Abstract
Electrohydrodynamic atomization (EHDA) has advantages in producing fine uniform droplets with low energy consumption. In this work, the droplets generated by the EHDA under the dripping mode have been captured by a backlit high-speed imaging system under various liquid temperatures T (293–343 K) and electric Bond numbers BoE (0–0.75). The temperature-viscosity relationships of the working fluids (G20, G40, G50, and G66) were fitted by an Arrhenius-type model. The effects of BoE, We, and T on the dimensionless droplet limiting length l* and oscillation frequency f were investigated through extensive experiments. The experimental results show that the droplet limiting length elongated with the higher liquid viscosity. However, the oscillation frequency of the meniscus declines with the liquid viscosity. By the elevation of liquid temperatures, the droplet limiting length diminishes, but the oscillation frequency of the liquid meniscus accelerates. Coefficients to estimate the effects of the flow rate and the temperature on oscillation frequency are introduced into the classical theory of meniscus oscillation to develop a semi-empirical model. The proposed model could estimate oscillation frequency for the meniscus under various electric Bond numbers, Weber numbers, and temperatures. The prediction achieves good agreements with experimental results.
Disclosure statement
No potential conflict of interest was reported by the author(s).
Additional information
Funding
Notes on contributors
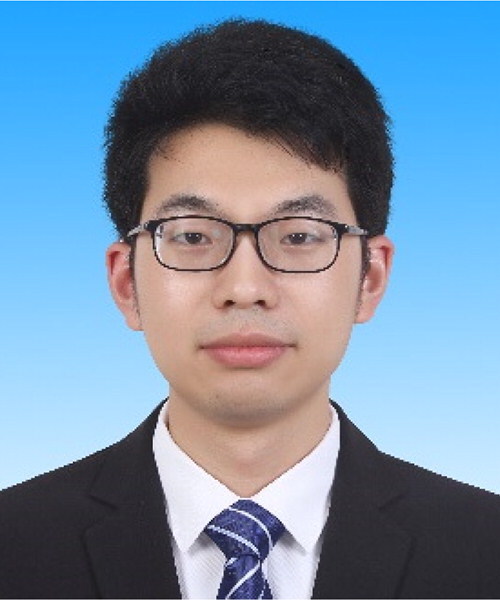
Honglei Wu
Honglei Wu is currently a master student in the School of Energy and Power Engineering at Jiangsu University, Zhenjiang, Jiangsu, China. He received his B.Eng. degree in Energy and Power Engineering at Jiangsu University, China, in 2019. He is interested in the electric spray and the research of atomization parameter analysis technology.
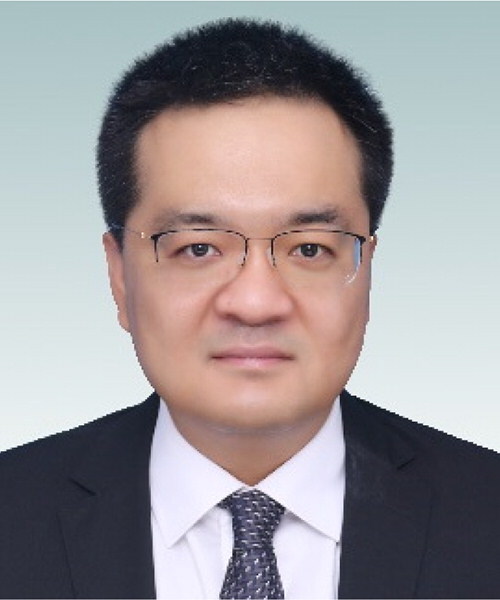
Hailong Liu
Hailong Liu is a Professor in the School of Energy and Power Engineering at Jiangsu University. He received his Ph.D. degree at Gyeongsang National University, South Korea in 2013. His research interests include non-Newtonian complex flow, flow measurement technology, multiphase flow, and heat transfer in new energy devices. He has undertaken more than 8 projects of the National Natural Science Foundation of China, the Ministry of Education and Jiangsu Provincial Department of Science and Technology.
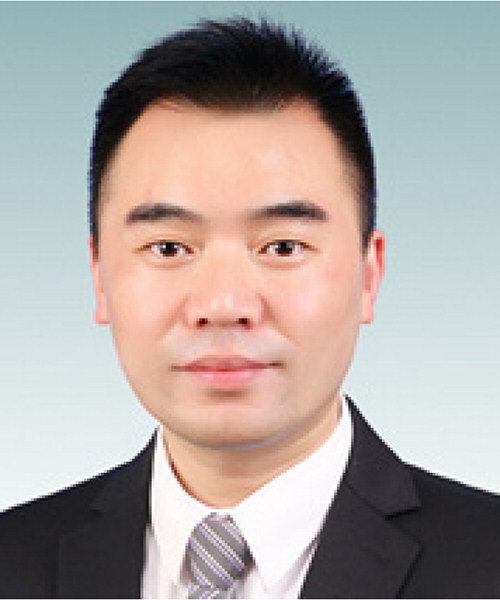
Junfeng Wang
Junfeng Wang is a Professor in the School of Energy and Power Engineering at Jiangsu University. He is mainly engaged in the research field of multiphase flow, heat transfer and complex flow in energy and power engineering, including electrostatic spray, CFD and modern measurement technology application in fluid flow. As the person in charge of the projects, he has undertaken more than 30 projects of the National Natural Science Foundation of China, the Ministry of Science and Technology and the projects from Industries. He has published more than 200 academic papers in journals and has been authorized more than 30 patents. He was awarded seven provincial and ministerial awards for scientific and technological achievements and two national awards for teaching achievements.