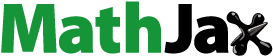
Abstract
Using 1.6 m marriages, 1837–1939, and a genealogy of 428,000 people 1600–2022, we estimate three new occupational status indices for England 1800–1939. The first, CCC-HISCO, re-estimates the HISCAM-GB index, using 30 times as much data. The second, CCC, uses the same association methodology behind HISCAM to assign status but employs richer occupation classifications than in HISCO-GB. The third, CCC2, links this richer set of occupations to measures of education and wealth, using principal component analysis. The close correlation between the CCC and CCC2 indices shows the HISCAM methodology generates occupational status indices, rather than just social proximity measures. All three new indices perform better than existing HISCAM indices, by the metric of father-son status correlation. They all imply less social mobility 1800–1939 than current indices.
1. Introduction
Using a large new database of 1.6 million marriages 1837–1939, and a genealogy of 428,000 people 1600–2021, this paper estimates three new occupational status indices for England in the interval 1800–1939. The first of these, CCC-HISCO is a refinement of the HISCAM-GB index, but constructed using 30 times as much data as the original from marriage records 1837–1939.Footnote1 The second, the CCC index, uses these same 1.6 million marriage records, and the same association method as HISCAM-GB, but with a richer set of occupational categories (462 versus 376). The third index, CCC2, again uses the richer set of occupational categories but combines instead six explicit measures of the social status associated with different occupations: specifically four measures of education and two of wealth.
We believe we have created improved social status indices for England 1800–1939 for the following reasons:
We have about 30 times as much data as was used to estimate the existing HISCAM indices, giving us much more precise estimates of the social status of occupations.Footnote2
We estimate directly a socio-economic status index based on education and wealth by occupation for the years 1800–1939 (CCC2). We use this to validate that the indices based on the association of occupations between fathers and sons, CCC and CCC-HISCO, correspond closely to socio-economic status.
In the CCC index by estimating status also for near 28,000 pairs with such status descriptors as Esquire, Gentleman, Landed Proprietor, Titled, Own Means, and Student we provide an index that better captures status for the upper tail of the status distribution.
Again in the CCC index, by expanding the occupational categories to 460, as opposed to the 376 occupational categories with status estimates in CCC-HISCO, we get a higher father-son correlation of status, which we argue below is the metric by which occupational status indices should be judged.Footnote3
We give online a CSV file with values for the CCC-HISCO index so that those with occupations classified using HISCO will have access to a better occupational status index for England 1800–1939. We do not know if the CCC-HISCO index will provide a better social status measure also for other countries in these years. That is an empirical question that will be determined by looking at the father-son correlations with CCC-HISCO versus other indices.
The creators of the HISCAM indices emphasize that these indices do not measure occupational social status, but instead are purely social interaction distance scales, and are not designed as proxies for the social prestige of occupations, or of income, or wealth.Footnote4 They measured only which occupations interacted in marriage and in families.Footnote5 However, in practice, the HISCAM indices have been widely used by researchers to measure socio-economic status. One recent paper noted, for example, “For Zeeland, we use the highest HISCAM SES (Lambert et al. Citation2013)… to measure socioeconomic status”(van Dijk, Janssens, and Smith Citation2019, 858).Footnote6
Further, a comparison of the three new indices developed here suggests that the association methodology does indeed also capture the social status of occupations. The explicit socio-economic status index, CCC2, is highly correlated with both the new association indices, with a correlation of 0.86 to the CCC index, and 0.82 to the CCC-HISCO index. The close correlation between the association indices and those that measure education and wealth provides a validation of the association methodology as also capturing occupational socio-economic status. The close correlation between the association indices and the socio-economic index just reflects the fact of social life that people associate in marriage and families with those close to them in socio-economic status.Footnote7
These three new indices all show that there was much less social mobility 1800–1939 than the current HISCAM indices imply. We argue that, in general, the measure of the quality of an occupational status index will be how high a correlation in father-son or father-in-law-son status the index produces. The first argument for this test of the quality of an index is that Goodman’s RCII association model which is used to produce the HISCAM and other indices sets occupational status scores to best predict the relative frequency of occupational pairings (Goodman Citation1979). But since the predicted frequency is higher the closer the rank scores of two occupations, this algorithm is also effectively maximizing the intergenerational correlation of occupational rankings. Since this is the criteria for the most predictive index, in comparing the quality of different indices it is consistent to use this as a benchmark for the quality of an index.
A second argument for using the father-son correlation as the test of quality of different indices is that there is evidence from a variety of sources that the true underlying correlation in social status between fathers and sons in England 1600–2022 is 0.75 or higher throughout this period (Clark Citation2023; Clark, Cummins, and Curtis Citation2022). Because any occupational index is imperfect, occupational status correlations will always be estimated as less than the true underlying correlation. A measure, however, of the quality of any index will be how close the estimated father-son correlation comes to the underlying intergenerational correlation.
Another argument for why the quality of an index is measured by the father-son correlation it produces is that if we take any existing index and make it worse by adding random noise to it, then it will lower the father-son correlation. If we estimate an index using just a small subsample of the available data, again we get a result with a lower father-son correlation. Thus the better an index captures true socio-economic status for occupations, the stronger will be the father-son correlation.
We also find good evidence in these new indices that occupational status is a continuum, best measured on a continuous scale as with CAMSIS and HISCAM. The popular alternative approach is to cluster occupations into a small number of discrete class categories, such as skilled manual workers, as was done for the UK by such as Robert Erikson and John Goldthorpe (Erikson and Goldthorpe Citation1992). This tradition was followed more recently by sociologists who deployed a seven-class system to measure social class in the UK in 2011 (Savage et al. Citation2013). However, such lumping of people into discrete social classes produces a poorer description of the movement of class across generations than does continuous measures of social status. This is illustrated in , which shows the average social status of sons 1837–1879 on the CCC scale relative to the average status of fathers. There is a very strong linear relationship, with the same slope all along the status distribution. A straightforward regression to the mean dominates. There are no signs of any unusual persistence for upper or lower classes. The CAMSIS and HISCAM continuous measures of occupational status are a better approach than the alternative discrete class schemas.
