychology 9:44–49. https://doi.org/10.1016/j.copsyc.2015.10.006.
- Asthana, P. 2018. “A Comparison of Machine Learning Techniques for Customer Churn Prediction.” International Journal of Pure and Applied Mathematics 119 (10): 1149–1169.
- Attu, R., and M. Terras. 2017. “What People Study when they Study Tumblr.” Journal of Documentation 73 (3): 528–534. https://doi.org/10.1108/JD-08-2016-0101.
- Au, T., G. Ma, and S. Li. 2003. “Applying and Evaluating Models to Predict Customer Attrition Using Data Mining Techniques.” Journal of Comparative International Management 6 (1): 10–22.
- Ayalon, O., and E. Toch. 2013. “Retrospective Privacy: Managing Longitudinal Privacy in Online Social Networks.” In Proceedings of the Ninth Symposium on Usable Privacy and Security, 1–13.
- Ayalon, O., and E. Toch. 2017. “Not Even Past: Information Aging and Temporal Privacy in Online Social Networks.” Human–Computer Interaction 32 (2): 73–102. https://doi.org/10.1080/07370024.2016.1203791.
- Baumer, E. P. 2018. “Socioeconomic Inequalities in the Non Use of Facebook.” In Proceedings of the 2018 CHI Conference on Human Factors in Computing Systems, 1–14.
- Baumer, E. P., S. Guha, E. Quan, D. Mimno, and G. K. Gay. 2015. “Missing Photos, Suffering Withdrawal, Or Finding Freedom? How Experiences of Social Media Non-Use Influence the Likelihood of Reversion.” Social Media + Society 1 (2): 2056305115614851.
- Baumer, E. P., S. Guha, P. Skeba, and G. Gay. 2019. “All Users are (not) Created Equal: Predictors Vary for Different Forms of Facebook Non/use.” Proceedings of the ACM on Human-Computer Interaction3 (CSCW): 1–28. https://doi.org/10.1145/3359182.
- Baumer, E. P., X. Xu, C. Chu, S. Guha, and G. K. Gay. 2017. “When Subjects Interpret the Data: Social Media Non-Use as a Case for Adapting the Delphi Method to CSCW.” In Proceedings of the 2017 ACM Conference on Computer Supported Cooperative Work and Social Computing, 1527–1543.
- Bharat, A. 2019. “Consumer Engagement Pattern Analysis Leading to Improved Churn Analytics: An Approach for Telecom Industry.” In Data Management, Analytics and Innovation, 203–211. Springer.
- Błachnio, A., and A. Przepiorka. 2016. “Personality and Positive Orientation in Internet and Facebook Addiction. An Empirical Report From Poland.” Computers in Human Behavior 59:230–236. https://doi.org/10.1016/j.chb.2016.02.018.
- Bourlai, E., and S. C. Herring. 2014. “Multimodal Communication on Tumblr: ‘I Have So Many Feels!’.” In Proceedings of the 2014 ACM Conference on Web Science, 171–175.
- Breines, M. R., C. Madge, and M. T. B. Dalu. 2020. “Social Media Among African Students: Recentring Typologies of Non-Use.” Digital Geography and Society 1:100006. https://doi.org/10.1016/j.diggeo.2020.100006.
- Bright, L. F., S. B. Kleiser, and S. L. Grau. 2015. “Too Much Facebook? An Exploratory Examination of Social Media Fatigue.” Computers in Human Behavior 44:148–155. https://doi.org/10.1016/j.chb.2014.11.048.
- Brown, A. J. 2020. “‘Should I Stay Or Should I Leave?’: Exploring (Dis) Continued Facebook Use After the Cambridge Analytica Scandal.” Social Media+ Society 6 (1): 2056305120913884.
- Buttle, F. A. 1996. Relationship Marketing: Theory and Practice. London: Sage.
- Cao, X., A. N. Khan, A. Ali, and N. A. Khan. 2020. “Consequences of Cyberbullying and Social Overload While Using Snss: A Study of Users' Discontinuous Usage Behavior in Snss.” Information Systems Frontiers 22 (6): 1343–1356. https://doi.org/10.1007/s10796-019-09936-8.
