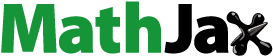
SYNOPTIC ABSTRACT
This article opens up new aging classes and new stochastic orders dependant on generalized moment-generating function, which plays a vital role in reliability theory, finance topics, stochastic orders, and economic theory. The article presents some important new implications concerning these classes and introduces characterizations of the Weibull distribution, exponential distribution, and Erlang distribution through newly suggested aging classes. In addition, we list a series of inequalities that provide bounds for generalized moment-generating classes. Furthermore, a sufficient condition for allowing a probability distribution to have a new class is provided. The article also demonstrates the preservation properties of a new stochastic order under certain reliability operations, such as mixture and convolution. Moreover, some novel applications of classes and stochastic orders in random sum and shock models are provided.
1. Introduction and Motivation
Consider the probability density function for a lifetime random variable X1 with the distribution function
survival function
and hazard rate function
In addition, we assume that the mean life
and the variance
are finite. Likewise, let X2 be the second lifetime random variable with the density function
, distribution function
, survival function
, and hazard rate function
. Furthermore, both the mean life
and variance
are assumed to be finite. Let
and
. Let
has a reversed hazard rate function
(
). The moment-generating functions for all
are defined as
Indeed, moment-generating class and stochastic ordering have received considerable attention in the literature; see ArdiÇ, Akdemir, and Özdemir (Citation2018), Zamani, Mohtashami Borzadaran, and Amini (Citation2017), Kayid, Izadkhah, and Alshami (Citation2016), Rajan and Tepedelenlioğlu (Citation2015), Gandotra, Gupta, and Bajaj (Citation2014), Mahmoud, Moshref, and Gadallah (Citation2014), Lee and Tepedelenlioglu (Citation2014), Rajan (Citation2014), Anis (Citation2011), Elbatal (Citation2007), Ahmed and Kayid (Citation2004), Belzunce, Ortega, and Ruiz (Citation2004), and Klar and Müller (Citation2003).
Recently, Michael, Anene, Akudo, and Nwabueze (Citation2017) defined the generalized moment-generating function as,
Now, we can define the generalized Laplace transform function as,
(1)
(1)
(2)
(2)
Let us observe that,
(3)
(3)
For any life variable , the residual life variable
, where
and
is a nonnegative random variable representing the remaining life of X at age α. Hence, if
is the distribution function of X and
is its survival function, the survival function of
is thus given by,
In realistic situations, the random variables are not necessarily related only to the future; rather, they can also refer to the past. This random variable may be referred to in the literature as inactivity time or the time elapsed after failure until time
, given that the unit has already failed by time α. It is also called reverse residual life, or time since failure, where
for fixed
, having the distribution function
The reliability function here can be represented as,
Let and
be residual lives at age α of the random variables X1 and X2, respectively, and let
and
be reversed residual lives at age
of the random variables X1 and X2, respectively.
Definition 1.
(Shaked and Shanthikumar, Citation2007, Kayid and Alamoudi, Citation2013, and Ali, Citation2018). X1 is smaller than or equal to X2 in the following cases.
The moment-generating function ordering (
), if
for all
or
and
for all
The moment-generating function ordering of residual lives (
), if
for all
The Laplace transform function ordering of residual lives (
), if
for all
The increasing convex (concave) ordering (
), if
for all increasing convex (concave) functions
and provided the expectations existed.
The exponential of inactivity time ordering (
), if
for all
The hazard rate ordering (
), if
for all
or
for all
The reversed hazard rate ordering (
), if
for all
or
for all
Now, we can define the generalized moment-generating (GL) function order as follows.
Definition 2.
Let X1 and X2 be two non-negative and absolutely continuous random variables, with the distribution functions and
, and the density functions
and
, respectively. Hence, X1 is smaller than or equal to X2 in generalized moment-generating ordering, denoted by
if,
(4)
(4)
for all
In addition, we can define the generalized moment-generating function class of residual (reversed residual) lives as follows.
Definition 3.
Let X1 be a non-negative and absolutely continuous random variables, with the distribution function , the survival function
, and the density function f1
. Hence,
The generalized moment-generating function of residual lives, for all
is defined as,
(5)
(5)
The generalized moment-generating function of reversed residual lives, for all
is defined as,
(6)
(6)
Next, we consider new aging classes following previous procedures for the generalized moment-generating function class of residual (reversed residual) lives.
