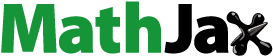
Abstract
We study the problem of forming groups of students so that the groups are as even as possible with respect to certain aspects and group members are changed as much as possible compared to previous groups, and formulate it as a mixed integer programming problem. We find that standard software cannot solve real life sized instances, so we develop several heuristics and metaheuristics for the problem. Computational tests are made on randomly generated instances as well as real life instances. Some of the heuristics give good solutions in short time, and tests on real life problems indicate that satisfactory solutions can be found within 60 seconds.
1. Introduction
In the IT-programme at Linköping University, the students are each semester divided into base groups, as a consequence of the problem based learning profile used. Each base group contains around seven students, depending on the total number of students, which is between 20 and 40. This is done in five semesters in sequence. Goals of the base group work are that each student should be active, participate in the discussions, and be able to work together with many different persons.
When forming a base group for a semester, some aspects are taken into account. First of all, the number of students should be the same, if that is possible, or otherwise as similar as possible.
One wish is that the students should as much as possible have different comrades in the base group from semester to semester. The students should learn to work together in groups, and this is best done if the groups are as different as possible.
Another aspect is that after some time, the students show different progress in their studies. This is quantified by the number of examination points each student has obtained. While this measure does not exactly show how well each student will do in the base group work, it is still preferred that all the best students should not be in the same group and all the worst students should not be in the same group.
There are more aspects that may be interesting, for example gender. One might want to have similar mixes between boys and girls in the groups, especially if one gender is much less represented than the other. There are also other aspects which one might like to divide evenly among the groups, such as age, various computer skills etc. We will include a list of aspects that may include whatever one wishes, and the goal will be to spread the measures of the aspects as evenly as possible between the groups.
The formation of base groups has in our case previously been done by hand, based on the information of each student’s points and all the previous groups the student has been in, and to some extent the gender. Sometimes additional information has been taken into account, such as two students not working well together, or two students working too well together (in which case they might dominate the group and give less room for the other students in the group).
In this paper, we describe a new optimisation model for the formation of good groups, and some experiences in using it. In our tests, we first take the three factors number (number of students in each group), points (total number of points of the students in a group) and repetition (a measure of how much two students have been in the same group in previous time periods) into account, but open the model for more aspects, such as gender etc, as discussed in Graf and Bekele (Citation2006).
Considering literature in the area, we find a recent overview over some existing group formation approaches in Maqtary, Mohsen, and Bechkoum (Citation2017). Another algorithm for group formation, GroupAL, can be found in Konert, Burlak, and Steinmetz (Citation2014). In Craig, Horton, and Pitt (Citation2010) “reasonably optimal” groups are formed with genetic algorithms, and in Agustín-Blas, Salcedo-Sanz, Ortíz-García, Pérez-Bellido, and Portilla-Figueras (Citation2008) a hybrid grouping genetic algorithm is used. In Graf and Bekele (Citation2006) ant colony optimisation is used, while Yeoh and Nor (Citation2011) propose an ad-hoc heuristic. Spoelstra, Rosmalen, van de Vrie, Obreza, and Sloep (Citation2013) discuss several different measures, while Dascalu, Bodea, Lytras, de Pablos, and Burlacu (Citation2014) focus on an interactive system, containing particle swarm optimisation. Other publications dealing with the issue in various ways are Ounnas, Davis, and Millard (Citation2009), Cocea and Magoulas (2010), Tourtoglou and Virvou (Citation2010), Abnar, Orooji, and Taghiyareh (Citation2012), and Cocea and Magoulas (Citation2012). We have not found any mixed-integer programming model in the literature that resembles ours and takes our aspects into account. Especially, the wish to avoid historical repetition is not present in any of the papers we have found. Often pairwise properties are used, rather then the group properties we use. Furthermore, we allow any number of aspects.
Another difference between our work and most others is that we do not use any input from the students, i.e. in principle all of our indata are undisputable numbers coming from our student registration system and from participation in previous groups. We do not use any subjective opinions from the students.
2. The mathematical model
Specifically we consider the following problem. We have n persons which shall be divided into m groups. As shown in Henry (Citation2013) there are
different ways of doing this, and enumerating all possibilities has complexity
, Konert (Citation2015), so complete enumeration is not a possibility.
There are q previous time periods, in which the persons have been allocated to different groups. We let gjt be the number of the group that person j was allocated to in time period t. Apart from the number of persons in each group, we have p different aspects that we wish to spread as evenly as possible between the groups, the first one being the points each person has obtained, and the following anything that is of interest (for example gender etc). We let alj be the measure of aspect l for person j. For example, is the number of points person j has obtained. and
may be 1 if person j is female and 0 if person j is male.
