ABSTRACT
Objective: The purpose of our study was to distinguish the changes in the microstructure of the cingulate cortex in patients with mild cognitive impairment (MCI) induced by cerebral small vessel disease (CSVD).
Method: 80 patients were diagnosed with CSVD in this study, including 55 patients with MCI and 25 patients without MCI. Diffusion kurtosis imaging (DKI) and Montreal cognitive assessment (MoCA) were performed in all patients. The anterior cingulate gyrus, posterior cingulate gyrus and middle cingulate gyrus were selected as the regions of interest, and some parameters were recorded.
Results: Compared with the non-MCI group, the MCI group mainly showed obviously higher mean diffusion (MD) and radial diffusion (RD) values (P = 0.022 and P = 0.029) but lower fractional anisotropy (FA), axial kurtosis (AK), mean kurtosis (MK) and radial kurtosis (RK) values (P = 0.047, P = 0.001, P < 0.01, and P = 0.001, respectively) in the right anterior cingulate gyrus. Meanwhile, in the right posterior cingulate gyrus, the MCI group also showed higher axial diffusion (AD) and MD (P = 0.027 and P = 0.030) and lower AK (P = 0.014). Additionally, negative correlations of AD, MD, and RD with MoCA scores and positive correlations of FA, AK, MK and RK with MoCA scores were observed in some regions of the cingulate gyrus.
Conclusions: DKI is a good method to examine microstructural damage in the cingulate cortex, and some parameters of DKI may be used as imaging biomarkers to detect early MCI in patients with CSVD.
1. Introduction
Mild cognitive impairment (MCI) is defined as a greater than expected cognitive decline for the age and education level with only a mild impact on daily life [Citation1]. The incidence rate of MCI is increasing annually, and 11–33% of subjects with MCI develop dementia within 2 years [Citation2–4]. MCI must be diagnosed and treated in a timely manner in the clinic due to its latent symptoms.
To the best of our knowledge, the cingulate gyrus is an important part of the limbic system that is involved in the regulation of cognitive function. Sambuchi et al. observed gray matter atrophy in regions of the cingulate cortex or other areas of the cerebral hemispheres in the pre-MCI stage using imaging, neurobiology and other methods [Citation5]. The cingulate cortex appears to play a crucial role in the default mode network, the dorsal attention network and the salience network. Previous studies also showed abnormal functional connectivity of the cingulate cortex in patients with MCI [Citation6,Citation7]. These results suggested that changes in the microstructure of the cingulate gyrus may lead to cognitive impairment by altering nerve fiber connections. Therefore, microstructural changes in the cingulate gyrus may play an important role in the development of MCI. However, research on the changes in the microstructure of the cingulate cortex in patients with MCI induced by cerebral small vessel disease (CSVD) is still lacking.
In previous studies, microstructural changes in patients with MCI have been observed using diffusion tensor imaging (DTI). Two of the most widely reported DTI metrics, fractional anisotropy (FA) and mean diffusion (MD), are altered in multiple white matter tracts of patients with MCI [Citation8,Citation9]. Diffusion kurtosis imaging (DKI) extends conventional DTI measures by detecting microstructural changes not only in white matter (WM, anisotropic tissues) but also in gray matter (GM, isotropic tissues) because it is independent of the spatial direction of the structures [Citation10]. DKI quantifies changes in brain tissue by describing the amount of diffusion of water molecules in tissues that deviates from the normal distribution, and kurtosis information reflects the non-Gaussian characteristics caused by the complex structure of multiple microcell compartments, especially in the GM region [Citation11,Citation12]. Due to the lack of reliable and sensitive biomarkers to identify early pathological changes, we first used DKI to explore the changes in the cingulate gyrus of patients with MCI induced by CSVD, and imaging data for microstructural changes in the cingulate cortex are likely to provide sensitive biomarkers for identifying subjects with MCI.