- Chang, Y., L. Tang, Y. Inagaki, and Y. Liu. 2014. “What is Tumblr: A Statistical Overview and Comparison.” ACM SIGKDD Explorations Newsletter 16 (1): 21–29. https://doi.org/10.1145/2674026.2674030.
- Cherubini, M., L. Reut, J. Tyler, and M. Ortlieb. 2021. “Inattentive, Imprudent and Inapt: Discovering Inadequacies of Ict During Life-Changing Events Through the Lens of Non-Users.” Behaviour & Information Technology 40 (15): 1643–1670. https://doi.org/10.1080/0144929X.2020.1772879.
- Dasgupta, K., R. Singh, B. Viswanathan, D. Chakraborty, S. Mukherjea, A. A. Nanavati, and A. Joshi. 2008. “Social Ties and Their Relevance to Churn in Mobile Telecom Networks.” In Proceedings of the 11th International Conference on Extending Database Technology: Advances in Database Technology, 668–677.
- De Caigny, A., K. Coussement, and K. W. De Bock. 2018. “A New Hybrid Classification Algorithm for Customer Churn Prediction Based on Logistic Regression and Decision Trees.” European Journal of Operational Research 269 (2): 760–772. https://doi.org/10.1016/j.ejor.2018.02.009.
- Dindar, M., and Y. Akbulut. 2014. “Why Do Pre-Service Teachers Quit Facebook? An Investigation on ‘quitters Forever’ and ‘quitters for a While’.” Computers in Human Behavior 39:170–176. https://doi.org/10.1016/j.chb.2014.07.007.
- Dror, G., D. Pelleg, O. Rokhlenko, and I. Szpektor. 2012. “Churn Prediction in New Users of Yahoo! Answers.” In Proceedings of the 21st International Conference on World Wide Web, 829–834.
- Edwards, E. J., and T. Boellstorff. 2021. “Migration, Non-Use, and the ‘Tumblrpocalypse’: Towards a Unified Theory of Digital Exodus.” Media, Culture & Society 43 (3): 582–592. https://doi.org/10.1177/0163443720968461.
- Encinas, E., M. Blythe, S. Lawson, J. Vines, J. Wallace, and P. Briggs. 2018. “Making Problems in Design Research: The Case of Teen Shoplifters on Tumblr.” In Proceedings of the 2018 CHI Conference on Human Factors in Computing Systems, 1–12.
- Farooq, A., L. Dahabiyeh, and C. Maier. 2023. “Social Media Discontinuation: A Systematic Literature Review on Drivers and Inhibitors.” Telematics and Informatics 77:101924. https://doi.org/10.1016/j.tele.2022.101924.
- Fiesler, C., and N. Proferes. 2018. “‘Participant’ Perceptions of Twitter Research Ethics.” Social Media+ Society 4 (1): 2056305118763366.
- Franks, J., R. Chenhall, and L. Keogh. 2018. “The Facebook Sabbatical As a Cycle: Describing the Gendered Experience of Young Adults As they Navigate Disconnection and Reconnection.” Social Media+ Society 4 (3): 2056305118801995.
- Gazizullina, A., and M. Mazzara. 2019. “Prediction of Twitter Message Deletion.” In 2019 12th International Conference on Developments in eSystems Engineering (DeSE), 117–122. IEEE.
- Graham, S., A. Mason, B. Riordan, T. Winter, and D. Scarf. 2021. “Taking a Break From Social Media Improves Wellbeing Through Sleep Quality.” Cyberpsychology, Behavior, and Social Networking 24 (6): 421–425. https://doi.org/10.1089/cyber.2020.0217.
- Grandhi, S. A., L. Plotnick, and S. R. Hiltz. 2019. “Do I Stay Or Do I Go? Motivations and Decision Making in Social Media Non-Use and Reversion.” Proceedings of the ACM on Human-Computer Interaction 3 (GROUP): 1–27. https://doi.org/10.1145/3361116.
- Grinberg, N., P. A. Dow, L. A. Adamic, and M. Naaman. 2016. “Changes in Engagement Before and After Posting to Facebook.” In Proceedings of the 2016 CHI Conference on Human Factors in Computing Systems, 564–574.