Also needed are weights reflecting the importance we put on the different parts of the objective. We let wN be the weight on the wish that the number of persons should be the same in each group, and wT the weight on the wish that the persons should have different group partners from term to term. Furthermore, we let be the weight on the measure of aspect l, so
will be the weight on the wish that each group should have a good mixture of high and low points. It is only the relation between these weights that is interesting. However, they must reflect the scaling of the aspects, since the number of persons in our real life examples will be below 10, while the number of points will be up to 120. Unlike the previous indata, which is given with certainty, these weights may need to be adjusted so that they accurately model our wishes.
Furthermore, the disadvantage of putting two persons, that previously were in the same group, in the same group again, may decrease by time. It may not be that serious if they were in the same group several time periods ago. We call this discounting. Therefore we let be the (relative) weight of time period t, i.e. reflect how much we take previous groups into account. We let
be one for the most recent time period (the last time period in the model), and decrease the weights as we go back in time. One possibility (which is used in our computational tests) is to set
. If we do not use discounting, we set
for all t.
The basic variable in the model is xij which is equal to 1 if person j is put in group i, and 0 if not. We also need the variable yjk which is equal to 1 if persons j and k are put in the same group.
Furthermore, in order to control the properties of each group, we let vi be the number of persons in group i and zli the sum of the measure of aspect l in group i (so is the total number of points in group i, and
could be the number of girls in group i, etc). We will also use vL and vU as the lowest and highest number of persons in a group, and
and
as the lowest and highest sum of measure of aspect l in a group.
The first and most obvious constraint in the model is that each person should be allocated to one group.
Then, we need to get correct values of the additional variables. yjk should be equal to 1 if both person j and person k belong to some group i, i.e. if xij = 1 and xik = 1. We get the following constraints.
The values of v and z are simply obtained by summing up.
Then, we get the variable upper and lower bounds on these variables as follows.
Finally, we have the domain of the variables: for all i and j,
for all j and k,
for all i and l,
for all i,
for all l,
. (v will automatically become integer, and the same holds for z if all measures a are integral. However, in our real life example, half points are possible.)
Now, we need to formulate the objective function. The slightly difficult part is to minimise the negative effect of putting two persons in the same group. For this, it is more convenient to have the coefficients djkt = 1 if person j and person k were in the same group in time period t. These coefficients are easily calculated by initiating d to zero, and then setting djkt = 1 if gjt = gkt.
The total disadvantage of putting person j in the same group as person k is then
We multiply this by yjk, sum this over all persons j and k and put it in the objective function. (If we are using discounting, all of c will not be integer, so non-integral objective function values will be possible.)
Next we need to address the number of persons and points in each group. Since we know that , we simply wish to minimise
, with weight wN. The same reasoning applies to the number of points and other aspects.
Thus, we obtain the following objective function.
(We let cjj = 0, and can remove yjj from the model.)
One may note that we only take pairwise effects into account for previous groups. It is conceivable that certain subgroups of three or more persons may have other effects that what is measured by this. These factors were not possible to take into account when this was done by hand. What is possible in this model is to introduce a new aspect with the single purpose of keeping three or more persons apart, by letting alj = 1 for those persons and alj = 0 for the others, similarly to what was done for gender. In general, this optimisation model allows taking more aspects into account then what is possible by hand.
Let us sum up the mathematical model.
(1)
(1)
(2)
(2)
(3)
(3)
(4)
(4)
(5)
(5)
(6)
(6)
(7)
(7)
(8)
(8)
(9)
(9)
(10)
(10)
(11)
(11)
(12)
(12)
The size of the model is as follows. There are mn x-variables, n2 y-variables, pm z-variables, m v-variables, plus the bounds, i.e. a total of
binary variables and
continuous variables. As for the constraints, there are n in the first group and
in the second. The following constraints are
, of which half are variable upper and lower bounds.
It is also possible to fix some of the bounds vL, vU, zL and zU. If n is an integer multiple of m, we may fix to get groups of equal size, otherwise we may fix
so that
.
This is a linear mixed integer programming model, with similarities to the generalised assignment problem and bin packing. The more specific part is the repetition, modelled by the y-variables.
3. Constructive heuristics
Preliminary tests (see section 5) reveal that the MIP-model is too hard to be solved by standard MIP-software. (We have tried GLPK and CPLEX.) Therefore we have developed a few constructive heuristics for finding feasible, hopefully good, solutions.
The heuristics sequentially build up a feasible solution, by allocating one person at a time to a group. Only information about the previously allocated persons are taken into account.
