Abstract
Over the last few years, smart speakers such as Amazon Echo and Google Home have become increasingly present within British households. Yet, privacy remains a prominent concern in the public discourse about smart speakers, as well as in the nascent academic literature. We argue that privacy in the context of smart speakers is more complex than in other settings due to smart speakers’ specific technological affordances and also the axial relationships between users, the device, device manufacturers, application developers, and other third parties such as moderation contractors and data brokers. With survey data from Amazon Echo and Google Home users in the UK, we explore users’ privacy concerns and privacy protection behaviors related to smart speakers. We rely on a contextual understanding of privacy, assessing the prevalence of seven distinct privacy concern types as well as three privacy protection behaviors. The results indicate that concerns about third parties, such as contractors listening to smart speaker recordings, are most pronounced. Privacy protection behaviors are uncommon but partly affected by privacy concerns and motives such as social presence and utilitarian benefits. Taken together, our research paints a picture of privacy pragmatism or privacy cynicism among smart speaker users.
Introduction
Smart speakers are voice-controlled mobile devices which use artificial intelligence (AI) and natural language processing to facilitate a variety of functional and hedonic tasks, such as playing music, setting reminders, and retrieving information (Lau, Zimmerman, and Schaub Citation2018). Normally located in the home and sometimes embedded within a larger “smart home” ecosystem, smart speakers are quickly becoming mainstream. Adoption statistics for the United States show that 66 million units had been sold as of December 2018, with Amazon Alexa-enabled products, such as the Amazon Echo, as the clear market leaders (70% market share) (Feiner Citation2019). Trailing behind are Google Assistant-enabled products (24%) and Apple Siri-enabled products (6%). Although smart speakers entered the UK market later, the adoption trend is similar, with a 20% adoption rate as of 2019 (Ofcom Citation2019).
While smart speakers have enjoyed rapid uptake among consumers, research has only started to investigate this technology (Hoy Citation2018; Smith Citation2020). Much of the early research has been published in the fields of computer science and human-computer interaction (Feng, Fawaz, and Shin Citation2017; Geeng and Roesner Citation2019; Lau, Zimmerman, and Schaub Citation2018; Luger and Sellen Citation2016; Malkin et al. Citation2019; Zheng et al. Citation2018), with only limited discussion in the social sciences (Brause and Blank Citation2020; Pridmore et al. Citation2019). Recent human-machine communication research, however, has quickly advanced our understanding of communication modalities with smart speakers and other non-embodied virtual personal assistants (Guzman Citation2017, Citation2019).
One important aspect that has received substantial attention in the media and academic literature is user privacy (Alepis and Patsakis Citation2017; Liao et al. Citation2019). When operational, smart speakers remain in a continuous listening-mode, awaiting audio recognition of a designated “wake-word” to activate and begin interacting with the user. Because activation of the smart speaker also commences a transmission of live audio data across Wi-Fi to the company (e.g., Amazon) for processing and storage, this “always on” mentality raises privacy risks for users (Liao et al. Citation2019). Indeed, prior research has identified microphones as one of the most intrusive sensor categories in home contexts (Bugeja, Jacobsson, and Davidsson Citation2016).
Martin and Shilton (Citation2016) argue for a fine-grained, contextual approach to privacy in the context of mobile devices. However, existing research around smart speakers continues to adopt an undifferentiated view of privacy that fails to do justice to the relational nature of the technology, embedded as smart speakers are within a varied stakeholder network. In our study, we therefore use a contextual and multi-axial understanding of privacy, distinguishing seven privacy concern modalities, based on seven distinct user-stakeholder relationships. This aligns with the existing framework separating social and institutional privacy concerns (Raynes-Goldie Citation2010; Young and Quan-Haase Citation2013). Three of the proposed privacy modalities relate to social privacy: privacy concerns about household members; privacy concerns about strangers (e.g., hackers); and privacy concerns about the smart speaker itself as a social agent. The remaining four types relate to institutional privacy: privacy concerns about the company, namely Amazon or Google; privacy concerns about contractors who analyze the voice recordings; privacy concerns about third party app/skill developers; and privacy concerns about the government. In adopting this differentiated understanding of privacy concerns, we are able to compare how each privacy modality affects privacy protection behavior in variegated ways. The article further engages with Nissenbaum’s (Citation2004, Citation2010) privacy as contextual integrity theory. While developed in relation to public surveillance and in a time of less technology-saturation, the theory, and especially its core concepts of norms of appropriateness and norms of flow, offers a fruitful approach to the study of smart speakers.
Our analysis suggests that institutional concerns, particularly about contractors and third party developers, are most pronounced. At the same time, privacy protection behavior is largely absent, with few users engaging regularly in technical, data-related, or social forms of privacy protection. Nevertheless, certain privacy concerns are significantly and positively associated with privacy protection behavior, partly refuting the notion of the privacy paradox.
Our study makes several contributions to user-centered research on AI within a media and communication perspective. The inclusion of previously overlooked relational dynamics, such as privacy concerns about household members and contractors, adds nuance to privacy scholarship in the context of emerging technologies. It also offers originality by integrating affordances and privacy as contextual integrity perspectives.
In the remainder of the article, we first provide a literature review of privacy in the context of smart speakers. We approach the literature review from a phenomenological perspective first, before introducing privacy as contextual integrity theory as a useful lens to study privacy issues surrounding smart speakers. We then discuss the methodology and data collection, including an overview of the sample, measures, and analytical procedure. This is followed by a presentation of descriptive and inferential results. Finally, we contextualize the findings within the privacy and human-machine communication literature, show their implications, and point to limitations of our approach.
Literature review: Privacy concerns in the context of smart speakers
Several studies have looked at privacy in the context of intelligent assistants and smart speakers. Users can engage with intelligent assistants, such as Alexa or Siri, in multiple locations and in diverse ways (Guzman Citation2019, Citation2020). From an affordances perspective (Evans et al. Citation2017), whereby affordances are possibilities for action emerging from the relational structure between a technology and the user, intelligent assistants enable interactivity, searchability, and recordability. Being accessible via a smartphone, users can maintain continuous, unimpeded access to the intelligent assistant’s functions, such as search, music playback, and calendar maintenance. However, accessing the intelligent assistant via a smartphone is purposeful; users must choose to engage with the assistant and manually activate it through clicking the “home” button or through the smartphone application. By contrast, smart speakers are embodied material devices, usually placed in a particular location and often within the home. As opposed to requiring manual activation, smart speakers remain in a continuous listening-mode, awaiting audio recognition of a designated “wake-word”.
McLean and Osei-Frimpong (Citation2019) found that privacy risks serve as a moderator, weakening the relationships between motives and reported use. However, they did not consider the prevalence of different types of privacy concerns and their measurement of perceived privacy risks conflated different aspects of privacy. Further, they did not clearly differentiate between privacy risks of the device itself, the manufacturer (Amazon), third parties, other users, or additional stakeholders. A more sophisticated understanding of privacy is developed by Manikonda, Deotale, and Kambhampati (Citation2018). Based on online reviews and a survey of Amazon Echo and Google Home users, as well as former users, they distinguished seven privacy themes: hacking, collection of personal information, recording private conversations, listening 24/7, respecting the users’ privacy, data storage repository, and creepy nature. In their results, they report that Amazon Echo users were more concerned about privacy than Google Home users, with roughly two thirds of Amazon Echo users reporting privacy concerns. While this research offers a more differentiated understanding of privacy, it remains descriptive and is not motivated by privacy theory. Liao et al. (Citation2019) conducted a survey of 1160 intelligent assistant users and non-users to study the motivations for use, privacy concerns, and trust. Using a social contract theory lens (Martin Citation2012, Citation2016a), they differentiate smartphone-based intelligent assistants (e.g., Apple Siri) from home-based intelligent assistants (i.e., smart speakers such as Amazon Echo). Users of both types showed fewer privacy concerns than non-users. While their study advances our understanding of privacy and smart speakers by introducing the social contract theory lens, the prevalence and predictors of privacy protection behaviors are not explored.