2. Methods
2.1. Participants
We retrospectively collected data from 80 patients with CSVD treated at the Department of Neurology, Beijing Chaoyang Hospital, Capital Medical University between July 2018 and March 2019. The inclusion criterion was a cranial MRI that confirmed the presence of CSVD [Citation13]. The exclusion criteria were as follows: (1) patients who were unable to care for themselves due to serious mental and physical diseases, (2) patients with severe chronic diseases and neurological diseases, such as tumors, heart diseases, liver diseases, renal diseases and motor neuron diseases, and (3) patients who could not undergo MRI scanning or MoCA. Then, all enrolled patients were assessed using a neuropsychological scale. The CSVD-MCI diagnostic criteria were as follows: (1) patients who had cognitive dysfunction that did not reach dementia, and an evaluation of overall cognitive function was performed using the Montreal cognitive assessment (MoCA),and (2) the determination that CSVD is the dominant, if not exclusive, pathology that accounts for cognitive deficits [Citation14,Citation15]. Eventually, 25 patients were included in the non-MCI group, and 55 patients were included in the MCI group. All individual participants included in the study provided informed consent. Approval for the study was obtained from the Research Ethics Committee of Beijing Chaoyang Hospital affiliated with Capital Medical University.
2.2. Assessment of cognitive function
All patients were assessed for cognitive function with scales at the time of the initial hospital consultation. We evaluated cognitive function using the MoCA, which included visuospatial/executive function, naming, memory, attention, language, abstraction, delayed recall and orientation. After the assessment, the scores were summed to yield a total score of 30 points. If the duration of education was less than 12 years, 1 point was added, and less than 26 points was regarded as a cognitive impairment [Citation16]. The assessment was performed by an experienced neurologist.
2.3. MRI scanning
All patients were scanned using a 3.0 Tesla MRI (MAGNETOM Skyra, Siemens Healthcare, Erlangen, Germany) with a 20 channel phased array head coil. The parameters of T1WI were as follows: repetition time (TR) = 2300 ms, inversion time = 900 ms, echo time (TE) = 89 ms, inversion angle = 8°, FOV = 240 mm × 240 mm, voxel = 0.9 mm, parallel acceleration factor (PAT) = 2, acquisition time = 5 minutes and 21 seconds. The first acquisition template parameters were TR = 7700 ms, imaging matrix = 74 × 74, TE = 89 ms, FOV = 222 mm × 222 mm, layer number = 50, layer thickness = 3 mm, b = 0.1000 and 2000 s/mm2, average once, 30 gradient directions, PAT = 2, acquisition time = 8 minutes and 14 seconds. The parameters of the second acquisition module and the first acquisition module were approximately the same, except that b = 0 s/mm2, and the average acquisition time was 1 minute and 34 seconds. The total scanning time of diffusion imaging was 9 minutes and 48 seconds.
2.4. DKI data analysis
All the collected DWI data were transformed into NIFTI format images using the dcm2nii tool and then input into the diffusion kurtosis estimator to generate a DKI parameter map. According to the Anatomical Automatic Labeling (AAL) template [Citation17], the anterior cingulate gyrus, posterior cingulate gyrus and middle cingulate gyrus were selected as the regions of interest (ROIs). The options for reducing eddy current sensitivity and dynamic field correction provided by the supplier in the agreement were applied to reduce the effect of the eddy current during the acquisition process. The T1WI sequence collected by 3D magnetization-prepared rapid acquisition gradient echo (MPRAGE) was input into SPM8 software, and the AAI template was used for automatic segmentation. Then, diffusion-weighted images (DWI) (b = 0 s/mm2) were strictly aligned to the T1WI space. The transformed matrix was applied to the DKI parameter map ().
