Abstract
In a communication network, point-to-point traffic volumes over time are critical for designing protocols that route information efficiently and for maintaining security, whether at the scale of an Internet service provider or within a corporation. While technically feasible, the direct measurement of point-to-point traffic imposes a heavy burden on network performance and is typically not implemented. Instead, indirect aggregate traffic volumes are routinely collected. We consider the problem of estimating point-to-point traffic volumes, , from aggregate traffic volumes,
, given information about the network routing protocol encoded in a matrix A. This estimation task can be reformulated as finding the solutions to a sequence of ill-posed linear inverse problems,
, since the number of origin-destination routes of interest is higher than the number of aggregate measurements available.
Here, we introduce a novel multilevel state-space model (SSM) of aggregate traffic volumes with realistic features. We implement a naïve strategy for estimating unobserved point-to-point traffic volumes from indirect measurements of aggregate traffic, based on particle filtering. We then develop a more efficient two-stage inference strategy that relies on model-based regularization: a simple model is used to calibrate regularization parameters that lead to efficient/scalable inference in the multilevel SSM. We apply our methods to corporate and academic networks, where we show that the proposed inference strategy outperforms existing approaches and scales to larger networks. We also design a simulation study to explore the factors that influence the performance. Our results suggest that model-based regularization may be an efficient strategy for inference in other complex multilevel models. Supplementary materials for this article are available online.
Acknowledgments
The authors thank Jin Cao and Matthew Roughan for providing point-to-point traffic volumes, and the referees for providing valuable suggestions. This work was partially supported by the Army Research Office under grant 58153-MA-MUR, and by the National Science Foundation under grants IIS-1017967, DMS-1106980, and CAREER IIS-1149662.
Notes
This information is encoded by the routing protocol.
Figure 1 Illustration of the mathematical quantities in network tomography. Traffic x 13 from sn 1 to sn 3 contributes to counter Y 1a into rs a, to counter Yab into rs b, to counter Ybc out of rs b (which is the same as counter Ybc into rs c), and to counter Y c3 out of rs c. Traffic volumes on these counters are recorded every few minutes. This routing information is summarized in the column of the routing matrix A that corresponds to the OD traffic volume x 13.
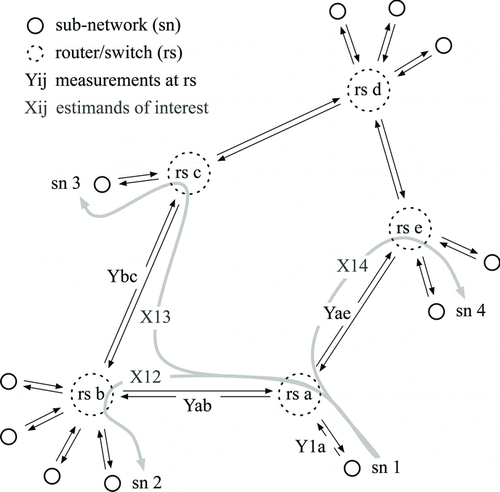
Any such two orderings can be arbitrary and defined independently of each other. Different pairs of orderings will lead to different A matrices.