We start by sorting the persons in order based on the points. Then, we allocate the best person first, then the second best, and so on. In each step, when considering a certain person, we calculate penalties for the existing groups based on c, i.e. add a penalty if the previously allocated persons in a group has been in the same group as the person in question in earlier time periods. This is heuristic A.
In heuristics B, C and D, we also add penalties based on the number of persons in each group, and the total number of points in each group etc, as described below.
Let be the persons for which the group allocation has been done, and let αj be the group person j is allocated to. Also, let
be the set of persons that are allocated to group i, i.e.
.
The measures to keep into account are the following.
for all i (the number of persons in each group)
for all i and l (the measure of aspect l in each group)
for all i (the total repetition in each group)
With these, we sum up a total cost for allocating person j to group i as follows.
where
is given below, in different ways for the different heuristics. Then we simply choose the group that yields the minimal total cost.
After this we set ,
, and repeat for the next person.
In heuristic A, we set .
In heuristic B, we use the following, inspired by the objective function.
In heuristic C, we do a more elaborate evaluation of including person j in group i for the numbers and other aspects. If the group has less than the maximal number of persons, i.e. if , then adding a person to the group does not increase the maximal number of students in a group. Then, the penalty is set to zero. If
, then adding a person to the group will increase the maximal number of students in a group, and the penalty will be wN. Furthermore, if the number of persons in the group is equal to the minimal number, and all other groups have more persons, i.e. if
and
for all
, then the minimal number of persons in a group will increase, which is good. Then, we set the penalty to
.
The other aspects are handled similarly, but is slightly more complicated, since the coefficient alj may be other than zero or one. If (and
), then the penalty will be zero, while if
, we use the penalty
. If
, and
for all
, we use the penalty
.
Therefore, in heuristic C, we let and
, and calculate
for all i.
Furthermore, for each i, we let if
,
if
and
for all
, and
otherwise. We then use
In heuristic D, we take this further and calculate the exact increase/decrease of the bounds for the aspects. For the numbers, there is no difference, but for the other aspects, we find the following. If , then the upper bound will not increase by alj, but only by
, so the penalty will be
.
Furthermore, the increase of the lower bound will be , so the penalty will be
.
So in heuristic D, we use
Otherwise, we use the same values as in heuristic C.
A partly manual version of the heuristics has been constructed. The difference is only that the allocation is made by the user. The method loops over the persons, highest points first, presents the current situation (of the partly fixed solutions, and
for each i) and asks the user to decide in which group to put the person. This is possible as long as the number of groups is not to large. In our real life case m is around 5, and we believe that the user will be able to quickly scan five values. It is also possible for the user to deviate from the given weights by taking certain aspects more into account, and to take other (non-numerical) aspects into consideration.
4. Local search and metaheuristics
It is also possible to use metaheuristics on the problem, and we have chosen tabu search, Glover (Citation1989), Glover (Citation1990), and simulated annealing, Kirkpatrick, Gelatt, and Vecchi (Citation1983), Černý (Citation1985). Considering neighbourhoods for local search, we can use move, i.e. moving a person from one group to another, and swap, i.e. swapping groups between two persons. We have implemented the following methods with these two neighbourhoods.
Method 20: Heuristic A.
Method 21: Start by heuristic A. Then check all move-neighbours once, directly do all changes that yield improvement.
Method 22: Start by heuristic A. Then check all swap-neighbours once, directly do all changes that yield improvement.
Method 23: Start by heuristic A. Then check all move-neighbours repeatedly, doing all changes that yield improvement, until no more improvement is obtained.
Method 24: Start by heuristic A. Then check all swap-neighbours repeatedly, doing all changes that yield improvement, until no more improvement is obtained.
Method 25: Start by heuristic A. Then use methods 23 and 24 mixed, i.e. iterate between move and swap moves, doing all changes that yield improvement, until no more improvement is obtained.
Method 26: Start by heuristic A. Then run tabu search with moves. In each step the best move is made.
Method 27: Start by heuristic A. Then run tabu search with swaps. In each step the best swap is made.
Method 28: Start by heuristic A. Then run simulated annealing with moves.
Method 29: Start by heuristic A. Then run simulated annealing with swaps.
Methods 30, …, 39 are the same as 20, …, 29, but initiated with heuristic B.
Methods 40, …, 49 are the same as 20, …, 29, but initiated with heuristic C.
Methods 50, …, 59 are the same as 20, …, 29, but initiated with heuristic D.
Methods 60, …, 69 are the same as 20, …, 29, but initiated with a random solution.