In effect, researchers to date had an undifferentiated view of privacy that fails to do justice to the multiple stakeholders involved in smart speaker ecosystems. In our study, we therefore rely on a contextual and multi-axial understanding of privacy, distinguishing seven privacy concern modalities. This approach aligns with the existing research separating social and institutional privacy concerns (Raynes-Goldie Citation2010; Young and Quan-Haase Citation2013) and approaches privacy from a contextual integrity perspective (Nissenbaum Citation2004).
Three of the proposed privacy modalities relate to social privacy.
Smart speaker as a social agent: Firstly, we consider privacy concerns about the smart speaker itself as a social agent. Increasingly, artificial companions and social robots are imbued with social agency and users can develop relationships with the device (Pfadenhauer Citation2015). As per the computers are social actors (CASA) paradigm, humans ascribe human traits to computers and AI (Nass, Steuer, and Tauber Citation1994; Nass and Moon Citation2000; Reeves and Nass Citation1996). Moreover, because of the smart speaker’s presence in the home, users also domesticate smart speakers, developing a rapport and using it for a variety of practical and hedonic tasks (Brause and Blank Citation2020). Smart speakers are different from other interactive technologies, such as video games or online browsing, in that users primarily interact through natural-language voice-based commands, mirroring human-to-human communication (Guzman Citation2017). Although the smart speaker is only one of many potential “homes” for the disembodied intelligent assistant, users frequently conflate the intelligent assistant and the device. Amazon Echo, the material smart speaker, is often thought of as being “Alexa”, anthropomorphized and attributed with social presence (Foehr and Germelmann Citation2020; Guzman Citation2017).
Household members: Privacy intrusion has been documented within the home, on a more personal level. Smart speakers could be used to track and surveil other household members, since all conversations with the smart speaker are logged by default and can be accessed by the account holder of the device. While smart speakers can be useful tools for parental oversight of young children, for instance, ensuring that they are not accessing age-inappropriate material, the surveillance affordances can also reinforce domestic power imbalances in the form of technological intimate partner violence (Geeng and Roesner Citation2019). For example, domestic abusers can monitor the movements, search activity, and conversations of their victims through smart speakers (Lopez-Neira et al. Citation2019). This issue is compounded in cases of unilateral control over connected smart-home systems, such as door locks, lights, or heating systems.
Strangers: Research has shown that smart speakers have security vulnerabilities which hackers can exploit (Chung, Park, and Lee Citation2017). Although the likelihood of malicious actors accessing an individual smart speaker is low, cases of Echo recordings being accidentally played to strangers have made headlines (Ingber Citation2018; Wamsley Citation2018). Consequently, smart speaker users face the possibility that their data may be accessed by unknown third parties. As smart speakers become more connected with other smart home devices, such as doorbells or locks, possibility of hacking will only increase.
The remaining four types relate to institutional privacy:
Company: In line with comprehensive dataveillance strategies of parent companies, data from Amazon Alexa and Google Home accounts can be used for profiling (Büchi et al. Citation2020; Hildebrandt Citation2008) and the provision of targeted advertisements. In this sense, data collected through smart speakers can be combined with data from other sources, creating fine-grained user profiles which are valuable and monetizable within the larger eco-system of the manufacturers’ offerings. Pridmore et al. (Citation2019), for instance, discuss how dataveillance serves different purposes depending on the company’s business model. For Google, dataveillance through Google Home leads to more sophisticated and streamlined targeted advertising. For Amazon, the integration with its shopping platform seems key. Indeed, as individuals increasingly use voice-based search to peruse and purchase products, Amazon’s stranglehold over the market will only grow. Meanwhile, concern about Internet companies may be mitigated by persistent misconceptions about companies’ privacy policies (Turow, Hennessy, and Draper Citation2018) and the social pressure of using services such as Google, Facebook, and Amazon (Lutz, Hoffmann, and Ranzini Citation2020).
Contractors: Data extracted from smart speakers is subject to human audit by third party contractors, who listen to recordings in order to improve the system (Day, Turner, and Drozdiak Citation2019a, Citation2019b). The privacy implications of personal conversations and sensitive information being heard by unknown individuals is already evident, especially since the human contractors are hidden from the end-user by additional institutional layers. Not employed directly by the parent company, these contractors reflect the messy ecosystem of data handling. Yet, audio recordings have been shown to be sometimes subject to mockery by these contractors, raising concerns about the sensitivity and professionalism of the invisible “listening ear” (Estes Citation2018, Citation2019). This type of privacy violation might be particularly problematic given that it violates norms of flow (Nissenbaum Citation2004). Within the framework of contextual integrity, Nissenbaum (Citation2004) differentiates norms of appropriateness and norms of flow. The first set of norms pertain to what type of information is appropriate to be disclosed in a given context, while the second set of norms pertain to whom information should be distributed to. While there might be few limits in terms of what is inappropriate to reveal to a smart speaker, violations in the norms of flow could be particularly serious privacy breaches.
Privacy concerns about third party app/skill developers: Similar to apps for smart phones and tablets, third parties can develop so-called “skills” (Amazon) or “apps” (Google) that enhance the user experience of smart speakers. Skills/apps may include credit-card balance checks, food delivery, or bedtime stories for children. They can also be integrated with additional devices, such as smart home systems or fitness trackers. Yet, in order to access such additional skills/apps, smart speaker data is often collected and shared with these developers. Indeed, handing usage data or recordings over to developers is sometimes the hidden cost for greater functionality. External developers often act as an entry point in the supply chain of customer data to data brokers (Martin Citation2016b). Indeed, the Cambridge Analytica scandal has shown that the data eco-system can be leaky and third party developers might not always have the best intentions. Given the sensitive nature of data collected by smart speakers, data breaches through third party developers could be particularly problematic.
Government: A key and growing concern is the involvement of smart speakers with the wider governmental and law enforcement system. Although smart speaker data flows operate worldwide, individual smart speaker data can be used in local courts (Lieber Citation2018). A US court case, for instance, shows that smart speaker data is subject to subpoena, raising concerns about how government and law enforcement could gain access to intimate and private recordings (Buhr Citation2016). Moreover, in more authoritarian regimes, the potential mass collection of data from “within the home” about personal habits, behaviors, and conversations, cannot be overlooked. To assess the prevalence of different privacy concern types, we ask the following research question.
RQ 1: To what extent do users of smart speakers have different privacy concern types?
We address this research question guided by Nissenbaum’s theory of privacy as contextual integrity. Nissenbaum (Citation2004) developed this theory in response to inadequate privacy policies and frameworks for addressing issues related to public surveillance in the United States. Existing privacy rationales in the US centered on three main principles: protection of the individual from government intrusion (e.g., protection from unwarranted search), protection of sensitive information (e.g., health data is considered sensitive data and protected by special regulation), and protection of the home as a sacred place. Public surveillance challenges these principles, as it “typically involves a new technology, or a newly developed application of entrenched technology that expands the capacity to observe people; gather information about them; and process, analyze, retrieve, and disseminate it” (133-134). The existing principles are not sufficiently equipped to deal with public surveillance and tend to neglect public surveillance as a privacy problem.
Nissenbaum’s (Citation2004) theory takes different social spheres to be characterized by different information norms. The norms of appropriateness and flow are particularly important and contextual integrity is upheld when both norms are maintained in a given context. Norms of appropriateness establish which types of information are acceptable to be shared in a given context. Violations of these norms can occur when contextually inappropriate information is disclosed or asked for, for example, when a teacher asks students about their sexual orientations. Norms of flow establish how information would be shared appropriately, involving elements such as free choice, discretion, confidentiality, need, entitlement, and obligation. Violations of the norms of flow can occur when information is unduly used in a different context, for example, when a doctor reveals the medical condition of a patient to her neighbor. Nissenbaum (Citation2010) later identified five parameters that characterize information flows in a given context: sender, recipient, attribute/information type, subject, and transmission principle.
