Abstract
For strategic planning of healthcare services, understanding and accounting for future demand can support resource management. A hybrid modelling approach is well-placed to address these issues using demand projections with simulation for capacity planning. We present a methodology which conceptualises each stage as a collaborative activity in a strategic process. Medium-to-long term demand is uncertain, and depends upon internal and external factors. Our novel demand projections use both drivers of internal demand, and environmental scanning to identify external influences on demand. These create demand trajectories for each of three procedures that are used with discrete-event simulation to inform strategic planning for endoscopic diagnostic services. The study involved collaboration with a major Healthcare Trust in England, and demonstrates the applicability of our methodology to support strategic change at the regional service level by aligning external demand with internal resource planning.
1. Introduction
The impact of unexpected changes in the landscape of healthcare is against a background of long-term demographic change, and the associated challenges of an increasing and ageing population. Healthcare organisations worldwide have been responding to the increase in demand for services by prioritising efficiency of delivery and optimisation of resource allocation. In a publicly-funded healthcare system such as the UK National Health Service (NHS), rising demand is met with constrained budgets, heightening the importance of strategic planning. Alongside internal process analysis, this requires monitoring the external environment and taking effective decisions and actions based upon that analysis. Dyson et al. (Citation2007) argued that OR is well-placed to address strategic issues by evaluating strategies prior to action. Systems approaches such as system dynamics (Torres et al., Citation2017) and participatory methodologies including facilitated modelling (Eden et al., Citation2019; Eden & Ackerman, Citation1998; Howick & Eden, Citation2011; Montibeller & Franco, Citation2011) have been commonly applied to strategic problems in OR, largely because the future is uncertain, and planning for extended horizons is rarely underpinned by adequate data. Discrete-event simulation (DES) and other simulation methods have been used to support strategic planning in healthcare (Brailsford et al., Citation2009; Katsaliaki & Mustafee, Citation2011, Brailsford et al., Citation2019), however aligning medium-long term demand and capacity planning can be challenging, with most simulation studies opting to focus on one or the other. This avoids a key issue in strategic planning, balancing the long-term resources of the organisation with the changing external environment.
Most M&S frameworks include a stage in which the boundaries of the model are determined, a conceptual model of the problem is formulated, and input data is identified and prepared. Robinson (Citation2020) identified conceptual modelling framework development – organising and structuring the knowledge gained during conceptual modelling – as a grand challenge for conceptual modelling. To address both strategic demand and capacity planning, the uncertainty surrounding long-term demand needs to be addressed in the conceptual modelling stage to ensure the usefulness of the overall approach. One way of conceptualising M&S for strategic DES is through strategic process representation. For strategic processes to be effective, certain activities need to take place (Dyson & Foster, Citation1983). Dyson et al. (Citation2007) framed strategic development using the notion of “rehearsing strategy”: exploring internal and external environments, creating strategic initiatives, and rehearsing virtual performance. Kunc and O’Brien (Citation2019) summarised five key stages in strategy processes, including those of “rehearsing strategy,” and mapped business analytics tools to each according to their functional category: descriptive, predictive, and prescriptive. To realise all stages, different analytical techniques are used to support different activities in the strategic process, for example descriptive methods can analyse the internal and external environments, while prescriptive methods can explore the future impact of different options. Hybrid modelling is the application of simulation – usually considered a prescriptive method – combined with other methods and techniques (Mustafee et al., Citation2020; Mustafee & Powell, Citation2018; Tolk et al., Citation2021). In our previous work we have used a hybrid modelling approach with descriptive, predictive and prescriptive methods for short-term decision-making (Harper & Mustafee, Citation2019; Mustafee et al., Citation2018). In this paper, we are extending this approach to strategic decision-support through application to activities in a strategic process.
The work contributes to the strategic OR literature, using a novel, pragmatic hybrid methodology for strategic planning at the regional service level in healthcare. Our hybrid conceptual model is mapped to key strategic activities in a process which expresses strategy as balancing internal organisational resources with the external environment (Kunc & O’Brien, Citation2019). This provides a well-aligned conceptualisation of the purpose of the methods used in this paper, employed in a case study of a healthcare diagnostic service which implements demand projections and simulation. Our study uses population projections as a basis of forecasts, integrated with local historical demand data, and evaluation of the external environment using an environmental scanning approach. We believe this approach provides plausible forecasts to support planning, accounting for both demographic change and the influence of future policy. The methodology is applied to an endoscopy diagnostic service in one Clinical Commissioning Group (CCG) in a major health service in the South of England. It demonstrates the applicability of the approach to inform service planning at the local (CCG) level by combining long-term, uncertain forecasts of future demand with DES to support capacity decisions.
The remainder of the paper is organised as follows. Section 2 reviews the literature on hybrid modelling and strategic planning. Section 3 describes the hybrid model application in an endoscopy service; Section 4 discusses the contribution of the paper with respect to its application; and Section 5 concludes the paper.