Figure 1. MRI images with ROIs marked. A: anterior cingulate gyrus, B: posterior cingulate gyrus, C: middle cingulate gyrus
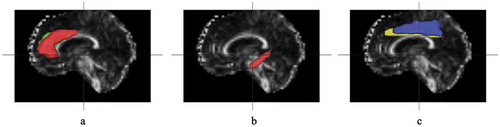
Multiple factors that potentially affect DKI measures were minimized during scanning, including fiber arrangement, axon density, partial volume with surrounding tissue, tissue density, subject motion and image resolution. Seven parameters (AD: axial diffusion, MD: mean diffusion, RD: radial diffusion, FA: fractional anisotropy, AK: axial kurtosis, MK: mean kurtosis, and RK: radial kurtosis) were obtained using DKI. Different parameters have different meanings, and MD reflects the diffusion level and diffusion resistance. FA is based on the diffusion estimation along the axonal fiber axis and reflects the arrangement of the neuronal structure and the integrity of fibers. AK represents the complexity or heterogeneity along the maximum diffusion direction. RK represents the complexity or heterogeneity perpendicular to the maximum diffusion direction, and MK represents the average complexity or heterogeneity of brain tissue [Citation18,Citation19].
2.5. Statistical analyses
The one-sample Kolmogorov–Smirnov test was used to test the normality of the data distribution. Subjects’ ages and MoCA scores met the normality assumptions, were reported as the means ± standard deviations (X ± SD) and analyzed using the independent sample t-test. The duration of education was reported as medians and interquartile ranges and analyzed using the Mann–Whitney U-test. Subjects’ sex and histories of smoking and drinking were examined using the χ2 test. Based on the results of the normality test, DKI data were examined using the independent sample t-test or Mann–Whitney U-test, we corrected the data for multiple comparisons using the Šídák Bonferroni method, and the corrected P-value was statistically significant when P < 0.008 (0.05/6 = 0.008). According to the results of the normality test, correlations with DKI parameters and MoCA scores were examined by calculating Pearson’s or Spearman’s rank correlation coefficients. All statistical tests were two sided, conducted at a significance level of 0.05, and reported as the P value. All analyses were performed using SPSS version 21 (SAS Institute, Cary, NC, USA).
3. Results
3.1. General characteristics of all patients
Of the 80 patients, 46 were male and 34 were female. The average age of the MCI group was 65 ± 8 years, and that of the non-MCI group was 62 ± 9 years. Patients with MCI had shorter duration of education (P = 0.003) and lower MoCA scores (P < 0.01). No differences in age and sex among two groups ().
Table 1. General characteristics of MCI and non-MCI patients
3.2. Comparison of microstructural changes in the cingulate gyrus between the two groups
Compared with the right anterior cingulate gyrus of the non-MCI group, the MCI group had higher MD and RD values (P = 0.022 and P = 0.029) and lower FA, AK, MK, and RK values (P = 0.047, P = 0.001, P < 0.01, and P = 0.001, respectively) (). In the right posterior cingulate gyrus, the MCI group had higher AD and MD values (P = 0.027 and P = 0.030), but lower AK (P = 0.014) (). Parameters with P values less than 0.008 in the right anterior cingulate gyrus included AK, MK and RK (P = 0.001, P < 0.01, and P = 0.001, respectively). No significant differences were observed in the middle cingulate gyrus (P > 0.05) ().
Table 2. Comparison of microstructural changes in the anterior cingulate gyrus between the two groups
Table 3. Comparison of microstructural changes in the posterior cingulate gyrus between the two groups
Table 4. Comparison of microstructural changes in the middle cingulate gyrus between the two groups
3.3. Differences in the microstructure of the left and right cingulate gyrus in the MCI group
The MCI group exhibited higher AD, MD, and RD (P = 0.016, P = 0.005, and P = 0.003) values and lower FA, MK, and RK (P < 0.01, P = 0.041, and P = 0.028) values in the left anterior cingulate than in the right anterior cingulate. The left posterior cingulate presented lower AD, MD, RD, and FA values (P < 0.01, P = 0.005, P = 0.048, and P = 0.006, respectively) and a higher AK (P < 0.01) than the right posterior cingulate. The left middle cingulate exhibited lower FA and MK values (P = 0.007 and P = 0.026) ().