Following this theory, Apthorpe et al. (Citation2018), in their study on smart home devices, tested the users’ perceptions of 3840 information flow scenarios. They found that users perceived certain configurations (e.g., fitness tracker sending audio data) as more severe violations of contextual integrity than others. The least acceptable recipients were government intelligence agencies, the owner’s social media account, and Internet service providers. Security cameras and refrigerators were the least acceptable senders. Consent and emergency situations emerged as the transmission principles that affected acceptability most strongly and positively. In general, Apthorpe et al. (Citation2018) found that “the average survey respondent still views information flows from smart home devices to recipients outside of the home as generally unacceptable unless the device owner has specifically granted consent” (2).
As a relatively static device, information flows of smart speakers remain consistent in terms of (domestic) context, but they vary in the specific parameters laid out by Nissenbaum (Citation2010). Due to the multi-directional nature of data flow from the smart speaker, the recipient and – partly – the transmission principle can vary, with multiple recipients receiving data simultaneously. This suggests that contextual integrity could be violated in some modalities and not others, particularly since the user’s awareness of the recipient and consent may differ among each of the five parameters.
Rather than smart speakers maintaining consistent privacy norms, it is thus important to explore whether and how these norms shift based on the potential recipient of data.
The privacy paradox speaks to the divergence between people’s privacy attitudes and privacy behavior (Barnes Citation2006). The most common explanation for the occurrence of this paradox is the privacy calculus, where users weigh the benefits of using a technology against its privacy risks (Dinev and Hart Citation2006). However, the privacy calculus has been criticized from various angles (Hoffmann, Lutz, and Ranzini Citation2016) and alternative approaches existFootnote1 (Kokolakis Citation2017). From an empirical perspective, the findings on the privacy paradox are inconclusive; privacy concerns seem to have a small effect on privacy behavior (Baruh, Secinti, and Cemalcilar Citation2017). In order to examine the relationship of privacy concerns with privacy protection behavior, we pursue our second research question.
RQ 2: How do different privacy concern types relate to privacy protection behavior?
Following the privacy paradox literature and emerging research on use modalities and benefits of smart speakers (Brause and Blank Citation2020; Foehr and Germelmann Citation2020; Liao et al. Citation2019; McLean Citation2019), we also consider motives for smart speaker use. In their investigation of Amazon Echo users in the UK, McLean and Osei-Frimpong (Citation2019) identified five benefits: utilitarian, hedonic, symbolic, social presence, and social attraction. They find that social benefits had the strongest effect on usage. Utilitarian benefits also had a pronounced effect, but the other benefits (hedonic and symbolic) had only a weak influence on usage. While desired benefits increase the likelihood of using smart speakers, privacy protection behaviors (PPB) may limit users from enjoying the smart speaker’s full technical and practical functionality. For instance, if users regularly curate their Alexa or Google Assistant data traces, the smart speaker would lose some of its personalization power. Thus, highly motivated users may have a stronger propensity to forgo certain privacy protections in order to be able to make use of the smart speaker’s full functionality. Given the exploratory nature of our research design, we formulate a research question rather than a hypothesis.
RQ 3: How do use motives impact privacy protection behavior?
Use frequency could affect the likelihood of privacy protection behavior. Smart speakers are mostly employed in people’s homes, thus entering the domestic sphere (Brause and Blank Citation2020; Foehr and Germelmann Citation2020). As seen with other media devices, users integrate smart speakers into everyday life in a gradual process of domestication (Quandt and von Pape Citation2010). Following this logic and adopting a habituation lens, the more users interact with smart speakers, the more they might want to rely on the full functionality of the technology and the more they take it for granted. We thereby formulate our fourth research question.
RQ 4: How does use frequency impact privacy protection behavior?
Social influence, also referred to as subjective norms, describes the “perceived social pressure to perform or not to perform the behavior” (Ajzen Citation1991, 188). In the case of smart speakers, social influence refers to the social environment’s role in fostering smart speaker use, rather than fostering privacy protection behavior. The more friends, family, and colleagues encourage and support smart speaker use, the more comfortable users feel in using it. Since privacy protection behavior might be seen as a form of disruption of routines, a negative relationship between social influence and privacy protection behavior could emerge. However, a positive relationship would also be possible, namely if users live in a social milieu that encourages trying out new technologies but also values privacy protection. We thereby formulate our fifth research question.
RQ 5: How does social influence impact privacy protection behavior?
Finally, we include Internet skills as a variable of interest. Internet skills have been shown to affect privacy protection behavior in general online settings (Büchi, Just, and Latzer Citation2017), as they “enable users to reduce risks of privacy loss while obtaining the benefits from online activities that increasingly depend on the revelation of personal data” (1261). However, the role of Internet skills in affecting online privacy protection behavior has not been investigated in the context of smart speakers and we thereby formulate our last research question, an open-ended one.
RQ 6: How do Internet skills affect privacy protection behavior?
Methods
Data collection
We conducted an online survey of smart speaker users in the United Kingdom. We used Prolific to recruit the respondents, as it enabled screening of participants based on smart speaker ownership. It also ensured good data quality, user friendliness, and ethical participant remuneration (Palan and Schitter Citation2018). The survey was launched in late October 2019 and sampled 375 individuals. Eight respondents were removed because their responses had missing values on all or most variables or were incorrectly screened into the survey (i.e., they did not use a smart speaker or had not used one in the past), resulting in a sample size of 367. Participants were 36 years old on average (median 34; SD = 12; range from 18 to 72), indicating a young to middle-aged profile. Two hundred and fourteen respondents (58.3%) reported being female, 152 (41.4%) being male, and one respondent chose (0.3%) “other.” Thus, our sample has an overrepresentation of individuals reporting being female. In terms of education, the sample was highly educated, with 57.5% of respondents having completed a tertiary qualification (42.8% bachelor’s, 12.0% master’s, and 2.7% doctorate or higher (where “higher” could refer to a habilitation or second doctorate). 9.3% reported having an apprenticeship or other vocational training as their highest level of education, 24.0% have completed upper secondary school (A levels or equivalent), and 9.3% lower secondary school (GCSEs or equivalent).
Measures
All respondents answered a series of demographic questions, followed by a six item scale on Internet skills (Hargittai Citation2005, Citation2009; Cronbach’s α = 0.88). These items were included because Internet skills have been shown to affect privacy protection behavior in general online settings (Büchi, Just, and Latzer Citation2017). Participants were filtered into different survey branches depending on their answer to a question about which smart speaker they use. If they used multiple smart speakers, we asked them to select the most frequently used model: Amazon Alexa-enabled products (i.e., Amazon Echo, Echo Dot), Google Assistant-enabled products (i.e., Google Home), Apple Siri-enabled products (i.e., Apple HomePod), and Other. The questions for Amazon Echo users, Google Home users, and Apple HomePod users were identical, except for the wording about the smart speaker the respondent was using. Respondents who used a smart speaker in the past but did not do so anymore were branched into an ex-user category and were provided with questions about why they stopped using the smart speaker.
Smart speaker users were asked about their motives for using the smart speaker, based on an existing scale (McLean and Osei-Frimpong Citation2019). This scale has 18 items and five dimensions in its original version: utilitarian, hedonic, symbolic, social presence, and social attraction. The scale was adjusted (see below in Exploratory Results) and in the Appendix shows the items and dimensionality.
Four items on social influence were included based on Venkatesh et al. (Citation2003; see in the Appendix). This scale had a Cronbach’s α of 0.78.
Sixteen items on privacy protection behavior () and an open text box on other privacy protection strategies initiated the privacy-related section of the survey. This was followed by question blocks on the seven privacy types mentioned above. Each privacy type was measured with between four and eight items. A full list of the privacy concern items is included in in the Appendix. The scales showed good internal consistency with Cronbach’s α values ranging from 0.88 to 0.96. For privacy concern items, 1 indicates “No concern at all”, 2 “Low concern”, 3 “Moderate concern”, 4 “High concern”, and 5 “Very high concern”. The question prompt for all privacy concern items was: “How concerned are you about the following privacy risks?” For behavioral items (e.g., privacy protection behaviors), the frequencies ranged from 1 “Never”, to 5 “Very often”. Unless otherwise specified, all other items used 1-5 Likert scales, where 1 indicates “Strongly disagree” and 5 indicates “Strongly agree”.
