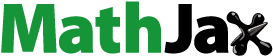
ABSTRACT
This study formalizes and compares two major hypotheses in speakers’ choices of referring expressions: the topicality model that chooses a form based on the topicality of the referent, and the rational model that chooses a form based on the informativity of the form and its speech cost. Simulations suggest that both the topicality of the referent and the informativity of the word are important to consider in speakers’ choices of reference forms, while a speech cost metric that prefers shorter forms may not be.
Introduction
Speakers and writers choose a reference form when they refer to someone or something. The range of reference forms varies between more-specific forms like “the 44th president of the United States” or “Barack Obama” and less specific forms like “he.” Researchers have suggested how speakers choose an appropriate form from these choices given the context. Recently, Arnold and Zerkle (Citation2019) suggest that models of reference production can fall into two classes: one that “proposes a mapping between cognitive/discourse representations and reference form” (Arnold & Zerkle, Citation2019, p. 2) and one that is “driven by two constraints: a need to be informative … and a desire to be efficient” (Arnold & Zerkle, Citation2019, p. 11).
Models of the first type suggest that speakers use more attenuated referring expressions such as pronouns when they think that a referent is salient/accessible/topical in its cognitive (discourse or information) status (Ariel, Citation1990; Chafe, Citation1994; Givón, Citation1983; Grosz et al., Citation1995; Gundel et al., Citation1993; Prince, Citation1981), wherein the status of the referent is often associated with its accessibility or activation in memory (Almor, Citation1999; Arnold, Citation2016; Bock & Warren, Citation1985; Chafe, Citation1974; Foraker & McElree, Citation2007; Sanford & Garrod, Citation1981). Crucially, these theories propose an explicit mapping between the referent’s cognitive status and the referring expression used to refer to it. Speakers signal the referent’s cognitive status by using a particular form to help the addressee identify the intended referent.
Models of the second type suggest that speakers choose a reference form based on the word’s informativity together with speech cost of the reference form: Speakers choose a less costly form when the word is informative and vice versa. The speech cost in this context predicts that, for example, shorter forms are easier to produce (Aylett & Turk, Citation2004; Fukumura & van Gompel, Citation2012). The Rational Speech Act model (RSA; M. Frank & Goodman, Citation2012) explicitly formalizes this idea. RSA models capture inferences between speakers and listeners in the context of Gricean pragmatics (Grice, Citation1975). These models take a game theoretic approach in which speakers optimize productions to convey information for listeners and listeners infer meaning based on speakers’ likely productions. These models have been argued to account for human communication (M. Frank & Goodman, Citation2012; Jager, Citation2007), and studies report that the models robustly predict various linguistic phenomena in experimental settings (see Goodman & Frank, Citation2016, for a comprehensive review). The speaker in the RSA framework chooses a word based on its informativity along with its speech cost that prefers less costly expressions for the speaker. A similar idea has also been suggested in other information theoretic studies. Tily and Piantadosi (Citation2009) estimated the predictability of referents (surprisal) based on participants’ accuracy of guessing the correct referents given a preceding discourse. They found that this measure of predictability was a significant predictor in writers’ choices of referring expressions: both pronouns and names were more likely to be used than definite descriptions when a referent was predictable. Though speech cost was not explicitly estimated and included in the analysis, they clearly hypothesized a relationship between predictability and cost: “More predictable meanings should be given shorter words” (Tily & Piantadosi, Citation2009, p. 1). The relation between predictability of the referent and the choice of referring expression has also been proposed in the context of the Uniform Information Density hypothesis: “Speakers should be more likely to produce pronouns (e.g., she) instead of full noun phrases (e.g., the girl) when reference to the expression’s referent is probable in that context” (Jaeger, Citation2010, p. 48).
The influence of referential predictability on speakers’ choices of referring expressions has been examined in various psycholinguistic experiments. In these studies, the referent’s predictability is manipulated using verb semantic bias, so called implicit causality (Garvey & Caramazza, Citation1974; Stevenson et al., Citation1994, among many). For example, a verb admire in a sentence “John admires Mary” creates a bias toward re-mentioning the referent that causes the event. In this example sentence, Mary is a causee, thus yielding a bias to re-mentioning Mary in the following sentence (i.e., Mary is a more predictable referent). Previous studies have examined whether this kind of referential predictability induced by the verb semantics affects the form choice. On one hand, speakers are more likely to refer to implicit causes, but this implicit causality bias does not affect speakers’ choices of reference forms (e.g., Fukumura & van Gompel, Citation2010; Kehler et al., Citation2008; Kehler & Rohde, Citation2013; Stevenson et al., Citation1994). On the other hand, speakers are more likely to use pronouns to refer to goals than sources (e.g., Rosa & Arnold, Citation2017; Zerkle & Arnold, Citation2016). Moreover, a recent experiment with a novel production task showed that speakers’ use of pronouns increases even with the implicit causality verbs (Weatherford & Arnold, Citation2020). Thus, the effect of verb semantic bias on pronoun production seems to depend on the verb types and task, and the influence of this kind of referential predictability on form choice still remains under debate. We show here that referential predictability, as estimated by recency of the referent, does contribute to capture speakers’ choices of reference forms, suggesting that referential predictability is still important to consider in reference production problems despite the lack of an effect found with implicit causality verbs in some studies.
While these two classes of theoretical models are well established, there have been few previous computational cognitive models that aim to account for speakers’ choices of referring expressions (Gatt et al., Citation2014, p. 904). Centering (Grosz et al., Citation1995) is a theory for discourse coherence and was not built to explain speakers’ choices of referring expressions (Poesio et al., Citation2004). Referring Expression Generation (REG) models mostly focus on speakers’ choices of properties (i.e., the content of descriptions) rather than forms (Dale & Reiter, Citation1995; Krahmer & Van Deemter, Citation2012; Van Deemter et al., Citation2012). There are a few exceptions among REG models, but these are engineering oriented and were not specifically built to explain speakers’ word choice (Callaway & Lester, Citation2002; Kibble & Power, Citation2004; Reiter et al., Citation2000).
In this paper we build two computational models and compare their ability to predict choices of referring expressions. The first model instantiates the hypothesis suggested in discourse theories that there is a mapping between the referent’s information status and reference form (e.g., Ariel, Citation1990; Givón, Citation1983; Gundel et al., Citation1993). Various factors that influence the referent’s information status have been suggested, such as given-new information (Chafe, Citation1976; Prince, Citation1981), recency (Chafe, Citation1994; Clancy, Citation1980; Fletcher, Citation1984), animacy (Fukumura & van Gompel, Citation2011; Vogels et al., Citation2013a), and topicality (Ariel, Citation1990; Arnold, Citation1998; Givón, Citation1983; Grosz & Sidner, Citation1986). A real discourse model in the speaker’s mind would make use of a combination of these factors, but for the purpose of this study, our first speaker model chooses a referring expression based on the topicality of the referent.Footnote1 Following previous literature (Kehler & Rohde, Citation2013; Rohde & Kehler, Citation2014), topicality here means the likelihood of being the topic, whereby the topic is a concept of information structure that indicates what the sentence is about (Kuno, Citation1972; Reinhart, Citation1981, inter alia). Note that the term topicality here does not represent the global topic (e.g., what an entire document is about; for the relation between the global topic and speakers’ choices of referring expressions, see Orita et al. (Citation2014)). Many researchers agree that speakers are more likely to use reduced forms such as pronouns when they think that a referent is topical (Ariel, Citation1990; Broadbent, Citation1973; Kehler et al., Citation2008; Rohde & Kehler, Citation2014; Sanford & Garrod, Citation1981) and that the referent is more likely to be topical when it has been mentioned in a subject position (Chafe, Citation1976; Givón, Citation1990).Footnote2 The correlation between subjecthood and the choices of referring expressions has been robustly supported in previous psycholinguistic experiments: a referent that is last mentioned in the subject position is more likely to be mentioned by a pronoun (Arnold, Citation1998; Fukumura & van Gompel, Citation2010; Kehler et al., Citation2008; Kehler & Rohde, Citation2013; Rohde & Kehler, Citation2014; Stevenson et al., Citation1994). The first model reflects this well-supported hypothesis in the literature: A speaker chooses a form based on the topicality of the referent. We operationalize the topicality of the referent by looking at its grammatical position. We call this model the topicality model.
The second model formalizes the information theoretic hypothesis by extending the Rational Speech Act model (M. Frank & Goodman, Citation2012). This model formalizes a speaker who chooses referring expressions by considering the amount of information that each word carries in the discourse and the speaker’s own speech cost. We call this model the rational model. In deriving our extension of the RSA model, we also show that predictions previously attributed to the notion of predictability in this domain (Jaeger, Citation2010; Levy & Jaeger, Citation2007; Tily & Piantadosi, Citation2009) can be derived from the rational speaker model in a fully explicit manner.