Table 5. Differences in the microstructure of the left and right cingulate gyrus in the MCI group
3.4. Differences in microstructures of the left and right cingulate gyrus in the non-MCI group
The non-MCI group presented higher AD, MD, and RD values (P < 0.01) and lower FA, AK, MK, and RK values (P < 0.01, P = 0.001, P < 0.01, and P < 0.01, respectively) in the left anterior cingulate than in the right anterior cingulate. The left posterior cingulate exhibited lower FA (P = 0.006) than the right posterior cingulate. The left middle cingulate displayed higher AD, MD, and RD (P = 0.014, P = 0.020, and P = 0.033) and lower FA, MK, and RK (P = 0.008, P = 0.007, and P = 0.002) ().
Table 6. Differences in microstructures of the left and right cingulate gyrus in the non-MCI group
3.5. Correlation of microstructural changes in the cingulate gyrus with MoCA scores
The correlations among the MD and RD of the left posterior cingulate gyrus, AD of the left middle cingulate gyrus and MD of the right middle cingulate gyrus were analyzed by calculating Spearman’s rank correlation coefficients, and correlations among other DKI parameters were analyzed by calculating Pearson’s rank correlation coefficients. A positive correlation was observed between AK in the left anterior cingulate gyrus and MoCA scores (r = 0.261, P = 0.021). Meanwhile, negative correlations between AD, MD, and RD (r = −0.252, r = −0.270, and r = −0.267; P = 0.026, P = 0.017, and P = 0.018, respectively) and positive correlations between AK, MK, and RK (r = 0.352, r = 0.337, and r = 0.310; P = 0.002, P = 0.003, and P = 0.006, respectively) in the right anterior cingulate gyrus with MoCA scores were observed ( and ). In the left posterior cingulate, AD, MD, RD and FA (r = −0.276, r = −0.324, r = −0.224, and r = −0.236; P = 0.014, P = 0.004, P = 0.048, and P = 0.038, respectively) showed a negative correlation and AK (r = 0.245, P = 0.030) displayed a positive correlation with MoCA scores. In the right posterior cingulate, AD, MD, and RD (r = −0.357, r = −0.349, and r = −0.301; P = 0.001, P = 0.002, and P = 0.007, respectively) were negatively correlated, and AK and MK (r = 0.379 and r = 0.271; P = 0.001 and P = 0.016, respectively) were positively correlated with MoCA scores ( and ). Negative correlations were observed between AD, MD, RD (r = −0.276, r = −0.342, and r = −0.278; P = 0.014, P = 0.002, and P = 0.014, respectively) and MoCA scores, and positive correlations between AK, MK, and RK (r = 0.256, r = 0.273, and r = 0.235; P = 0.024, P = 0.015, and P = 0.038, respectively) in the right middle cingulate gyrus with MoCA scores were observed ().
Table 7. Correlation of microstructural changes in the cingulate gyrus with MoCA scores
4. Discussion
Patients with CSVD presenting with MCI are at higher risk of developing dementia than age-matched controls. The diagnosis and prevention of MCI in a timely manner in patients with CSVD during clinical treatment are important due to its latent symptoms. DKI might be an effective examination to discover MCI in patients with CSVD and enable preventive measures to be implemented earlier.