- Grinberg, N., S. Kalyanaraman, L. A. Adamic, and M. Naaman. 2017. “Understanding Feedback Expectations on Facebook.” In Proceedings of the 2017 ACM Conference on Computer Supported Cooperative Work and Social Computing, 726–739.
- Guha, S., E. P. Baumer, and G. K. Gay. 2018. “Regrets, I've Had a Few: When Regretful Experiences Do (And Don't) Compel Users to Leave Facebook.” In proceedings of the 2018 ACM Conference on Supporting Groupwork, 166–177.
- Haenlein, M. 2013. “Social Interactions in Customer Churn Decisions: The Impact of Relationship Directionality.” International Journal of Research in Marketing 30 (3): 236–248. https://doi.org/10.1016/j.ijresmar.2013.03.003.
- Haimson, O. 2018. “Social Media As Social Transition Machinery.” Proceedings of the ACM on Human-Computer Interaction 2 (CSCW): 1–21. https://doi.org/10.1145/3274332.
- Hair, J. F., R. E. Anderson, B. J. Babin, and W. C. Black. 2010. Multivariate Data Analysis: A Global Perspective, Vol. 7.
- Hall, J. A., C. Xing, E. M. Ross, and R. M. Johnson. 2021. “Experimentally Manipulating Social Media Abstinence: Results of a Four-Week Diary Study.” Media Psychology 24 (2): 259–275. https://doi.org/10.1080/15213269.2019.1688171.
- Hamilton, W. L., J. Zhang, C. Danescu-Niculescu-Mizil, D. Jurafsky, and J. Leskovec. 2017. “Loyalty in Online Communities.” In Proceedings of the…International AAAI Conference on Weblogs and Social Media. International AAAI Conference on Weblogs and Social Media, Vol. 2017, 540. NIH Public Access.
- Han, B. 2018. “Social Media Burnout: Definition, Measurement Instrument, and why We Care.” Journal of Computer Information Systems 58 (2): 122–130. https://doi.org/10.1080/08874417.2016.1208064.
- Hargittai, E., and Y.-L. P. Hsieh. 2010. “From Dabblers to Omnivores: A Typology of Social Network Site Usage.” In A Networked Self, 154–176. London: Routledge.
- Harmon, E., and M. Mazmanian. 2013. “Stories of the Smartphone in Everyday Discourse: Conflict, Tension & Instability.” In Proceedings of the SIGCHI Conference on Human Factors in Computing Systems, 1051–1060.
- He, J., H. B. Zia, I. Castro, A. Raman, N. Sastry, and G. Tyson. 2023. “Flocking to Mastodon: Tracking the Great Twitter Migration.” In Proceedings of the 2023 ACM on Internet Measurement Conference, 111–123.
- Hesselberth, P. 2018. “Discourses on Disconnectivity and the Right to Disconnect.” New Media & Society 20 (5): 1994–2010. https://doi.org/10.1177/1461444817711449.
- Hillman, S., J. Procyk, and C. Neustaedter. 2014. “Tumblr Fandoms, Community & Culture.” In Proceedings of the Companion Publication of the 17th ACM Conference on Computer Supported Cooperative Work & Social Computing, 285–288.
- Hong, S., and S. K. Oh. 2020. “Why People Don't Use Facebook Anymore? An Investigation Into the Relationship Between the Big Five Personality Traits and the Motivation to Leave Facebook.” Frontiers in Psychology 11:1497. https://doi.org/10.3389/fpsyg.2020.01497.
- Jorge, A. 2019. “Social Media, Interrupted: Users Recounting Temporary Disconnection on Instagram.” Social Media + Society 5 (4): 2056305119881691.
- Karnstedt, M., T. Hennessy, J. Chan, and C. Hayes. 2010. “Churn in Social Networks: A Discussion Boards Case Study.” In 2010 IEEE Second International Conference on Social Computing, 233–240. IEEE.
- Karnstedt, M., M. Rowe, J. Chan, H. Alani, and C. Hayes. 2011. “The Effect of User Features on Churn in Social Networks.” In Proceedings of the 3rd International Web Science Conference, 1–8.