There are two major differences between the topicality model and the rational model. First, these two models differ in whether the form per se is considered when choosing the form. On the one hand, the topicality model chooses the form solely based on the referent’s status. On the other hand, the rational model considers the informativity of the form, together with speech cost of that form. As we describe later in this paper, the notion of informativity in the rational model basically corresponds to specificity. When the use of a particular form increases the number of competitors in the discourse representation that are potentially compatible with the form (e.g., “he” may have more competitors than “Barack Obama”), the informativity of that form decreases. The influence of competitors on speakers’ choices of referring expressions has been supported by several experiments: speakers are less likely to use pronouns when there was an additional character in the discourse (Arnold & Griffin, Citation2007; Fukumura & van Gompel, Citation2010). Here we test a model in which competitors influence choices of referring expressions by decreasing the informativity of ambiguous referring expressions, though it is possible that the effect of competitors instead comes from their influence on the salience of the referent (Ariel, Citation1990; Givón, Citation1983) or their effect on cognitive load (Arnold & Griffin, Citation2007).
Second, the two models differ in whether speech cost is taken into account. While the topicality model chooses a reference form based solely on the topicality of the referent, the rational model chooses a form by considering both informativity of the form and speech cost of that form. Speech cost in the rational model represents a speaker’s preference to use a less costly form. For example, with the informativity of two different forms being equal, the rational speaker prefers to use a form that is easier to produce (e.g., shorter or involving easier lexical access). However, the preference for using an easier form has relatively little empirical support in experimental studies on reference production (for a comprehensive review, see Arnold & Zerkle, Citation2019).
Computational modeling is a good tool to make all components and information sources explicit and measure to what extent each component helps to capture observed behavior. In this study, we explicitly examine whether and to what extent the topicality of the referent, informativity of the word, and speech cost can predict speakers’ choices between third-person singular names and pronouns. We choose to focus on third-person singular names and pronouns because these are the most-well-studied items among various types of referents and expressions. We evaluate models’ predictions using AUC (Area Under the Curve: a metric for binary classification) and BIC (Bayesian information criterion: a probabilistic metric). Simulation 1 shows that the two models achieve similar performance in our prediction task when measured with both AUC and BIC but that they capture different aspects of speakers’ behavior. Simulation 2 conducts an ablation test to examine which components in the rational model are critical for predicting speakers’ choice between names and pronouns. We find that when the rational model is unable to compute the informativity of the form—that is, when it lacks either knowledge of referential predictability or knowledge of unseen competitors—it performs worse on both AUC and BIC measures. On the other hand, the rational model without speech cost actually performs slightly better than the complete rational model on the AUC metric. These results together suggest that both the topicality of the referent and the predictability of the referent are important to consider in the problem of referential production, but that a speech cost that prefers shorter forms may not play a significant role in speakers’ choices of reference forms, in line with the previous behavioral experiments (Arnold & Zerkle, Citation2019).
We begin by describing our implementation of the topicality model, then move to our extension to the rational model, showing how predictions suggested from UID in this domain can be derived in that framework. We then describe our simulations and their results. We conclude by discussing the implications of this study.
Topicality model
The topicality model instantiates the hypothesis suggested in discourse theories that there is a mapping between a referent’s information status and reference form (e.g., Ariel, Citation1990; Givón, Citation1983; Gundel et al., Citation1993). In particular, the model reflects a hypothesis that speakers are more likely to use reduced forms such as pronouns when they think that a referent is topical (e.g., Ariel, Citation1990; Broadbent, Citation1973; Kehler et al., Citation2008; Rohde & Kehler, Citation2014; Sanford & Garrod, Citation1981) and that the referent is more likely to be topical when it has been mentioned in a subject position (cf. Chafe, Citation1976; Givón, Citation1990). The topicality model implements this relation between grammatical position and the choices of referring expressions.
For each possible grammatical position of the previous mention of a referent, there is a different probability of the form, a name or a pronoun, in the current mention. To formalize this probability, we used a corpus to count the grammatical position of the referents whose next mention is either a name or a pronoun. The details of the corpus we used are given in the following simulation section. We then broke these counts into the number of referents that occur in the previous adjacent sentences and the number of referents that occur elsewhere. The latter consists of first mentions that have no previous referent and referents in preceding non-adjacent sentences as in .Footnote3 To identify the grammatical position of the referent, we use annotated dependency relation tags in a corpus: subject, object, oblique object, and other (e.g., appositive and vocative).
Table 1. Counts of third-person pronouns’ and names’ referents in each grammatical position and maximum likelihood estimates of pronoun choice bias based on these counts
We use these counts to compute maximum likelihood estimates of form-choice bias based on the grammatical position of the referent. For example, the maximum likelihood estimate of pronoun choice for a subject referent in a previous sentence can be obtained as in EquationEquation (1)
(1)
(1) :
where indicates the number of pronouns that have the subject referents in the previous sentence and
indicates the number of proper names that have the subject referents in the previous sentence. In this way, each position of the referent is mapped to particular forms (name and pronoun) with a particular probability; for example, the referent in the subject position of the immediately previous sentence is likely to be referred to by a pronoun with
and by a name with
. The following describes a procedure of how the topicality model selects the reference form using these maximum likelihood estimates.
For each mention position, (a) check the position of its previous antecedent mention (if any) and (b) look up the maximum likelihood estimates () and get the
value for that position.
For the AUC measure, check whether the
value crosses the threshold. If it does, the model predicts a pronoun. If not, the model predicts a name.
For the BIC measure, treat the
value as the probability of producing a pronoun.
The values of are between zero and one and they influence the model’s tendency to use a pronoun to refer to an entity. Note that the model’s threshold for deciding to use a pronoun is not necessarily 0.5; that is, the topicality model does not predict that a reference form will always be a pronoun when the referent is in the subject position in the previous sentence nor does it always predict a proper name in other situations. Our analyses in this paper explore performance over all possible thresholds (see Section 4.2.1 for details).
Our description of the topicality model is relatively simple compared to the description of the rational model in the next section.Footnote4 However, the topicality model nevertheless reflects a well-supported hypothesis in the literature: Speakers are more likely to use reduced forms such as pronouns when they think that a referent is topical (e.g., Ariel, Citation1990; Broadbent, Citation1973; Kehler et al., Citation2008; Rohde & Kehler, Citation2014; Sanford & Garrod, Citation1981) and that there is a correlation between topicality and the grammatical position of the referent (Chafe, Citation1976; Givón, Citation1990). Thus, evaluating this model is important relative to previous literature. While the grammatical position of the previous mention of a referent is only one heuristic for that referent’s topicality, we show in our simulations that this heuristic allows us to predict the forms of referring expressions with considerable accuracy.
Rational model
Original RSA model
RSA models capture inferences between speakers and listeners in the context of Gricean pragmatics (Grice, Citation1975). These models take a game theoretic approach in which speakers optimize productions to convey information for listeners and listeners infer meaning based on speakers’ likely productions. These models have been argued to account for human communication (M. Frank & Goodman, Citation2012; Jager, Citation2007), and studies report that they robustly predict various linguistic phenomena in experimental settings (see Goodman & Frank, Citation2016, for a comprehensive review).
The main idea of the RSA model is that a rational pragmatic listener uses Bayesian inference to infer the speaker’s intended referent given the word
that they hear, their vocabulary (e.g., “blue”, “circle”), and shared context that consists of a set of objects
(e.g., visual access to object referents) as in EquationEquation (2)
(2)
(2) . The following describes a representative RSA model in M. Frank and Goodman (Citation2012). While our work does not make use of this pragmatic listener, it does build on the speaker model assumed by the pragmatic listener.
This listener infers a speaker’s intended referent based on three terms: the likelihood
representing a speaker model in the listener’s mind; the prior
representing salience of the referent
; and the denominator, which is a normalizing constant. This listener assumes that a speaker is rational and that she has chosen the word informatively. The listener’s speaker model
is defined using an exponentiated utility function as in EquationEquation (3)
(3)
(3) .
The parameter specifies the extent to which the speaker rationally chooses the word and is typically set to 1 to approximate a rational decision process; hereafter we set
to 1. The exponentiated utility
is defined as
where represents informativeness of word
(quantified as surprisal) and
represents its speech cost. In other words, this speaker chooses a word that is maximally informative and minimally expensive to speak.