Increases in AD, MD and RD and decreases in FA, AK, MK and RK are generally believed to indicate changes in the microstructure. The MCI group presented higher MD and RD and lower FA and AK in the right anterior cingulate gyrus. This group also exhibited higher AD and MD and lower AK in the right posterior cingulate gyrus. Therefore, significant microstructural changes were observed in the right anterior and posterior cingulate of patients with MCI. In addition, increased values for MD, AD, and RD and decreased FA, MK, AK, and RK were observed in the corpus callosum, bilateral cingulate bundle, bilateral temporal and frontal regions of patients with early AD [Citation20]. According to a previous study, the FA and MK values of DKI are usually reduced due to microstructure damage to the cingulate cortex [Citation8]. Hong et al. used DTI to scan the cortex and hippocampus of patients with MCI and found decreased FA and increased MD [Citation21]. In addition, a meta-analysis of multimodal MRI also showed that neuronal activity in the right posterior cingulate gyrus and anterior cingulate gyrus of patients with MCI is decreased [Citation22]. These results are also consistent with the changes in the parameters observed in our study. Loss of the cell body and disintegration of the axons lead to decreases in the complexity of the microstructure and an increase in the free diffusion space outside the cell. The changes in these microstructures are further manifested as changes in DKI parameters. DKI provides supplementary information reflecting these changes and may be a sensitive marker for the early diagnosis of MCI [Citation23]. The posterior cingulate cortex combines bottom-up (uncontrolled) attention with information from memory and perception. The ventral (lower) activation of the posterior cingulate cortex involves all tasks of the default mode network, including self-related tasks, others-related tasks, remembering the past, thinking about the future, processing concepts and spatial navigation. The dorsal (upper) portion of the posterior cingulate cortex involves involuntary awareness and arousal [Citation24,Citation25]. These findings may explain why patients with MCI have cognitive dysfunction, especially in memory and learning.
Interestingly, our study found more severe changes in the right cingulate gyrus than in the left cingulate gyrus in the MCI group compared with the non-MCI group. Other studies have also reported inconsistent changes in bilateral DKI parameters, including other ROIs of patients with MCI or other neurological diseases [Citation26–29]. When we compared the DKI parameters of the cingulate gyrus in the left and right cerebral hemispheres of the MCI group, we observed substantial differences in the microstructures of the left and right cerebral hemispheres. Similar results were obtained for the non-MCI group. The arrangement of fiber bundles in the left and right hemispheres of the brain is not completely consistent, and some differences in functional regulation may exist. Because of the structural differences, the right hemisphere may be more sensitive to ischemia, resulting in severe damage to the microstructure of the right side and alterations in DKI parameters. At present, a final conclusion on this issue is unavailable, which is worthy of further discussion.
The severity of MCI is potentially reflected by MoCA scores, and we analyzed the correlation between MoCA scores and different DKI parameters. Pearson’s and Spearman’s correlation analyses also suggested closer correlations between some DKI parameters of the cingulate gyrus and MoCA scores, and the degree of microstructural changes may be related to the severity of MCI induced by CSVD. Our study revealed negative correlations between AD, MD, and RD and positive correlations between FA, AK, MK, and RK with MoCA scores. The kurtosis index appeared to provide increased sensitivity to group differences that occur in areas with complex fiber arrangements [Citation12]. Therefore, the changes in DKI parameters may indicate the degree of symptoms in patients with MCI induced by CSVD. Our results provide a basis for the microstructural changes in the cingulate gyrus and earlier identification of cognitive impairment in patients with CSVD.
Our study has some limitations. First, our sample size was small, and the results must be confirmed in studies investigating larger sample sizes. Second, the study lacked a normal control group, which must be added in future studies. Third, we only discussed MCI induced by CSVD but not other subtypes of vascular MCI. This study was designed to evaluate whether the major DKI indices would identify microstructural alterations in the brains of patients with MCI induced by CSVD, but the reversibility of these lesions could not be determined. Finally, given the inaccessibility of this technique compared with the traditional diagnostic method (cognitive assessment), the accuracy of DKI requires further verification.
Taken together, our study highlights the variation in microstructural alterations of the cingulate cortex in patients with MCI caused by CSVD. The results may be helpful for obtaining a better understanding of the pathological processes of MCI induced by CSVD and may have potential early diagnostic value or potential clinical value for intervening in the progression from MCI to dementia in patients with CSVD.
Acknowledgments
The authors would like to thank Dongtao Liu for excellent technical support and Professor Lichun Zhou for critically reviewing the manuscript.