2. The HISCAM indices
The standard indices to measure male intergenerational occupational status mobility before 1939 in Britain have been those from the HISCAM project. The HISCAM measures for Britain 1800–1938 were derived using data on pairs of occupations, mainly father-son or father-in-law-son pairings. An algorithm was employed to give rank scores to each occupation in a way that best predicted the observed relative frequency of occupational pairs.
Occupations for the HISCAM status scores were coded to a standardized international occupation classification system HISCO, which set out to have an internationally comparable set of occupation codes based on the 1,300 most common male and female occupations 1800–1938 in Belgium, England, France, Germany, Netherlands, Norway, Quebec, and Sweden.Footnote8 Because of the desire for a comparable international coding of occupations the occupational classifications are detailed. A weaver, for example, can be coded as Cloth Weaver (hand), Cloth Weaver (Machine, except Jacquard Loom), Cloth Weaver (Hand or Machine), Weaver (Specialization Unknown), or Other Weavers and Related Workers.
In HISCAM-GB there are only 376 occupational categories, out of a potential 1,300, with assigned scores. These assignments were based on 51,419 occupational pairings.Footnote9 Relative to the number of occupational categories used, the data is modest, so that for many of the less frequent occupational categories, the assigned status will be measured with significant error.
To rank occupations on a single rank scale CAMSIS and HISCAM used Goodman’s RCII association model (Goodman Citation1979).Footnote10 The resulting estimates for HISCAM are normalized to have mean of 50 and standard deviation of 15, then truncated to have a minimum value of 1 and a maximum of 99.
The alternative general HISCAM-U2 index has a much larger empirical base, 1.3 million occupation pairs, again composed almost equally of father-son and father-in-law-son occupational pairings. The number of pairings underlying the index is, in Belgium, 56,774, Britain, 51,419, France, 55,459, Germany, 12,301, Netherlands, 564,726, Quebec, 552,521, and Sweden, 31,219. Because of the small sample sizes for Germany and Sweden, the developers of HISCAM suggest for these countries, that using the universal scale may be preferable.
The HISCAM and CAMSIS indices have two modifications from the Goodman association model to address several common practical problems in estimating association models. The first is that of sparse categories. The fine grid of occupations, together with the modest numbers of occupational pairings means that many occupations appear infrequently. Where an occupational category has few individuals, the RCII estimator often will not converge to a stable set of occupational status rankings. HISCAM and CAMSIS address this by combining any occupation with fewer than 30 observations with other similar occupations.Footnote11 But this, of course, introduces further error into the index.
The second problem are so-called diagonals and pseudo-diagonals. Diagonals are cases where each person in the pair has the same occupation. Pseudo-diagonals are cases where even though the occupations have different statuses, they are frequently found together in pairings. These would include particularly farmer and farm-worker which are found commonly both in husband-wife pairings and also father-son pairings.Footnote12 To avoid the distortions in status rankings CAMSIS and HISCAM typically drop pairs of diagonals and pseudo-diagonals. The HISCAM project, however, concluded that dropping diagonals was insufficient, and dropped the agricultural sector from their analysis entirely. Farm jobs were assigned scores equal to the average of all occupations paired with farming occupations.
With the new data assembled below, we find that these two problems do not arise, and we can estimate association models without any restrictions. In particular, the small size of the farm sector in England by 1837 means that there are plenty of father-son and father-in-law-son pairings with one party in agriculture and the other outside farming with which to estimate the status of farming occupations. shows for marriages 1837–1879, the numbers of pairings of farm occupations father-son with other farm occupations and with non-farm occupations. Thus, for example, while there are 28,843 father-son pairings where both are farmers, there are 50,511 pairings where one is a farmer and the other in a non-farm occupation.
Another occupation where there was relative isolation from other sectors of the economy was coal mining, where coal villages could have a workforce concentrated in mining. Here we might expect a complete dominance of father-son pairs who were both in coal mining, making it hard to estimate the social status of coal miners. However, while we do find in marriages 1837–1879, 27,050 father-son pairs who were both coal miners, we also find 30,082 pairs where only one in the pair was a coal miner. So even here there is plenty of connection between coal miners and other occupations to allow an estimate of the average social status of coal miners.
3. Three new occupational status indices, England and Wales, 1800–1939
Using two large new databases, in this paper we construct three new occupational status indices for men in England 1800–1939. The first of these indices is a refinement of the HISCAM-GB index for England, which we label CCC-HISCO. Here we estimate for 319 identified HISCO categories a new RCII index using occupational data for 2.36 million father-son and father-son-in-law pairs, from 1.6 million marriages 1837–1939. This new index is thus based on nearly 30 times as much data as the HISCAM-GB index.
This index also uses more father-son pairs than in the entire eight country HISCAM occupational database.Footnote13 We carry out the index estimation separately using the father-son occupation associations, and the father-in-law-son associations, and then take the average of these occupational rankings in forming the overall index.
Because of this much greater set of data, we are able to avoid the improvisations forced on the HISCAM creators by data limitations, such as amalgamating occupations in the estimation. Because of the close interconnections shown in above between farm and non-farm occupations, we can estimate the model without having to drop and then approximate occupations in the agricultural sector. Lastly, we are able to implement the RCII model without dropping diagonal, or quasi-diagonal, observations. However, only 319 out of 1,300 potential HISCO occupations are matched to the occupation labels in our data.