2. Literature review
Hybrid modelling is the application of a simulation method with methods and techniques from the wider OR literature, claiming to better capture a representation of a problem situation (Mustafee et al., Citation2020; Mustafee & Powell, Citation2018). Gu and Kunc (Citation2019) argued that hybrid techniques for strategic problems possess important advantages by providing a more comprehensive and unified perspective to a real-world problem. Several studies have used demand forecasting combined with DES for strategic healthcare planning (Demir et al., Citation2017; Citation2018; Ordu et al., Citation2021) using statistical forecasting techniques. However this type of forecasting utilises historical data and comes with certain assumptions, namely that future patterns of demand for health services will replicate historical demand. Worrall and Chaussalet (Citation2015) described healthcare as dynamic by nature; reforms in policy and scope mean that modellers have the dilemma of taking into account the influences of past changes on increasingly limited intervals of historical data. Forecasting demand for a service can assist with capacity planning and allocation of resources, but when demand is influenced most strongly by external forces for which natural limits to governing factors exist, traditional extrapolative methods can be problematic. The use of Office for National Statistics (ONS) population projections has the advantage of taking into account the effects of growth in different sectors of the population on future demand, providing projections which influence demand for healthcare services (Mielczarek & Zabawa, Citation2021). However, population projections can account for only one factor driving demand for a service. Other external factors will influence demand, and these are likely to be specific to the service (Willis et al., Citation2018). As strategic planning has become more established in healthcare, forming strategy at the individual departmental level is becoming more integrated into the enterprise-wide planning process, enabling investigation of policy-level decisions which are likely to influence future demand for a service.
The uncertainty surrounding future demand for healthcare services is often addressed using two complementary strategies: that of effectuation, whereby organisations make strategies based on their current resources and contingencies, and causation, which requires predicting the future for planning goals and policies (Hauser et al., Citation2020; Sarasvathy, Citation2001). The more uncertain the future, the more organisations rely on effectuation, however effectuation offers no concrete goals for future planning (Jiang & Rüling, Citation2019; Yu et al., Citation2018). Public sector organisations differ from the private sector, in that they exist to serve the needs of society, and while they must adapt to external environmental conditions, these conditions differ between the two sectors (Kearney & Meynhardt, Citation2016). Environmental uncertainty for the public sector can be influenced by technology change, policy change, or fluctuations in priority at a strategic level. Simultaneously, there is a requirement for public sector organisations such as healthcare to be effective and efficient in their service delivery and use of public funds. While effectuation is an important strategy for the public sector, where the future can be hard to predict and resources are limited, managers are required to meet organisational goals and objectives which may be external directives, such as government mandated operational targets. Hence, dealing with uncertainty requires an approach which monitors indicators of change in demand for a service, and analyses the meaning of these indicators. This has similarities with techniques used for technology scanning forecasting, which predicts future characteristics and timing of technology by continuously monitoring the signals of change in the technological landscape for the purpose of future planning (Van Wyk, Citation1997). The method involves scanning the environment to collect all available information about the field under investigation; filtering the information for relevance; then analysis and development of the forecasting model. Porter and Detampel (Citation1995) referred to this as Technology Opportunity Analysis, and described the monitoring or scanning phase as “the most useful technique in forecasting” (p. 238). For example, monitoring allows identification of causal variables with a view to exploiting opportunities for competitive success, positioning technology as a strategic asset. It is akin to horizon scanning, used to explore uncertain influencing forces for strategic planning (Willis et al., Citation2018), and can be applied in healthcare to identify and analyse environmental variables that influence demand to support the strategic goal of sustainable healthcare delivery.
For strategic planning, Kunc and O’Brien (Citation2019) summarised five key stages: (1) direction setting, concerned with the desired future direction that key decision-makers are aiming to follow; (2) analysing the environment consists of assessing the internal and external environments which may impact the service; (3) formulating strategic options consists of generating ideas for future strategic initiatives; (4) rehearsing and choosing options involves assessing and evaluating scenarios; (5) implementing strategy involves implementation, measuring and reviewing performance. illustrates these strategic activities, with applications in this study mapped to each stage. Stages 1 and 5 were instigated by the NHS host organisation, hence this paper describes the activities within the “Rehearsing Strategy” components of the strategic process (Dyson et al., Citation2007), i.e., Stages 2–4. Stage 2 analyses both the internal and external demand environments, Stage 3 formulates strategic options through simulation scenarios, and Stage 4 implements the DES. The arrows within the framework indicate the direction of action, and that activities are inter-related, in particular implementing chosen strategies does not suggest the end of the process. On the contrary, the process should be continuous and adaptable to respond to the changing environment through reconfiguration of resources and goals.
Figure 1. Activities within strategic processes: application approach (adapted from Kunc & O’Brien, Citation2019).
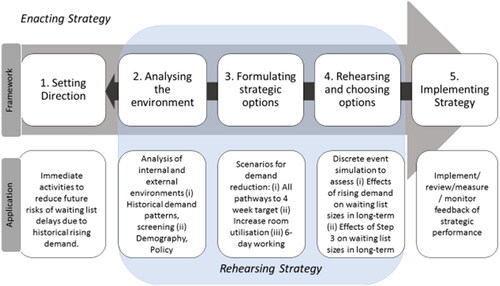
The next section describes the background to the problem, and implements Stage 2–4. The study was initiated in 2016/17 by the host organisation, triggered by unexpected rises in demand for endoscopy services caused by national policy changes aimed at improving cancer diagnoses. The service was interested in initiating a plan for the following 5–10 years to match resources to demand. Strategies for releasing capacity were well-understood but the effects of those strategies on uncertain demand were open questions.