Table 1. Exploratory factors analysis of privacy protection behavior.
Data analysis
We used a combination of descriptive, exploratory, and inferential approaches to analyze the data quantitatively. This included exploratory factor analysis (EFA) and linear regression analysis. All analyses were conducted with IBM SPSS Statistics (v.25), except for the regression, where we used Stata (v.15). For the EFA of privacy protection behaviors, we used principal axis factoring with Promax rotation and the Kaiser criterion. Principal axis factoring, and thus an EFA rather than a principal component analysis approach, was chosen because we assumed that the items would build relatively concise factors that follow existing research (Brown Citation2009a). Promax rotation was chosen because we preferred an oblique over an orthogonal approach, assuming that the dimensions would be substantially correlated (Brown Citation2009b). Finally, the Kaiser criterion was chosen because it is conventionally used (Brown Citation2009c). The EFA showed a good fit to the data, with a KMO value of 0.87, corresponding to a “meritorious” solution (Kaiser Citation1974). For the linear regression, we used robust standard errors to account for potential heteroscedasticity in the data. We also checked for multicollinearity but the largest variance inflation factor was 3.27 (for third party privacy concerns), suggesting the absence of severe multicollinearity.
Results
Descriptive and exploratory results
Of the 367 respondents, 263 reported using an Amazon Echo (72%), 73 a Google Home (20%), 12 an Apple HomePod (3%), and 3 (1%) another speaker (all of whom indicated that they used a Sonos). The remaining 16 respondents (4%) were ex-users. These numbers correspond with a representative survey by YouGov (Feldman Citation2018), which showed that among the one in ten UK adults who own a smart speaker, Amazon is the dominant brand (69%), followed by Google (19%), Sonos (5%), Apple (1%), and other (2%). In the following, we focus on the Amazon Echo and Google Home group (n = 336) due to the small number of Apple HomePod and Sonos users in our sample.
RQ1 asked to what extent different privacy concerns were present among users of smart speakers. displays the arithmetic means of the seven privacy concerns dimensions for Amazon Echo and Google Home users.
Figure 1. Arithmetic means for seven privacy concern types.
Notes: 1-5 Likert scales; n Amazon Echo: 263; n Google Home: 73; errors bars = standard deviations; values above boxes = arithmetic means for privacy concern type and smart speaker brand.
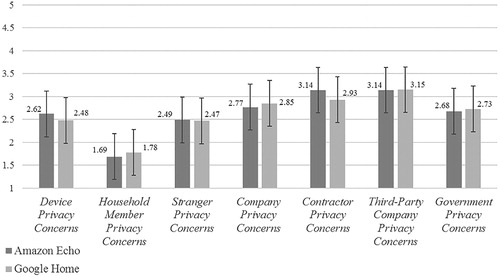
While users overall report low to moderate levels of concern about their privacy, we can identify variations in level of concern based on the relational dimension. Users demonstrate the least concern about issues of social privacy, with concerns about other household members (M = 1.69/1.78; SD = 1.00/1.10) ranking the lowest of all types. Users report relatively higher concerns about institutional privacy issues, where concerns about contractors (M = 3.14/2.93; SD = 1.32/1.37) and third party developers (M = 3.14/3.15; SD = 1.30/1.15) accessing their smart speaker data rank the highest. On a more fine-grained level, responses for the item “Amazon/Google contractors listening to my private conversations” (M = 3.44/3.11; SD = 1.28/1.31) showed the highest level of concern. We used t-tests to compare the arithmetic means of the seven privacy concerns types between Amazon Echo and Google Home respondents. However, none of the differences were statistically significant. The insignificant results suggest that Amazon Echo and Google Home devices are perceived similarly in terms of privacy threats.
Turning to privacy protection behavior, shows that the EFA of the privacy protection behavior items yielded three distinct factors.
The first factor (Cronbach’s α = 0.87) has six items that describe technical privacy protection behavior (TPPB). Items include turning the smart speaker off, unplugging it, muting it and covering the smart speaker. The second factor (Cronbach’s α = 0.89) has five items that describe data privacy protection behavior (DPPB). The items describe interventions users perform after interacting with the smart speaker for the purpose of protecting their data. Items include reviewing smart speaker recordings, deleting smart speaker recordings, and changing the account password. The third factor (Cronbach’s α = 0.82) has five items that describe social privacy protection behavior (SPPB). Items include speaking quietly around the smart speaker and avoiding talking about sensitive topics around the smart speaker. The factors are moderately correlated with each other: 0.36 (p < 0.001) between TPPB and DPPB, 0.55 (p < 0.001) between TPBB and SPPB, and 0.53 (p < 0.001) between DPPB and SPPB. All three privacy protection behavior factors show low prevalence, with arithmetic mean values of 1.76 (SD = 1.16) for TPPB, 1.64 (SD = 0.92) for DPPB, and 1.44 (SD = 0.88) for SPPB. Thus, users tend to perform privacy protection behaviors to a very limited extent. Among the individual items, turning off the smart speaker when not using it (M = 2.13, SD = 1.42) is reported as the most frequent activity and the only one that has an arithmetic mean larger than 2.00. Very few users report covering the smart speaker microphone (M = 1.31, SD = 0.75), giving misleading information to the smart speaker (1.35, SD = 0.74), and moderating their language around the smart speaker (M = 1.36, SD = 0.78).
EFA was also used to check the dimensionality of the motives. Unlike McLean and Osei-Frimpong (Citation2019), we found four dimensions and had to exclude one item due to low loadings (“I think the Amazon Echo/Google Home could be a friend of mine.”). We could not extract their social attraction dimensions. The two remaining items from that dimension (“I have a good time with the Amazon Echo/Google Home.” and “I would like to spend more time with the Amazon Echo/Google Home.”) fell into the hedonic dimension. Overall, the EFA revealed the following motives dimensions: utilitarian (4 items; Cronbach’s α = 0.89; arithmetic mean across all items = 3.71; SD = 0.94); symbolic (4 items; Cronbach’s α = 0.89; arithmetic mean across all items = 2.02; SD = 1.10); social presence (4 items; Cronbach’s α = 0.87; arithmetic mean across all items = 2.34; SD = 1.21); and hedonic (5 items; Cronbach’s α = 0.82; arithmetic mean across all items = 3.52; SD = 0.94). in the Appendix shows the full scale.
Explanatory results
RQ2 asked how privacy concerns impact privacy protection behaviors among smart speaker users. To address this research question, we report the results of the linear regression analysis. The three privacy protection behavior factors identified in the previous section served as the dependent variables. The seven privacy concern factors served as the key independent variables. Motives, use frequency, social influence, and Internet skills are also included as antecedents, following RQ3-RQ6. In addition, we included demographic variables (age, gender, education, household size, income) as control variables. shows the results of the linear regression.
Table 2. Linear regression of privacy protection behavior on antecedents.
We find overall that privacy concerns have a limited influence on privacy protection behaviors. Although contractor and third party privacy concerns are the most prevalent among respondents, they do not significantly influence privacy protection behaviors. The same is true for stranger privacy concerns. However, of all types, institutional privacy concerns have the strongest impact, with company privacy concerns standing out as the key variable affecting the first two forms of privacy protection behavior (TPPB and DPPB). Among the social privacy concern types, household member privacy concerns form the strongest predictor across the privacy protection behavior types. They positively affect data privacy protection behavior (DPPB) and social privacy protection behavior (SPPB).
RQ3 asked whether motives have a distinct effect on the three privacy protection behavior dimensions. We find that utilitarian motives curb TPPB and SPPB but do not affect DPPB. Symbolic and social presence motives increase SPPB but have no significant effect on TPPB and DPPB. Finally, hedonic motives are weakly but positively associated with TPPB and DPPB. RQ4 asked whether frequency of use has a varied effect on privacy protection behavior. We find that it enhances TPBB and weakens SPPB but does not significantly affect DPPB. RQ5 asked whether social influence has a significant effect on any of the privacy protection behavior dimensions. We find that social influence does not have a significant effect on any privacy protection behavior dimensions. RQ6 asked whether Internet skills affect privacy protection behavior dimensions. We find that Internet skills affect DPPB significantly and positively and have a weak and positive effect on SPPB.