The second of these new indices, CCC, is an association index, as with CAMSIS and HISCAM. We also employ an occupational scheme with a richer set of 462 occupational categories (as opposed to the 376 in HISCAM-GB). These categories were those that showed up most often in the nearly 5 million occupational titles that occur in our marriage database. In Appendix A, we list these occupational titles and the corresponding HISCO occupation numbers. We also included status titles that were not included in HISCO, such as Esquire, Gentleman, Landed Proprietor, Titled, Own Means, Student, and Pauper. In this case, the correlation between the RCII index created using fathers and sons versus the RCII index using fathers-in-law and sons is 0.80.
The third new index, CCC2, is constructed in part using a large genealogical database for England that has information on such outcomes as occupation, wealth at death, and educational status. It also employs data from the marriage records on groom literacy by occupation. This index is a much more direct estimate of average socio-economic status by occupation. Nicely, it is constructed completely independently of the information underlying the CCC index. It serves to validate that the CCC index is indeed capturing occupational status, rather than just social proximity.
Scholars use occupational status indices in part to measure the degree of occupational status inheritance, and also to measure the degree of occupational status assortment in marriage. It is thus potentially problematic to compare status inheritance and marital assortment over time when the indices to measure this are estimated by maximizing both of these correlations. The CCC2 occupational status index has one virtue in being completely independent from parent-child occupation correlations, and also from marital occupation correlations. This index has six components.Footnote14
Literacy rates by occupation, 1837–1879
Probate Rate by occupation, 1858–1939
Average log wealth at death by occupation, 1858–1939
Average attainment of higher education by occupation, 1800–1939
Proportion in schooling ages 12–18 by occupation, 1851–1939
Proportion at work ages 12–18 by occupation, 1851–1939
The literacy rate by occupation is estimated from 0.4 million observations of the signature literacy of grooms 1837–1879 and their occupations. The period 1837–1879 was used even though there is literacy data all the way to 1939 because after 1880 signature literacy rates for grooms are near 100% so that this measure contributes little information for 1880 and later. For marriages 1837–1879 only 64% of grooms could sign the register, so that this measure contributes significant information on educational status by occupation. This measure will discriminate more on the status of lower status occupation since almost all men in higher status occupations will be literate.
The second measure, the probate rate, shows the fraction of men by occupation that had some wealth at death, for deaths 1858 and later. The third measure is the average ln wealth at death, measured relative to average estimated ln wealth at death for each decade in England. For those not probated, wealth at death is taken as half the level of wealth at which probate was legally required in the year of death. These two wealth measures correlate highly. But the first better measures differences in wealth for occupations lower in the wealth distribution, while the second better measures wealth differences for higher status occupations.
The fourth measure is an indicator of what fraction of men by occupation attended university, or achieved an equivalent higher education, such as medical training in a teaching hospital, or membership of an engineering society, or qualification as a chartered accountant. This again is a measure which discriminates more for higher status occupations.
The final two measures are whether the person was observed in schooling, or at work, when recorded in a census or population register 1851–1939 ages 12–18.Footnote15
We construct a composite index of our six occupational status variables using Principal Components Analysis (PCA).Footnote16 PCA, originally created by Pearson (Citation1901) and later developed by Hotelling (Citation1933), is a widely used technique to simplify multidimensional data. PCA generates linear transformations of the six status measures into a set of new variables: uncorrelated principal components. By construction, the first principle component captures the greatest variation possible by any single linear transformation.
We use this first principal component as our unidimensional index of occupational status. The specific formula for the CCC2 index in this case is,
where LITERACY is the average male literacy rate by occupation, DPROB is the fraction of men probated by occupation, LNW is the average ln wealth of men by occupation, DED is the average share achieving higher education, DWORK is the average share at work 12–18, DSCHOOL is the average share in school 12–18. Where one of the six measures was missing we estimated occupational status using the other five in the same fashion, or interpolated the missing values from similar status occupations.
4. Data
We use two sources of data to construct these new indices. The first is a set of 1.6 million marriage records in England 1837–1939 which were transcribed by volunteers to the FreeREG organization, and posted on their web page.Footnote17 The FreeREG marriage records, where the information comes from marriage record copies deposited in local record offices, all come from church weddings, and exclude civil marriages. But though civil marriage was introduced in England in 1837, such marriages remained a small minority of all weddings before 1914. In 1841 civil marriages were 1.7% of all marriages. In 1914, they were still only 24%, and even in 1952, 31% (Haskey Citation2015).
These marriage registers typically record whether the bride and groom were literate (through their ability to sign the marriage register). They also give occupations for the groom, his father, and his father-in-law.Footnote18 The data we have available by period is shown in . Because transcribing these marriage records is a volunteer effort based on local interests, the number of marriages recorded by county for the years 1837–1939 varies considerably by county. Four counties contain about 50% of the marriages transcribed for England: Kent, Lancashire, Lincolnshire, and Staffordshire. But these counties were very different in terms of occupations and urbanization so that the overall sample generated seems representative of England as a whole.
From the resulting database Marriages of England (MOE) we construct our CCC-HISCO and CCC index of male occupational status 1837–1939. We also construct from the literacy data for grooms 1837–1879, a measure of literacy by occupation.
In constructing the CCC index, and in estimating literacy by occupation we convert the more than 100,000 individual occupation description strings in these 1.6 million marriage records into 462 simplified occupations. The more than 2,000 different types of clerks listed, for example, were translated into Bank Clark, Civil Servant-Clerk, Clergy-Church of England, Commercial Clerk, Legal Clerk, and Parish Clerk. We also coded these occupations by their HISCO equivalent, and as noted constructed a new index CCC-HISCO using this occupational scheme.
shows the distribution of occupational status scores on the CCC index, on a 0–100 scale, across the whole population of grooms. The distribution is modestly skewed, with a median status of 42 out of 100. Where multiple occupations were given in the marital records we used the first listed, except in the case that the first was a military occupation. In that case, we coded the person to their civilian occupation.
