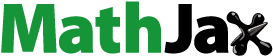
Saharon Rosseta and Ryan J. Tibshiranib
aDepartment of Statistics and Operations Research, Tel Aviv University, Tel Aviv, Israel; bDepartment of Statistics and Data Science, Carnegie Mellon University, Pittsburgh, PA
In our article (Rosset and Tibshirani Citation2020) Theorem 3 is incorrect as stated. Recalling the definition earlier in the articlethe original Theorem states:
Assume that is generated as follows: we draw
, having iid components
, where F is any distribution with zero mean and unit variance, and then set
, where
is positive definite and
is its symmetric square root. Consider an asymptotic setup where
as
. Then, for the OLS estimator,
This theorem is incorrect as stated because V + is defined in terms of an expectation over X. However, under the assumptions of the theorem, the eigenvalues of the empirical covariance matrix may not be bounded away from zero, and consequently the almost sure convergence of the eigenspectrum used in the proof does not guarantee convergence (or existence) of the expectation. One can generate counterexamples along the lines of the classic examples of almost sure convergence not implying convergence in expectation.
We note that under the same conditions (with the added requirement that the fourth moment of F is finite), Hastie et al. (Citation2021) proved (in their proof of Proposition 2) that
In words, for almost any sequence of training covariate matrices X generated by this mechanism, the random variable whose expectation is V + converges to this same fixed limit.
Thus, we conclude that the failure of Theorem 3 as written in the original article is really somewhat of an “edge case”: Although it may not apply to our formal definition of V +, which integrates over the distribution of X, the excess variance result does apply in asymptotic almost sure sense over X. In terms of practical use of the corrections proposed later in the article, we believe it still supports their wide applicability in estimating Random-X prediction error.
Acknowledgments
We thank an anonymous reviewer of a separate article for pointing out the difficulty with the original result.
ORCID
Saharon Rosset http://orcid.org/0000-0002-4458-9545
References
- Hastie, T., Montanari, A., Rosset, S., and Tibshirani, R. (2021), “Surprises in High-Dimensional Ridgeless Least Squares Interpolation,” arXiv: 1903.08560.
- Rosset, S., and Tibshirani, R. J. (2020), “From Fixed-x to Random-x Regression: Bias-Variance Decompositions, Covariance Penalties, and Prediction Error Estimation,” Journal of the American Statistical Association, 115, 138–151. DOI: https://doi.org/10.1080/01621459.2018.1424632.