Definition 4.
X is said to have,
Increasing (decreasing) the generalized moment generation of residual lives class (
if,
(7)
(7)
Increasing (decreasing) the generalized moment generation of reversed residual lives class (
if,
(8)
(8)
Definition 5.
Let U and V be two non-negative and absolutely continuous random variables, with the distribution functions and
, density functions f
and
, generalized moment generation of residual lifetime functions
and
, for t > 0, and generalized moment generation of reversed residual lifetime functions
and
, for t > 0, respectively. Hence,
U is smaller than or equal to V in the generalized moment generation of residual lives ordering, denoted by
if,
(9)
(9)
U is smaller than or equal to V in the generalized moment generation of reversed residual lives ordering, denoted by
is defined as, for all
(10)
(10)
Definition 6.
A function f is said to be star-shaped (ani-star-shaped) if and
is increasing (decreasing) in
For an application of the new class GL in the field of insurance, if we represent the distribution by the appropriate random variable Y and let β present the risk measure functional, therefore,
Let an insurance contract in a specified period and
be the state space. If none of the risks specified in the policy contract occur during the policy term, then the policy holder receives no monetary compensation for the paid premiums. The loss in compensation that occurs is the difference between the amount of compensation
and the total losses resulting from achieve state θ (denoted by
is log-concave function) is
, where Y is the premium state θ. Suppose that we associate a risk as
, which is convex. We define it using definition 3; thus, it is natural to demand that the risk function includes this as non-decreasing. Then, the expected random variable
based on the convex function,
can be used to study the effects of both investor and analyst beliefs on securities trading. Moreover, we can use GL to measure the dispersion of returns for an investment portfolio. In addition, GL measures the variability of a security’s returns relative to the market index or a particular benchmark. If
is greater than that of the benchmark, then the financial instrument is thought to be more perilous than the benchmark price. Low
may indicate lower risk.
There is also the possibility of applying the new class in the field of investment; for example, admissible investment strategies, which can be defined by , and is defined in EquationEquations (2)
(2)
(2) and Equation(3)
(3)
(3) .
As a result, this study has three aims. The first aim is to consider new nonparametric classes of distributions (, and
), those dependent on
, and
, while introducing certain characterizations and preservations. The second aim is to suggest a new technique that improves comparison between two distributions with certain characterizations and thus, preservations, which depend upon
and
The third goal is to apply these results in random sum and shock models. Section 2 provides sufficient conditions for new classes, introduces their useful properties and characterizations, and studies certain characterizations of the Weibull, exponential, and Erlang distributions through newly suggested aging classes. In addition, we offer a series of inequalities that provide the bounds for
and
functions. In Section 3, relationships between the strong risk order and other stochastic orders are discussed. Furthermore, useful properties and characterizations of the
and
orders are analyzed. We also establish closure properties of
and
orders under relevant reliability operations, such as convolution and mixture. Finally, Section 4 discusses useful applications in random sum and shock models by using new aging classes involving the
and
orders.
2. Characterizations of GL Class
By using G for generalized moment-generating residual life, for decreasing, and I for increasing, we can define the following two classes of a lifetime.
Clearly, and
form a pair of dual classes based on distinguishing
and
and
form a pair of dual classes based on distinguishing
. Next, the results reveal certain properties and applications of the
and
classes of life distributions.
Now, we provide the sufficient conditions for a probability distribution to have the and
property.
Theorem 1.
Let T denote the lifetime of equipment with and
.
If,
(11)
(11) Then, T
.
If,
(12)
(12)
Hence, T .
Proof.
It is easy to prove that,
By using the definition of hazard rate and EquationEquation (5)(5)
(5) , we can decide that,
(13)
(13)
Hence, the proof is complete (i). Now, we prove the sufficient condition for a probability distribution must have the and
properties. First, we have,
By using the definition of the reversed hazard rate and EquationEquation (6)(6)
(6) , we can decide that,
(14)
(14)
Therefore, the proof is complete (ii).
For X to be a non-negative and absolutely continuous random variable, with the distribution function and density function f
, respectively, we denote by,
The generalized Laplace-Stieltjes transformation of f (GL) (or the Laplace transformation of X) and the generalized Laplace transformation of
, respectively.
Next, we characterize the GL class using a function of the respective moments.
Proposition 1.
If X is a nonnegative random variable with the distribution function F and survival function , such that all its moments exist, then we get,
(15)
(15)
Proof.