The second source of data we have is a genealogical database 1600–2022 of 424,000 linked persons in rare surname lineages [Families of England (FOE)] where we can obtain for a subsample of men their wealth at death, their probate status, their educational status ages 12–18, and their attainment of higher educational qualifications.Footnote19 shows the amount of data available for men by occupation from this source.
The schooling 12–18 variable is estimated from a set of census reports on whether a person in this age range was at work, in schooling, or an apprenticeship, or nothing was recorded. To allow for the cases with nothing recorded we take the raw measure of schooling as the average of an indicator variable for in schooling and one minus an indicator variable for at work. However, we correct this variable for the average age people were observed at in each occupation by regressing the fraction in schooling against average age and adjusting all the raw measures to a standard age of 15. This results in some cases in a negative estimate of the proportion of schooling on this adjusted measure. The two wealth measures are the fraction of men whose estates were probated at death by occupation, and the average ln wealth of those probated normalized by average wealth at death for all men by decade 1850–1939.
These six measures of educational and wealth status correlate reasonably well, as shows. Though the quantity of data here is much smaller than for the marriage database, we shall see that it produces an index that is nearly as good in terms of intergenerational correlations as the family association index.
The principal component analysis decomposition works well with the six status indices we employ here. The first principal component accounts for 67% of the variance in the six status measures. We normalize the resulting CCC2 index to a scale of 0–100. also shows the distribution of the status values of the CCC2 index on this 0–100 scale, across the whole population of grooms. The distribution is asymmetrical, with the mass of men having occupations in the 20–50 occupational status range. But there is a long tail of upper status occupations in the 50–100 range. shows the characteristics of the top 10 and bottom 10 occupations in the CCC2 ranking. The top and bottom occupations seem very plausible for those positions.
shows the ranking of the top 10 occupations in the CCC index, and their comparable ranking in the CCC2 index.
Though the CCC and CCC2 indices were produced using entirely different methods, and completely different data, they show a 0.86 correlation in the status assigned to occupations. , for example, shows the estimated status of the 462 occupations in the CCC index versus the CCC2 index. The graph also shows the most significant outliers. There is no obvious pattern to these. This shows that family association style status indices produce occupational status rankings that are very close to those implied using direct socio-economic measures, such as education and wealth. This is confirmation of the validity of the HISCAM approach also as a measure of the socio-economic status of occupations.
The CCC2 index also produces much higher intergenerational correlations in occupational status than the existing indices. Where we estimate, however, familial correlations using the marriages database we potentially run into the problem that the CCC index was constructed using the same data and with an algorithm based on maximizing the father-son correlations in occupational status. However, we can test whether this will be a significant source of bias by taking the marriage data, dividing it randomly into two halves, and then estimating the CCC index on the first part. We can take this 50% index and estimate the father-son and father-in-law-son correlations using both the training 50% of the data and the testing 50%. If these estimates do not differ significantly across the two sub-samples of the marriage data, then we are getting an unbiased estimate of intergenerational mobility even using the marriage sample and the RCII status index derived from that same sample.
shows the results of this test. The evidence from the table is that there is no significant upward bias in intergenerational correlation estimates when we use an RCII status index derived from the same data with which we estimate the intergenerational correlation. Thus on either database, we can do a test of the quality of the different occupational indices.
In Appendix A.1, we give the CCC-HISCO scores, as well as the HISCAM-U2 and HISCAM-GB status scores, for the 319 HISCO occupations we are able to rank. In Appendix A.2, we give the status scores of each of the 462 FOE occupations on both the CCC and CCC2 indices.
5. Comparing the new indices with HISCAM
shows the correlation in occupational status as measured with the three new indices CCC, CCC2, and CCC-HISCO compared to HISCAM-GB and HISCAM-U2. As can be seen, all these indices correlate strongly. Note in particular that the CCC2 index, which is constructed both in a different manner and using completely separate data, correlates well with all the association type indices.
However, the best index of occupational status will be the index which produces the highest correlations of son to father and groom to father-in-law. shows these father-son correlations for all five indices 1837–1939. Though the CCC indices correlate well with the two HISCAM indices, all the CCC indices produce substantially greater father-son and father-in-law-son correlations than does either HISCAM-GB or HISCAM-U2. Thus on this criterion of fit, they are a better index of social status for England 1837–1939. The true correlation in status on the CCC index, for example, averages at 0.68 for these years, well above the 0.54 correlation found with HISCAM-GB.
and show in detail how the individual occupations compare in estimated status between HISCAM-GB and CCC-HISCO, and HISCAM-U2 and CCC-HISCO. The ten occupations with the greatest sum of squared deviations in status are labeled by name. In general, the CCC-HISCO index shows much greater differences in status for occupations at the lower end of the status scale, such as coal miners and laborers, than do the HISCAM indices. Other than that, however, there is no particular pattern to the deviations across the indices.
The CCC2 index performs somewhat less well than the CCC index, as measured by intergenerational correlations. But it must be remembered that five of the six sub-indices that compose this index were created using sample sizes in the order of 10,000–50,000, as opposed to the 2.4 million observations used to construct the CCC and CCC-HISCO indices. If sample sizes for constructing the CCC2 index were substantially increased it might well correlate better across generations than the CCC index.
also shows that on all these indices there appears to be an increase in social mobility rates from 1837 to 1939. For the CCC index, for example, the measured father-son intergenerational correlation falls from 0.71 in 1837–1859 to 0.60 in 1900–1939. On HISCAM-GB the fall is from 0.59 to 0.44.
However, the 0.68 intergenerational correlation recorded using the CCC index can be shown to be still well below the true correlation for 1837–1939. This is because of two forms of remaining error in the index. The first is the mismeasurement of the exact average status of each of the FOE 462 occupation categories. The second is that people whose occupation is assigned to the same of the 462 categories will often actually differ in occupational status. The category “clerk,” for example, covers occupations that differ widely in earnings, and in other measures of occupational status.