We are able to explain between 23% (TPPB, DPPB) and 31% (SPPB) in the overall variance of the privacy protection factors. Thus, we are better able to predict the social elements of privacy protection behavior than the technical and data-related ones.
Discussion
Summary and implications
Taken together, the descriptive findings point to differentiated concerns and a general absence of privacy protection behaviors among smart speaker users. We find varied levels of concern depending on the source of the privacy risk, though the findings may be impacted by the perceived likeliness of each type of privacy violation. This aligns with the theory of privacy as contextual integrity and the conception of norms of flow in particular (Nissenbaum Citation2004). While users seem relatively unconcerned about privacy in general (Liao et al. Citation2019), third party developers and contractors evoke most concerns. This is in line with research into contextual privacy norms of smart speakers by Apthorpe et al. (Citation2018), who find that information flows to third parties not specifically related to core device features are universally viewed as privacy violations.
Generally, our participants worried more about institutional privacy aspects than social aspects, contradicting research in other contexts such as social media (Raynes-Goldie Citation2010; Young and Quan-Haase Citation2013) but aligning with privacy research on more affective technologies such as mobile dating and social robots (Lutz and Ranzini Citation2017; Lutz and Tamo-Larrieux Citation2020). While the risks of institutional privacy infringement are frequently acknowledged in the media (Day, Turner, and Drozdiak Citation2019a, Citation2019b; Lieber Citation2018), creating visibility and awareness, smart speaker users might not be aware of how other people could abuse the smart speaker for privacy infringements. That household members evoked by far the least privacy concerns also aligns with privacy as contextual integrity theory. Household members usually have strong ties among themselves, often familial, romantic or based on friendship (Office for National Statistics Citation2019). According to Nissenbaum (Citation2004, 141), close relationships have “relatively few general norms of appropriateness”. Thus, most information, including sensitive information, can be disclosed without violating norms of appropriateness, as long as it stays within the confines of the household. The low values of perceived privacy risks from other members can stem from several sources. First, the respondents might be confident that their other household members would not abuse the smart speaker in a way to infringe on their privacy, particularly if that confidence is based on sole access to the user account. Second, they think such an act would not be a violation of contextual integrity norms. Finally, smart speakers tend to be located in shared spaces within households, so that other household members are likely to have heard some audio recorded by the speaker, thus rendering interactions commonplace rather than illicit. However, given reports of Amazon Echo devices being used for domestic abuse (Chang Citation2018), the lower concern levels about household members in our sample does not reflect that a minority of users might have serious and valid domestic privacy concerns.
In terms of privacy protection behavior, we show that such behaviors group into three distinct types: technical, data-related, and social. Technical strategies are quite pragmatic and include turning off the smart speaker, unplugging it, and muting it. While these measures seem straightforward, few users regularly engage in them. For example, more than half of the respondents (51%) never turn off the smart speaker when not using it and almost three-fourths (72%) never turn off the smart speaker when having serious/private conversations. Data-related privacy protection behaviors align with the information privacy literature (Smith, Dinev, and Xu Citation2011) and include reviewing and deleting the information collected by the speaker and aggregated in the Amazon Alexa/Google/Apple user profile. Again, few people engage frequently in such activities and the overall prevalence is lower than for technical privacy protection. Social privacy protection behaviors are the least pronounced. Few respondents moderate their language around the smart speaker and give misleading information to the smart speaker.
Users also seem unconcerned about visitors and guests being recorded. The low prevalence of social privacy protection behavior points to domestication and habituation, where smart speakers become a normal part of everyday life and the overarching surveillance assemblage is neglected. However, our study only includes users, who might have lowered concerns and a more pragmatic approach to privacy than non-users (Liao et al. Citation2019).
The regression showed how the privacy concern types had a differentiated impact on the three privacy protection behavior dimensions. Across all seven concern types, company privacy concerns were the strongest predictor, affecting all three protection behavior dimensions significantly and positively. This could indicate that individuals see the companies behind the smart speaker (Amazon, Google, etc.) as main threats when it comes to privacy and use the protection behaviors to safeguard themselves. Users might recognize that data from smart speakers is complemented with other data these companies collect as part of their business models (Pridmore et al. Citation2019).
Household privacy concerns had a significant and positive effect on two of the three protection behaviors (DPPB, SPPB). Thus, smart speaker users do transfer their social privacy concerns to a certain extent into behavior, but they do so more in data-related and social ways than in technical waysFootnote2. Government privacy concerns had a negative effect on DPPB, showing that the more smart speaker users are concerned about the government using data collected by the smart speaker, the less they engage in protecting their data. This could point to privacy pragmatism (Elueze and Quaan-Haase Citation2018; Westin Citation2000), apathy (Hargittai and Marwick Citation2016), fatigue (Choi, Park, and Jung Citation2018), or even cynicism (Hoffmann, Lutz, and Ranzini Citation2016; Lutz, Hoffmann, and Ranzini Citation2020).
Use motives (RQ3), use frequency (RQ4), social influence (RQ5) and Internet skills (RQ6) all had distinct effects on the three types of privacy protection behavior. Regarding RQ3, social presence motivations fostered SPPB, while hedonic motivations weakly enhanced TPPB and DPPB. Stressing the social and hedonic roles of smart speakers more strongly could make users reflect on their interaction and ramp up their privacy protection. Human-robot interaction research has shown how robots can be perceived as eerie, and thus unlikable, when they are too similar to humans (Mori, MacDorman, and Kageki Citation2012; Wang, Lilienfeld, and Rochat Citation2015). However, less evidence exists on disembodied (see Ciechanowski et al. Citation2019 for an exception on chatbots) and non-robot smart technologies, such as smart speakers, and the uncanny valley. Our findings suggest that users who score higher on social presence (measured in a way that captures the perceived similarity between the smart speaker and a human) have higher levels of social privacy protection. While we cannot say whether this is due to an uncanny valley effect, such a tendency seems plausible. Privacy advocates could therefore focus on hyper-social and hyper-entertaining features in awareness campaigns that sensitize users about the privacy risks of this technology.
Frequency of use showed an inconsistent effect across the three privacy protection behaviors (RQ4), increasing TPBB, lowering SPPB, but not affecting DPPB significantly. This could have to do with the type of activities captured by TPPB vs. SPPB. While TPBB involves engagement with the smart speaker itself (e.g., turning it off, muting it), SPPB is more about the (interaction) environment and aspects of avoidance. Through frequent interaction with the smart speaker, users habituate the device and integrate it into their everyday routines (Brause and Blank Citation2020), thus potentially forgetting to modify their interaction with other people when around it. At the same time, the frequent interaction might sometimes afford technical interactions. The absence of a significant effect of social influence (RQ5) suggests that privacy protection behavior is not much seen as a socially relevant or present topic, potentially due to a lack of awareness or interest. Future research should study the social embeddedness of privacy protection behavior (or its lack) in more depth. Finally, Internet skills had the strongest effect on DPPB, while it affected the two other privacy protection behaviors less (RQ6). This could be explained by the fact that DPPB is more connected to digital platform and general Internet use, as it involves profile management. Fostering Internet skills through literacy training could thus lead to more protected smart speaker users.