- Kawale, J., A. Pal, and J. Srivastava. 2009. “Churn Prediction in MMORPGs: A Social Influence Based Approach.” In 2009 International Conference on Computational Science and Engineering, Vol. 4, 423–428. IEEE.
- Kim, J.-Y. 2022. “Exploring Perceptional Typology of Social Media Quitters and Associations Among Self-Esteem, Personality, and Motivation.” Behaviour & Information Technology 41 (2): 262–275. https://doi.org/10.1080/0144929X.2020.1801841.
- Lai, H.-M., and T. T. Chen. 2014. “Knowledge Sharing in Interest Online Communities: A Comparison of Posters and Lurkers.” Computers in Human Behavior 35:295–306. https://doi.org/10.1016/j.chb.2014.02.004.
- Lampe, C., J. Vitak, and N. Ellison. 2013. “Users and Nonusers: Interactions Between Levels of Adoption and Social Capital.” In Proceedings of the 2013 Conference on Computer Supported Cooperative Work, 809–820.
- Leetaru, K. 2021. “In The Name Of Research, Will Facebook Stop Allowing Us To Delete Our Data?”.
- Li, H., N. Vincent, J. Tsai, J. Kaye, and B. Hecht. 2019. “How Do People Change Their Technology Use in Protest? Understanding.” Proceedings of the ACM on Human-Computer Interaction 3 (CSCW): 1–22.
- Lin, K.-M. 2015. “Predicting Asian Undergraduates' Intention to Continue Using Social Network Services From Negative Perspectives.” Behaviour & Information Technology 34 (9): 882–892. https://doi.org/10.1080/0144929X.2015.1027736.
- Lin, J., S. Lin, O. Turel, and F. Xu. 2020. “The Buffering Effect of Flow Experience on the Relationship Between Overload and Social Media Users' Discontinuance Intentions.” Telematics and Informatics 49:101374. https://doi.org/10.1016/j.tele.2020.101374.
- Ling, C. X., and V. S. Sheng. 2008. “Cost-Sensitive Learning and the Class Imbalance Problem.” In Encyclopedia of Machine Learning, edited by C. Sammut. Heidelberg: Springer.
- Liu, D., and W. K. Campbell. 2017. “The Big Five Personality Traits, Big Two Metatraits and Social Media: A Meta-Analysis.” Journal of Research in Personality 70:229–240. https://doi.org/10.1016/j.jrp.2017.08.004.
- Liu, D.-R., H.-Y. Liao, K.-Y. Chen, and Y.-L. Chiu. 2019. “Churn Prediction and Social Neighbour Influences for Different Types of User Groups in Virtual Worlds.” Expert Systems 36 (3): e12384. https://doi.org/10.1111/exsy.v36.3.
- Lu, Y., L. Yu, P. Cui, C. Zang, R. Xu, Y. Liu, L. Li, and W. Zhu. 2019. “Uncovering the Co-Driven Mechanism of Social and Content Links in User Churn Phenomena.” In Proceedings of the 25th ACM SIGKDD International Conference on Knowledge Discovery & Data Mining, 3093–3101.
- Mahmud, I., S. R. Das, S. Ahmed, S. C. J-Ho, and T. W. Andalib. 2020. “Fun Or Frustration: Modelling Discontinuance Intention of Social Media Users.” International Journal of Electronic Commerce Studies11 (2): 107–118. https://doi.org/10.7903/ijecs.
- Mayer-Schönberger, V. 2011. Delete: The Virtue of Forgetting in the Digital Age. Princeton, NJ: Princeton University Press.
- Mendel, T., and E. Toch. 2017. “Susceptibility to Social Influence of Privacy Behaviors: Peer Versus Authoritative Sources.” In Proceedings of the 2017 ACM Conference on Computer Supported Cooperative Work and Social Computing, 581–593.
- Mendel, T., and E. Toch. 2023. “Social Support for Mobile Security: Comparing Close Connections and Community Volunteers in a Field Experiment.” In Proceedings of the 2023 CHI Conference on Human Factors in Computing Systems, 1–18.