3. Case study: Hybrid model application
3.1. Background
Gastrointestinal (GI) endoscopy describes a set of procedures for diagnosing cancers of the upper and lower gastrointestinal tract (stomach, oesophagus and bowel) and other conditions of the digestive tract. In the UK NHS, endoscopic investigations are requested by General Practitioners (GP) via an urgent two-week (2WW) referral for suspected cancer, or a routine six-week pathway (6WW) as NHS-measured operational targets. From June 2015, new referral guidelines increased the sensitivity of GP referral criteria for endoscopy (NICE, Citation2015). Where presentation criteria suggested a lower risk of cancer, this was interpreted as a four-week wait (4WW), measured within the 6WW target in some parts of the UK. The ongoing surveillance of high-risk groups is performed in conjunction with national guidelines, for example for those patients with a history of cancer. Evidence suggested that patients on surveillance programs were experiencing unacceptable waiting times due to delays within high-priority 2WW pathways (Brown et al., Citation2015). Those on 4WW pathways were a cause of delay to 6WW routine referrals, and subsequent cancer diagnoses within these referral pathways had the potential for negative downstream consequences.
Endoscopic demand is both age- and gender-related. Cancer is primarily a disease of the elderly, with approximately 12% of the population aged over 70 years accounting for more than half of the total cancer diagnoses in 2014 (ONS, Citation2014). Hence demand is regionally-specific, as population profiles vary. The health service involved in this case study serves a resident population of approximately half a million people. The area is attractive for retirement, so the proportion of those aged over 75 years, at 11%, is higher than the UK national average of 8% (ONS, Citation2016a, Citation2016b).
3.2. Stage 2: Analysing the internal and external environments
3.2.1. Data and methodology
The ONS provides subnational population projections to indicate the future size and age structure of the populations of English regions, local authorities and Clinical Commissioning Groups (CCGs). CCGs form the lowest level of health geography in England. ONS subnational projections were used for baseline forecasts (ONS, Citation2016b); older datasets were used for regression modelling in half years. CCGs were created in 2013, so the data available for CCG-level population prior to this was back-casted from the 2011 census (ONS, Citation2013, Citation2014). For short-term validation of ONS projections, “Number of Patients Registered at a GP practice by CCG” was used at April 2016, a quarterly snapshot of the number of patients per GP practice per CCG in England by age and gender (NHS Digital, Citation2016).
Anonymised hospital demand data was extracted from the patient administration system from June 2009 to June 2016. The data was filtered to three procedures: colonoscopy, esophagogastroduodenoscopy (OGD), and sigmoidoscopy. A primary assumption in the described method is that only patients within the CCG are referred into the health service; at more than 99.33% this assumption is deemed valid.
The demand projections in the next subsection describe: (i) A baseline minimum (demographic change), (ii) A baseline maximum (demographic change plus time-series regression); (iii) External demand projections using the baseline minimum combined with environmental scanning. This can be compared with the baseline maximum projections to account for those factors which influence future demand for judicious planning; (iv) The external forecasts are then adjusted for the effect of the Bowel Cancer Screening Programme (BCSP). summarises these processes.
3.2.2. External demand projections: Demographic change as a baseline minimum
Historical endoscopy demand data from 2012 was disaggregated into three procedures, gender, and five-year age groups. Patients presenting as part of the national bowel-cancer screening program (BCSP) and patients undergoing surveillance were analysed separately. Percentage-changes for each year of the 2016 ONS population projections were calculated by age-group and gender to 2026 and applied as a % uplift to endoscopy demand age-gender cohorts at 2015. Projections for each half-year were based on the previous actual half-year period. The data was then re-aggregated to give demand per procedure, with a static base rate of demand at 2015 (). This baseline projection assumes that the rate of demand per age-gender cohort will remain in line with projected population rates, isolating the effect of population growth and ageing on endoscopy demand.
Figure 3. Baseline minimum projections: demand (patient count) per procedure generated by demographic change (ONS, Citation2013, Citation2014, Citation2016a, Citation2016b). 2012–2016 are historical demand data.
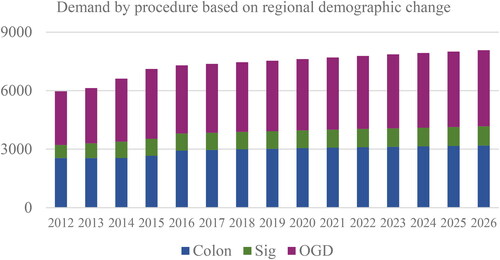
The demand as a percentage of population is relatively stable, as the projections use ONS data from a static historical rate of demand in 2015/16. The rate of projected population change (ONS, Citation2016a) is slow, increasing over ten years from 0.52% per year to 0.55% per year. However baseline projected demand is expected to outpace the rate of population growth (seen in ) because a disproportionate number of older patients use the service.
3.2.3. Internal demand projections as a baseline maximum
Historical proportion of demand relative to the population was calculated for each half year from 2012 to 2016. Using the disaggregated data for each procedure and age-group per year, a regression of demand as a percentage per unit population, and year, was calculated and projected to 2026. This was adjusted as a proportion of the 2015 demand rate, which was already accounted for in the population projection forecasts. These adjusted figures were multiplied by the baseline demand counts for 2016 onwards then re-aggregated to give projections per age-group, procedure and year. This gave projections that used both ONS population projections and historical demand per procedure. This is considered to be a maximum demand projections, accounting for demographic change, and assuming that historical trends will continue.
Historical hospital data shows an increase in overall demand from roughly 6000 procedures per year in 2009, to nearly 9000 in 2015, with females consistently higher users. Historical demand overall is strongly age-related for all procedures and all years, with the highest demand from those aged 55–80 years. A largely steady overall increase in population is seen in the CCG area from 2009 to 2015, with the highest proportion of population increase for those aged 71–80 years. A steady linear increase in the proportion of overall endoscopy demand (%) relative to the population is seen, from 2.09% in 2009 to 3.16% in 2015.