In M. Frank and Goodman (Citation2012), the meaning of word in context
is defined as the set of objects that the word applied to, where
denotes the number of referents that the word
can be used to refer to:
The informativeness of word can be expanded using the above definition of word meaning and the notion of surprisal. In information theory (Shannon, Citation1948), surprisal or the information content of an event is defined as the negative log probability of that event:
. Speakers in this model consider the information content that a word carries about its referent—that is, the probability of the referent given the word, which we denote as
. A higher surprisal (lower probability) means that an event is less predictable, and the rational speaker would be less willing to use a word with high surprisal. Because the meaning of word
is defined as the distribution over referents that the word applied to (EquationEquation (5)
(5)
(5) ), this probability distribution corresponds to the meaning of the word in their model, and the information content of the word is
Frank and Goodman use the negative of the surprisal from EquationEquation (7)(7)
(7) ,
as the informativeness of a word
in their utility function (EquationEquation (4)
(4)
(4) ). If a listener interprets word
literally and cost
is constant, the exponentiated utility function in EquationEquation (3)
(3)
(3) can be reduced to EquationEquation (8)
(8)
(8) by plugging EquationEquation (7)
(7)
(7) into Equation (3).
Thus, the default speaker model in M. Frank and Goodman (Citation2012) chooses a word based on its specificity. We will show next that this model corresponds to a speaker who is optimizing informativeness for a listener with uniform beliefs about what will be referred to in the discourse.
The assumption of uniform beliefs about referents works well in a simple language game situation wherein there are a limited number of referents that have roughly equal salience, but we show in our simulations that it falls short in more realistic settings. Here we extend the RSA model to predict speakers’ choices of referring expressions using referential predictability that changes as discourse proceeds.
Rational model for predicting speakers’ choices of referring expressions
To extend Frank and Goodman’s model to a natural linguistic situation, the rational model in this study considers referential predictability that changes as discourse proceeds, in contrast to their speaker model that chooses a word with uniform predictability of referents. Here we describe general assumptions of the rational model. We show that the rational model predicts that speakers choose a word based on its information content—that is, referential predictability, deriving the predictions that had been suggested in the context of UID.
We extend the speaker model from EquationEquation (8)(8)
(8) by allowing the speaker to estimate the listener’s interpretation of a word
based on discourse information, by incorporating a non-uniform distribution over referents in the speaker’s listener model. Following Frank and Goodman, we assume that a speaker
chooses
to optimize a listener’s belief in the speaker’s intended referent
relative to the speaker’s own speech cost
. EquationEquation (9)
(9)
(9) represents this speaker:
This speaker model corresponds to Frank and Goodman’s exponentiated utility function in Equation (3), with equal to one (as in Frank and Goodman’s simulations) and with their cost
being the log of our cost
.
The term in EquationEquation (9)
(9)
(9) is a cost function: The speaker prefers
when it is less costly to speak. In general, the cost function roughly corresponds to utterance complexity such as word length, though it was constant in Frank and Goodman’s simulations (see supplementary materials in M. Frank & Goodman, Citation2012).
The listener model in the speaker’s mind in EquationEquation (9)
(9)
(9) represents informativeness of word
: The speaker chooses a
that most helps a listener in the speaker’s mind
to infer referent
. This listener model infers a referent
that is referred to by word
according to Bayes’s rule as in EquationEquation (10)
(10)
(10) .
The first term in the numerator, , is a word probability: The listener in the speaker’s mind guesses how likely the speaker would be to use
to refer to
. The second term in the numerator,
, is the predictability of referent
—that is, the likelihood that referent
will be mentioned at a particular point in the discourse. This term enables the model to update a referent’s predictability as the discourse proceeds.
The denominator is a sum of potential referents
that could be referred to by word
. The terms in this sum are non-zero only for referents that are compatible with the meaning of word
. If there are many potential referents that could be referred to by word
, that word would be more ambiguous and thus less informative.
The whole of the right side in EquationEquation (10)(10)
(10) represents the speaker’s assumption about the listener: Given word
, the listener would infer referent
that is probable in a discourse and less ambiguously referred to by word
. If
is uniform over referents and
is constant across words and referents, this listener model reduces to
. Thus, M. Frank and Goodman’s (Citation2012) speaker model in EquationEquation (8)
(8)
(8) is a special case of this speaker model in EquationEquation (9)
(9)
(9) that assumes uniform referential predictability and constant cost.
More generally, this model predicts that the speaker’s probability of choosing a word for a given referent should depend on its cost relative to its information content. To see this, we combine EquationEquations (9)(9)
(9) and (Equation10
(10)
(10) ), yielding
Because the speaker is deciding what word to use for an intended referent, and the term denotes the predictability of this referent,
is constant in the speaker model and does not affect the relative probability of a speaker producing different words. For example,
for choosing word “she” to refer to an entity Alice and
for choosing word “Alice” to refer to an entity Alice are the same:
is independent from the selection of a particular word.
We further assume for simplicity that is constant across words and referents and the word probability for competitor referents,
, is zero for all incompatible referents. Having a constant value for
means that all referents have about the same number of words that can be used to refer to them and that all words for a given referent are equally probable for a naive listener.
Given these assumptions, the speaker’s probability of choosing a word is derived as follows (see Appendix A for a full derivation).
The denominator in the first term in EquationEquation (12)(12)
(12) is a sum over the predictability of potential referents that are compatible with word
. In this scenario, the information conveyed by a word is the logarithm of the first term in Equation (12):
This logarithm of the first term corresponds to the word’s information content (surprisal), which is the negative sum of predictability of potential referents in the discourse. More potential referents, such as using a pronoun, decreases its information content and fewer potential referents, such as using a name, increases its information content. In this way, the first term explicitly captures the contribution of discourse salience to the informativity of the word.
Plugging the right side term in EquationEquation (13)(13)
(13) into EquationEquation (12)
(12)
(12) suggests that in deciding which word to use, the highest cost a speaker should be willing to pay for a word should depend directly on that word’s information content. This relationship between cost and information content allows us to derive the prediction tested by Tily and Piantadosi (Citation2009). For referents that are highly predictable from the discourse, different referring expressions (e.g., pronouns and proper names) will have roughly equal information content and speakers should choose the referring expression that has the lowest cost, such as pronouns, which are shorter and less costly than proper names. In contrast, for less predictable referents, proper names will carry substantially more information than pronouns, leading speakers to pay a higher cost for the proper names.
These are the same predictions that have been discussed in the context of the Uniform Information Density hypothesis (UID; Levy & Jaeger, Citation2007). For example, Jaeger (Citation2010, p. 48) states that “speakers should be more likely to produce pronouns (e.g., she) instead of full noun phrases (e.g., the girl) when reference to the expression’s referent is probable in that context.” However, this case differs in important ways from previous cases in which UID was applied. Previous UID studies all focused on deciding between forms of different length that carry the same information content (Aylett & Turk, Citation2006; Bell et al., Citation2003; A. Frank & Jaeger, Citation2008; Mahowald et al., Citation2013; Van Son & Van Santen, Citation2005), but the problem of choosing referring expressions is fundamentally different. Different forms that can refer to the same referent convey different amounts of information and different content. For example, “she,” “the girl,” and “Alice” can be used to refer to the referent Alice, but “she” could refer to any singular and female entity and “Alice” refers to a particular person. Therefore, it is not clear how the relation between referential predictability and speakers’ choices of referring expression is predicted from the UID framework. Here we have instead shown that the predictions are directly derived from an explicit model of a rational speaker who is trying to provide information to listeners.
Implementing the rational model
Implementing the above rational model requires computing word probabilities , discourse salience
, and word costs
. The following illustrates how we implement each term in turn.
Word probability
We simplify the word probability in the embedded listener model as in EquationEquation (14)
(14)
(14) :
where the count is the number of words that can refer to referent
. There could be many ways to refer to a single entity. For example, to refer to entity Barack Obama, we could say “he,” “the U.S. president,” “Barack,” and so on. As a first pass, we assume that
is constant across all referents—that is, there are the same number of referring expressions for each entity. We also assume that each referring expression is equally probable under the listener’s likelihood model in the speaker’s mind. We set these assumptions as a first step, because to our knowledge no explicit model of
in the embedded listener model has previously been proposed.
In our simulations, we assume that a speaker is choosing between a proper name and a pronoun (i.e., ); for example, we assume that an entity Barack Obama has one and only one proper name “Barack Obama,” and this entity is unambiguously associated with male and singular. Although we use an example with two possible referring expressions, as long as
is constant across all referents and words, it does not make a difference to the computation in EquationEquation (10)
(10)
(10) how many competing words we assume for each referent.
Referential predictability
To estimate the predictability of a referent, , we use recency as a proxy that is straightforward to quantify. Previous studies have suggested that recently mentioned entities correlate with what speakers are more likely to refer to next in the discourse (e.g., Arnold, Citation1998; Chafe, Citation1994; Givón, Citation1983). There is another well-studied factor of referential predictability, next-mention bias induced by verb semantics (thematic roles). However, the findings thus far are conflicting, as described in this article’s introduction. Thus, our study focuses on recency to estimate referential predictability.