By Equation (1), we have,
(16)
(16)
By EquationEquations (2)(2)
(2) and Equation(3)
(3)
(3) in Michael et al. (Citation2017), we have,
Moreover, by using Theorem 5.A.5 in Shaked and Shanthikumar (Citation2007, pp. 235), we can complete the proof.
The Weibull distribution has been utilized in many applications, such as reliability centered maintenance, in which a shape parameter , allows the reliability-centered decision for preventive maintenance to be carried out (no). We use the following example to illustrate the importance of Theorem 1 in recognizing
class.
Example 1.
Suppose that T is a Weibull random variable with the density function for
and
. One can easily prove that,
(17)
(17)
and
In addition, if
and
is the lower incomplete gamma, we have,
and
By using EquationEquation (6)(6)
(6) , we have,
if
and
is the lower incomplete gamma. By using EquationEquation (17)
(17)
(17) , we can easily check that the right-hand side of inequality EquationEquation (12)
(12)
(12) is
According to Theorem 1, this means that T
The characteristics of GL will be obtained in the following results.
Theorem 2.
Let
and
(18)
(18)
Then, is a log-concave function.
Proof.
Let,
Then,
(19)
(19)
Therefore, EquationEquation (19)(19)
(19) represents,
Thus, the following equation is obtained.
(20)
(20)
EquationEquation (20)(20)
(20) demonstrates that,
Then, is decreasing. In addition, we get,
and
The proof is complete.
Proposition 2.
Let,
Then,
Proof.
Let,
The proof is complete.
Exponential distribution is extensively and widely used in reliability engineering to model the lifetime of electronic and electrical components and systems.
We use the following example to illustrate the importance of Theorem 2 in recognizing class.
Example 2.
Suppose that T is an exponential random variable with the density function and distribution function,
(21)
(21)
for t > 0, and
. Let,
(22)
(22)
Substituting EquationEquation (21)(21)
(21) in to EquationEquation (22)
(22)
(22) , we obtain
The second derivative of is obtained as,
(23)
(23)
In addition, we have,
(24)
(24)
for
and
From relation (24), we deduce that
is twice differentiable with a log-concave function. It remains to be proven whether
is a log concave or logarithmically concave function. When we know that,
We can now proceed analogously to , and we can deduce that
is logarithmically concave. From Proposition 2, we see that
.
Proposition 3.
Let,
Then,
Proof.
Let,
The proof has been completed.
For several years, great effort has been devoted to the study of the Erlang distribution due to its relationship with exponential and gamma distributions and because of its uses in the field of stochastic processes. Suppose that T is an Erlang random variable with the density function,
where
is the base of the natural logarithm and
is the factorial function. Parameter b is called the shape parameter, and parameter a is called the rate (scale) parameter; the cumulative distribution function of the Erlang distribution can be expressed as,
Now, we can discuss the behavior of in the Erlang distribution.
Example 3.
Suppose that T belongs to the Erlang family with the shape parameter b and rate (scale) parameter a,; then, we have,
According to the transformation technique, we obtain,
for
It easy to prove that,
for and
From Proposition 3, we deduce that
We list a series of inequalities that provide bounds for the
and
functions according to the following theorem.
Theorem 3.
If X is a nonnegative random variable with density function f and distribution function F such that all its moments exist, suppose that
and for
and
Then,
Proof.
By using Theorem (2) and EquationEquations (1)(1)
(1) , Equation(5)
(5)
(5) , and Equation(6)
(6)
(6) , with applied Theorem 3 in Zhang and Jiang (Citation2012), we can complete the proof.
3. Properties of GL Ordering Under Operations
Next, we characterize GL ordering by a function of respective moments.
Proposition 4.
Let X1 and X2 be two non-negative and absolutely continuous random variables, with the distribution functions and
, density functions
and
and survival functions
and
, respectively. Hence,
Proof.
By EquationEquation (16)(16)
(16) and Lemma 2.2 in Chan, Proschan, and Sethuraman (Citation1990), we can complete the proof.
Proposition 5.
Let X1 and X2 be two non-negative and absolutely continuous random variables, with the distribution functions and
, density functions
and
and survival functions
and
, respectively. Hence, if,
Proof.
By Definition 1 (iii) and Equation (15), we can complete the proof.
Next, we check that the order is closed under certain operations by the following results.