Limitations
Our study has several limitations. First, we collected cross-sectional rather than longitudinal data, preventing strong causal claims. Smart speakers are an emerging technology and increased awareness about the benefits and risks could change people’s privacy protection behavior. Therefore, we encourage future research to study privacy concerns and privacy protection behavior over time, including additional factors such as media coverage (Von Pape, Trepte, and Mothes Citation2017). Second, our data focuses on one country and extant research has shown that countries differ in their privacy attitudes and behavior (Trepte et al. Citation2017). Future research should adopt a cross-cultural approach to compare privacy attitudes and behavior relating to smart speakers. Third, we had to rely on self-reported data to measure privacy protection behavior. Trace data and actual interaction data with the smart speaker would offer a richer picture of privacy behavior (Bentley et al. Citation2018; Porcheron et al. Citation2018). However, such data is subject to confidentiality and ethical concerns could be raised. Therefore, user-focused qualitative research or action research could strike a balance between confidentiality and richness, adding potentially unseen perspectives. Fourth and finally, the way our privacy concerns items were worded do not factor in aspects of likelihood. For example, it could be that the participants were relatively unconcerned about third party hacking because they thought this was unlikely. Future research could disentangle this more and differentiate between perceived likelihood and perceived severity of a privacy risk.
Conclusion
Relying on human-machine communication literature and privacy as contextual integrity, we developed a differentiated typology of privacy concerns in the context of smart speakers. The features of this technology, particularly the voice-enabled interaction modalities, create social presence between the user and the speaker. This could lead to a neglect of privacy – a key concern about this new technology. Our empirical analyses showed that users’ privacy concerns about smart speakers are generally quite low. However, user concerns vary depending on the source of the concern. Institutional actors, such as third party companies developing apps and the manufacturers of the smart speaker (Amazon, Google), evoked more concerns than social actors such as fellow household members. Privacy as contextual integrity (Nissenbaum Citation2010, Citation2019) offers an appropriate theoretical lens to explain the findings, demonstrating how variations in the recipient of data, with all other parameters being equal, can lead to perceived norm violations.
We also found that privacy protection behavior is rare. Users refrain from engaging in technical, data-related and social privacy protection behavior on a regular basis. Taken together, these findings align with recent discourses on privacy apathy (Hargittai and Marwick Citation2016), privacy cynicism (Hoffmann, Lutz, and Ranzini Citation2016; Lutz, Hoffmann, and Ranzini Citation2020) and privacy apathy (Choi, Park, and Jung Citation2018). Fostering Internet skills and stressing the social presence aspects of smart speakers in awareness campaigns and media stories, potentially by evoking an uncanny valley effect, could sensitize users about privacy aspects and increase privacy protection.
Declaration of interest statement
The author(s) declared no potential conflicts of interest with respect to the research, authorship, and/or publication of this article.
utis_a_1897914_sm1205.docx
Download MS Word (34 KB)Acknowledgements
The author(s) disclosed receipt of the following financial support for the research, authorship, and/or publication of this article: The research received funding by the Research Council of Norway within grant agreement 275347 “Future Ways of Working in the Digital Economy”. The authors would like to thank Shruthi Velidi for her support in the early stages of the project.
Additional information
Funding
Notes
1 Common criticisms of the privacy calculus include the lack of consideration of privacy literacy (i.e., users might not be aware of privacy risks or unable to assess the seriousness of a privacy threat), the neglect of habitual, emotional, and situational factors, as well as methodological issues (Dienlin and Metzger Citation2016; Hoffmann, Lutz, and Ranzini Citation2016). Kokolakis (Citation2017) describes alternative approaches such as social theory-based studies, which rely on sociological theories such as structuration theory (Zafeiropoulou et al. Citation2013) or Tönnies’s distinction of gemeinschaft and gesellschaft (Lutz and Strathoff Citation2014), cognitive biases and heuristics (Baek, Kim, and Bae Citation2014), as well as quantum theory homomorphism.
2 We ran a linear regression analysis, where we calculated a summative index of the first three privacy concern dimensions (device, household member, stranger) and of the last four privacy concern dimensions (company, contractor, third party, government) and then used these indices as independent variables. While the summative index of the first three dimensions – capturing social privacy concerns broadly speaking – had a significant and positive effect on all three protection behaviors (TPPB, DPPB, SPPB), the summative index of the last four dimensions – capturing institutional privacy concerns broadly speaking – was insignificant across all three regressions.
References
- Ajzen, I. 1991. The theory of planned behavior. Organizational Behavior and Human Decision Processes 50 (2):179–211. doi: 10.1016/0749-5978(91)90020-T.
- Alepis, E., and C. Patsakis. 2017. Monkey says, Monkey does: Security and privacy on voice assistants. IEEE Access 5:17841–51. doi: 10.1109/ACCESS.2017.2747626.
- Apthorpe, N., Y. Shvartzshnaider, A. Mathur, D. Reisman, and N. Feamster. 2018. Discovering smart home Internet of things privacy norms using contextual integrity. In Proceedings of the ACM on interactive, mobile, wearable and ubiquitous technologies, 1–23. New York: ACM. doi: 10.1145/3214262.
- Baek, Y. M., E.-M. Kim, and Y. Bae. 2014. My privacy is okay, but theirs is endangered: Why comparative optimism matters in online privacy concerns. Computers in Human Behavior 31:48–56. doi: 10.1016/j.chb.2013.10.010.
- Barnes, S. B. 2006. A privacy paradox: Social networking in the United States. First Monday 11 (9):n.p. doi: 10.5210/fm.v11i9.1394.
- Baruh, L., E. Secinti, and Z. Cemalcilar. 2017. Online privacy concerns and privacy management: A meta-analytical review. Journal of Communication 67 (1):26–53. doi: 10.1111/jcom.12276.
- Bentley, F., C. Luvogt, M. Silverman, R. Wirasinghe, B. White, and D. Lottridge. 2018. Understanding the long-term use of smart speaker assistants. Proceedings of the ACM on Interactive, Mobile, Wearable and Ubiquitous Technologies 2 (3):1–91.24. Accessed February 14, 2021. https://dl-acm-org.proxyiub.uits.iu.edu/doi/pdf/10.1145/3264901. doi: 10.1145/3264901.
- Brause, S. R., and G. Blank. 2020. Externalized domestication: Smart speaker assistants, networks and domestication theory. Information Communication, & Society 23 (5):751–63. doi: 10.1080/1369118X.2020.1713845.
- Brown, J. D. 2009a. Principal components analysis and exploratory factor analysis - Definitions, differences, and choices. Shiken: JALT Testing & Evaluation SIG Newsletter 13 (1):26–30.
- Brown, J. D. 2009b. Choosing the right type of rotation in PCA and EFA. Shiken: JALT Testing & Evaluation SIG Newsletter 13 (1):20–5.
- Brown, J. D. 2009c. Choosing the right number of components or factors in PCA and EFA. Shiken: JALT Testing & Evaluation SIG Newsletter 13 (1):19–23.
- Büchi, M., E. Fosch-Villaronga, C. Lutz, A. Tamò-Larrieux, S. Velidi, and S. Viljoen. 2020. The chilling effects of algorithmic profiling: Mapping the issues. Computer Law & Security Review 36:105367–15. doi: 10.1016/j.clsr.2019.105367.
- Büchi, M., N. Just, and M. Latzer. 2017. Caring is not enough: The importance of Internet skills for online privacy protection. Information, Communication & Society 20 (8):1261–78. doi: 10.1080/1369118X.2016.1229001.
- Bugeja, J., A. Jacobsson, A., and P. Davidsson. 2016. On privacy and security challenges in smart connected homes. In 2016 European Intelligence and Security Informatics Conference (EISIC), 172–175. New York: IEEE. doi:10.1109/EISIC.2016.044.
- Buhr, S. 2016. An Amazon Echo may be the key to solving a murder case. TechCrunch, December 27. Accessed March 21, 2020. http://tcrn.ch/2iC6frz
- Chang, L. 2018. Smart home devices are being used in domestic abuse, report finds. Digital Trends, July 9. Accessed March 21, 2020. https://www.digitaltrends.com/home/smart-home-domestic-abuse/
- Choi, H., J. Park, and Y. Jung. 2018. The role of privacy fatigue in online privacy behavior. Computers in Human Behavior 81:42–51. doi: 10.1016/j.chb.2017.12.001.
- Chung, H., J. Park, and S. Lee. 2017. Digital forensic approaches for Amazon Alexa ecosystem. Digital Investigation 22:S15–S25. doi: 10.1016/j.diin.2017.06.010.