We assume that the speaker’s listener model does not know the number of entities nor the referential predictability of each entity in a discourse a priori. To represent this assumption in a principle way, we adopt a prior distribution of a Bayesian nonparametric model (Blei & Frazier, Citation2011) that has been used to represent the distribution over entities in a discourse (Haghighi & Klein, Citation2010). Nonparametric Bayesian methods assume that the data distribution can be defined by an infinite-dimensional parameter space to flexibly capture the data as the size of the data grows. By using the Bayesian nonparametric prior, we can flexibly capture the embedded listener’s prior distribution over what will be referred to next in the discourse. EquationEquation (15)(15)
(15) illustrates the speaker’s assumptions about the listener’s recency-based discourse model:
For each referent , the speaker’s listener model decides whether it is new or old with respect to the preceding discourse. If the referent has been mentioned before,
is estimated in proportion to
, which captures recency, where the recency function
decays exponentially with the distance
. The distance
represents the distance between the current mention
and the mention
that most recently refers to the same referent. In this study, we measure the distance between mentions by counting the number of words between them. The parameter
controls memory decay.
If the referent is new, is estimated in proportion to two terms: (a) a hyperparameter
that controls how likely the speaker is to refer to a new referent and (b) a probability for any particular new referent
that is sampled from the distribution over unseen entities (the term
denotes a total number of unseen entities). The unseen entities here represent entities that the speaker already knows as a part of her world knowledge and that have not yet been introduced into the discourse model.
Cost
In our simulations, the speaker’s cost function is estimated based on word length (number of letters) as in EquationEquation (16)
(16)
(16) . We assume that longer words are more costly to produce.
Note that there are other possible cost functions. Recent work using the RSA framework has shown that word length (longer words are more costly to speak) and word frequency (less frequent words are harder to retrieve) independently contribute to speech cost (Bennett & Goodman, Citation2018). Though it seems reasonable to test speech cost based on word frequency, there is a practical obstacle with respect to speakers’ choices of referring expressions. For example, proper names that are often replaced with pronouns will not appear as often in the corpus because they are being replaced with pronouns. Infrequent uses of these names would be coded as high cost. To avoid this confound, we use only word length as speech cost.
Competitors
The denominator in EquationEquation (10)(10)
(10) represents the sum of potential referents that could be referred to by word
. We assume that a pronoun can refer to a large number of unseen referents if gender and number match but a proper name cannot. For example, “he” could in principle refer to all singular and male referents, including those that have not yet been introduced into the discourse, but “Barack Obama” can only refer to Barack Obama. This assumption is reflected as a probability of unseen referents for the pronoun
as we illustrate below.
Suppose that the speaker is considering using “he” to refer to Barack Obama, which has been previously referred to distance away from the current point in the discourse. There is another singular and male entity, Joe Biden, in the preceding discourse that has been previously referred to
distance away. In this situation, the model computes the probability that the speaker uses “he” to refer to Barack Obama as follows:
where count in the denominator of the last line denotes the number of unseen singular and male entities that could be referred to by he and count
denotes a total number of unseen entities. The term
is the sum of probabilities of unseen referents that could be referred to by the pronoun he. The unseen referents can be interpreted as a penalty for the inexplicitness of pronouns. In the case of proper names, the denominator is always the same as the numerator, under the assumption that each entity has one unique proper name.
In practice, we estimate these numbers of unseen entities from a named entity list in Bergsma and Lin (Citation2006). This named entity list has been created from a large number of online news articles and contains 3,092,611 entities, including 1,489,692 singular-male entities, 616,463 singular-female entities, 699,997 singular-neuter entities, and 286,459 plural entities. We use this list because we will model speakers in news contexts, but the validity of these estimates should be investigated in future studies.
Note that the notion of unseen referents was not incorporated in the original RSA model because the original RSA model has been run in a controlled setting where there are a fixed number of referents and words in a shared context. However, the notion of unseen referents becomes crucial when modeling speakers in a more natural situation because speakers often start a conversation with a new referent in a discourse. The following simulations demonstrate that the knowledge of unseen referents does play a role in distinguishing names and pronouns.
Simulations
Data
We use the SemEval-2010 Task 1 subset of OntoNotes (Recasens et al., Citation2011). The corpus contains 353 documents (total 5,530 sentences; 120,310 words; mean length per document: 340 words) from news wire and broadcast news.Footnote5 The corpus has different annotation layers including part of speech, dependency parse, and coreference that are necessary for simulations in this study. Simulations require coreference chains, grammatical position, part of speech, and agreement information. Coreference, grammatical position, and part of speech were automatically extracted from the corpus. Agreement information was manually annotated as follows.
The coreference chains let us easily count how many times or how recently each referent is mentioned in the discourse, which is necessary for computing discourse salience. We considered only maximally spanning noun phrases as mentions, ignoring nested NPs and nested coreference chains. For example, for the sentence “Both Al Gore and George W. Bush have different ideas on how to spend that extra money” from OntoNotes, the extracted NPs are Both Al Gore and George W. Bush and different ideas about how to spend that extra money. These maximally spanning NPs were automatically extracted from the OntoNotes data.
Dependency tags “SUBJ” (subject), “OBJ” (object), and “PMOD” (oblique object) are used to capture the grammatical position that each proper name occupies. This determines the form of the alternative pronoun that could be used there. For example, the difference between he and him is the grammatical position that each can appear in.
The part of speech is used to identify the form of the referring expression (pronouns and proper names), which is what our model aims to predict. The parts of speech “PRP” (pronoun), “NNP” (proper name), and “NNPS” (plural proper name) were used to extract the target NPs.
The agreement information (gender and number of each referent) is required so that the model can identify all possible competing referents for pronouns. For instance, Barack Obama will be ruled out as a possible competitor for the pronoun she. However, OntoNotes does not have this kind of information. The following describes manual annotation that we have done for this study.
Many mentions (46,246 out of 56,575 mentions in OntoNotes) were automatically annotated using agreement information from the named entity list in Bergsma and Lin (Citation2006), leaving 10,329 to be manually annotated (about 18%). Inter-annotator agreement for the manual annotation of agreement information was 97% (for 500 mentions). The guidelines followed for this manual agreement annotation were largely based on pronoun replacement tests. NPs that referred to a single man and could be replaced with he or him were labeled “male singular,” NPs that could be replaced by it, such as KKR, were labeled “neuter singular,” and so on. NPs that could not be replaced with a pronoun, such as about 30 years earnings for the average peasant, who makes 145 USD a year, were excluded from the analysis.
Filtering data
We selected pronouns and proper names for evaluation according to several criteria. First, the referring expression had to be in a coreference chain that had at least one proper name to facilitate computing the cost of the proper name alternative. Second, pronouns were only included if they were third-person pronouns in subject or object position, and indexicals such as I and you were excluded. After filtering pronouns and proper names according to these criteria, 553 pronouns and 1,332 proper names (total 1,885 items) remained.
We also filtered pronouns whose alternative choice (proper name) would violate syntactic constraints, under the assumption that speakers decide which form to use given the space of possible referring expressions that are provided by the grammar. In particular, we excluded reflexive pronouns such as herself and pronouns whose alternative choices (proper names) would violate Principle C in binding theory (Chomsky, Citation1973, Citation1981; Reinhart, Citation1976). Binding principles determine which forms are available in a certain sentence-internal context. Principle C states that referential expressions such as John and the president must not be c-commandedFootnote6 by their coreferential referent (Chomsky, Citation1981). The bolded names in the following sentences (1) show examples that violate Principle C. For example, in (1a), Mary in the object position is c-commanded by its coreferential Mary in the subject position, so using Mary in that position is banned by Principle C.
(1) a. Maryi likes *Maryi/herselfi.b. Maryi thinks that *Maryi/shei is kind. c. Shei thinks that *Maryi/shei is kind. d. Shei had a cup of coffee while *Maryi/shei was reading the book.
We manually checked pronouns that occur in such positions and did not include them in the evaluation because their alternative, a name, violates Principle C. If the alternative name choice violates Principle C, it would not even be an option for the choice that we aim to formalize here, since this filtering at a syntactic level according to Principle C is likely to be a distinct process from the choice of a form based on discourse information that we are modeling here. After filtering these pronouns, 367 pronouns and 1,332 proper names (total 1,699 items) remained for use in the analysis.
Evaluation measures
Each model chooses referring expressions, pronoun or name, given information extracted from the corpus as described above. For evaluation, we computed AUC (Area Under the Curve) and BIC (Bayesian information criterion). These measures capture different aspects of the results. The following sections describe the measures in turn.