Proposition 6.
Let X1 and X2 be two non-negative and absolutely continuous random variables, with the distribution functions and
, density functions
and
and survival functions
and
, respectively. Hence,
If
for any
If
for any
, that is, the order
is invariant under the scale transformation.
Proof.
By EquationEquation (1)(1)
(1) and Definition 2, following the steps of Shaked and Shanthikumar (Citation1994), 3.B.4, we can complete proofs (i) and (ii).
Theorem 4.
Let and V be (n + 1) non-negative and absolutely continuous random variables. If
therefore,
and
if
Proof.
Suppose that ψ is a convex function. Therefore,
(25)
(25)
since
and
; therefore, inequality (25) becomes,
and we obtain a complete proof (a). If and
then we get a complete proof (b).
Convolution is a mathematical tool with multiple applications, including statistics, computer vision, imaging, signal processing, electrical engineering, and differential equations. In probability theory, the distribution function of the sum of two independent random variables is the convolution of their individual distribution. Now, we want to clarify whether GL ordering is closed under convolution.
Theorem 5.
Let
be independent, non-negative, and absolutely continuous random variables, and let
be other independent, non-negative, and absolutely continuous random variables with
. Let A and B be independent of
and
with A
. Hence,
Let
be independent non-negative and absolutely continuous random variables, and let
be other independent non-negative and absolutely continuous random variables. If
we have,
that is, the order is closed under convolution.
Proof.
This proof involved similar steps to those used in the Shaked and Shanthikumar (Citation2007) theorem 5.C.16.
Suppose that we are given a one-parameter family of life distribution {
}, with parameter
We look at θ as a random variable and let K be its distribution function; in the literature K, it is said to be a mixing distribution. Then, the mixture H of the family
with respect to K and the mixture
of
are defined by the following equations.
and
As usual, we assume that family and mixing distribution G satisfy certain conditions, and we want to derive the properties of the resulting mixture H.
Theorem 6.
Suppose that for any
. For arbitrary mixing distribution K, then,
In other words, order is in general, closed under an arbitrary mixture.
Proof.
The proof follows similar steps to those used by Shaked and Shanthikumar (Citation1994), 3.B.4.
Next, we introduce the characteristics of
Theorem 7.
Proof.
satisfies the following relation.
(26)
(26)
By using EquationEquations (26)(26)
(26) and Equation(13)
(13)
(13) , we have,
The next result is an analog of Theorem 7; we will not detail the proof.
Theorem 8.
4. Applications of GL Ordering
Belzunce, Gao, Hu, and Pellerey (1999) examined new better (worse) than used use of the Laplace transform, NBULt as follows.
or
for all
. In addition, Belzunce et al. (Citation1999) introduced the new Laplace transform function as,
When c = 1 in EquationEq. (3)(3)
(3) , we get,
(27)
(27)
Now, we can define the new better (worse) than used with respect to the generalized Laplace transform, NBUGLt, as follows.
for all
and the exponentially better (worse) than used with respect to the generalized Laplace transformation, EBUGLt,
as follows.
for all
The following results can be derived in an analogous manner to Proposition 2 of Belzunce et al. (Citation1999) and Proposition 2.4 in Wang and Ma (Citation2009); the proof is thus omitted here.
Proposition 7.
Let U be a non-negative and absolutely continuous random variable with the distribution function and
, where h is increasing. Suppose that f is the inverse of h. Hence,
If
and
is a star-shaped (ani-star-shaped) function, then
If
and
is a star-shaped (ani-star-shaped) function, with
, hence
Now, we apply our results in random sums of variables and shock models in the following propositions.
For the following definitions, we use a compound modified geometric distribution, which possesses the discrete property of non-aging.
Definition 7.
Suppose that is a random variable with the survival function
for
; then,
, if and if only if,
, if and if only if,
, if and if only if,
Definition 8.
A function f: is said to be totally positive of order 2
if for all x1 < x2 and y1 < y2
and,
In the following results, we provide and
in random sums applications.
Theorem 9.
Let
be independent, non-negative, and random variables, such as
for all
and let
and N2
which are independent of Ui. If
, then,
Let
be independent, non-negative, and random variables, such as
for all
and let
and N2
which are independent of Ui. If
, then,
Proof.