Suppose a person’s true occupational status is z. Suppose also their assigned status on an occupational index is Z. Then there will be two independent errors linking their assigned status to their true status. Z = z + u + e, where e is the error in measuring the true average occupational status of the assigned occupation Z. u is the error caused by the range of occupations that fall under the label Z, each with a different underlying status.
When we measure intergenerational mobility with such a social status index the estimate is biased downwards by a factor:
(1)
(1)
For the CCC and CCC2 indices, because of their entirely independent construction, the error component e attached to errors in the average occupational status by category will be independent, but not the within-category component u. Assuming the error term e variance is the same for each index, the correlation between these indices 0.86 will be
(2)
(2)
This implies that the error component in these indices we have derived has to be at least 12% of the variance in measured status. It also implies that if we multiply our father-son correlations by 1.16 we will get an estimate closer, but still not as large as, the true underlying persistence of occupational status across generations. Since that correlation for the CCC index is 0.68, the true intergenerational correlation in occupational status has to be at least 0.78. When we add the attenuation caused by the variance within occupational categories, the true underlying correlation of occupational status in England 1837–1939 must be above 0.8. This is well above the 0.51–0.53 correlation reported for this period using the HISCAM-U2 and HISCAM-GB occupational status indices.
As noted above, the parish register data we relied upon to construct the CCC and CCC-HISCO indices was over-sampled in four counties—Kent, Lancashire, Lincolnshire, and Staffordshire—which together accounted for almost half the observations. shows the estimated intergenerational correlation from each of these counties, as well as from the rest of the data. Are there significant geographic differences in intergenerational social mobility that might explain the much stronger intergenerational correlations found with the CCC and CCC-HISCO indices than for the HISCAM indices? As can be seen in , for three of the four counties there is no substantial difference in the intergenerational occupational status correlation and that for the rest of the country. Only for one county, Lancashire, do we observe a substantially different correlation compared with the rest of the country, and that is lower at 0.56 versus 0.68. If we were to reweight the data to be nationally representative, if anything we would likely observe the same or an even higher intergenerational occupational status correlation. Thus the particular geographic concentration of the marriage records does not in any way explain the very high intergenerational correlations we observe.
6. Conclusion
Using large quantities of new data, we construct three new independent occupational status indices for England in the years 1800–1939, the CCC, CCC2, and CCC-HISCO indices. These new indices all provide more accurate measures of the social status of occupations in these years than the existing HISCAM indices (HISCAM-GB and HISCAM-U2). Appendix A gives the estimated status for all occupations on these new indices.
Second, we validate that association indices of occupational status do successfully capture the socioeconomic rank of different occupations as measured by the educational and wealth status of the holders. The two new association indices, CCC and CCC-HISCO are both highly correlated with the third new index, CCC2, which was entirely constructed from six measures purely of socioeconomic status. Though some scholars continue to emphasize the difference between social interaction distance scales and social status scales, we show above that effectively such scales measure the same thing, the social status of occupations. Association indices thus are very good measures not just of social networks, but also of socio-economic status. An important contribution of this paper is thus to show that association indices do not capture a distinct feature of social life. Social interactions seem to be dominated by social status.
We also find that with sufficiently large sets of data, these association indices can be estimated for England without having to make the various adjustments and status assignments found in the HISCAM indices. Thus this paper is a strong validation of the strength of the association approach to constructing status indices and of the general validity of the indices so constructed.
Third, we show how dependent measures of intergenerational occupational status mobility are on the quality of occupational indices. The more accurate the status index the lower are measured rates of intergenerational mobility. While this shows that social mobility rates in England 1800–1939 were low, it also shows that all comparisons of intergenerational occupational mobility over time and place using such indices are suspect. The measurement errors embedded in occupational status indices depend on the quantity of data available to construct the index, the employment structure in the society in question, and the way occupations are described in different societies. Traditional comparisons of social mobility across time and place using such indices are therefore unreliable. We explore this issue in more depth in a related working paper and suggest a method of extracting the true underlying intergenerational correlations in status (Clark, Cummins, and Curtis Citation2022).
However, there are many purposes for which these imperfect indices are still highly useful. One example is that the popular conception that women tended to marry-up socially is not true for England 1837–1939. We can measure the social status of both bride and groom using the occupational status of their fathers. When we do this the status of the groom’s father, on average, equaled the status of the bride’s father throughout these years. Women were not marrying men whose family background, on average, showed higher social status (Clark and Cummins Citation2023).
The CCC-HISCO index is a higher quality index for England than HISCAM-GB or HISCAM-U2. It is likely that this index will also be of better quality than the HISCAM indices for Germany, Sweden, Belgium, and France given the much larger size of the dataset it is based upon. Potential users will be able to determine if it works better by comparing the resulting father-son or father-in-law-son-in-law correlations. For the convenience of users, we have provided a link to the CCC-HISCO scores.Footnote20
Disclosure statement
No potential conflict of interest was reported by the author(s)
Additional information
Funding
Notes
1 For details on the construction of the HISCAM-GB and HISCAM-U2 association indices see Lambert et al. (Citation2013).
2 The source of the HISCAM-GB index, as without association indices, is a much smaller set of marital records 1837–1938 for England (Lambert et al. Citation2013).
3 A CSV file with the three new indices and the existing HISCO_GB and HISCO_U2 indices is available for download on the Harvard Dataverse https://doi.org/10.7910/DVN/0AZTNV.
4 https://www.camsis.stir.ac.uk/Data/Britain91.html. See Lambert et al. (Citation2013) and Prandy and Lambert (Citation2003).
5 http://www.camsis.stir.ac.uk/hiscam/. HISCAM is an empirical estimate of the average relative position within the structure of social stratification occupied by the incumbents of occupational unit groups based on patterns of intergenerational occupational connections.