AUC
The model is making a binary choice between pronouns and proper names, and illustrates that different thresholds return different decisions. In this kind of setting, a fair comparison should be to evaluate the model’s performance across all possible thresholds because we do not know what the appropriate threshold is a priori. To evaluate the model’s performance irrespective of what threshold is chosen, we use AUC, area under the ROC curve. The ROC curve is a plot that shows the model’s discrimination performance at all possible thresholds, with the true positive rate (TPR) on the y-axis and the false positive rate (FPR) on x-axis. AUC measures the entire area under the ROC curve and it provides an aggregate measure of the model’s performance across all possible thresholds. A perfect model (all correct) has an AUC of 1.0 and a model that guesses at random would have an AUC of 0.5. AUC has two important properties: (a) it is scale invariant in that only the ordering of scores matters—that is, the absolute value of the score does not change the measure and (b) it is threshold invariant in that it aggregates the model’s performance across all possible thresholds.
BIC
BIC consists of the model log likelihood and the penalty for additional free parameters. The model log likelihood is computed by summing for all pronouns and proper names in the corpus, which measures how likely it is that the model produces the observed words. Higher log likelihood signals a better fit to the data. The BIC penalizes this model likelihood with additional free parameters. A lower BIC score signals a better model. For example, the topicality model is penalized more than the rational model because it has nine free parameters, whereas the rational model has two free parameters.Footnote7
On the one hand, AUC captures the model’s ability to discriminate between forms. It evaluates the ordered outcomes of the model without regard to the absolute likelihood of the outcomes. On the other hand, BIC captures probabilistic aspects of the results. Although it does not assume a deterministic threshold, it does assume a fixed mapping between absolute predicted likelihood of an outcome in the model and production probabilities.
Simulation 1: model comparison
Simulation 1 compares the topicality model and the rational model.Footnote8 summarizes how each model decides which form to use. While the topicality model decides a form based only on topicality of the referent, the rational model decides a form based on informativity (or specificity) of the form and speech cost.
The topicality model uses the maximum likelihood estimates in as a pronoun-choice bias. We chose the best parameter values for the rational model by exploring the following parameter space (optimized for model likelihood): range 0.1 to 10.0 with step 0.1 for the new referent parameter and range 1.0 to 30.0 with step 0.1 for the decay parameter
.
summarizes the results. The rational model performed slightly better than the topicality model on both measures (higher AUC and lower BIC). show the ROC curve for each model. The ROC curve of the topicality model is more angular than that of the rational model because the number of possible thresholds in the topicality model is much lower than in the rational model (the topicality model uses pre-estimated MLEs as in ). Both models perform considerably better than chance, which would correspond to an AUC of 0.5 and a BIC of 2,355.31 (computed as 50–50 coin flips and zero free parameters).
shows the distribution of log likelihoods of names and pronouns computed by each model. The range of log likelihoods of names in both models looks comparable except that the rational model has a longer negative tail. In comparison to the topicality model, the bulk of pronouns’ log-likelihoods in the rational model are concentrated higher with a long negative outlier tail. This suggests that the rational model predicts pronouns with higher probabilities in most cases, but this model also predicts a few pronouns with very low probabilities, resulting in decreasing the model’s log likelihood and thus increasing the BIC.
The topicality model predicts a pronoun with higher probability when its referent occurred in the subject position of the previous sentence. This is a reasonable strategy for predicting pronouns, because about 60% of pronouns in the corpus have their referent mentioned in the subject position of the immediately previous sentence. On the other hand, there are pronouns whose referents are not mentioned in the subject position of the immediately previous sentence. For example, there are pronouns whose referents are mentioned in the non-subject position as in (2). There are also cases in which multiple sentences intervene between the pronoun and its referent, as in (3).
(2) Here’s ABC’s Gillian Findlay. This is how bad it has gotten for Ahmad Al-dour.
Out of work, out of savings, he
is now trying to sell one of the few valuables he has left.
(3) In presenting the study late last week, Warshaw
estimated the cost of these types of disorders to business is substantial. Occupational disability related to anxiety, depression and stress costs about 8,000 USD a case in terms of worker’s compensation. In terms of days lost on the job, the study estimated that each affected employee loses about 16 work days a year because of stress, anxiety or depression. He
added that the cost for stress-related compensation claims is about twice the average for all injury claims.
For these instances, the rational model assigns higher probability to pronouns but the topicality model assigns a higher probability to proper names. On the other hand, when there are many competitors in the preceding discourse, the rational model is less likely to predict a pronoun even when the referent appeared recently, as in (4). The topicality model assigns a higher probability for a pronoun in this case because the most recent referent occurred in the subject position in the previous sentence.
(4) (there are 22 third singular male competitors in the preceding discourse) Mike Huber,
a roustabout,
is even making it in his new career as an entrepreneur. He
started Arrow Roustabouts inc. a year ago with a loan from a friend, since repaid, and now employs 15. He
got three trucks and a backhoe cheap.
In sum, Simulation 1 showed that the two models performed comparably, with slightly better AUC and BIC in the rational model. The qualitative analysis suggests that two models capture different aspects of the speakers’ choices of reference forms. However, given that the rational model contains several components, including specificity and cost, it remains unclear exactly which component contributes to predict speakers’ behavior. Our next simulation conducts an ablation test to examine the contribution of each component in the rational model.
Simulation 2: testing the contribution of components in the rational model
To quantify the contribution of each component in the rational model, Simulation 2 contrasts the rational model in Simulation 1 with three impoverished models that each lack one of the following components: referential predictability, unseen competitors, and speech cost.
Here we refer to the rational model in Simulation 1 as the Complete model. The model without referential predictability, -Predictability, uses a uniform distribution: All referents in the preceding discourse have equal predictability. This model assigns the same probability to all old referents. For a probability of a new or unseen referent, it uses the same estimate of predictability as the Complete model. The model without good estimates of unseen competitors, -Unseen, does not have estimates of unseen referents like the Complete model does, and it always assigns probability to unseen referents in the denominator of Equation (10), regardless of whether the word is a proper name or pronoun. In other words, the representation of unseen competitors in the -Unseen model is poorer than in the Complete model. The comparison model without cost, -Cost, uses constant speech cost. This model assigns the same cost value across pronouns and proper names. Since the informativity term in the Complete model always prefers names to pronouns (because names are more specific), this model always predicts names when evaluated against an absolute threshold of 0.5, but it still assigns a non-zero probability to pronouns.
summarizes the results of each model. The Complete model achieved the best BIC and was comparable to the -Cost model in AUC. The comparison between the Complete model and the -Predictability model suggests that it is important to incorporate updated referential predictability to speakers’ listeners’ beliefs as discourse proceeds. The comparison between the Complete model and the -Unseen model suggests that it is important to incorporate estimates of unseen competitors (e.g., “he” can potentially refer to many singular and masculine entities, but “Obama” cannot).
The -Cost model was slightly better than the Complete model in the AUC, and its AUC and BIC were considerably better than the -Predictability model and the -Unseen model. The high AUC in the -Cost model is due to the fact that although it always gives a higher probability to proper names (which are more informative), the AUC metric is not sensitive to an absolute threshold of 0.5. Instead, it integrates over all thresholds. The speech cost estimated by word length in this simulation roughly corresponds to a constant penalty for proper names (i.e., the lengths of names are normally longer than pronouns). Thus, including or omitting the cost does not substantially change the value of AUC, because only ordering of the scores matters in this metric. On the other hand, BIC is based on likelihood, which is sensitive to absolute scores. It captures how well the model fits the observed data if speakers translate the scores that the model produces directly into production probabilities. When using absolute scores, the Complete model best predicted the speakers’ word choices.
These results suggest that with a flexible decision threshold, a speech cost that penalizes pronouns less does not considerably help predict speakers’ choices between names and pronouns, but the components that affect the computation of informativity of the word—namely, referential predictability and estimates of unseen competitors—do play an important role regardless of threshold. Together with the results of Simulation 1, these results suggest that the topicality of the referent and informativity of the word (which incorporates referential predictability) are both important to consider in the problems of speakers’ choices of reference forms. On the other hand, we did not find strong evidence that supports the role of a speech cost metric that prefers shorter forms.
General discussion
This study formalized and compared two major models in speakers’ choices of referring expressions: the topicality model, which chooses a form based on the topicality of the referent, and the rational model, which chooses a form based on the informativity of the form and its speech cost. In deriving the rational model from the original RSA model, we also showed that predictions previously attributed to the Uniform Information Density hypothesis in this domain can be derived from the rational model in a fully explicit manner.