Random variable belongs to the compound modified geometric survival function
for
=
, and
, where
is an independent and identically distributed sequence of positive random variable. Since
, we also have,
In addition, by Lemma 2.1(b) in Pellerey (Citation1993), we obtain is TP2. By Proposition 4 and Definition 1(vi), we have,
We then have the complete proof of (i), and the proof of (ii) can be obtained using a similar argument.
Next, we introduce some new applications of classes and stochastic orders in the sums of independent exponential random variables via Wilks’ integral representation.
Theorem 10.
Let be independent and identically exponential random variables with parameters
suppose that
is a random variable with a modified geometric density function that is independent of
Let,
(28)
(28)
where
,
,
(29)
(29)
(30)
(30)
and
Then,
If
is decreasing (increasing) in t, then
If
is decreasing (increasing) for all t > 0, then
Proof.
The random variable belongs to the compound modified geometric survival function,
(31)
(31)
for
,
and
where
are independent and identically exponential random variables with parameters
. Following Favaro and Walker (Citation2010), we have the following approximation.
By using the generalized hypergeometric series as shown below,
(32)
(32)
as
which is Pochhammer’s polynomial. Inserting this expansion into the expression of Favaro and Walker (Citation2010, pp. 2041), we have the below approximation as,
(33)
(33)
where
,
, and
Then, by EquationEquation (33)
(33)
(33) we get,
(34)
(34)
where,
and
By EquationEquation (32)(32)
(32) , we have,
With this binomial expansion and when,
we get,
By using Pochhammer’s polynomial (), we have the following result.
With similar steps, we can obtain B as follows.
which is equivalent to,
where,
Therefore, by EquationEquations (34)(34)
(34) and Equation(31)
(31)
(31) , we can conclude that,
Once is probability, then it is TP2. In addition, we note that
is TP2; then,
is
Therefore, following Karlin (Citation1968, p. 21), and using the variation diminishing property, we obtain that
has at most one change of sign for all t if one change occurs; it occurs from + to –. Hence,
The proof (ii) follows similar arguments.
Shock models have been studied by several authors, and such research has provided a realistic formulation for modeling certain reliability systems situated in random environments. Various models collected here are physically motivated. For instance, the extreme and cumulative shock models may be appropriate descriptions for the fracture of brittle materials, such as glass, and for damage due to the earthquakes or volcanic activity. In such cases, the time between two consecutive shocks (inter-arrival times), the damage caused by a shock, the system failure and the relationships among all these elements are modeled and characterized within a shock model. In the literature, two major types have been distinguished depending on whether or not the effect of the shock on the system is independent of its arrival time. We can assume independence between the shock effect and the arrival time; we can let N be the number of shocks survived by the system and be the random inter-arrival time between the
and k – th shocks; and we can denote a sequence of surviving probabilities
which is defined by the probability that the system still runs after the kth shock. The lifetime
of the system is then given by
Therefore, this shock model represents the particular case of random sums. We can apply Theorem 9 to shock models where the interarrival times are exponentially distributed, where we consider that the survival function of the shock model can be written as follows.
(35)
(35)
where
and
as described in EquationEq. (31)
(31)
(31) . Hence, we can apply Theorem 10 in a non-stationary model as follows.
Theorem 11.
Suppose that U is the lifetime of advice with survival function given in Equation (35) and
and
which is described in Equations (28) – (30). If
. Then, if
for all t > 0 and if
is star-shaped (anti-star-shaped), therefore
Now, we mention the closure properties of the IMIT class under Non-homogeneous Poisson shock models.
Proposition 8.
Let be increasing (decreasing) in k, for all
and let
be decreasing in t,
. Then, the lifetime U with survival function
defined as in Equation (35), satisfies the
Acknowledgements
The author would like to express their sincere gratitude to the anonymous editor, the associate editor, and the referees for their very constructive and valuable comments and suggestions that add to the quality of the manuscript and increase its readability.
References
- Ahmed, H., & Kayid, M. (2004). Preservation properties for the Laplace transform ordering of residual lives. Statistical Papers, 45(4), 583–590. doi: 10.1007/BF02760570
- Ali, N. S. A. (2018). On the properties of the UBAC (2) class of life distributions. Journal of Testing and Evaluation, 46(2), 730–735.
- Anis, M. Z. (2011). Testing exponentiality against NBUL alternatives using positive and negative fractional moments. Economic Quality Control, 26(2), 215–234.