6 See also, as examples using HISCAM scores as measures of social status, Bailey, Hatton, and Inwood (Citation2016), Brea-Martínez and Pujadas-Mora (Citation2018), Connor (Citation2017, Citation2019), Cummins (Citation2020), Debiasi and Dribe (Citation2020), Dribe and Helgertz (Citation2016), Dribe and Karlsson (Citation2022), Dribe and Quaranta (Citation2020), Fernihough (Citation2017), Hällsten and Kolk (Citation2023), Jaadla et al. (Citation2020), Knigge (Citation2016), Knigge et al. (Citation2014), Knigge, Van Leeuwen, and Maas (Citation2014), Lan and Longley (Citation2021), Rosenbaum-Feldbrügge (Citation2019), Van Leeuwen and Maas (Citation2023), and Zhu (Citation2022).
7 One referee was insistent, nonetheless, that “the sociological term ‘status’, when used in the context of measures of social stratification, is a different concept to that of ‘socio-economic status’.” We do not deny that there are other interpretations of status distinct from socio-economic status. All we claim here is that association status measures are highly correlated with socio-economic status. Thus those interested in socio-economic status can employ association index methods to construct measures of socio-economic status.
8 HISCO, or Historical ISCO, is a modification of the 1968 version of the International Standard Classification of Occupations (ISCO-68) (Van Leeuwen, Maas, and Miles Citation2004). http://historyofwork.iisg.nl/index.php.
9 Lambert et al. (Citation2013), .
10 Hendrickx (Citation2004) and Xie (Citation1992, Citation2003) provide less theoretical introductions to the RCII model.
11 In the HISCAM indices estimated using only data from one country, when a category was small and its score varied substantially from the category’s score in the universal scale, its score was replaced by the average of the original score and the score in the universal scale.
12 In the intergenerational mobility literature, farmers are often a problem regardless of methodology. See, for example, Feigenbaum (Citation2018), Xie and Killewald (Citation2013), and Appendix IV of Abramitzky, Platt Boustan, and Eriksson (Citation2012).
13 The HISCAM database has 1.2 m father-son occupational pairs, but 0.5 m of these come from Quebec, where the occupations are mostly in agriculture.
14 Using information from six components gives our measure an advantage over occupational status scores that rely just on income. Occupational income scores often overlook a lot of within-occupation variation; see, for example, Espín-Sánchez et al. (Citation2019), Inwood, Minns, and Summer Eld (Citation2019), and Saavedra and Twinam (Citation2020). By adding the additional six series, we will capture a lot more variation within-occupation.
15 The censuses of 1851–1921 give such information, as does the population register of 1939.
16 Simple averaging would be inefficient as information would be lost by combining high variability measures, such as average wealth, with those with low variability such as education or literacy. PCA allows the data to tell us the weights that maximize variability, without reference to any target, or output, measure. In this way, PCA is a type of “unsupervised learning.”
17 We added to these records 21,339 marriages in Essex parishes 1837–1939 that we ourselves collected. Much less often in earlier years they give an occupation also for the bride.
18 See note 15 above.
19 This dataset is described in detail in Clark (Citation2023) Supplementary Materials.
20 http://neilcummins.com/CCC-HISCO.csv.
References
- Abramitzky, R., L. Platt Boustan, and K. Eriksson. 2012. Europe’s tired, poor, huddled masses: Self-selection and economic outcomes in the age of mass migration. The American Economic Review 102 (5):1832–56. doi: 10.1257/aer.102.5.1832.
- Bailey, R. E., T. J. Hatton, and K. Inwood. 2016. Health, height, and the household at the turn of the twentieth century. The Economic History Review 69 (1):35–53. doi: 10.1111/ehr.12099.
- Brea-Martínez, G., and J.-M. Pujadas-Mora. 2018. Estimating long-term socioeconomic inequality in southern Europe: The Barcelona area, 1481–1880. European Review of Economic History 23 (4):397–420. doi: 10.1093/ereh/hey017.
- Clark, G. 2023. The inheritance of social status: England, 1600 to 2022. Proceedings of the National Academy of Sciences of the United States of America 120 (27):e2300926120. doi: 10.1073/pnas.2300926120.
- Clark, G., and N. Cummins. 2023. Hypergamy revisited: Marriage in England, 1837–2021. Discussion Papers on Economics, University of Southern Denmark, Department of Economics, Odense.
- Clark, G., N. Cummins, and M. Curtis. 2022. The mismeasure of man: Why intergenerational occupational mobility is much lower than conventionally measured, England, 1800–2021. Technical Report DP17346, Centre for Economic Policy Research, London.
- Connor, D. S. 2017. Poverty, religious differences, and child mortality in the early twentieth century: The case of Dublin. Annals of the American Association of Geographers 107 (3):625–46. doi: 10.1080/24694452.2016.1261682.
- Connor, D. S. 2019. The cream of the crop? Geography, networks, and Irish migrant selection in the age of mass migration. The Journal of Economic History 79 (1):139–75. doi: 10.1017/S0022050718000682.
- Cummins, N. 2020. The micro-evidence for the Malthusian system. France, 1670–1840. European Economic Review 129 (C):103544. doi: 10.1016/j.euroecorev.2020.103544.
- Debiasi, E., and M. Dribe. 2020. SES inequalities in cause-specific adult mortality: A study of the long-term trends using longitudinal individual data for Sweden (1813–2014). European Journal of Epidemiology 35 (11):1043–56. doi: 10.1007/s10654-020-00685-6.
- Dribe, M., and J. Helgertz. 2016. The lasting impact of grandfathers: Class, occupational status, and earnings over three generations in Sweden, 1815–2011. The Journal of Economic History 76 (4):969–1000. doi: 10.1017/S0022050716000991.