3.2.4. External demand projections: Demographic change with environmental scanning
The external projections are developed using an environmental scanning approach, which examines factors which have contributed to demand in the past, and factors that are likely to contribute to demand in the future, explicitly attempting to predict the impact of these factors on endoscopy demand. These are combined with the baseline minimum projections in Section 3.2.2.
Historical influences indicate that the major contributors to demand for diagnostic services are policy changes promoting earlier presentation of symptoms. A national set of public awareness campaigns across 2012–2015 (CRUK, Citation2016a, CRUK, Citation2016b) increased endoscopy demand by promoting early presentation and diagnosis for cancer symptoms. Reviewing the academic and grey literature with stakeholder collaboration identified:
Further campaigns were called for by the Independent Cancer Taskforce (Citation2015), the National Awareness and Early Diagnosis Initiative (NAEDI) (CRUK & NAIDI, Citation2016) and the Five Year Forward View Next Steps (NHS, Citation2017). In a commissioned report, Brown et al. (Citation2015) calculated that future public awareness campaigns would increase demand by 3.1% and it was assumed in our analysis that the campaign would be launched nationally before 2021. These came to fruition with a regional pilot launched in 2017, and a full campaign in 2020. The relatively low figure suggests that awareness does not necessarily translate into action.
A new 28-day diagnostic metric (now undergoing regional piloting) was expected to be launched nationally in April 2020 as recommended by the Independent Cancer Taskforce (Citation2015). It stated that 95% of patients referred for testing by a GP should be given a diagnosis within four weeks of referral (NHS, Citation2016; Citation2019a). The NHS was planning for half a million extra endoscopies as a result; 2014 figures suggested 1.37 million tests nationally excluding screening and surveillance, so national endoscopy counts were estimated for 2020 assuming the same proportion of the population are undergoing endoscopy in 2020, which may be an underestimate. This translated to a 26% increase for the regional service by 2020. This increase is expected to remain stable, with cancer targets named as a national priority until 2028 in the Long Term Plan (NHS, Citation2019b).
The combined influence of these two policies with demographic change, without taking into account public expectation, changes in technology, or shifts in migration, deprivation or modifiable risk factors suggested that future policies will continue to have a similar influence on future demand as historical influences, predicting 81% of the baseline maximum projection in Section 3.2.3. The demand projections (combined procedures) are plotted in . For individual procedures, this translates to an increase over ten years in OGD demand of 71%; for Colonoscopy a 40% increase; and for Sigmoidoscopy a 65% increase from 2016. In 2026, overall demand for endoscopy was predicted to rise 58% from 2016, with 3.9% of the projected population undergoing endoscopic investigation, compared to 3.16% in 2016. SPSS time-series modeller was used to generate comparison outputs to provide further confidence in the external demand projections (Supplementary Material A).
3.2.5. Adjusting colonoscopy demand projections for the effect of screening
The Bowel Cancer Screening Programme (BCSP) aims to detect bowel cancers at an early stage, before patients have any symptom. Screening has a direct effect on future patient arrivals, hence projections require adjustments. In 2016, there were two national programmes: (i) BCSP: Colonoscopy was offered every 2 years to those aged 60–74 years (changed from 60 to 69 years in 2012; 50–74 in 2021). Patients are sent a kit. If they choose to return it, and have a positive result, they are offered a colonoscopy; (ii) The Bowel Scope programme was a (now discontinued) one-off sigmoidoscopy offered to those aged 55 years. Patients diagnosed at screening will enter the system for ongoing surveillance. While this will have a future reduction effect on 2WW and 6WW arrivals, it is likely to generate more patients for surveillance. A model was constructed to calculate the effect of the two BCSPs on demand for colonoscopy services until 2026, using regional ONS population projections for the relevant age brackets, and cancer progression rates (CRUK, Citation2017). The projected numbers diagnosed by screening until 2026 were calculated as a proportion of the total projected colonoscopy demand to generate the expected proportional reduction in demand for 2WW/6WW appointments over time. This reduces over time (such that the impact on demand increases) as the effects of the enhanced BCSP in 2012 and the Sigmoidoscopy screening in 2014 begin to take effect (). Note that subsequent to this work, the BCSP has changed to include those aged over 50, and the Bowel Scope has been discontinued. Nonetheless, plausible estimates were calculated to determine the impact, given current circumstances at the time, of screening on demand for diagnostic endoscopy services. The approach used for these calculations is detailed in Supplementary Material B.
3.3. Stage 3: Formulating strategic options
In consultation with the host organisation, a set of scenarios were investigated:
The effects of changes to the distribution of 2WW/4WW/6WW referrals per procedure, including changing all pathways to 4WW (as per 28-day proposed operational target, Section 3.2.4).
To experiment with alternative capacity management strategies with the objective of proactive service planning toward the projected increases in demand:
Increasing room utilisation from average 90% to 99%.
6-day working.
3.4. Stage 4: Rehearsing and choosing options with DES
The DES model, implemented in Simul8 (https://www.simul8.com), was used for the stakeholder objective of estimating waiting list sizes and times year-on-year for endoscopic procedures by urgency route by taking into account the expected changes in demand annually over time. shows a flowchart of the process. The generation of GP patient referrals for each of the three procedures (colonoscopy, OGD and sigmoidoscopy) are based on the external demand projections (Section 3.2.4). Discrete distributions were fitted for each procedure/year (3 procedures over ten-year period, with a 1-year warm-up, a total of 33 Poisson distributions) to update input distributions each year at runtime. Each patient entity was assigned a value for a 2WW/4WW/6WW wait based on current proportions, and priority routing was used for each of these pathways.