- Ciechanowski, L., A. Przegalinska, M. Magnuski, and P. Gloor. 2019. In the shades of the uncanny valley: An experimental study of human-chatbot interaction. Future Generation Computer Systems 92:539–48. doi: 10.1016/j.future.2018.01.055.
- Day, M., G. Turner, and N. Drozdiak. 2019a. Amazon workers are listening to what you tell Alexa. Bloomberg.com, April 11. Accessed March 21, 2020. https://www.bloomberg.com/news/articles/2019-04-10/is-anyone-listening-to-you-on-alexa-a-global-team-reviews-audio
- Day, M., G. Turner, and N. Drozdiak. 2019b. Amazon’s Alexa team can access users’ home addresses. Bloomberg.com, April 24. Accessed March 21, 2020. https://www.bloomberg.com/news/articles/2019-04-24/amazon-s-alexa-reviewers-can-access-customers-home-addresses
- Dienlin, T., and M. J. Metzger. 2016. An extended privacy calculus model for SNSs: Analyzing self-disclosure and self-withdrawal in a representative US sample. Journal of Computer-Mediated Communication 21 (5):368–83. doi: 10.1111/jcc4.12163.
- Dinev, T., and P. Hart. 2006. An extended privacy calculus model for e-commerce transactions. Information Systems Research 17 (1):61–80. doi: 10.1287/isre.1060.0080.
- Elueze, I., and A. Quan-Haase. 2018. Privacy attitudes and concerns in the digital lives of older adults: Westin’s privacy attitude typology revisited. American Behavioral Scientist 62 (10):1372–91. doi: 10.1177/0002764218787026.
- Estes, A. C. 2018. Your worst Alexa nightmares are coming true. Gizmodo.com, May 25. Accessed March 21, 2020. https://gizmodo.com/your-worst-alexa-nightmares-are-coming-true-1826327301
- Estes, A. C. 2019. The terrible truth about Alexa. Gizmodo.com, April 27. Accessed March 21, 2020. https://gizmodo.com/the-terrible-truth-about-alexa-1834075404
- Evans, S., K. Pearce, J. Vitak, and J. Treem. 2017. Explicating affordances: A conceptual framework for understanding affordances in communication research. Journal of Computer-Mediated Communication 22 (1):35–52. doi: 10.1111/jcc4.12180.
- Feiner, L. 2019. Apple’s smart speaker is struggling against rivals from Amazon and Google. CNBC.com, February 5. Accessed March 21, 2020. https://www.cnbc.com/2019/02/05/apple-homepod-smart-speaker-market-share.html
- Feldman, R. 2018. Smart speaker ownership doubles in six months. YouGov.com, April 19. Accessed March 21, 2020. https://yougov.co.uk/topics/politics/articles-reports/2018/04/19/smart-speaker-ownership-doubles-six-months
- Feng, H., K. Fawaz, and K. G. Shin. 2017. Continuous authentication for voice assistants. In Proceedings of the 23rd Annual International Conference on Mobile Computing and Networking, 343–55. New York: ACM Press. doi: 10.1145/3117811.3117823.
- Foehr, J., and C. C. Germelmann. 2020. Alexa, can I trust you? Exploring consumer paths to trust in smart voice-interaction technologies. Journal of the Association for Consumer Research 5 (2):181–205. doi: 10.1086/707731.
- Geeng, C., and F. Roesner. 2019. Who’s in control? Interactions in multi-user smart homes. In Proceedings of the 2019 CHI Conference on Human Factors in Computing Systems, 1–13. New York: ACM.
- Guzman, A. L. 2017. Making AI safe for humans: A conversation with Siri. In Socialbots and their friends, ed. R. Gehl and M. Bakardjieva, 85–101. London: Routledge.
- Guzman, A. L. 2019. Voices in and of the machine: Source orientation toward mobile virtual assistants. Computers in Human Behavior 90:343–50. doi: 10.1016/j.chb.2018.08.009.
- Guzman, A. L. 2020. Ontological boundaries between humans and computers and the implications for human-machine communication. Human-Machine Communication 1:37–54. doi: 10.30658/hmc.1.3.
- Hargittai, E. 2005. Survey measures of web-oriented digital literacy. Social Science Computer Review 23 (3):371–9. doi: 10.1177/0894439305275911.
- Hargittai, E. 2009. An update on survey measures of web-oriented digital literacy. Social Science Computer Review 27 (1):130–7. doi: 10.1177/0894439308318213.
- Hargittai, E., and A. Marwick. 2016. What can I really do?” Explaining the privacy paradox with online apathy. International Journal of Communication 10:3737–57.
- Hildebrandt, M. 2008. Defining profiling: A new type of knowledge. In Profiling the European citizen: Cross-disciplinary perspectives, ed. M. Hildebrandt and S. Gutwirth, 17–30. Berlin: Springer.
- Hoffmann, C. P., C. Lutz, and G. Ranzini. 2016. Privacy cynicism: A new approach to the privacy paradox. Cyberpsychology: Journal of Psychosocial Research on Cyberspace 10 (4):7. doi: 10.5817/CP2016-4-7.
- Hoy, M. B. 2018. Alexa, Siri, Cortana, and more: An introduction to voice assistants. Medical Reference Services Quarterly 37 (1):81–8. doi: 10.1080/02763869.2018.1404391.
- Ingber, S. 2018. Amazon customer receives 1,700 audio files of a stranger who used Alexa. NPR.org, December 20. Accessed March 21, 2020. https://www.npr.org/2018/12/20/678631013/amazon-customer-receives-1-700-audio-files-of-a-stranger-who-used-alexa
- Kaiser, H. F. 1974. An index of factorial simplicity. Psychometrika 39 (1):31–6. doi: 10.1007/BF02291575.
- Kokolakis, S. 2017. Privacy attitudes and privacy behaviour: A review of current research on the privacy paradox phenomenon. Computers & Security 64:122–34. doi: 10.1016/j.cose.2015.07.002.
- Lau, J., B. Zimmerman, and F. Schaub. 2018. Alexa, are you listening? Privacy perceptions, concerns and privacy-seeking behaviors with smart speakers. In Proceedings of the ACM on Human-Computer Interaction, 102.1–.31. Accessed February 14, 2021. https://dl-acm-org.proxyiub.uits.iu.edu/doi/pdf/10.1145/3274371.
- Liao, Y., J. Vitak, P. Kumar, M. Zimmer, and K. Kritikos. 2019. Understanding the role of privacy and trust in intelligent personal assistant adoption. In Information in contemporary society: 14th International Conference, iConference 2019, Washington, DC, March 31-April 3, Proceedings, eds. N. G. Taylor, C. Christian-Lamb, M. H. Martin, and B. Nardi, 102–13. Berlin: Springer.
- Lieber, C. 2018. Amazon’s Alexa might be a key witness in a murder case. Vox.com, November 12. Accessed March 21, 2020. https://www.vox.com/the-goods/2018/11/12/18089090/amazon-echo-alexa-smart-speaker-privacy-data
- Lopez-Neira, I., T. Patel, S. Parkin, G. Danezis, and L. Tanczer. 2019. Internet of Things”: How abuse is getting smarter. Safe: The Domestic Abuse Quarterly 63:22–6.
- Luger, E., and A. Sellen. 2016. Like having a really bad PA: The gulf between user expectation and experience of conversational agents. In Proceedings of the 2016 CHI Conference on Human Factors in Computing Systems, 5286–97. New York: ACM Press.
- Lutz, C., and A. Tamò-Larrieux. 2020. The robot privacy paradox: Understanding how privacy concerns shape intentions to use social robots. Human-Machine Communication 1 (1):87–111. doi: 10.30658/hmc.1.6.
- Lutz, C., and G. Ranzini. 2017. Where dating meets data: Investigating social and institutional privacy concerns on tinder. Social Media + Society 3 (1):1–12.
- Lutz, C., C. P. Hoffmann, and G. Ranzini. 2020. Data capitalism and the user: An exploration of privacy cynicism in Germany. New Media & Society 22 (7):1168–87. doi: 10.1177/1461444820912544.