- Dribe, M., and L. Quaranta. 2020. The Scanian Economic-Demographic Database (SEDD). Historical Life Course Studies 9:158–72. doi: 10.51964/hlcs9302.
- Dribe, M., and O. Karlsson. 2022. Inequality in early life: Social class differences in childhood mortality in southern Sweden, 1815–1967. The Economic History Review 75 (2):475–502. doi: 10.1111/ehr.13089.
- Erikson, R., and J. H. Goldthorpe. 1992. The constant flux: A study of class mobility in industrial societies. Oxford: Clarendon Press.
- Espín-Sánchez, J.-A., S. Gil-Guirado, W. D. Giraldo-Paez, and C. Vickers. 2019. Labor income inequality in pre-industrial Mediterranean Spain: The city of Murcia in the 18th century. Explorations in Economic History 73:101274. doi: 10.1016/j.eeh.2019.05.002.
- Feigenbaum, J. J. 2018. Multiple measures of historical intergenerational mobility: Iowa 1915 to 1940. The Economic Journal 128 (612):F446–81. doi: 10.1111/ecoj.12525.
- Fernihough, A. 2017. Human capital and the quantity-quality trade-off during the demographic transition. Journal of Economic Growth 22 (1):35–65. doi: 10.1007/s10887-016-9138-3.
- Goodman, L. 1979. Simple models for the analysis of association in cross-classifications having ordered categories. Journal of the American Statistical Association 74 (367):537–52. doi: 10.2307/2286971.
- Hällsten, M., and M. Kolk. 2023. The shadow of peasant past: Seven generations of inequality persistence in Northern Sweden. American Journal of Sociology 128 (6):1716–60. doi: 10.1086/724835.
- Haskey, J. 2015. Marriage rites: Trends in marriages by manner of solemnisation and denomination in England and Wales, 1841–2012. In Marriage rites and rights, ed. R. Probert. London: Hart Publishing.
- Hendrickx, J. 2004. RC2: Stata module to estimate Goodman’s row and columns model 2, statistical software components. Boston, USA: Boston College, Department of Economics.
- Hotelling, H. 1933. Analysis of a complex of statistical variables into principal components. Journal of Educational Psychology 24 (6):417–41, 498–520. doi: 10.1037/h0070888.
- Inwood, K., C. Minns, and F. Summer Eld. 2019. Occupational income scores and immigrant assimilation. Evidence from the Canadian census. Explorations in Economic History 72:114–22. doi: 10.1016/j.eeh.2019.02.001.
- Jaadla, H., E. Potter, S. Keibek, and R. Davenport. 2020. Infant and child mortality by socio-economic status in early nineteenth-century England. Economic History Review 73 (4):991–1022.
- Knigge, A. 2016. Beyond the parental generation: The influence of grandfathers and greatgrandfathers on status attainment. Demography 53 (4):1219–44. doi: 10.1007/s13524-016-0486-6.
- Knigge, A., M. H. D. Van Leeuwen, and I. Maas. 2014. Sources of sibling (dis) similarity: Total family impact on status variation in the Netherlands in the nineteenth century. American Journal of Sociology 120 (3):908–48. doi: 10.1086/679104.
- Knigge, A., M. H. D. Van Leeuwen, I. Maas, and K. Mandemakers. 2014. Status attainment of siblings during modernization. American Sociological Review 79 (3):549–74. doi: 10.1177/0003122414529586.
- Lambert, P. S., R. L. Zijdeman, M. H. D. Van Leeuwen, I. Maas, and K. Prandy. 2013. The construction of HISCAM: A stratification scale based on social interactions for historical comparative research. Historical Methods: A Journal of Quantitative and Interdisciplinary History 46 (2):77–89. doi: 10.1080/01615440.2012.715569.
- Lan, T., and P. A. Longley. 2021. Urban morphology and residential differentiation across Great Britain, 1881–1901. Annals of the American Association of Geographers 111 (6):1–20. doi: 10.1080/24694452.2020.1859982.
- Pearson, K. 1901. On lines and planes of closest t to systems of points in space. Philosophical Magazine 2 (11):559–72. doi: 10.1080/14786440109462720.
- Prandy, K., and P. Lambert. 2003. Marriage, social distance and the social space: An alternative derivation and validation of the Cambridge scale. Sociology 37 (3):397–411. doi: 10.1177/00380385030373001.
- Rosenbaum-Feldbrügge, M. 2019. The impact of parental death in childhood on sons’ and daughters’ status attainment in young adulthood in the Netherlands, 1850–1952. Demography 56 (5):1827–54. doi: 10.1007/s13524-019-00808-z.
- Saavedra, M., and T. Twinam. 2020. A machine learning approach to improving occupational income scores. Explorations in Economic History 75:101304. doi: 10.1016/j.eeh.2019.101304.
- Savage, M., F. Devine, N. Cunningham, M. Taylor, Y. Li, J. Hjellbrekke, B. Le Roux, S. Friedman, and A. Miles. 2013. A new model of social class? Findings from the BBC’s Great British Class Survey experiment. Sociology 47 (2):219–50. doi: 10.1177/0038038513481128.
- van Dijk, I. K., A. Janssens, and K. R. Smith. 2019. The long harm of childhood: Childhood exposure to mortality and subsequent risk of adult mortality in Utah and The Netherlands. European Journal of Population 35 (5):851–71. doi: 10.1007/s10680-018-9505-1.
- Van Leeuwen, M. H. D., and I. Maas. 2023. A historical community approach to social homogamy in the past. The History of the Family 24 (1):1–14. doi: 10.1080/1081602X.2019.1570532.
- Van Leeuwen, M. H. D., I. Maas, and A. Miles. 2004. Creating a historical international standard classification of occupations: An exercise in multinational interdisciplinary cooperation. Historical Methods: A Journal of Quantitative and Interdisciplinary History 37 (4):186–97. doi: 10.3200/HMTS.37.4.186-197.