- Minaei, M., M. Mondal, and A. Kate. 2022. “Empirical Understanding of Deletion Privacy: Experiences, Expectations, and Measures.” In 31st USENIX Security Symposium (USENIX Security 22), 3415–3432.
- Mondal, M., J. Messias, S. Ghosh, K. P. Gummadi, and A. Kate. 2016. “Forgetting in Social Media: Understanding and Controlling Longitudinal Exposure of Socially Shared Data.” In Twelfth Symposium on Usable Privacy and Security ({;SOUPS}; 2016), 287–299.
- Nanath, K., and G. Joy. 2021. “Leveraging Twitter Data to Analyze the Virality of Covid-19 Tweets: A Text Mining Approach.” Behaviour & Information Technology 42 (2): 196–210. https://doi.org/10.1080/0144929X.2021.1941259.
- Nassen, L.-M., H. Vandebosch, K. Poels, and K. Karsay. 2023. “Opt-Out, Abstain, Unplug. A Systematic Review of the Voluntary Digital Disconnection Literature.” Telematics and Informatics, 101980. 81: 101980. https://doi.org/10.1016/j.tele.2023.101980.
- Neslin, S. A., S. Gupta, W. Kamakura, J. Lu, and C. H. Mason. 2006. “Defection Detection: Measuring and Understanding the Predictive Accuracy of Customer Churn Models.” Journal of Marketing Research 43 (2): 204–211. https://doi.org/10.1509/jmkr.43.2.204.
- Ng, Y. M. M. 2020. “Re-Examining the Innovation Post-Adoption Process: The Case of Twitter Discontinuance.” Computers in Human Behavior 103:48–56. https://doi.org/10.1016/j.chb.2019.09.019.
- Ngonmang, B., E. Viennet, and M. Tchuente. 2012. “Churn Prediction in a Real Online Social Network Using Local Community Analysis.” In 2012 IEEE/ACM International Conference on Advances in Social Networks Analysis and Mining, 282–288. IEEE.
- Nie, G., W. Rowe, L. Zhang, Y. Tian, and Y. Shi. 2011. “Credit Card Churn Forecasting by Logistic Regression and Decision Tree.” Expert Systems with Applications 38 (12): 15273–15285. https://doi.org/10.1016/j.eswa.2011.06.028.
- Noel, H., and B. Nyhan. 2011. “The ‘Unfriending’ Problem: The Consequences of Homophily in Friendship Retention for Causal Estimates of Social Influence.” Social Networks 33 (3): 211–218. https://doi.org/10.1016/j.socnet.2011.05.003.
- Noman, A. S. M., S. Das, and S. Patil. 2019. “Techies Against Facebook: Understanding Negative Sentiment Toward Facebook via User Generated Content.” In Proceedings of the 2019 CHI Conference on Human Factors in Computing Systems, 1–15.
- Oentaryo, R. J., E.-P. Lim, D. Lo, F. Zhu, and P. K. Prasetyo. 2012. “Collective Churn Prediction in Social Network.” In 2012 IEEE/ACM International Conference on Advances in Social Networks Analysis and Mining, 210–214. IEEE.
- Ogden, J. 2022. “‘Everything on the Internet Can Be Saved’: Archive Team, Tumblr and the Cultural Significance of Web Archiving.” Internet Histories 6 (1–2): 113–132. https://doi.org/10.1080/24701475.2021.1985835.
- Olteanu, A., C. Castillo, F. Diaz, and E. Kıcıman. 2019. “Social Data: Biases, Methodological Pitfalls, and Ethical Boundaries.” Frontiers in Big Data 2:13. https://doi.org/10.3389/fdata.2019.00013.
- Óskarsdóttir, M., K. E. Gísladóttir, R. Stefánsson, D. Aleman, and C. Sarraute. 2022. “Social Networks for Enhanced Player Churn Prediction in Mobile Free-To-Play Games.” Applied Network Science 7 (1): 82. https://doi.org/10.1007/s41109-022-00524-5.
- Page, X., P. Wisniewski, B. P. Knijnenburg, and M. Namara. 2018. “Social Media's Have-Nots: An Era of Social Disenfranchisement.” Internet Research 28 (5): 1253–1274. https://doi.org/10.1108/IntR-03-2017-0123.