As described by the flowchart in , patients may go straight from GP referral to endoscopy, where the 2WW/6WW operational target clock will stop (4WW are measured under the 6WW target). Alternatively, based on referral data (proportions vary per procedure) patients may wait for consultant review in a clinic (2WW/6WW target clock stops). All conditional probabilities are static 2016 parameters.
Triangular distributions define the time for each procedure, including turn-around time. The model runs for two, four-hour sessions per day, five days per week. The number of replications was investigated using cumulative means and confidence intervals (Robinson, Citation2004). These began to converge at 10 runs, but were stable by 20 runs, hence each scenario used 20 trials.
The hybrid model was used to investigate the effects of projected demand on the current daily wait list size, and on wait times and operational targets, per procedure and pathway over a ten-year period.
Both of these were examined with and without the reduction effect of screening over time on colonoscopy arrivals, by adjusting forecasted arrivals by the calculated annual reduction seen in ( and ). Note that the actual screening procedures use a separate allocated capacity pool to the diagnostic service. The simulation was calibrated with 2015/16 wait-list data. Supplementary Material C contains a STRESS checklist used to ensure unambiguous reporting of our study (Monks et al., Citation2019).
Figure 7. Simulated waiting list number of patients in queue (top) and waiting time in weeks (bottom) not accounting for screening until 2026.
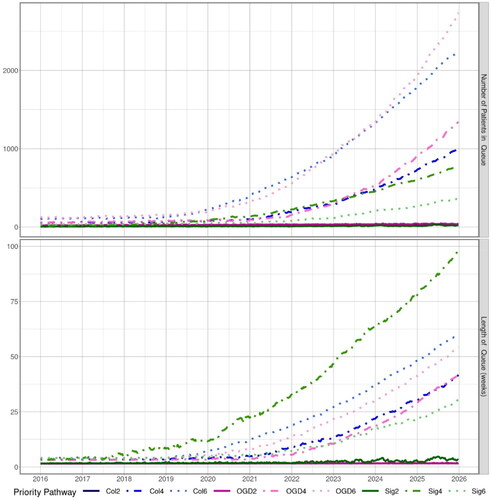
Figure 8. Simulated waiting list number of patients in queue (top) and waiting time in weeks (bottom) accounting for screening until 2026.
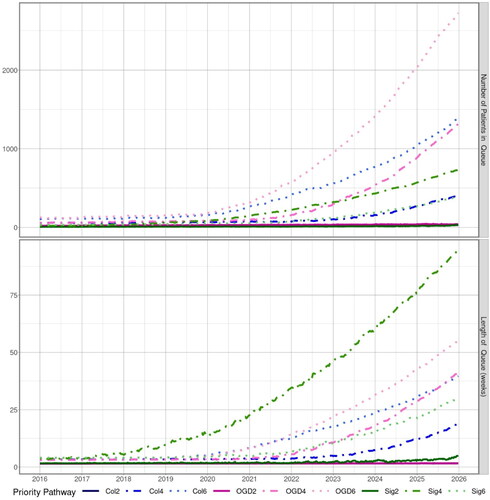
3.4.1. Results and implementation
The baseline cases (with and without screening) for patient numbers in the wait list, and waiting time in weeks are in and . In the first case, from 2018 onward waiting lists become unmanageable, in particular for 6WW and 4WW. The urgent 2WW targets are able to be met; queuing time outputs found that these patients do not exceed a 14 day wait by 2022. However patients waiting for a 4WW begin to exceed this in 2020, and 6WW waiting lists rose exponentially. Screening has a demonstrable effect on colonoscopy queues by directly reducing numbers arriving for appointments (). Despite this, the results indicated that demand was rapidly outstripping capacity, and the effects on surveillance (not shown) were an exponential rise.
Simulating scenarios (Section 3.3) found that when using a combined approach (), waiting times remained below 4 weeks until 2020. This approach simulated 6-day working and enhanced room utilisation, and set all targets to 4WW. Our stakeholders used these and other outputs as part of a wider decision-support process for strategic management of endoscopy demand, enabling internal efficiency changes to reduce the current wait list to a more manageable size over the subsequent twelve months. Additionally, the pragmatic process of exploring uncertain demand and capacity modelling with key stakeholders had wider benefits than model outputs. By enabling an understanding of the strategy process, this work initiated ongoing responses to the changing environment during the pandemic as the service commissioned additional modelling work to support future reconfiguration of resources and goals.
4. Discussion
Within the cancer pathway, endoscopy services have diagnostic, treatment and surveillance functions. In 2013–2015, UK endoscopy services found themselves managing a surge in demand resulting from a set of new national policies encouraging early presentation and referral. However, this demand was not entirely unpredictable. This study, in collaboration with healthcare stakeholders, had the specific objective of investigating how the host organisation might future-proof their service, given the increasing pressure nationally to accommodate improved outcomes in early cancer diagnosis and survival (NHS, Citation2019b).
As a diagnostic service, endoscopy demand is relatively unaffected by changes in disease prevalence or socioeconomic factors, but can be heavily impacted by policy changes, technical advances, fluctuations in government priority, and public expectations (Brown et al., Citation2015). Lack of anticipation of demand has been found to be a feature common in countries where endoscopy services do not directly control their income (Valori & Johnston, Citation2016). The sustainability of the NHS critically depends on having sufficient capacity, and local decision-makers can be supported to better anticipate long-term demand and plan their resourcing.
