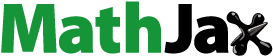
Statistical Modeling with R, subtitled A Dual Frequentist and Bayesian Approach for Life Scientists, is a recent book written by Pablo Inchausti. It is composed in a highly personal and congenial style, witness the highly original preface that contains many biographical details.
The very first sentence of the introduction is a quote from the late Steve Fienberg (Citation2014) about definitions of statistic, which is in my opinion definitely starting on the right foot! The exposition of the motivations for writing the book is quite convincing, with more emphasis than in usual textbooks put on the notion and limitations of modeling, and rightly so. The message is overall inspirational and the text contains many relevant remarks and links that make the book worth reading as a whole. While heavily connected with a few R packages like fitdist, fitistrplus, brms (a front for Stan), glm, and glmer, the book is wisely bypassing the perilous reef of recalling R bases. Similarly it mostly avoids resetting the foundations of probability and statistics. While lacking in formal definitions, imho, its buildup of statistical modeling reads well enough to somehow compensate for this very lack. I also appreciate the coherent and throughout continuation of a parallel description of Bayesian and non-Bayesian analyses, since this an attempt that often too often quickly disappears in other books. (As a minor aside, I would like to point out that hardly anyone claims to be a frequentist.) In the repeating pattern of (many) chapters, a new model is almost invariably backed by a new dataset, oten inspired from life sciences, if a few of them are somewhat inappropriate as in the mammal sleep patterns of Chapter 5.
Given that the main motivation for the book (when compared with heavyweight references like Gelman et al. Citation2013) is strongly leaning toward the practical implementation of statistical modeling via R packages, it is inevitable that a large fraction of Statistical Modeling with R is spent on the analysis of R outputs, even though it sometimes feels a wee bit too heavy for yours truly. The R screen-copies are however produced in moderate quantity and size, even though the variations in typography/fonts (at least in my copy) may prove confusing. Obviously the high number of flavors of regression models may eventually prove challenging for the novice reader. The specific issue of prior input (or “defining priors”) is briefly addressed in a non-chapter (p. 323), although mentions are made throughout preceeding chapters. I commend the nice appearance of hierarchical models and experimental designs toward the end, but would have appreciated some discussions on other topics such as time series, causality, connections with machine learning, non-parameterics, model misspecification. As an aside, I also appreciated being reminded about the apocryphal nature of Ockham’s much cited quote “Pluralitas non est ponenda sine necessitate.”
The book is carefully composed but I still noticed a wrongly spelled Jeffries found in Fig. 2.1, And Jon Wakefield’s Citation2013 book (with related goal of presenting both versions of parametric inference) was mistakenly entered as Wakenfield’s in the bibliography file. The unavoidable “the the” typo occurs at least twice (pp. 174 and 422). While rare, some repetitions occur. I do not much like the use of the equivalence symbol for proportionality. I also found the timeline representation of the history (!) of both frequentist and Bayesian statistics a rather sketchy one (Fig. 2.1) and presumably lost on students. I also had trouble with some sentences like “long-run, hypothetical distribution of parameter estimates known as the sampling distribution” (p. 27), “maximum likelihood estimates [being] sufficient” (p. 28), “Jeffreys’ (1939) conjugate priors” [which were introduced by Raiffa and Schlaifer] (p. 35), “A posteriori tests in frequentist models” (p. 130), “exponential families [having] limited practical implications for non-statisticians” (p. 190), “choice of priors being correct” (p. 339), or calling MCMC sample terms “estimates” (p. 42), and have issues with some repetitions (joke), while missing indexes for acronyms, packages, and datasets, but I do not bemoan the lack of homework sections (beyond suggesting new datasets for analysis).
A problematic MCMC entry (for me) is occuring when calibrating the choice of the Metropolis–Hastings proposal toward avoiding negative values “that will generate an error when calculating the log-likelihood” (p. 43) since (a) this is not the case with a Normal proposal and, more importantly, (b) it suggests proposed values should not exceed the support of the posterior, while pointing out a poor coding of the log-likelihood! I also find the motivation for the full conditional decomposition behind the Gibbs sampler (p. 47) unnecessarily confusing. (And automatically having a Metropolis–Hastings within Gibbs step as on Fig. 3.9 brings another magnitude of confusion.) The Bayes factor section is terse to the point of turning into a ghost section. The derivation of the Kullback-Leibler representation (7.3) as an expected log likelihood ratio seems to be missing a reference measure. Of course, as a matter of personal taste (DeIorio and Robert Citation2002), seeing a detailed coverage of DIC (Section 7.4) did not suit me either, even though the issue with mixtures was alluded to (with no detail whatsoever). The Nelder presentation of the generalized linear models felt somewhat antiquated, since the addition of the scale factor sounds over-parameterized.
But those are minor issues in relation to a book that should attract curious minds of various background, knowledge, and expertise in statistics, as well as work nicely to support an enthusiastic teacher of statistical modeling. I thus recommend this book most enthusiastically.
Paris Dauphine University
Paris Cedex 16, France
[email protected]
References
- DeIorio, M., and Robert, C. P. (2002), “Discussion of Spiegelhalter et al.” Journal of the Royal Statistical Society, Series B, 64, 629–630.
- Fienberg, S. (2014), “What Is Statistics?” Annual Review of Statistics and Applications, 1, 1–19. DOI: 10.1146/annurev-statistics-022513-115703.
- Gelman, A., Carlin, J. B., Stern, H. S., Dunson, D. B., Vehtari, A., and Rubin, D. B. (2013), Bayesian Data Analysis (3rd ed.), London: Chapman and Hall/CRC.
- Wakefield, J. (2013), Bayesian and Frequentist Regression Methods, New York: Springer.