- Pilipets, E., and S. Paasonen. 2022. “Nipples, Memes, and Algorithmic Failure: NSFW Critique of Tumblr Censorship.” New Media & Society 24 (6): 1459–1480. https://doi.org/10.1177/1461444820979280.
- Portwood-Stacer, L. 2013. “Media Refusal and Conspicuous Non-Consumption: The Performative and Political Dimensions of Facebook Abstention.” New Media & Society 15 (7): 1041–1057. https://doi.org/10.1177/1461444812465139.
- Radtke, T., T. Apel, K. Schenkel, J. Keller, and E. von Lindern. 2022. “Digital Detox: An Effective Solution in the Smartphone Era? a Systematic Literature Review.” Mobile Media & Communication10 (2): 190–215. https://doi.org/10.1177/20501579211028647.
- Rainie, L., A. Smith, and M. Duggan. 2013. Coming and Going on Facebook. Technical report, Pew Research Center.
- Rehman, A. U., R. Tariq, A. Rehman, and A. Paul. 2020. “Collapse of Online Social Networks: Structural Evaluation, Open Challenges, and Proposed Solutions.” In 2020 IEEE Globecom Workshops (GC Wkshps, 1–6. IEEE.
- Roberts, S. G., R. Wilson, P. Fedurek, and R. I. Dunbar. 2008. “Individual Differences and Personal Social Network Size and Structure.” Personality and Individual Differences 44 (4): 954–964. https://doi.org/10.1016/j.paid.2007.10.033.
- Ryan, T., and S. Xenos. 2011. “Who Uses Facebook? An Investigation Into the Relationship Between the Big Five, Shyness, Narcissism, Loneliness, and Facebook Usage.” Computers in Human Behavior27 (5): 1658–1664. https://doi.org/10.1016/j.chb.2011.02.004.
- Sadeque, F., and S. Bethard. 2019. “Predicting Engagement in Online Social Networks: Challenges and Opportunities.” arXiv preprint arXiv:1907.05442.
- Satchell, C., and P. Dourish. 2009. “Beyond the User: Use and Non-Use in HCI.” In Proceedings of the 21st Annual Conference of the Australian Computer-Human Interaction Special Interest Group: Design: Open 24/7, 9–16.
- Saxena, D., P. Skeba, S. Guha, and E. P. Baumer. 2020. “Methods for Generating Typologies of Non/use.” Proceedings of the ACM on Human-Computer Interaction 4 (CSCW1): 1–26. https://doi.org/10.1145/3392832.
- Schmuck, D. 2020. “Does Digital Detox Work? Exploring the Role of Digital Detox Applications for Problematic Smartphone Use and Well-Being of Young Adults Using Multigroup Analysis.” Cyberpsychology, Behavior, and Social Networking 23 (8): 526–532. https://doi.org/10.1089/cyber.2019.0578.
- Schoenebeck, S. Y. 2014. “Giving up Twitter for Lent: How and Why we Take Breaks from Social Media.” In Proceedings of the SIGCHI Conference on Human Factors in Computing Systems, 773–782.
- Sleeper, M., R. Balebako, S. Das, A. L. McConahy, J. Wiese, and L. F. Cranor. 2013. “The Post That Wasn't: Exploring Self-Censorship on Facebook.” In Proceedings of the 2013 Conference on Computer Supported Cooperative Work, 793–802.
- Slot, M., and S. J. Opree. 2021. “Saying No to Facebook: Uncovering Motivations to Resist Or Reject Social Media Platforms.” The Information Society 37 (4): 214–226. https://doi.org/10.1080/01972243.2021.1924905.
- Soliman, W., and T. Rinta-Kahila. 2020. “Toward a Refined Conceptualization of is Discontinuance: Reflection on the Past and a Way Forward.” Information & Management 57 (2): 103167. https://doi.org/10.1016/j.im.2019.05.002.
- Spiliotopoulos, T., and I. Oakley. 2020. “An Exploration of Motives and Behavior Across Facebook and Twitter.” Journal of Systems and Information Technology 22 (2): 201–222. https://doi.org/10.1108/JSIT-12-2019-0258.