5. Computational tests
The model is implemented in GMPL and solved by glpsol. Calculation of the coefficients d and c is done by a code in Python, which also reads indata in a simple format and writes an indata file in GMPL/AMPL-format. The heuristics are also implemented in Python. Some tests are also done with CPLEX, just in order to check if a better code easily solves all problems of interest.
The main tests are run on computer 1, which is an Acer Aspire X3 X3995, Intel Core i7-3770, 3.4 GHz, running Linux, Fedora Core 19, and the CPLEX-tests are run on computer 2, which is a HP Compaq 8100 Elite, Intel Core i5-650 3,2 GHz, running Linux, CentOS 6. Only one processor was used during the runs with the Python code and GLPK, while CPLEX uses more (up to 4). The CPU-time was limited to 60 seconds.
The tabu search was made with a tabu list length of 7, and 100 iterations. For simulated annealing, the starting temperature was 100, the cooling factor 0.7, and 50 outer iterations was made, each with 20 inner iterations with the same temperature. We did not attempt to optimise the values of these parameters, so it is likely that the results can be improved somewhat by tuning of the methods. (Therefore, we believe that a comparison to GLPK is more relevant than to CPLEX or another highly optimised code.)
5.1. Test problems
Some artificial test problems of different sizes were generated and solved. There is only one aspect in the first set of instances, namely the points, which were randomly generated between 10 and 100. The group allocation in the first of the previous time periods was to put person 1 in group 1, person 2 in group 2, …person m in group m, person m + 1 in group 1, person m + 2 in group 2, etc. The subsequent allocations were random permutations of the first. The parameter values are and
, and we use discounting. The maximal size of these instances is n = 35, m = 5 and q = 4, which is the approximate size of the real life instance we wish to solve.
The second set of test problems contains more instances of different sizes. They are similar to the first, but with other weights, namely and
.
The third set of test problems has two aspects (points and gender), but the same parameter setting as the second set. In order to better investigate the limits of our solutions methods, more instances with sizes at the higher end are used. The maximal size of these instances is n = 40, m = 6 and q = 5.
Finally a fourth set of problems contains three real life instances, which however are rather similar.
The two first sets of problems have been used for preliminary tests, mainly to see how far exact optimisation with the MIP-model can go. The third and the fourth sets of problems are considered to be the main tests.
5.2. Computational results
In , we give the results by the first tests with GLPK on computer 1. The table gives problem size, in the form of n, m and q, as well as the number of rows, columns and binary variables. The number of nodes in the branch-and-bound tree is given, as well as the solution time and the objective function value (rounded). If the CPU-time of 60 seconds was not enough to finish the solution procedure, we report the number of nodes at termination, the remaining nodes + those investigated, as a lower bound on the total number of nodes, and the best integer solution found, as an upper bound on the optimal value.
Table 1. Results by GLPK on the first problem set.
give the resulting objective function values of the initial constructive heuristics, on computer 1. Here we see that several methods yield the optimal solution to inst1-1, and a couple for inst1-2. Some other heuristics, however, yield solutions that are not very good. For inst-13, inst1-4 and inst1-5, GLPK yields the best solutions, which are optimal for inst1-3 and inst1-4. For inst1-6, method 32 gives the best solution, while for inst1-7, method 22 gives the best solution. Preliminary conclusions are that GLPK cannot solve the larger problems to optimality, and that heuristics using swap seem to be stronger than heuristics using move. The simple methods 20 and 30 may give quite bad solutions, see for example inst1-3 and inst1-4.
Table 2. Results (objective function values) by the heuristics on the first problem set.
give the results for CPLEX (on computer 2). Here we see that CPLEX is better than GLPK, but not good enough to solve the larger problems to optimality in 60 seconds. (There is one unit difference in the optimal objective function value for some instances, which is due to different rounding in the output.)
Table 3. Results by CPLEX, computer 2, on the first problem set.
A conclusion of the firsts tests is that problems of realistic size cannot be solved by GLPK or CPLEX. Tests allowing more than 60 seconds reveal that the time is increasing quickly, and solving for example inst1-5 would take several hours. Another conclusion is that the initial heuristics sometimes yield good solutions, but sometimes quite bad.
The second set of tests have and
, and discounting. The weight on the points is more realistic than in the first set, so we rely a bit more on these tests. Here we only made tests with the MIP-model, which again were unable to solve problems of interesting sizes. The results can be found in in the Appendix. We see that CPLEX is better than GLPK, but not efficient enough. Both codes run into difficulties when the number of persons is around 20 or more. Another observation is that inst2-7 is easier to solve than inst2-6, although inst2-7 has one group more, and thus is a larger problem. The same holds for inst2-8 and inst2-9.