- ArdiÇ, M. A., Akdemir, A. O., & Özdemir, M. E. (2018). Several inequalities for log-convex functions. Transactions of A. Razmadze Mathematical Institute, 172(2), 140–145. doi: 10.1016/j.trmi.2018.03.004
- Belzunce, F., Gao, X., Hu, T., & Pellerey, F. (2004). Characterizations of the hazard rate order and IFR aging notion. Statistics & Probability Letters, 70(4), 235–242. doi: 10.1016/j.spl.2004.10.007
- Belzunce, F., Ortega, E., & Ruiz, J. M. (1999). The Laplace order and ordering of residual lives. Statistics & Probability Letters, 42(2), 145–156. doi: 10.1016/S0167-7152(98)00202-8
- Chan, W., Proschan, F., & Sethuraman, J. (1990). Convex-ordering among functions, with applications to reliability and mathematical statistics. Lecture Notes-Monograph Series, 121, 134.
- Elbatal, I. (2007). The Laplace order and ordering of reversed residual life. Applied Mathematical Sciences, 36, 1773–1788.
- Favaro, S., & Walker, S. G. (2010). On the distribution of sums of independent exponential random variables via Wilks’ integral representation. Acta Applicandae Mathematicae, 109(3), 1035–1042. doi: 10.1007/s10440-008-9357-5
- Gandotra, N., Gupta, N., & Bajaj, R. K. (2014). Preservation properties of the Laplace transform and moment-generating function ordering of residual life and inactivity time. Journal of Statistics and Management Systems, 17(4), 301–310. doi: 10.1080/09720510.2014.914294
- Karlin, S. (1968). Total Positivity, Vol. I. Stanford, CA: Stanford University Press.
- Kayid, M., & Alamoudi, L. (2013). Some results about the exponential ordering of inactivity time. Economic Modelling, 33, 159–163. doi: 10.1016/j.econmod.2013.04.002
- Kayid, M., Izadkhah, S., & Alshami, S. (2016). Laplace transform ordering of time to failure in age replacement models. Journal of the Korean Statistical Society, 45(1), 101–113. doi: 10.1016/j.jkss.2015.08.001
- Klar, B., & Müller, A. (2003). Characterizations of classes of lifetime distributions generalizing the NBUE class. Journal of Applied Probability, 40(1), 20–32. doi: 10.1017/S0021900200022245
- Lee, J., & Tepedelenlioglu, C. (2014). Laplace functional ordering of point processes in large-scale wireless networks. arXiv preprint arXiv:1408.4528.
- Mahmoud, M. A. W., Moshref, M. E., & Gadallah, A. M. (2014). On NBUL class at specific age. International Journal of Reliability and Applications, 15(1), 11–22.
- Michael, M. C., Anene, O. C., Akudo, A. M., & Nwabueze, I. J. (2017). Generalized Moment Generating Functions of Random Variables and Their Probability Density Functions. American Journal of Applied Mathematics and Statistics, 5(2), 49–53.
- Pellerey, F. (1993). Partial orderings under cumulative damage shock models. Advances in Applied Probability, 25(4), 939–946. doi: 10.2307/1427800
- Rajan, A. (2014). On the ordering of communication channels. Arizona: Arizona State University.
- Rajan, A., & Tepedelenlioğlu, C. (2015). Stochastic ordering of fading channels through the Shannon transform. IEEE Transactions on Information Theory, 61(4), 1619–1628. doi: 10.1109/TIT.2015.2400432
- Shaked, M., & Shanthikumar, J. G. (1994). Stochastic orders and their applications. San Diego: Academic Press.
- Shaked, M., & Shanthikumar, J. G. (2007). Stochastic orders. New York: Springer Science & Business Media.
- Wang, W., & Ma, Y. (2009). Stochastic orders and aging notions based upon the moment generating function order: theory. Journal of the Korean Statistical Society, 38(1), 87–94. doi: 10.1016/j.jkss.2008.07.004
- Zamani, Z., Mohtashami Borzadaran, G. R., & Amini, M. (2017). A note on some preservation results on the Laplace transform ordering of residual lives. Risk and Decision Analysis, 6(3), 225–229. doi: 10.3233/RDA-170129
- Zhang, X., & Jiang, W. (2012). Some properties of log-convex function and applications for the exponential function. Computers & Mathematics with Applications, 63(6), 1111–1116. doi: 10.1016/j.camwa.2011.12.019