Willis et al. (Citation2018) described a multi-methodological OR approach to strategic workforce planning which started with external horizon scanning to undertake a systematic exploration of uncertain driving forces that could influence future requirements. For our study, stakeholders were interested in large perturbations in demand, as scenarios represented considerable time and financial investments. Effectuation as a strategic approach to planning requires comfort with risk: assessing available resources to achieve goals, while continuously balancing these goals with resources and actions (Sarasvathy, Citation2001). This is in contrast to causation, where decision-makers start with a predetermined goal. For health-service planning, arguably both approaches are required. Some demand can be reasonably predicted, and planned for. However, plans should be flexible enough to adapt to shifts in the environment. The process of exploring potential future demand enables enhanced stakeholder understanding of both the potential demand, and the practice of anticipating uncertain demand in itself.
In this study, future uncertainty was explored as part of a set of activities within the strategic process defined by Kunc and O’Brien (Citation2019) and Dyson et al. (Citation2007). We implemented this using a hybrid model with projections accounting for both internal (historical demand, bowel cancer screening) and external (future policy change, demographics) environments to create linear demand profiles. These were inputted into a DES to investigate capacity planning scenarios over 10 years. Within our host organisation, there existed a good understanding of potential interventions for strategic change, but limited awareness of future demand, and the impact of interventions on future demand. Our methods map to the stages of “rehearsing strategy” (Dyson et al., Citation2007), with Stages 1 and 5 (setting direction, and implementation) instigated by the NHS host organisation. Hence we were able to deliver value without utilising significant stakeholder time by supporting those stages that were outside the reach of the service using a pragmatic, easily replicated approach.
The hybrid modelling suggested that without investment, steady rises in demand would likely become exponential in a few years. Even prior to COVID-19, these findings were corroborated at the national level with a clear trend of increasing activity, with fewer UK services able to meet national waiting time between 2016 and 2019 (Ravindran et al., Citation2021). The host organisation indicated that both 6-day working and efficiency improvements toward theatre utilisation were implemented in the service to bring down waiting times and future-proof the service against prospective disruptions. However, the evolution and recovery from the pandemic and its resultant impact on health services, including endoscopy, have demonstrated that strategic planning is not a one-off exercise, and that not all perturbations can be planned for.
5. Conclusions and limitations
Our study contributes to the strategic OR literature by demonstrating a novel, real-world application of hybrid analytical modelling using a methodology which focuses on strategic activities to analyse the environment, formulate strategic options and rehearse and choose options. Descriptive, predictive and prescriptive methods often focus on solving complex operational problems using historical datasets. Kunc and O’Brien (Citation2019) and O’Brien (Citation2011) argued that by combining data analytics with insights and intelligence, data becomes a more valuable strategic resource. Stakeholder knowledge and assumptions should be combined with appropriate quantitative methods within the needs of strategic activities to balance internal organisational resources with the external environment (Kunc & O’Brien, Citation2019). Utilising stakeholder engagement within an M&S study enables qualitative data, including domain-expert knowledge and assumptions, to inform traditional methods using open-source and secondary hospital datasets. This collaborative approach supports the future-facing, visionary component of strategic development, combining causation with effectuation for future planning.
The model required several assumptions, for example, that the proportion of future use of services will remain stable per age-group. Given that endoscopy demand beyond demographic change is driven mostly by policy, this is considered an acceptable assumption. Additionally, accounting for the effects of screening on demand incorporated multiple high-level assumptions, however screening significantly impacts patterns of demand and cannot be excluded. Finally, the DES could only be validated using current data. Nonetheless, plausible demand projections present a more realistic baseline than static long-term inputs into a DES to support regional service-level planning.
Our study implemented a hybrid model for service-level strategic planning applied to endoscopy diagnostic services, though the application of key strategic activities. Strategic decisions are characterised by uncertainty, which requires an approach which monitors indicators of change in demand for a service, and analyses the meaning of these indicators, starting with an examination of past influences on demand. In a service whose demand and referral criteria are age-related, and which is volatile to changes in referral policy and public awareness, we identified future sources of internal and external demand, and estimated their likely future impact. DES enabled an estimate of the impact of this projected demand on waiting list sizes and lengths, and explored scenario options for increasing capacity to deal with this demand. Our hybrid approach is readily transferable to other regional endoscopy services, or other diagnostic services, such as breast screening or diabetic eye screening with a view to supporting long-term planning decisions and mitigating the impact of rapid demand fluctuations as a result of policy decisions on service delivery.
Supplemental Material
Download MS Word (175.4 KB)Acknowledgments
This report is independent research supported by the National Institute for Health Research Applied Research Collaboration South West Peninsula. The views expressed in this publication are those of the author(s) and not necessarily those of the National Institute for Health Research or the Department of Health and Social Care.
Disclosure statement
No potential conflict of interest was reported by the authors.
Additional information
Funding
References
- Brailsford, S. C., Eldabi, T., Kunc, M., Mustafee, N., & Osorio, A. F. (2019). Hybrid simulation modelling in operational research: A state-of-the-art review. European Journal of Operational Research, 278(3), 721–737. https://doi.org/10.1016/j.ejor.2018.10.025
- Brailsford, S., Harper, P. R., Patel, B., & Pitt, M. (2009). An analysis of the academic literature on simulation and modeling in health care. Journal of Simulation, 3(3), 130–140. https://doi.org/10.1057/jos.2009.10
- Brown, H., Wyatt, S., Croft, S., Gale, N., Turner, N., & Mulla, A. (2015). Scoping the future: An evaluation of endoscopy capacity across the NHS in England. Commissioned by Cancer Research UK. 2015.