Simulations tested to what extent each model captures the choice between names and pronouns. Simulation 1 showed that despite the simple estimates of topicality and referential predictability, both models reasonably predicted the choices between names and pronouns. These two models were comparable in AUC and BIC metrics, while each model captures different aspects of speakers’ choices of names and pronouns. Simulation 2 identified which model components in the rational model help predict speakers’ choices between names and pronouns. Simulations showed that speech cost that prefers a shorter form (thus pronouns) did not play a prominent role relative to the other model components that are used to compute the informativity of the word—namely, referential predictability and knowledge of unseen competitors. These results together suggest that both topicality of the referent and informativity of the word are important to consider with respect to speakers’ choices of reference forms, while speech cost may not be.
These results have two important implications. First, unlike previous studies (Kehler et al., Citation2008; Rohde & Kehler, Citation2014) have suggested, the topicality of the referent may not be the only factor that determines speakers’ choices of reference forms. This is in line with previous experiments that show that verb-based predictability affects reference production with different types of verbs (Rosa & Arnold, Citation2017; Zerkle & Arnold, Citation2016) or a different experimental setting (Weatherford & Arnold, Citation2020). Second, simple speech cost that prefers shorter forms may not be relevant to speakers’ choices of reference forms. As we discuss below, there are several possibilities for exploring other types of cost metrics.
Our simulation results suggest at least two possibilities about the role of speech cost. First, speakers’ choices of referring expressions might not depend on speech cost, as several experiments have suggested (Arnold & Zerkle, Citation2019). The topicality model instantiates this idea in that it does not include a term for speech cost. On the other hand, the idea of speech cost is crucial in information theoretic accounts because this kind of theory predicts that there is a trade-off between word’s informativity and speech cost: Speakers use a shorter/easier form when the referent is informative (M. Frank & Goodman, Citation2012; Levy & Jaeger, Citation2007; Tily & Piantadosi, Citation2009). This line of hypothesis has the advantage of generality, in that RSA models account for various kinds of speakers’ behavior (e.g., Goodman & Frank, Citation2016). If speakers’ choices of referring expressions do not depend on speech cost, then the question arises as to why this phenomenon is special despite the fact that the theory generalizes other kinds of word choices. Alternatively, the kind of speech cost employed in this study—that is, shorter being less costly—may not be appropriate for speakers’ choices of reference forms, and other types of cost might be more relevant. For example, in the RSA framework, competitors are considered when computing informativity of the word (e.g., the denominator in EquationEquations (7)(7)
(7) and (Equation10
(10)
(10) )). However, this computation may require an additional cost in the speaker’s mind because representing and using multiple referents in the mental discourse representation would consume more attentional resources (Arnold & Griffin, Citation2007). Thus, choosing a pronoun would incur more cost because a pronoun originally has more potential referents than a name. This kind of cost could be more complex than the cost estimated using word length because it requires computation of the potential referents, not the form. In this scenario, pronouns would be less likely to be chosen in both on the cost and on the informativity. If this is the case, the model would never predict that pronouns would be chosen, at least on an absolute threshold basis, but in reality they sometimes are. It remains to be investigated what kind of cost in the speaker’s mind, if any, affects the choices of reference forms.
Both models presuppose a threshold value at which a decision would be made to use a particular form given the relative value of possible forms. We used AUC for the evaluation measure to compute an aggregated value of outcomes given all possible thresholds. However, it is not clear what a psychological correspondence of this kind of threshold is. One possibility is that the threshold value might differ among speakers, styles, or contexts. Previous experiments have shown that there is a great variation in speakers’ uses of pronouns within a fixed discourse context (Zerkle & Arnold, Citation2016). Multiple factors would influence this individual variation, such as working-memory capacity (Hendriks, Citation2016), and we speculate that the variation in decision threshold would be one factor that results in the observed individual differences. In an extreme view, the threshold value could be one of lexical features of a reference form.
We tested the models with a news corpus, which involves heavily edited texts compared with other styles such as spontaneous speech. The replicability of our results in different kinds of texts or speech would depend on whether and to what extent we could incorporate nonlinguistic information, such as visual information and shared background knowledge, which may play a crucial role in reference production (Clark & Marshall, Citation1981; Fukumura et al., Citation2010; Horton & Keysar, Citation1996; Vogels et al., Citation2013b). Interlocutors in spontaneous speech are essentially different from the readers/audience of the news texts in that they tend to share more common ground. Furthermore, while the current simulations with news texts do not incorporate visual information, some referents would be visually available in other types of contexts. If that is the case, previous mentions would not be as effective for estimating salience, because a person or object that exists in front of the speaker could also be salient and, thus, more likely to be referred to by a pronoun. To investigate these possibilities, we would need a more sophisticated discourse model along with a corpus that contains annotations of such information.
The other important aspect of speakers’ form choice is whether and to what extent speakers take the listener’s perspective into account (e.g., Bard & Aylett, Citation2005; Barr & Keysar, Citation2006; Clark & Murphy, Citation1982; Dell & Brown, Citation1991; Ferreira & Dell, Citation2000; Gerrig et al., Citation2000; Pate & Goldwater, Citation2015; Pickering & Garrod, Citation2004). Many discourse theories have assumed that speakers consider a listener’s discourse model when they choose referring expressions (Ariel, Citation1990; Chafe, Citation1976; Givón, Citation1983; Gundel et al., Citation1993). This kind of form selection driven by audience design has also been assumed in the information theoretic approaches: Speakers choose words to optimize informativeness to their listeners (M. Frank & Goodman, Citation2012; Levy & Jaeger, Citation2007; Pate & Goldwater, Citation2015). On the other hand, some experimental studies have demonstrated that speakers choose referring expressions without considering how salient the referent is to their listeners (Bard & Aylett, Citation2005; Fukumura & van Gompel, Citation2012; Horton & Keysar, Citation1996), suggesting that speakers’ ability to adopt or take the listener’s perspective into account may be limited in its extent and consistency. While it was not possible to determine from this study whether speakers are using their own discourse model versus a listener’s discourse model, this could be possible to test in the future using parallel data sets that specifically manipulate the degree to which the discourse context is shared between speakers and listeners.
In previous research on each model, the experimental settings have been homogeneous and controlled (e.g., M. Frank & Goodman, Citation2012; Rohde & Kehler, Citation2014).Footnote9 In contrast, the current corpus has various uses of pronouns and proper names with respect to predicate types, sentence structures, discourse, and types of referents. Despite these complexities, simulations show that both models capture the natural uses of referring expressions to some extent. In particular, we showed that both topicality of the referent and informativity of the word are important to consider in speakers’ choices of reference forms but speech cost that prefers shorter forms may not play a crucial role. Simulations with more-realistic estimates of various factors will provide conclusive and more detailed evidence.
Conclusion
This study formalized and compared two major models in speakers’ choices of referring expressions: the topicality model, which chooses a form based on the topicality of the referent, and the rational model, which chooses a form based on the informativity of the form and its speech cost. We showed that despite using simple estimates of topicality and referential predictability, both models reasonably predicted the choice between names and pronouns and each model captured different aspects of speakers’ behavior. Simulations also suggest that both topicality of the referent and informativity of the word are important to consider in the problems of referential production, while we did not find strong evidence that supports the role of a speech cost that prefers shorter forms.
Acknowledgements
We thank Jennifer Arnold, Hal Daumé III, Noah Goodman, Roger Levy, three anonymous reviewers, and the UMD probabilistic modeling reading group for helpful comments and discussion. A previous version of this work was included in the Proceedings of the 53rd Annual Meeting of the Association for Computational Linguistics.
Disclosure statement
No potential conflict of interest was reported by the authors.
Notes
1. Incorporating various discourse factors into a model may empirically affect the simulation results. Building a more realistic discourse model will be an important step toward capturing actual speakers.
2. Though the correlation between topicality and subjecthood is strong, nonsubject positions can be a place for topic. Rohde and Kehler (Citation2014) manipulated the topicality of referents while keeping grammatical role constant and showed that pronoun production is influenced by the topicality, but not the subject position.
3. The unit is not a clause but a sentence, as it is originally specified in the corpus, thus there are some sentences containing multiple clauses. In these cases, we counted the grammatical position of the most recent occurrence of the referent.
4. Note, however, that the topicality model is actually more complex than the rational model in terms of free parameters.
5. The corpus consists of a development set (39 documents), a training set (229 documents), and a test set (85 documents). Since our simulations do not require such division of the data set, we use all documents together.
6. C-command is a relationship between nodes in a hierarchical tree structure: c-commands
when
is contained in the sister node of its antecedent
.
7. For example, we compute the BIC score of the rational model as follows:
8. The code is available at https://osf.io/g7npy/
9. Recent RSA models have started incorporating estimates such as cost and frequency from naturally distributed data (Graf et al., Citation2016; Monroe et al., Citation2017).
References
- Almor, A. (1999). Noun-phrase anaphora and focus: The informational load hypothesis. Psychological Review, 106(4), 748. https://doi.org/10.1037/0033-295X.106.4.748
- Ariel, M. (1990). Accessing noun-phrase antecedents. Routledge.