The third set of instances has two aspects, the points and the gender (or any other binary measure). In , we give the results from GLPK (on computer 1). We give n, m, q, p, followed by the number of rows and columns of the MIP-model, the total number of simplex iterations and the number of branch-and-bound nodes that were investigated. We give two objective function values, the first, obj1, is the optimal value, if optimality was verified, which can be seen by a solution time less than 60, or the best upper bound that was found in 60 seconds. The second value is only given if optimality is not verified, and is the best lower bound that was obtained in 60 seconds.
Table 4. Results by GLPK on the third problem set.
Some reflections of are that increasing q, as done between inst3-3 and inst3-4 does not seem to increase the difficulty of the problem. Increasing n, as between inst3-5 and inst3-6, definitely makes the problem harder to solve. And again we find that increasing m, as between inst3-6 and inst3-7, may make the problem easier to solve.
In in the Appendix, we give the results for all the heuristics, methods 20 - 69. We have also added the results from GLPK, as method 1, for comparison. The tables give the instance name on top, the name of the method, followed by the objective function value and the solution time for each instance. All these test are made on computer 1.
Here we find that for small problems, some heuristics give the optimal solution in a fraction of the time needed by GLPK. For larger problems, some of the heuristics yield better solutions in much shorter time. In no case does GLPK yield a better solution than all heuristics (in 60 seconds).
In more detail, we find the following. For the smallest problem, inst3-1, many methods yield the optimal solution (377). The fastest of those is method 22 (as well as 32, 42, 52 and 62), which only needs 0.02 seconds. For inst3-2 even more methods yield the optimal value (339), and here methods 41 and 50 are the fastest. Similar results hold for inst3-3 and inst3-4, where the best method 62 (and 32) yield the optimal solution in 0.03 seconds.
For problems up to this, GLPK is a viable option. However, for inst3-5 and upwards, GLPK needs more time than the alternatives. For inst3-5 only four methods give the optimal value (2534), with method 49 as the fastest, 0.57 seconds.
For inst3-6, GLPK failed to find the optimum within 60 seconds. The best solution was found by five methods, of which method 64 is the fastest, 0.17 seconds.
Inst3-7 is slightly easier than inst3-6, and here GLPK and six other methods give the optimal solution. Method 62 does this in 0.12 seconds, while GLPK needs 26 seconds.
From inst3-8 and upwards, GLPK fails to find and/or verify the optimum within 60 seconds, so for these problems, we do not know the optimal solution. For inst3-8, method 57 gives the best solution in 17.63 seconds. One interesting question is how fast a method can yield a solution that is better than what GLPK gave in 60 seconds. Here, method 64 does this in 0.36 seconds.
For inst3-9, method 37 gives the best solution in 21.33 seconds. Here 29 methods give better solutions than GLPK, often in much shorter time, the fastest being 0.21 seconds for methods 32 and 42.
For inst3-10, method 47 gives the best solution, in 29.29 seconds. Also, here many (32) methods give better solutions than GLPK, and the fastest is 0.06 seconds for method 61.
For inst3-11, method 57 gives the best solution, in 30.18 seconds. Here, the GLPK-solution is rather good, and only 6 methods find better solutions.
For inst3-12, method 67 gives the best solution, in 40.61 seconds, while method 32 finds a better solution than GLPK in 0.40 seconds.
For inst3-13, method 67 gives the best solution, in 53.10 seconds, while methods 22 and 32 give a better solution than GLPK in 0.52 seconds. For inst3-14, method 37 gives the best solution, in 60 seconds. Here, the method is terminated because of the time limit of 60 seconds. Even so, it found a much better solution than GLPK. A slightly better solution than GLPK was found in 0.02 seconds by method 50. For these more difficult problems, we find that GLPK does not find a good solution at all within 60 seconds, so it is easy for a heuristic to find a better one.
For inst3-15, method 65 gives the best solution, in 2.71 seconds. For inst3-16, method 67 gives the best solution, in 60 seconds. For both these instances, a simple method as 20 finds a better solution than GLPK in 0.02 seconds.
For inst3-17, method 64 gives the best solution, in 5.23 seconds. For inst3-18, method 47 gives the best solution, in 60 seconds. For inst3-19, method 47 gives the best solution, in 60 seconds. For inst3-20, method 67 gives the best solution, in 60 seconds. For these harder problem, the stronger heuristics are terminated because of the maximal time allowed.