- Stieger, S., C. Burger, M. Bohn, and M. Voracek. 2013. “Who Commits Virtual Identity Suicide? Differences in Privacy Concerns, Internet Addiction, and Personality Between Facebook Users and Quitters.” Cyberpsychology, Behavior, and Social Networking 16 (9): 629–634. https://doi.org/10.1089/cyber.2012.0323.
- Sutton, T. 2017. “Disconnect to Reconnect: The Food/technology Metaphor in Digital Detoxing.” First Monday 22 (6): 1–41.
- Utz, S., M. Tanis, and I. Vermeulen. 2012. “It is All About Being Popular: The Effects of Need for Popularity on Social Network Site Use.” Cyberpsychology, Behavior, and Social Networking 15 (1): 37–42. https://doi.org/10.1089/cyber.2010.0651.
- Vafeiadis, T., K. I. Diamantaras, G. Sarigiannidis, and K. C. Chatzisavvas. 2015. “A Comparison of Machine Learning Techniques for Customer Churn Prediction.” Simulation Modelling Practice and Theory 55:1–9. https://doi.org/10.1016/j.simpat.2015.03.003.
- Vilnai-Yavetz, I., and O. Levina. 2018. “Motivating Social Sharing of E-business Content: Intrinsic Motivation, Extrinsic Motivation, Or Crowding-Out Effect?.” Computers in Human Behavior 79:181–191. https://doi.org/10.1016/j.chb.2017.10.034.
- Vogels, E. A., R. Gelles-Watnick, and N. Massarat. 2022. Teens, Social Media and Technology 2022. Technical report, Pew Research Center.
- Volkova, S., and E. Bell. 2017. “Identifying Effective Signals to Predict Deleted and Suspended Accounts on Twitter Across Languages.” In Proceedings of the International AAAI Conference on Web and Social Media, Vol. 11, 290–298.
- Wang, Y., S. Ahmed, and A. W. T. Bee. 2023. “Selective Avoidance As a Cognitive Response: Examining the Political Use of Social Media and Surveillance Anxiety in Avoidance Behaviours.” Behaviour & Information Technology 43 (3): 590–604.
- Wang, Y., G. Norcie, S. Komanduri, A. Acquisti, P. G. Leon, and L. F. Cranor. 2011. “‘I Regretted the Minute I Pressed Share’ a Qualitative Study of Regrets on Facebook.” In Proceedings of the Seventh Symposium on Usable Privacy and Security, 1–16.
- Wang, J., B. Zheng, H. Liu, and L. Yu. 2021. “A Two-Factor Theoretical Model of Social Media Discontinuance: Role of Regret, Inertia, and Their Antecedents.” Information Technology & People34 (1): 1–24. https://doi.org/10.1108/ITP-10-2018-0483.
- Wyatt, S. M. 2003. “Non-Users Also Matter: The Construction of Users and Non-Users of the Internet.” In Now Users Matter: The Co-Construction of Users and Technology, 67–79.
- Xu, J., R. Compton, T.-C. Lu, and D. Allen. 2014. “Rolling Through Tumblr: Characterizing Behavioral Patterns of the Microblogging Platform.” In Proceedings of the 2014 ACM Conference on Web Science, 13–22.
- Ya, L., L. Yan-Bing, and X. Yun-Peng. 2016. “A Churn Prediction Iterative Model in Social Network Based on Relationship Strength.” DEStech Transactions on Computer Science and Engineering (AICE-NCS).
- Yılmaz, G. S., F. Gasaway, B. Ur, and M. Mondal. 2021. “Perceptions of Retrospective Edits, Changes, and Deletion on Social Media.” In Proceedings of the International AAAI Conference on Web and Social Media, Vol. 15, 841–852.
- Yoon, C., and E. Rolland. 2015. “Understanding Continuance Use in Social Networking Services.” Journal of Computer Information Systems 55 (2): 1–8. https://doi.org/10.1080/08874417.2015.11645751.
- Zhang, S., L. Zhao, Y. Lu, and J. Yang. 2016. “Do You Get Tired of Socializing? An Empirical Explanation of Discontinuous Usage Behaviour in Social Network Services.” Information & Management 53 (7): 904–914. https://doi.org/10.1016/j.im.2016.03.006.