- CRUK. (2016a). Bowel Cancer Campaign: Impact on Services- preparing for the campaign. Cancer Research UK. http://www.cancerresearchuk.org/health-professional/early-diagnosis-activities/be-clear-on-cancer/bowel-cancer-campaign/impact-on-services Accessed 22/04/2021
- CRUK. (2016b). Oesophago-Gastric cancers campaign: Impact on Services- preparing for the campaign. Cancer Research UK. www.cancerresearchuk.org/health-professional/early-diagnosis-activities/be-clear-on-cancer/oesophago-gastric-cancers-campaign/impact-on-services. Accessed 22/04/2021.
- CRUK & NAIDI. (2016). Early diagnosis initiative. https://www.cancerresearchuk.org/health-professional/diagnosis/early-diagnosis-initiative. Accessed 14/04/21.
- CRUK. (2017). Bowel cancer: Survival. https://www.cancerresearchuk.org/about-cancer/bowel-cancer/survival. Accessed 14/04/21.
- Demir, E., Gunal, M., & Southern, D. (2017). Demand and capacity modeling for acute services using discrete event simulation. Health Systems, 6(1), 33–40. https://doi.org/10.1057/hs.2016.1
- Demir, E., Southern, D., Rashid, S., & Lebcir, R. (2018). A discrete event simulation model to evaluate the treatment pathways of patients with cataract in the United Kingdom. BMC Health Services Research, 18(1), 1–15.
- Dyson, R. G., Bryan, J., Morecroft, J., & O’Brien, F. (2007). The strategic development process. In F. A. O’Brien & R. G. Dyson (Eds.), Supporting strategy: Frameworks, methods and models. Wiley.
- Dyson, R. G., & Foster, M. J. (1983). Effectiveness in strategic planning revisited. European Journal of Operational Research, 12(2), 146–158. https://doi.org/10.1016/0377-2217(83)90218-7
- Eden, C., & Ackerman, F. (1998). Making strategy: The journey of strategic management. Sage.
- Eden, C., Ackermann, F., & McKiernan, P. (2019). Stakeholders in Strategy: Their ‘invisible’ but significant presence. In Academy of Management Proceedings (Vol. 2019, No. 1, p. 10678). Briarcliff Manor, NY 10510: Academy of Management. https://doi.org/10.5465/AMBPP.2019.10678abstract
- Gu, Y., & Kunc, M. (2019). Using hybrid modelling to simulate and analyse strategies. Journal of Modelling in Management, 15(2), 459–490. https://doi.org/10.1108/JM2-02-2019-0039
- Harper, A., & Mustafee, N. (2019). Proactive service recovery in emergency departments: A hybrid modelling approach using forecasting and real-time simulation. In Proceedings of the 2019 ACM SIGSIM Conference on Principles of Advanced Discrete Simulation (pp. 201–204).
- Hauser, A., Eggers, F., & Güldenberg, S. (2020). Strategic decision-making in SMEs: Effectuation, causation, and the absence of strategy. Small Business Economics, 54(3), 775–790. https://doi.org/10.1007/s11187-019-00152-x
- Howick, S., & Eden, C. (2011). Supporting strategic conversations: The significance of a quantitative model building process. Journal of the Operational Research Society, 62(5), 868–878. https://doi.org/10.1057/jors.2010.103
- Independent Cancer Taskforce. (2015). Achieving world-class cancer outcomes: A strategy for England 2015-2020. https://www.cancerresearchuk.org/sites/default/files/achieving_world-class_cancer_outcomes_-_a_strategy_for_england_2015-2020.pdf Accessed 18.04.21
- Jiang, Y., & Rüling, C. C. (2019). Opening the black box of effectuation processes: Characteristics and dominant types. Entrepreneurship Theory and Practice, 43(1), 171–202. https://doi.org/10.1177/1042258717744204
- Katsaliaki, K., & Mustafee, N. (2011). Applications of simulation within the healthcare context. The Journal of the Operational Research Society, 62(8), 1431–1451. https://doi.org/10.1057/jors.2010.20
- Kearney, C., & Meynhardt, T. (2016). Directing corporate entrepreneurship strategy in the public sector to public value: Antecedents, components, and outcomes. International Public Management Journal, 19(4), 543–572. https://doi.org/10.1080/10967494.2016.1160013
- Kunc, M., & O’Brien, F. A. (2019). The role of business analytics in supporting strategy processes: Opportunities and limitations. Journal of the Operational Research Society, 70(6), 974–985. https://doi.org/10.1080/01605682.2018.1475104
- Mielczarek, B., & Zabawa, J. (2021). Modelling demographic changes using simulation: Supportive analyses for socioeconomic studies. Socio-Economic Planning Sciences, 74, 100938. https://doi.org/10.1016/j.seps.2020.100938
- Monks, T., Currie, C. S., Onggo, B. S., Robinson, S., Kunc, M., & Taylor, S. J. (2019). Strengthening the reporting of empirical simulation studies: Introducing the STRESS guidelines. Journal of Simulation, 13(1), 55–67. https://doi.org/10.1080/17477778.2018.1442155
- Montibeller, G., & Franco, L. A. (2011). Raising the bar: Strategic multi-criteria decision analysis. Journal of the Operational Research Society, 62(5), 855–867. https://doi.org/10.1057/jors.2009.178
- Mustafee, N., & Powell, J. H. (2018). From hybrid simulation to hybrid systems modelling. In 2018 Winter Simulation Conference (WSC) (pp. 1430–1439). IEEE. https://doi.org/10.1109/WSC.2018.8632528
- Mustafee, N., Harper, A., & Onggo, B. S. (2020). Hybrid Modelling and Simulation (M&S): Driving Innovation in the Theory and Practice of M&S. In 2020 Winter Simulation Conference (WSC) (pp. 3140–3151). IEEE. https://doi.org/10.1109/WSC48552.2020.9383892
- Mustafee, N., Powell, J. H., & Harper, A. (2018). RH-RT: A data analytics framework for reducing wait time at emergency departments and centres for urgent care. In 2018 Winter Simulation Conference (WSC) (pp. 100–110). IEEE. https://doi.org/10.1109/WSC.2018.8632378
- NHS Digital. (2016). Number of patients registered at a GP practice CCG, April 2016. http://digital.nhs.uk Accessed 18/04/21.