Disclosure statement
No potential conflict of interest was reported by the authors.
Additional information
Funding
Notes on contributors
Huilin Liu
Huilin Liu is a postgraduate of Capital Medical University. She majors in cerebral small vessel disease and has several related publications.
Dongtao Liu
Dongtao Liu is a neurologist in Chaoyang Hospital. He majors in cerebrovascular intervention and cerebral small vessel disease and has several related publications.
Kun Li
Kun Liis a radiologist in Chaoyang Hospital. She got a PhD degree on radiology at Capital Medical University, Beijing China. She has several publications on cerebral vascular disease.
Xiaofan Xue
Xiaofan Xue is a neurologist in Chaoyang Hospital. She majors in cerebral vascular disease and neurodegenerative diseases and has several related publications.
Xiangke Ma
Xiangke Ma is neurosurgeon in Chaoyang Hospital. He got a PhD degree on neurosurgery at Capital Medical University, Beijing China. He has several publications on cerebrovascular intervention
Qiao Bu
Qiao Bu is a radiologist in Chaoyang Hospital. She majors in cerebral small vessel disease and has several related publications.
Jing Ma
Jing Ma is an ultrasound physician in Shanghai xuhui central hospital, Zhongshan-xuhui hospital, Fudan University, Shanghai, China. She is good at ultrasound diagnosis and has several related publications.
Zhenyu Pan
Zhenyu Pan is a radiologist in Chaoyang Hospital. She is good at MRI and CT in the diagnosis of musculoskeletal diseases and neurological diseases. She also has several related publications.
Lichun Zhou
Lichun Zhou is a neurologist in Chaoyang Hospital, and a vice-professor of Capital Medical University. He got a PhD degree on neurology at Capital Medical University, Beijing China. He has several publications in clinical research of ichemic stroke thrombolysis and cerebrovascular reserve.
References
- Petersen RC. Mild cognitive impairment as a diagnostic entity. J Intern Med. 2004;256:183–194.
- Sachdev PS, Lipnicki DM, Kochan NA, et al. The prevalence of mild cognitive impairment in diverse geographical and ethnocultural regions: the COSMIC collaboration. PLoS One. 2015;10:e0142388.
- Bruscoli M, Lovestone S. Is MCI really just early dementia? A systematic review of conversion studies. Int Psychogeriatr. 2004;16:129–140.
- Luis CA, Loewenstein DA, Acevedo A, et al. Mild cognitive impairment: directions for future research. Neurology. 2003;61:438–444.
- Sambuchi N, Geda YE, Michel BF. Cingulate cortex in pre-MCI cognition. Handb Clin Neurol. 2019;166:281–295.
- Cera N, Esposito R, Cieri F, et al. Altered cingulate cortex functional connectivity in normal aging and mild cognitive impairment. Front Neurosci. 2019;13:857.
- Chen X, Huang L, Ye Q, et al. Disrupted functional and structural connectivity within default mode network contribute to WMH-related cognitive impairment. Neuroimage Clin. 2019;24:102088.
- Allen JW, Yazdani M, Kang J, et al. Patients with mild cognitive impairment may be stratified by advanced diffusion metrics and neurocognitive testing. J Neuroimaging. 2019;29:79–84.
- Amlien IK, Fjell AM. Diffusion tensor imaging of white matter degeneration in Alzheimer’s disease and mild cognitive impairment. Neuroscience. 2014;276:206–215.
- Andica C, Kamagata K, Hatano T, et al. MR biomarkers of degenerative brain disorders derived from diffusion imaging. J Magn Reson Imaging. 2020;52:1620-1636.
- Arab A, Wojna-Pelczar A, Khairnar A, et al. Principles of diffusion kurtosis imaging and its role in early diagnosis of neurodegenerative disorders. Brain Res Bull. 2018;139:91–98.