Concerning the comparison to GLPK, we find that for easy problems, is is not that hard to find the optimal solution. For hard problems, GLPK gives a quite bad solution in 60 seconds, so then it is easy to find a better solution. In intermediate cases, GLPK finds a rather good solution, so the heuristics need to work more to find a better solution, and some of them fail to find a better solution. However, we can note that in all cases, some heuristic finds a better or equal solution in much shorter time.
Summing up the number of times a method gives the best solution in shortest time (of the ones found), we get the following result. One best solution was found by the methods 22, 41, 42, 49, 50, 52 and 65. Many of these were best only for the smallest problems. Two best solutions were found by methods 32, 57 and 64. Three best solutions were found by method 47. Four best solutions were found by methods 62 and 67. Five best solutions were found by method 37, and this is the maximum, so by this measure, method 37 would be a winner. However, methods 47 and 67 are also rather strong.
All in all we find that methods ending with 7, i.e. use tabu search with swaps, are quite strong. They take more time than many other heuristics, but yield better solutions.
A comparison of the simple and very fast one-shot heuristics 20, 30, 40, 50 and 60 is made in . The time is in principle negligible for all of them, so the question is simply which one gives the best solutions. Obviously the random solution (60) is worse by far (which however does not stop it from giving good final results of the metaheuristics). Apart from this, the conclusions are not very evident. Summing up, method 20 gives the best solution in 4 cases, method 30 in 4 cases, method 40 in 10 cases and method 50 in 5 cases. Thus each method has its merits, but method 40 seems to be slightly better than the others. It is interesting to see that the simple method 20 is almost as good as the more advanced method 50.
Table 5. Results for one-shot heuristics.
Finally, tests were made on real life data. The first problem has n = 31, m = 5, q = 4 and p = 1, which yields 4868 rows, 1130 columns, 15,284 non-zeros and 1116 binary variables in the MIP-model. The second problem has n = 30, which gives 4562 rows, 1064 columns, 14,327 non-zeros and 1050 binary variables. The third problem has p = 2 (points and gender), but is otherwise similar to the first problem. gives the results of these tests for GLPK, and in the Appendix gives the results for the heuristics.
Table 6. Results by GLPK, computer 1, on the fourth problem set.
For all of these three problems, method 20 gives a better solution in 0.01 seconds than GLPK did in 60 seconds. These three problems also have in common that method 37 yields the best solution (using all the 60 seconds).
For the first problem, the objective function value of GLPK was 2592 after 60 seconds, while heuristic 20 yields objective function value 2530 after 0.01 second. Local search with move-neighbourhood (method 21) decreased it slightly to 2526. Local search with swap-neighbourhood (method 22) decreased it to 2493. Continued local search (methods 23 and 24) does not improve these values, but using both neighbourhoods (method 25) improves it to 2323. Tabu search with move-neighbourhood (method 26) gave the value 2396, while tabu search with swap-neighbourhood (method 27) decreased it to 2129. Using simulated annealing (instead of tabu search) (methods 28 and 29) yields 2478 and 2530, so here tabu search with swaps is the best method.
Starting with heuristic B (methods 30-39) gives similar results, most solutions somewhat worse, but slightly better for method 37. Starting with heuristic C (methods 40-49) gives in general worse solutions, and the same applies for heuristic D (methods 50-59) as well as starting with a random solution (methods 60-69).
Similar results were obtained for the second and third problems.
Methods 30 and 20 are very similar to the procedure used when this is done by hand, and it is interesting to see that both these give a better solution than GLPK in a much shorter time. For these problems, the simple heuristics give better solutions than GLPK very quickly, and tabu search improves these solutions further. However, in a fair amount of cases, a random starting solution (which may be very bad) enables the metaheuristics to find even better solutions.
5.3. Summary of the computational tests
We find that for small instances, a MIP-solver can be used, but when the size grows, it is better to use heuristics. For large problems, even the simplest heuristics produce better solutions than the MIP-solver. Further improvement can be obtained by many of the methods, but the best method seems to be tabu search with swap neighbourhood. However, the performance of simulated annealing can probably be improved by tuning the parameters.
Considering the starting solution, it is interesting to see that although method 20 sometimes gives lower objective function value than method 30, the final result is often worse than starting by 30. Also, random starting points can yield good results. Heuristics C and D can be seen as more intricate extensions of A and B, so it is slightly surprising to see that they are not always better.
6. Practical use
As mentioned in the introduction, the formation of base groups has previously been done manually, five times each year. At each occasion two or three teachers were present and it took approximately two hours. The method used was a simplified version of heuristic A, in the following sense. Penalities were not explicitely calculated. An attempt to minimise repetition was made by considering previous groups. By allocating the sorted list of students in a cyclic manner, the best students were not allocated to the same group.