- Xie, Y. 1992. The log-multiplicative layer effect model for comparing mobility tables. American Sociological Review 57 (3):380–95. doi: 10.2307/2096242.
- Xie, Y. 2003. Association models. In Encyclopedia of social science research methods, ed. Michael S. Lewis-Beck, A. Bryman, and T. Futing Liao, 29–33. California, USA.: Sage.
- Xie, Y., and A. Killewald. 2013. Intergenerational occupational mobility in Great Britain and the United States since 1850: Comment. American Economic Review 103 (5):2003–20. doi: 10.1257/aer.103.5.2003.
- Zhu, Z. 2022. Like father like son? Intergenerational immobility in England, 1851–1911. Economic History Working Papers 117588, London School of Economics and Political Science, Department of Economic History, London.
Appendix A
A.1. Tabular summary of the CCC-HISCO index
shows CCC-HISCO occupational status values in comparison with those of the HISCAM-U2 and HISCAM-GB index. The occupations are listed in order of the HISCO codes. A brief description of each occupation is given. This table is available online at http://neilcummins.com/CCC-HISCO.csv.
Table A1. CCC-HISCO, HISCAM-U2, and HISCAM-GB indices by HISCO.
A.2. Tabular summary of the CCC and CCC2 indices
shows the six components of the CCC2 index for 462 FOE occupational categories, as well as the CCC and CCC2 indices. The occupations are listed in alphabetical order. For 40 of the 462 occupations one or more of the components of the CCC2 index is missing. In these cases, where possible, the CCC2 index values were interpolated based on the other components.
Figure 2. The distribution of occupational status, CCC and CCC2 scores. Note: Each occupation is weighted equally, not by number of observations.
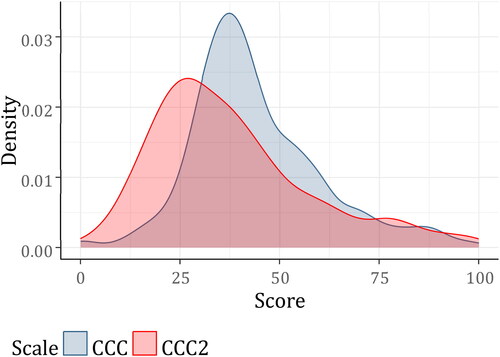
Figure 3. Comparison of CCC and CCC2 scores. Note: The 10 occupations with the highest sum of square deviation between the scores are highlighted.
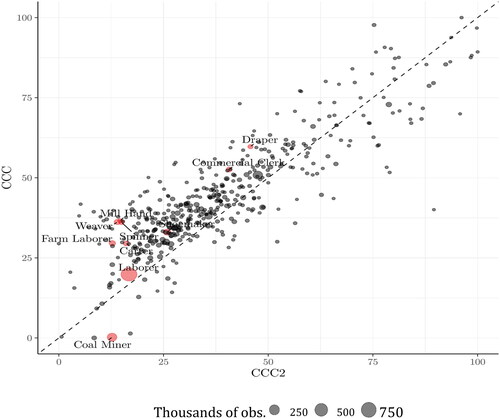
Figure 4. Comparison of HISCAM-GB and CCC-HISCO scores. Note: The ten occupations with the highest sum of square deviation between the scores are highlighted.
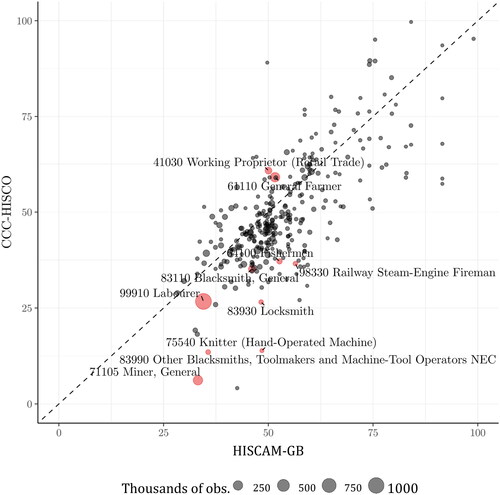
Figure 5. Comparison of HISCAM-U2 and CCC-HISCO scores. Note: The ten occupations with the highest sum of square deviation between the scores are highlighted.
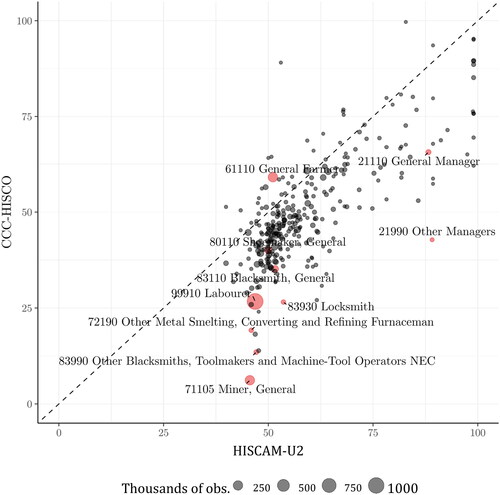
Table A2. The CCC and CCC2 indices and components.
Table 1. Father-son occupational pairings, 1837–1879.
Table 2. Parish register marriage data, 1837–2021.
Table 3. FOE social status data, males.
Table 4. Correlations between the components of the CCC2 index.
Table 5. Top and bottom 10 occupations by CCC2 score.
Table 6. Top and bottom 10 ranked occupations by CCC score.
Table 7. Estimating potential biases in the CCC index, marriage sample, 1837–1939.
Table 8. Correlation between occupational status indices, 1800–1939.
Table 9. Intergenerational correlations in marriage database, 1837–1939, males.
Table 10. Father-son correlation of occupation rank (CCC), by location, 1837–1939.