- NHS. (2016). Achieving world-class cancer outcomes: A strategy for England 2015 – 2020 one year on 2015–2016. https://www.england.nhs.uk/wp-content/uploads/2016/10/cancer-one-year-on.pdf Accessed 18/04/21.
- NHS. (2017). Next steps on the NHS Five Year Forward View. https://www.england.nhs.uk/wp-content/uploads/2017/03/NEXT-STEPS-ON-THE-NHS-FIVE-YEAR-FORWARD-VIEW.pdf Accessed 18/04/21.
- NHS. (2019a). Diagnosing cancer earlier and faster. https://www.england.nhs.uk/cancer/early-diagnosis/ Accessed 18/04/21.
- NHS. (2019b). The NHS Long Term Plan. https://www.longtermplan.nhs.uk/publication/nhs-long-term-plan/. Accessed 18/04/21.
- NICE. (2015). Suspected cancer: Recognition and referral. https://www.nice.org.uk/guidance/ng12 Accessed 18/04/21
- O’Brien, F. (2011). Supporting the strategy process: A survey of UK OR/MS practitioners. Journal of the Operational Research Society, 62(5), 900–920. https://doi.org/10.1057/jors.2011.2
- ONS. (2013). Mid-2002 to Mid-2010 Population Estimates for Clinical Commissioning Groups (CCGs) in England by Single Age and Sex Based on the Results of the 2011 Census. Office of National Statistics. http://www.ons.gov.uk.
- ONS. (2014). Clinical Commissioning Group Mid-year Population Estimates. Office of National Statistics. http://www.ons.gov.uk.
- ONS. (2016a). Subnational Population Projections, Clinical Commissioning Groups in England: Z2. Office of National Statistics. http://www.ons.gov.uk.
- ONS. (2016b). National population projections: 2014-based statistical bulletin. Office of National Statistics. http://www.ons.gov.uk.
- Ordu, M., Demir, E., Tofallis, C., & Gunal, M. M. (2021). A novel healthcare resource allocation decision support tool: A forecasting-simulation-optimization approach. Journal of the Operational Research Society, 72(3), 485–500. https://doi.org/10.1080/01605682.2019.1700186
- Porter, A. L., & Detampel, M. J. (1995). Technology opportunities analysis. Technological Forecasting and Social Change, 49(3), 237–255. https://doi.org/10.1016/0040-1625(95)00022-3
- Ravindran, S., Bassett, P., Shaw, T., Dron, M., Broughton, R., Johnston, D., Healey, C. J., Green, J., Ashrafian, H., Darzi, A., Coleman, M., & Thomas-Gibson, S. (2021). National census of UK endoscopy services in 2019. Frontline Gastroenterology, 12(6), 451–460. https://doi.org/10.1136/flgastro-2020-101538
- Robinson, S. (2004). Simulation: The practice of model development and use. Wiley.
- Robinson, S. (2020). Conceptual modelling for simulation: Progress and grand challenges. Journal of Simulation, 14(1), 1–20. https://doi.org/10.1080/17477778.2019.1604466
- Sarasvathy, S. (2001). Toward causation and effectuation: A theoritical shift from inevitability to economic entrepreneurial contingency. Academy of Management Review, 26(2), 243–263. https://doi.org/10.5465/amr.2001.4378020
- Tolk, A., Harper, A., & Mustafee, N. (2021). Hybrid models as transdisciplinary research enablers. European Journal of Operational Research, 291(3), 1075–1090.
- Torres, J. P., Kunc, M., & O’brien, F. (2017). Supporting strategy using system dynamics. European Journal of Operational Research, 260(3), 1081–1094. https://doi.org/10.1016/j.ejor.2017.01.018
- Valori, R. M., & Johnston, D. J. (2016). Leadership and team building in gastrointestinal endoscopy. Best Practice & Research. Clinical Gastroenterology, 30(3), 497–509.
- Van Wyk, R. J. (1997). Strategic technology scanning. Technological Forecasting and Social Change, 55(1), 21–38. https://doi.org/10.1016/S0040-1625(97)83077-6
- Willis, G., Cave, S., & Kunc, M. (2018). Strategic workforce planning in healthcare: A multi-methodology approach. European Journal of Operational Research, 267(1), 250–263. https://doi.org/10.1016/j.ejor.2017.11.008
- Worrall, P., & Chaussalet, T. (2015). A structured review of long-term care demand modelling. Health Care Management Science, 18(2), 173–194. https://doi.org/10.1007/s10729-014-9299-6
- Yu, X., Tao, Y., Tao, X., Xia, F., & Li, Y. (2018). Managing uncertainty in emerging economies: The interaction effects between causation and effectuation on firm performance. Technological Forecasting and Social Change, 135, 121–131. https://doi.org/10.1016/j.techfore.2017.11.017