- Zhou, L., W. Wang, and K. Chen. 2016. “Tweet Properly: Analyzing Deleted Tweets to Understand and Identify Regrettable Ones.” In Proceedings of the 25th International Conference on World Wide Web, 603–612.
- Zimmer, M. 2010. “‘But the Data is Already Public’: on the Ethics of Research in Facebook.” Ethics and Information Technology 12 (4): 313–325. https://doi.org/10.1007/s10676-010-9227-5.
Appendix: Additional material
Figure A1. A screenshot of the user interface on Tumblr for account deletion. The interface is displayed to users after clicking the settings page's ‘Delete Account’ link. The UI asks them to authenticate to complete the permanent account deletion process, click the ‘Nevermind’ link, or click the browser's ‘back’ button to cancel.
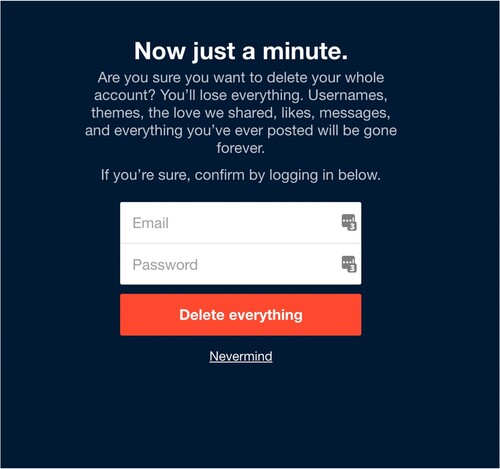
Figure A2. Distribution of deleted followees and deleted followers. (a) The distribution of the number of deleted followees and (b) The distribution of the number of deleted followers
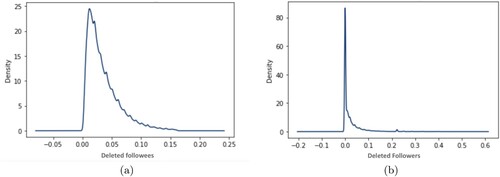
Figure A3. The distribution of social reactiveness.
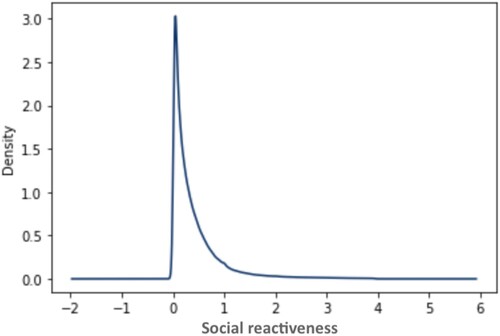
Figure A4. The Sum of Squares Error (SSE) is plotted against the number of clusters.
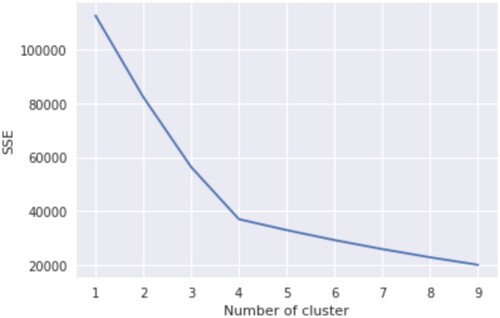
Figure A5. Distribution of users' originality and NSFW Flag. (a) The distribution of the users' originality: the proportion of original content and (b) NSFW Flag distribution. 1 -- blogs with adult content, 0- blogs without adult content.
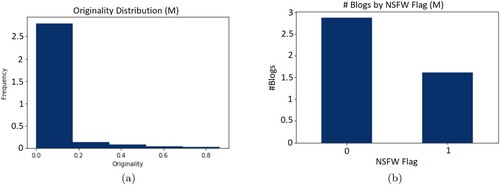
Table A1. Feature stability over the four-month study period, which presents the mean and variability of each feature.
Table A2. The goodness of fit for the logistic regression models by segments was evaluated using a deviance test between each model and the 0-Model.
Table A3. Clusters centroid values and interpretation.