- Marrale M, Collura G, Brai M, et al. Physics, techniques and review of neuroradiological applications of diffusion kurtosis imaging (DKI). Clin Neuroradiol. 2016;26:391–403.
- Shi Y, Wardlaw JM. Update on cerebral small vessel disease: a dynamic whole-brain disease. Stroke Vasc Neurol. 2016;1:83–92.
- Nasreddine ZS, Phillips NA, Bédirian V, et al. The montreal cognitive assessment, MoCA: a brief screening tool for mild cognitive impairment. J Am Geriatr Soc. 2005;53:695–699.
- Sachdev P, Kalaria R, O’Brien J, et al. Diagnostic criteria for vascular cognitive disorders: a VASCOG statement. Alzheimer Dis Assoc Disord. 2014;28:206–218.
- Suda S, Muraga K, Ishiwata A, et al. Early cognitive assessment following acute stroke: feasibility and comparison between mini-mental state examination and montreal cognitive assessment. J Stroke Cerebrovasc Dis. 2020;29:104688.
- Tzourio-Mazoyer N, Landeau B, Papathanassiou D, et al. Automated anatomical labeling of activations in SPM using a macroscopic anatomical parcellation of the MNI MRI single-subject brain. Neuroimage. 2002;15:273–289.
- Gatto RG, Amin M, Finkielsztein A, et al. Unveiling early cortical and subcortical neuronal degeneration in ALS mice by ultra-high field diffusion MRI. Amyotroph Lateral Scler Frontotemporal Degener. 2019;20:549–561.
- Qin L, Guo Z, McClure MA, et al. White matter changes from mild cognitive impairment to Alzheimer’s disease: a meta-analysis. Acta Neurol Belg. 2020.
- Yuan L, Sun M, Chen Y, et al. Non-gaussian diffusion alterations on diffusion kurtosis imaging in patients with early Alzheimer’s disease. Neurosci Lett. 2016;616:11–18.
- Hong YJ, Yoon B, Shim YS, et al. Gray and white matter degenerations in subjective memory impairment: comparisons with normal controls and mild cognitive impairment. J Korean Med Sci. 2015;30:1652–1658.
- Gu L, Zhang Z. Exploring structural and functional brain changes in mild cognitive impairment: a whole brain ALE meta-analysis for multimodal MRI. ACS Chem Neurosci. 2019;10:2823–2829.
- Gong NJ, Chan CC, Leung LM, et al. Differential microstructural and morphological abnormalities in mild cognitive impairment and Alzheimer’s disease: evidence from cortical and deep gray matter. Hum Brain Mapp. 2017;38:2495–2508.
- Andrews-Hanna JR, Smallwood J, Spreng RN. The default network and self-generated thought: component processes, dynamic control, and clinical relevance. Ann N Y Acad Sci. 2014;1316:29–52.
- Andrews-Hanna JR. The brain’s default network and its adaptive role in internal mentation. Neuroscientist. 2012;18:251–270.
- Li JL, Jiang H, Zhang XD, et al. Microstructural brain abnormalities correlate with neurocognitive dysfunction in minimal hepatic encephalopathy: a diffusion kurtosis imaging study. Neuroradiology. 2019;61:685–694.
- Liu D, Li K, Ma X, et al. Correlations between the microstructural changes of the medial temporal cortex and mild cognitive impairment in patients with cerebral small vascular disease (cSVD): a diffusion kurtosis imaging study. Front Neurol. 2019;10:1378.
- Vyas S, Singh P, Khandelwal N, et al. Evaluation of cerebral microstructural changes in adult patients with obstructive sleep apnea by MR diffusion kurtosis imaging using a whole-brain atlas. Indian J Radiol Imaging. 2019;29:356–363.
- Deardorff RL, Falangola MF, Babb JS, et al. Non-Gaussian diffusion MRI assessment of brain microstructure in mild cognitive impairment and Alzheimer’s disease. Magn Reson Imaging. 2013;31:840–846.