- Arnold, J. (1998). Reference form and discourse patterns [Unpublished doctoral dissertation]. Stanford University, Stanford.
- Arnold, J. (2016). Explicit and emergent mechanisms of information status. Topics in Cognitive Science, 8(4), 737–760. https://doi.org/10.1111/tops.12220
- Arnold, J., & Griffin, Z. (2007). The effect of additional characters on choice of referring expression: Everyone counts. Journal of Memory and Language, 56(4), 521–536. https://doi.org/10.1016/j.jml.2006.09.007
- Arnold, J., & Zerkle, S. (2019). Why do people produce pronouns? Pragmatic selection vs. rational models. Language, Cognition and Neuroscience, 34(9), 1152–1175. https://doi.org/10.1080/23273798.2019.1636103
- Aylett, M., & Turk, A. (2004). The smooth signal redundancy hypothesis: A functional explanation for relationships between redundancy, prosodic prominence, and duration in spontaneous speech. Language and Speech, 47(1), 31–56. https://doi.org/10.1177/00238309040470010201
- Aylett, M., & Turk, A. (2006). Language redundancy predicts syllabic duration and the spectral characteristics of vocalic syllable nuclei. The Journal of the Acoustical Society of America, 119(5), 3048–3058. https://doi.org/10.1121/1.2188331
- Bard, E. G., & Aylett, M. (2005). Referential form, duration, and modelling the listener in spoken dialogue. In J. C. Trueswell & M. K. Tanenhaus (Eds.), Approaches to studying world-situated language use: Bridging the language-as-product and language-as-action traditions (pp. 173–191). MIT Press.
- Barr, D. J., & Keysar, B. (2006). Perspective taking and the coordination of meaning in language use. In M. J. Traxler & M. A. Gernsbacher (Eds.), Handbook of Psycholinguistics (2nd ed. pp. 901–938). Academic Press. https://doi.org/10.1016/B978-012369374-7/50024-9
- Bell, A., Jurafsky, D., Fosler-Lussier, E., Girand, C., Gregory, M., & Gildea, D. (2003). Effects of disfluencies, predictability, and utterance position on word form variation in English conversation. The Journal of the Acoustical Society of America, 113(2), 1001–1024. https://doi.org/10.1121/1.1534836
- Bennett, E., & Goodman, N. (2018). Extremely costly intensifiers are stronger than quite costly ones. Cognition, 178, 147–161. https://doi.org/10.1016/j.cognition.2018.05.011
- Bergsma, S., & Lin, D. (2006). Bootstrapping path-based pronoun resolution. In Proceedings of the 21st international conference on computational linguistics and 44th annual meeting of the association for computational linguistics (pp. 33–40). Association for Computational Linguistics.
- Blei, D. M., & Frazier, P. I. (2011). Distance dependent chinese restaurant processes. The Journal of Machine Learning Research, 12(74), 2461–2488.
- Bock, J. K., & Warren, R. K. (1985). Conceptual accessibility and syntactic structure in sentence formulation. Cognition, 21(1), 47–67. https://doi.org/10.1016/0010-0277(85)90023-X
- Broadbent, D. E. (1973). In defence of empirical psychology. Methuen.
- Callaway, C. B., & Lester, J. C. (2002). Pronominalization in generated discourse and dialogue. In Proceedings of the 40th annual meeting on association for computational linguistics (pp. 88–95).
- Chafe, W. (1974). Language and consciousness. Language, 50(1), 111–133. https://doi.org/10.2307/412014
- Chafe, W. (1976). Givenness, contrastiveness, definiteness, subjects, topics, and point of view in subject and topic. In C.N. Li (Ed.), Subject and topic (pp. 25–56). Academic Press.
- Chafe, W. (1994). Discourse, consciousness, and time: The flow and displacement of conscious experience in speaking and writing. University of Chicago Press.
- Chomsky, N. (1973). Conditions on Transformations. In S. R. Anderson & P. Kiparsky (Eds.), A festschrift for Morris Halle (pp. 232–286). Holt, Rinehart & Winston.
- Chomsky, N. (1981). Lectures on government and binding. Dordrecht.
- Clancy, P. M. (1980). Referential choice in English and Japanese narrative discourse. In W. Chafe (Ed.), The pear stories: Cognitive, cultural, and linguistic aspects of narrative production (Vol. 3, pp. 127–201). Norwood, NJ: Ablex.
- Clark, H. H., & Marshall, C. R. (1981). Definite reference and mutual knowledge. In A. K. Joshi, B. Webber, & I. Sag (Eds.), Elements of discourse understanding (pp. 10–63). Cambridge University Press.
- Clark, H. H., & Murphy, G. L. (1982). Audience design in meaning and reference. In J. F. Le Ny & W. Kintsch (Eds.), Language and comprehension (pp. 287–299). North Holland.
- Dale, R., & Reiter, E. (1995). Computational Interpretations of the Gricean Maxims in the Generation of Referring Expressions. Cognitive Science, 19(2), 233–263. https://doi.org/10.1207/s15516709cog1902_3
- Dell, G. S., & Brown, P. M. (1991). Mechanisms for listener-adaptation in language production: Limiting the role of the “model of the listener”. In D. Napoli & J. Kegl (Eds.), Bridges between psychology and linguistics (pp. 105–129). Academic Press.
- Ferreira, V. S., & Dell, G. S. (2000). Effect of ambiguity and lexical availability on syntactic and lexical production. Cognitive Psychology, 40(4), 296–340. https://doi.org/10.1006/cogp.1999.0730
- Fletcher, C. R. (1984). Markedness and topic continuity in discourse processing. Journal of Verbal Learning and Verbal Behavior, 23(4), 487–493. https://doi.org/10.1016/S0022-5371(84)90309-8
- Foraker, S., & McElree, B. (2007). The role of prominence in pronoun resolution: Active versus passive representations. Journal of Memory and Language, 56(3), 357–383. https://doi.org/10.1016/j.jml.2006.07.004
- Frank, A., & Jaeger, T. F. (2008). Speaking rationally: Uniform information density as an optimal strategy for language production. In Proceedings of the 30th annual meeting of the cognitive science society (pp. 933–938).
- Frank, M., & Goodman, N. (2012). Predicting pragmatic reasoning in language games. Science, 336(6084), 998–998. https://doi.org/10.1126/science.1218633
- Fukumura, K., & van Gompel, R. P. (2010). Choosing anaphoric expressions: Do people take into account likelihood of reference? Journal of Memory and Language, 62(1), 52–66. https://doi.org/10.1016/j.jml.2009.09.001
- Fukumura, K., & van Gompel, R. P. (2011). The effect of animacy on the choice of referring expression. Language and Cognitive Processes, 26(10), 1472–1504. https://doi.org/10.1080/01690965.2010.506444
- Fukumura, K., & van Gompel, R. P. (2012). Producing pronouns and definite noun phrases: Do speakers use the addressee’s discourse model? Cognitive Science, 36(7), 1289–1311. https://doi.org/10.1111/j.1551-6709.2012.01255.x
- Fukumura, K., van Gompel, R. P., & Pickering, M. J. (2010). The use of visual context during the production of referring expressions. The Quarterly Journal of Experimental Psychology, 63(9), 1700–1715. https://doi.org/10.1080/17470210903490969
- Garvey, C., & Caramazza, A. (1974). Implicit causality in verbs. Linguistic Inquiry 5(3), 459–464. https://www.jstor.org/stable/4177835
- Gatt, A., Krahmer, E., van Deemter, K., & van Gompel, R. P. (2014). Models and empirical data for the production of referring expressions. Language, Cognition and Neuroscience, 29(8), 899–911. https://doi.org/10.1080/23273798.2014.933242
- Gerrig, R. J., Brennan, S. E., & Ohaeri, J. O. (2000). What can we conclude from speakers behaving badly? Discourse Processes, 29(2), 173–178. https://doi.org/10.1207/S15326950dp2902_5
- Givón, T. (1983). Topic continuity in discourse: A quantitative cross-language study (Vol. 3). John Benjamins.
- Givón, T. (1990). Syntax: A functional-typological introduction (Vol. II). John Benjamins.
- Goodman, N., & Frank, M. (2016). Pragmatic language interpretation as probabilistic inference. Trends in Cognitive Sciences, 20(11), 818–829. https://doi.org/10.1016/j.tics.2016.08.005
- Graf, C., Degen, J., Hawkins, R., & Goodman, N. (2016). Animal, dog, or dalmatian? Level of abstraction in nominal referring expressions. In Proceedings of the 38th annual conference of the cognitive science society (pp. 2261–2266).
