ABSTRACT
This paper reports the use of Markov Chain Monte Carlo (MCMC) and Metropolis Hastings (MH) approach, to solve an inverse heat transfer problem. Three-dimensional, steady state, conjugate heat transfer from a Teflon cylinder of dimensions 100 mm diameter and 100 mm length with uniform volumetric internal heat generation is considered. The goal is to estimate volumetric heat generation and heat transfer coefficient, given the temperature data at certain fixed location on the surface of the cylinder. The internal volumetric heat generation is specified as input and the temperature and heat transfer coefficient values are obtained by a numerical solution to the governing equation. The temperature values also depend on heat transfer coefficient which is obtained by solving Navier–Stokes equation to obtain flow information. In order to reduce the computational cost, a neural network is trained from the computational fluid dynamics simulations. This is posed as an inverse problem wherein volumetric heat generation and heat transfer coefficient are unknown but the temperature data is known by conducting experiments. The novelty of the paper is the simultaneous determination of volumetric heat generation and heat transfer coefficient for the experimentally measured steady-state temperatures from a Teflon cylinder using MCMC-MH as an inverse model in a Bayesian framework and finally, the estimates are reported in terms of mean, maximum a posteriori, and the standard deviation which is the uncertainty associated with the estimated parameters.
Additional information
Notes on contributors
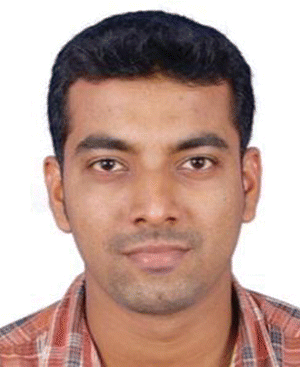
Harsha Kumar
Harsha Kumar is a research scholar in the Department of Mechanical Engineering at National Institute of Technology Karnataka, India. He graduated in Mechanical Engineering from Malnad College of Engineering, Hassan, Karnataka in the year 2006 and obtained his Master's degree from NMAMIT, Nitte in Energy Engineering during the year 2010. His research interests include numerical simulations, inverse methods, probabilistic techniques, artificial neural network, and experimental heat transfer.
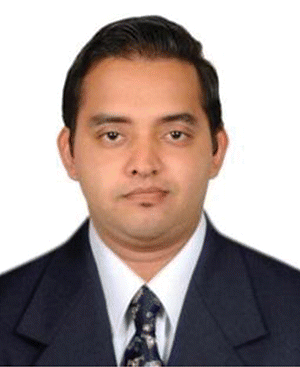
Sharath Kumar
Sharath Kumar is a Senior Section Engineer in South Western Railways, Bangalore. He obtained his B.E. degree in Mechanical Engineering from National Institute of Engineering, Mysore, and he completed his M. Tech. degree in 2015 at National Institute of Technology Karnataka, Surathkal in the Department of Mechanical Engineering, India, under the supervision of Dr. N. Gnanasekaran. His research interests include experimental and numerical studies on conjugate heat transfer, artificial neural networks, inverse methods, and CFD simulations.
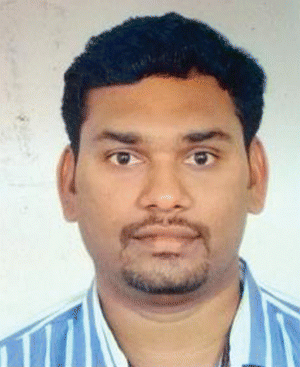
Nagarajan Gnanasekaran
Nagarajan Gnanasekaran is an Assistant Professor in the Department of Mechanical Engineering at the National Institute of Technology Karnataka, India. He graduated in Mechanical Engineering (2002) from Bharathidasan University and obtained his Master of Engineering in Thermal Engineering (2006) from Government College of Technology and Ph.D. from Indian Institute of Technology Madras (2012), Chennai under the supervision of Prof. C. Balaji, IIT Madras. His research interests include computational inverse problems, stochastic modeling techniques, experimental and numerical heat transfer and hybrid optimization.

Chakravarthy Balaji
Chakravarthy Balaji is a professor in the Department of Mechanical Engineering at the Indian Institute of Technology (IIT) Madras. He graduated in Mechanical Engineering from Guindy Engineering College, Chennai (1990), and obtained his M. Tech. (1992) and Ph.D. (1995) both from IIT Madras. His research interests include computational and experimental heat transfer, optimization in thermal sciences, inverse heat transfer, satellite meteorology, and numerical weather prediction.