ABSTRACT
Multi-joint movement is realized by controlling the nonlinear dynamics induced by the mass of each link. Collaborative link-inertia action towards a goal achieves energy-efficient motion, such as inertial movement. Inertial movement-based motion analysis is beneficial but challenging when walking is considered, owing to the alterations in the nonlinear equations of motion, which correspond to the holonomic constraints between the foot and ground. In this study, we clarified that the shortest curve between two points on holonomically constrained Riemannian manifolds corresponds to the constrained inertial movement of a multi-joint system, and that every constrained inertial movement can be consistently considered by the shortest-curve lengths (Riemannian distances). Accordingly, by comparing the curve length of the measured motion with the Riemannian distance, we developed a quantitative analysis method for measuring the proximity of a measured walk to the inertial movement. This was applied to healthy persons walking under different inertial cadences and load conditions. The results indicate the proximity to the inertial movement during the swing phase under all conditions, including the similarity between the proximity profiles in normal and high-inertia walks. These findings validate the introduction of a Riemannian distance-based evaluation quantity in the motion analysis and control of multi-joint systems.
GRAPHICAL ABSTRACT
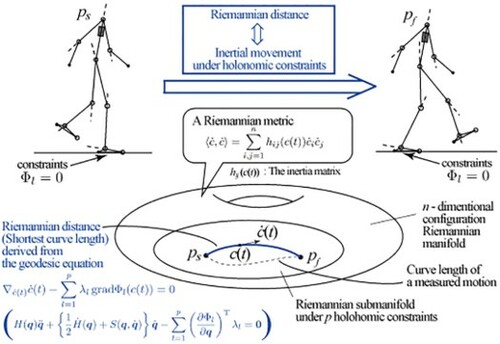
Acknowledgments
The authors thank I. Tsuchikura and S. Takashima for their help with the experiments. The authors also thank the anonymous reviewers and the associate editor for their helpful suggestions for improving the manuscript.
Disclosure statement
No potential conflict of interest was reported by the author(s).
Additional information
Funding
Notes on contributors
Masahiro Sekimoto
Masahiro Sekimoto received his B.S., M.S., and Ph.D. degrees from Ritsumeikan University, Japan, in 2003, 2005, and 2007, respectively. He was a Postdoctoral Fellow of Ritsumeikan University from 2007 to 2010. In 2010 he joined University of Toyama, where he is a full-time lecturer. His research interests include dynamics analysis and control design of multi-joint robots and human movements. He is a member of robotics related academic societies: IEEE, RSJ, JSME, and SICE. He was awarded the RSJ Best Paper Award in 2007 and the SICE Research Encouragement Award in 2009.
Suguru Arimoto
Suguru Arimoto received B.S. degree from Kyoto University in 1959 and Dr. Eng. degree from the University of Tokyo in 1967. He was an Associate Professor of Osaka University during 1968–1973, a Professor during 1973–1990, a Professor of the University of Tokyo during 1988–1997, a Professor of Ritsumeikan University during 1997–2007, and a Guest Professor during 2007–2014. He is an Emeritus Professor of Osaka University. He is an IEEE Fellow (1983), an IEICE Fellow (2000), an RSJ Fellow (2003), a JSME Fellow (2005), and an IEEE Life Fellow (2007). He was awarded the Royal Medal with Purple Ribbon from the Japanese Government in 2000, the IEEE 3rd Millennium Memorial Medal in 2000, the Pioneer Award from the IEEE R&A Society in 2006, and the 2007 Rufus Oldenburger Medal from the ASME.