We cannot really compare our methods with the manual method, but we know that method 20 is better than the manual, so a comparison between method 20 and method 37 (which we here pick as the best one) gives a lower bound on the improvement obtained. The relative improvements are shown in .
Table 7. Comparison between methods 20 and 37.
We see that the size of the improvements vary much, but the average improvement is 40%. In other words, letting the computer work in 60 seconds gives in average 40% better results than manual work for a couple of hours.
The code was supplied with an interface in Python and Tkinter that allows selection of solution method and changing of all parameters. The groups and all names are displayed on the screen (which is possible for 30 students and 5 groups but not much more). In addition, manual changes, such as inserting students in groups, removing students, fixing parts of the solution etc. are possible.
First the code was used by myself for a couple of years, yielding groups for all semesters for the IT-programme, and also for the psychology programme.
Then, the code was made available to the teachers responsible for the semesters, together with a manual. It turned out that removing many possibilities from the interface improved usability for inexperienced users (albeit university lecturers). By now all groups are made by the responsible teachers themselves. They are able to test different values of the parameters and observe the differences in the result.
In practice, this tool has enabled fast decisions, so that more recent data can be used. There are exams just before the groups should start, but previously the results of these exams could not be included, since the grouping was planned before the exams were corrected. Now the grouping only takes 10 minutes, so indata have a better quality.
Futhermore, there are sometimes defections, i.e. students leaving at short notice. Now more information of that type can be included when doing the grouping.
An interesting aspect is that a good optimisation tool such as this puts focus on the quality of the indata. Previously, the grouping was done so approximately that inaccurate indata was not considered a problem. There was never time to reflect on this when using the manual method. Now everyone can see how a change in indata affects the solution.
A general opinion is that the groups work well, and no complaints have been made. If there were better ways of obtaining feedback, one could put more effort in trying to find the best values for the weights w.
In the psychology programme (where there are more girls than boys), it turned out that one does not want a single boy in a group. Instead there should be either zero or at least two. This introduced a complicated non-convexity, which in theory requires further development of the model and methods.
However, in practice it was easier to include a possibility of fixing one gender and optimise over the other. First, an optimal allocation of only the (few) boys were made to the number of groups that were needed, and then this solution was fixed and the girls added. It meant deciding a priori the number of groups that should consists of only girls.
7. Conclusions and future work
We have formulated the problem of forming suitable groups as a MIP-problem. Computational tests reveal that standard MIP-codes, such as GLPK and CPLEX, cannot solve problems of realistic size.
We present a couple of constructive heuristics for the problem, and describe how metaheuristics, such as tabu search and simulated annealing, can be used to improve the solutions. Computational results are presented, showing that tabu search with swap neighbourhood is a good method for the problem.
The code, together with an interface in Python and Tkinter that allows manual changes, has been used when forming certain student groups at Linköping University. It has saves much manual time, and produces good groups, as reported by the users.
The code is presently not public domain, but such a version might be made later. Also, the test data may be available. Please contact the author, [email protected], for more information.
A direction for future research is to model and handle non-convexities, for example that a group should contain either zero or at least two of a certain type of students. Another obvious direction is to improve the solution methods. Furthermore, the current interface is mostly suitable for research, since it allows changing all method parameters. Another version for users only interested in the result, not in comparing different solution methods, will be made. That version may also contain a more general/standardized input format. Finally we are searching for better ways of evaluating the final groups, so that feedback can enable further improvements.
In practice, this tool has enabled fast decisions, so that more recent and accurate data can be used. The speed also enables testing of different weights and even new aspects.
Disclosure statement
No potential conflict of interest was reported by the authors.
References
- Abnar, S., Orooji, F., & Taghiyareh, F. (2012). An evolutionary algorithm for forming mixed groups of learners in web based collaborative learning environments. In IEEE International Conference on Technology Enhanced Education (ICTEE). Amritapuri, India.
- Agustín-Blas, L. E., Salcedo-Sanz, S., Ortíz-García, E. G., Pérez-Bellido, Á. M., & Portilla-Figueras, A. (2008). Assignment of students to preferred laboratory groups using a hybrid grouping genetic algorithm. In 8th International Conference on hybrid intelligent systems (HIS 2008), September 10–12, 2008, Barcelona, Spain (pp. 48–52). Retrieved from https://doi.org/10.1109/HIS.2008.37
- Černý, V. (1985). Thermodynamical approach to the traveling salesman problem: An efficient simulation algorithm. Journal of Optimization Theory and Applications, 45(1), 41–51. Retrieved from http://dx.doi.org/10.1007/BF00940812
- Cocea, M., & Magoulas, G. D. (2010). Group formation for collaboration in exploratory learning using group technology techniques. In R. Setchi, I. Jordanov, R. Howlett, & L. Jain (Eds.), 14th Interntional Conference on knowledge-based and intelligent information and engineering systems (Vol. 6277, pp. 103–113).