- Grice, H. (1975). Logic and conversation. In P. Cole & J. L. Morgan (Eds.), Syntax and Semantics, Vol. 3, Speech Acts (pp. 41–58). Academic Press.
- Grosz, B. J., & Sidner, C. L. (1986). Attention, intentions, and the structure of discourse. Computational Linguistics, 12(3), 175–204.
- Grosz, B. J., Weinstein, S., & Joshi, A. K. (1995). Centering: A framework for modeling the local coherence of discourse. Computational Linguistics, 21(2), 203–225. https://www.aclweb.org/anthology/J95-2003/
- Gundel, J. K., Hedberg, N., & Zacharski, R. (1993). Cognitive status and the form of referring expressions in discourse. Language, 69(2), 274–307. https://doi.org/10.2307/416535
- Haghighi, A., & Klein, D. (2010). Coreference resolution in a modular, entity-centered model. In Human language technologies: The 2010 annual conference of the north american chapter of the association for computational linguistics (pp. 385–393). https://dl.acm.org/doi/10.5555/1857999.1858060
- Hendriks, P. (2016). Cognitive modeling of individual variation in reference production and comprehension. Frontiers in Psychology, 7, 506. https://doi.org/10.3389/fpsyg.2016.00506
- Horton, W. S., & Keysar, B. (1996). When do speakers take into account common ground? Cognition, 59(1), 91–117. https://doi.org/10.1016/0010-0277(96)81418-1
- Jaeger, F. T. (2010). Redundancy and reduction: Speakers manage syntactic information density. Cognitive Psychology, 61(1), 23–62. https://doi.org/10.1016/j.cogpsych.2010.02.002
- Jager, G. (2007). Game dynamics connects semantics and pragmatics. In A-V. Pietarinen (Ed.), Game theory and linguistic meaning (pp. 89–102). Elsevier.
- Kehler, A., Kertz, L., Rohde, H., & Elman, J. L. (2008). Coherence and coreference revisited. Journal of Semantics, 25(1), 1–44. https://doi.org/10.1093/jos/ffm018
- Kehler, A., & Rohde, H. (2013). A probabilistic reconciliation of coherence-driven and centering-driven theories of pronoun interpretation. Theoretical Linguistics, 39(1–2), 1–37. https://doi.org/10.1515/tl-2013-0001
- Kibble, R., & Power, R. (2004). Optimizing referential coherence in text generation. Computational Linguistics, 30(4), 401–416. https://doi.org/10.1162/0891201042544893
- Krahmer, E., & Van Deemter, K. (2012). Computational generation of referring expressions: A survey. Computational Linguistics, 38(1), 173–218. https://doi.org/10.1162/COLI_a_00088
- Kuno, S. (1972). Functional sentence perspective: A case study from Japanese and English. Linguistic Inquiry, 3(3), 269–320.
- Levy, R., & Jaeger, T. F. (2007). Speakers optimize information density through syntactic reduction. In Proceedings of the 20th conference on neural information processing systems (nips).
- Mahowald, K., Fedorenko, E., Piantadosi, S. T., & Gibson, E. (2013). Info/information theory: Speakers choose shorter words in predictive contexts. Cognition, 126(2), 313–318. https://doi.org/10.1016/j.cognition.2012.09.010
- Monroe, W., Hawkins, R., Goodman, N., & Potts, C. (2017). Colors in context: A pragmatic neural model for grounded language understanding. Transactions of the Association for Computational Linguistics, 5, 325–338. https://doi.org/10.1162/tacl_a_00064
- Orita, N., Vornov, E., Feldman, N., & Boyd-Graber, J. (2014). Quantifying the role of discourse topicality in speakers’ choices of referring expressions. In Association for computational linguistics, workshop on cognitive modeling and computational linguistics. https://www.aclweb.org/anthology/W14-2008
- Pate, J. K., & Goldwater, S. (2015). Talkers account for listener and channel characteristics to communicate efficiently. Journal of Memory and Language, 78, 1–17. https://doi.org/10.1016/j.jml.2014.10.003
- Pickering, M. J., & Garrod, S. (2004). Toward a mechanistic psychology of dialogue. Behavioral and Brain Sciences, 27(2), 169–190. https://doi.org/10.1017/S0140525X04000056
- Poesio, M., Stevenson, R., Di Eugenio, B., & Hitzeman, J. (2004). Centering: A parametric theory and its instantiations. Computational Linguistics, 30(3), 309–363. https://doi.org/10.1162/0891201041850911
- Prince, E. F. (1981). Toward a taxonomy of given-new information. In P. Cole (Ed.), Radical pragmatics (pp. 223-233). Academic Press.
- Recasens, M., Marquez, L., Sapena, E., Martí, M. A., & Taulé, M. (2011). SemEval-2010 task 1 OntoNotes English: Coreference resolution in multiple languages. LDC2011T01. Web Download. Philadelphia: Linguistic Data Consortium. https://doi.org/10.35111/bmpd-n944
- Reinhart, T. (1976). The syntactic domain of anaphora [Unpublished doctoral dissertation]. MIT.
- Reinhart, T. (1981). Pragmatics and linguistics: An analysis of sentence topics. Philosophica, 27(1), 53–94.
- Reiter, E., Dale, R., & Feng, Z. (2000). Building natural language generation systems. MIT Press.
- Rohde, H., & Kehler, A. (2014). Grammatical and information-structural influences on pronoun production. Language, Cognition and Neuroscience, 29(8), 912–927. https://doi.org/10.1080/01690965.2013.854918
- Rosa, E., & Arnold, J. (2017). Predictability affects production: Thematic roles can affect reference form selection. Journal of Memory and Language, 94, 43–60. https://doi.org/10.1016/j.jml.2016.07.007
- Sanford, A. J., & Garrod, S. C. (1981). Understanding written language: Explorations of comprehension beyond the sentence. Wiley.
- Shannon, C. (1948). A mathematical theory of communications. Bell Systems Technical Journal, 27(4), 623–656. https://doi.org/10.1002/j.1538-7305.1948.tb00917.x
- Stevenson, R. J., Crawley, R. A., & Kleinman, D. (1994). Thematic roles, focus and the representation of events. Language and Cognitive Processes, 9(4), 519–548. https://doi.org/10.1080/01690969408402130
- Tily, H., & Piantadosi, S. (2009). Refer efficiently: Use less informative expressions for more predictable meanings. In Proceedings of the workshop on the production of referring expressions: Bridging the gap between computational and empirical approaches to reference.
- Van Deemter, K., Gatt, A., van Gompel, R. P., & Krahmer, E. (2012). Toward a computational psycholinguistics of reference production. Topics in Cognitive Science, 4(2), 166–183. https://doi.org/10.1111/j.1756-8765.2012.01187.x
- Van Son, R. J., & Van Santen, J. P. (2005). Duration and spectral balance of intervocalic consonants: A case for efficient communication. Speech Communication, 47(1), 100–123. https://doi.org/10.1016/j.specom.2005.06.005
- Vogels, J., Krahmer, E., & Maes, A. (2013a). When a stone tries to climb up a slope: The interplay between lexical and perceptual animacy in referential choices. Frontiers in Psychology, 4, 154. https://doi.org/10.3389/fpsyg.2013.00154
- Vogels, J., Krahmer, E., & Maes, A. (2013b). Who is where referred to how, and why? the influence of visual saliency on referent accessibility in spoken language production. Language and Cognitive Processes, 28(9), 1323–1349. https://doi.org/10.1080/01690965.2012.682072
- Weatherford, K., & Arnold, J. (2020). Semantic predictability of implicit causes affects referential form choice [ Poster presentation]. Cuny conference on human sentence processing, Amherst.
- Zerkle, S., & Arnold, J. (2016). Discourse attention during utterance planning affects referential form choice. Linguistics Vanguard, 2(s1). https://doi.org/10.1515/lingvan-2016-0067
Appendix A.
Derivation of EquationEquation 12
(12)
(12)
We define the rational speaker model as follows:
where the first term in the numerator, , is a word probability: The listener in the speaker’s mind guesses how likely the speaker would be to use
to refer to
. The second term in the numerator,
, is the discourse salience of referent
. The denominator
is a sum of potential referents
that could be referred to by word
.
Suppose that there are words to refer to referent
. The speaker’s probability of choosing word
to refer to
is
Plugging Equation (A1) into Equation (A2), we have
Assuming that is constant across words and referents and that
is zero for all incompatible referents, Equation (A3) can be reduced to
where in Equation (A4) denotes all referents that are compatible with word
, as opposed to denoting all possible referents as in Equation (A3). Because
is independent from the selection of a particular word, Equation (A4) can then be reduced to
Table 2. Simplified (unnormalized) representation of each model: denotes a referent and
denotes a word
Table 3. Simulation 1 results
Table 4. Simulation 2 results