- Lutz, C., and P. Strathoff. 2014. Privacy concerns and online behavior – Not so paradoxical after all? Viewing the privacy paradox through different theoretical lenses. In Multinationale unternehmen und Institutionen im wandel: Herausforderungen für wirtschaft, recht und gesellschaft, eds S. Brändli, R. Schister, and A. Tamò, 81–99. Bern: Stämpfli.
- Malkin, N., J. Deatrick, A. Tong, P. Wijesekera, S. Egelman, and D. Wagner. 2019. Privacy attitudes of smart speaker users. Proceedings on Privacy Enhancing Technologies 2019 (4):250–71. doi: 10.2478/popets-2019-0068.
- Manikonda, L., A. Deotale, and S. Kambhampati. 2018. What's up with privacy? User preferences and privacy concerns in intelligent personal assistants. In Proceedings of the 2018 AAAI/ACM Conference on AI, Ethics, and Society, 229–35. New York: ACM Press.
- Martin, K. 2012. Diminished or just different? A factorial vignette study of privacy as a social contract. Journal of Business Ethics 111 (4):519–39. doi: 10.1007/s10551-012-1215-8.
- Martin, K. 2016a. Understanding privacy online: Development of a social contract approach to privacy. Journal of Business Ethics 137 (3):551–69. doi: 10.1007/s10551-015-2565-9.
- Martin, K. 2016b. Data aggregators, consumer data, and responsibility online: Who is tracking consumers online and should they stop? The Information Society 32 (1):51–63. doi: 10.1080/01972243.2015.1107166.
- Martin, K., and K. Shilton. 2016. Putting mobile application privacy in context: An empirical study of user privacy expectations for mobile devices. The Information Society 32 (3):200–16. doi: 10.1080/01972243.2016.1153012.
- McLean, G., K. Osei-Frimpong. 2019. Hey Alexa… examine the variables influencing the use of artificial intelligent in-home voice assistants. Computers in Human Behavior 99:28–37. doi: 10.1016/j.chb.2019.05.009.
- Mori, M.,. K. MacDorman, and N. Kageki. 2012. The uncanny valley (trans. K. F. MacDorman, and N. Kageki). IEEE Robotics & Automation Magazine 19 (2):98–100. doi: 10.1109/MRA.2012.2192811.
- Nass, C., and Y. Moon. 2000. Machines and mindlessness: Social responses to computers. Journal of Social Issues 56 (1):81–103. doi: 10.1111/0022-4537.00153.
- Nass, C., J. Steuer, and E. R. Tauber. 1994. Computers are social actors. In Proceedings of the SIGCHI Conference on Human Factors in Computing Systems, 72–8. New York: ACM Press.
- Nissenbaum, H. 2004. Privacy as contextual integrity. Washington Law Review 79:101–39.
- Nissenbaum, H. 2010. Privacy in context: Technology, policy, and the integrity of social life. Stanford, CA: Stanford University Press.
- Nissenbaum, H. 2019. Contextual integrity up and down the data food chain. Theoretical Inquiries in Law 20 (1):221–56. doi: 10.1515/til-2019-0008.
- Ofcom. 2019. Media nations: UK 2019 (Report No.2). Accessed March 21, 2020. https://www.ofcom.org.uk/__data/assets/pdf_file/0019/160714/media-nations-2019-uk-report.pdf
- Office for National Statistics. 2019. Families and households in the UK: 2019. Accessed June 18, 2020. https://www.ons.gov.uk/peoplepopulationandcommunity/birthsdeathsandmarriages/families/bulletins/familiesandhouseholds/2019
- Palan, S., and C. Schitter. 2018. Prolific.ac: A subject pool for online experiments. Journal of Behavioral and Experimental Finance 17:22–7. doi: 10.1016/j.jbef.2017.12.004.
- Pfadenhauer, M. 2015. The contemporary appeal of artificial companions: Social robots as vehicles to cultural worlds of experience. The Information Society 31 (3):284–93. doi: 10.1080/01972243.2015.1020213.
- Porcheron, M., J. E. Fischer, S. Reeves, and S. Sharples. 2018. Voice interfaces in everyday life. In Proceedings of the 2018 CHI Conference on Human Factors in Computing Systems, 1–12. New York: ACM Press. doi: 10.1145/3173574.3174214.
- Pridmore, J., M. Zimmer, J. Vitak, A. Mols, D. Trottier, P. C. Kumar, and Y. Liao. 2019. Intelligent personal assistants and the intercultural negotiations of dataveillance in platformed households. Surveillance & Society 17 (1/2):125–31. doi: 10.24908/ss.v17i1/2.12936.
- Quandt, T., and T. von Pape. 2010. Living in the mediatope: A multimethod study on the evolution of media technologies in the domestic environment. The Information Society 26 (5):330–45. doi: 10.1080/01972243.2010.511557.
- Raynes-Goldie, K. 2010. Aliases, creeping, and wall cleaning: Understanding privacy in the age of Facebook. First Monday 15 (1):n.p. doi: 10.5210/fm.v15i1.2775.
- Reeves, B., and C. I. Nass. 1996. The media equation: How people treat computers, television, and new media like real people and places. Stanford, CA: CSLI Publications.
- Smith, H. J., T. Dinev, and H. Xu. 2011. Information privacy research: An interdisciplinary review. MIS Quarterly 35 (4):989–1016. doi: 10.2307/41409970.
- Smith, K. T. 2020. Marketing via smart speakers: What should Alexa say? Journal of Strategic Marketing 28 (4):350–65. doi: 10.1080/0965254X.2018.1541924.
- Trepte, S., L. Reinecke, N. B. Ellison, O. Quiring, M. Z. Yao, and M. Ziegele. 2017. A cross-cultural perspective on the privacy calculus. Social Media + Society 3 (1):205630511668803–13. doi: 10.1177/2056305116688035.
- Turow, J., M. Hennessy, and N. Draper. 2018. Persistent misperceptions: Americans’ misplaced confidence in privacy policies, 2003–2015. Journal of Broadcasting & Electronic Media 62 (3):461–78. doi: 10.1080/08838151.2018.1451867.
- Venkatesh, V.,. M. G. Morris, G. B. Davis, and F. D. Davis. 2003. User acceptance of information technology: Toward a unified view. MIS Quarterly 27 (3):425–78. doi: 10.2307/30036540.
- Von Pape, T., S. Trepte, and C. Mothes. 2017. Privacy by disaster? Press coverage of privacy and digital technology. European Journal of Communication 32 (3):189–207. doi: 10.1177/0267323117689994.
- Wamsley, L. 2018. Amazon Echo recorded and sent couple’s conversation: All without their knowledge. NPR, May 25. Accessed March 21, 2020. https://www.npr.org/sections/thetwo-way/2018/05/25/614470096/amazon-echo-recorded-and-sent-couples-conversation-all-without-their-knowledge
- Wang, S., S. O. Lilienfeld, and P. Rochat. 2015. The uncanny valley: Existence and explanations. Review of General Psychology 19 (4):393–407. doi: 10.1037/gpr0000056.
- Westin, A. F. 2000. Intrusions. Public Perspective 11 (6):8–11.
- Young, A. L., and A. Quan-Haase. 2013. Privacy protection strategies on Facebook: The Internet privacy paradox revisited. Information, Communication & Society 16 (4):479–500. doi: 10.1080/1369118X.2013.777757.
- Zafeiropoulou, A. M., D. E. Millard, C. Webber, and K. O’Hara. 2013. Unpicking the privacy paradox: Can structuration theory help to explain location-based privacy decisions? In Proceedings of the 5th Annual ACM Web Science Conference, 463–72. New York: ACM.
- Zheng, S., N. Apthorpe, M. Chetty, and N. Feamster. 2018. User perceptions of smart home IoT privacy. In Proceedings of the ACM on Human-Computer Interaction, 1–20. New York: ACM. doi: 10.1145/3274469.
Appendix
Table A1. Questionnaire: Privacy concerns items, motives and social influence.