- Cocea, M., & Magoulas, G. D. (2012). User behaviour-driven group formation through casebased reasoning and clustering. Expert Systems with Applications, 39, 8756–8768.
- Craig, M., Horton, D., & Pitt, F. (2010). Forming reasonably optimal groups: (Frog). In Proceedings of the 16th ACM International Conference on supporting group work (pp. 141–150). ACM, New York, NY, USA. Retrieved from http://doi.acm.org/10.1145/1880071.1880094
- Dascalu, M.-I., Bodea, C.-N., Lytras, M., de Pablos, P. O., & Burlacu, A. (2014). Improving e-learning communities through optimal composition of multidisciplinary learning groups. Computers in Human Behavior, 30(0), 362–371. Retrieved from http://www.sciencedirect.com/science/article/pii/S0747563213000253
- Glover, F. (1989). Tabu search - part I. ORSA Journal on Computing, 1(3), 190–206.
- Glover, F. (1990). Tabu search-part II. ORSA Journal on Computing, 2 (1), 4–32.
- Graf, S., & Bekele, R. (2006). Forming heterogeneous groups for intelligent collaborative learning systems with ant colony optimization. In Intelligent Tutoring Systems (pp. 217–226). Springer, Berlin, Heidelberg.
- Henry, T. R. (2013). Creating effective students groups: An introduction to groupformation.org. In (pp. 645–650). Proceeding SIGCSE '13, Proceeding of the 44th ACM technical symposium on Computer science education. ACM New York, NY, USA.
- Kirkpatrick, S., Gelatt, C. D., & Vecchi, M. P. (1983). Optimization by simulated annealing. Science (New York, N.Y.), 220(4598), 671–680. Retrieved from http://www.sciencemag.org/content/220/4598/671.abstract
- Konert, J. (2015). Interactive multimedia learning. Springer Theses, Switzerland: Springer International Publishing.
- Konert, J., Burlak, D., & Steinmetz, R. (2014). The group formation problem: An algorithmic approach to learning group formation. In C. Rensing, S. de Freitas, T. Ley, & P. J. Muñoz-Merino (Eds.), Open learning and teaching in educational communities (pp. 221–234). Cham: Springer International Publishing.
- Maqtary, N., Mohsen, A., & Bechkoum, K. (2017). Group formation techniques in computer-supported collaborative learning: A systematic literature review. Technology, Knowledge and Learning. Retrieved from https://doi.org/10.1007/s10758-017-9332-1
- Ounnas, A., Davis, H. C., & Millard, D. E. (2009). A framework for semantic group formation in education. Educational Technology & Society (Vol. 12, pp. 43–55). In 8th IEEE International Conference on Advanced Learning Technologies. Santander, Cantabria, Spain.
- Spoelstra, H. V., Rosmalen, P., van de Vrie, E., Obreza, M., & Sloep, P. (2013). A team formation and project-based learning support service for social learning networks. Journal of Universal Computer Science, 19, 1474–1495.
- Tourtoglou, K., & Virvou, M. (2010). Simulated annealing in finding optimum groups of learners of UML. In G. Tsihrintzis, E. Damiani, M. Virvou, & R. Howlett (Eds.), 3rd international symposium on intelligent interactive multimedia systems and services (Vol. 6, pp. 147–156). Springer, Berlin, Heidelberg.
- Yeoh, H. K., & Nor, M. I. M. (2011). An algorithm to form balanced and diverse groups of students. Computer Applications in Engineering Education, 19, 582–590.
Appendix
Table A1. Results by GLPK, computer 1, on the second problem set.
Table A2. Results by CPLEX, computer 2, on the second problem set.
Table A3. Results for instances inst3-1, inst3-2, inst3-3 and inst3-4.
Table A4. Results for instances inst3-5, inst3-6, inst3-7 and inst3-8.
Table A5. Results for instances inst3-9, inst3-10, inst3-11 and inst3-12.
Table A6. Results for instances inst3-13, inst3-14, inst3-15 and inst3-16.
Table A7. Results for instances inst3-17, inst3-18, inst3-19 and inst3-20.
Table A8. Results for instances instre1, instre2 and instre3.