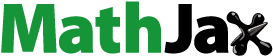
ABSTRACT
This paper aims to analyze the relationship between innovation and per capita economic growth over the long-run for 12 Latin American countries for the period 1996–2015. This study uses six different indicators of innovation. Using Granger causality test, the study finds the presence of unidirectional and bidirectional causalities between innovation and per capita economic growth. These results vary. Latin America is a diverse region, depending upon the types of innovation indicators that we use in the empirical investigation process. It is important to note that all these innovation indicators are considerably linked with per capita economic growth.
1. Introduction
There is an extensive and long-standing support that innovation leads to economic growth (Beneki, Giannias, & Moustakas, Citation2012; Segerstrom, Citation1991; Verspagen, Citation2005; Wong, Ho, & Autio, Citation2005). This study is based on Maradana et al. (Citation2017), and uses six different indicators of innovation and examines their relation to long-run economic growth:
Patents-residents
Patents-non-residents
Research and development expenditure
Researchers in research and development activities
High-technology exports
Scientific and technical journal articles
Latin America lags in term of economic growth and innovation:
Despite the recent, rapid economic growth experienced by several Latin American countries during the commodity boom, the fall in commodity export prices, including oil, coal, other minerals and agricultural products, has underscored the many competitiveness challenges required for new growth sectors to emerge. Improvements could be made in many areas, and the skills and innovation gap ranks high on the list. Other areas for improvement include education, on-the-job training, scientific and technological investments by both government and business, and enhancing the innovation environment. (World Economic Forum, Citation2008)
Some other regions, such as Eastern Europe or Asia, have experienced a recent economic growth, especially in the East Asian region (Hu, Citation2015); some authors attribute this growth to the process of turning imitation to innovation (Hobday, Citation1995; Mathews, Citation1995).
According to Hu (Citation2015),
The economies that have made the leap from imitation to innovation in recent history are Japan, Korea, and Taiwan. Certain sectors and regions of mainland China are also in the process of accomplishing the same. A number of lessons emerge from this literature. (P.21)
2. Related literature
We based the relationship between innovation and growth in the theory of endogenous growth addressed by Grossman and Helpman (Citation1994). Literature specifies that innovation and new technology have emerged as one of the primary driving force of growth. Robert Solow described this perspective in his so-called “neoclassical growth theory” (Solow, Citation1979), Solow based his theory in standard neoclassical assumptions, such as perfect competition, maximizing behavior, no externalities, positive and decreasing marginal products and absence of scale economies. After this work, Solow added and exogenous term, labeled “technological progress” to allow for long-run growth in GDP per capita.
Several works such as Aghion (Citation1992) and Maradana et al. (Citation2017) have also found technological innovation to be the main determinant of growth. In a research made by Mendoza (Citation2017), the Mexican states that had a level of technological innovation in 1995, are those states that had faster growth. This result highlights not only the role of technological innovation as a growth factor economic but also it implies the fact that those regions that carried out greater activity of technological innovation are the regions that had a greater economic growth. That is, technological innovation has encouraged the economic divergence.
Where does innovation come from? Traditionally scholars supported the idea of public sector. In this regard, Schumpeter (Citation1961) suggests that technological advance is the result of a never-ending cycle of incoming innovative firms. However, some years later, Schumpeter (Citation1975) moves his position from only firms to public policies. Martin and Scott (Citation2000) imply the need to establish a long-term institutional framework for the support of basic research, generic-enabling research, and commercialization. The extent to which support should be directed to each area will vary with the sources of sectoral innovation market failure.
Rudimentary efforts are also made to identify the dimensions of firms in terms of their resources rather than in terms of their products that can be sources of advantage, and to explain how combinations of competences and resources can be developed, deployed, and protected. Teece, Pisano, and Shuen (Citation1997) refer to this as the “dynamic capabilities” approach in order to stress exploiting existing internal and external firm specific competences to address changing environments. Bureaucracy and lack of investment might be an obstacle to accomplish the dynamic capabilities approach, in particular, Fuentes and Mendoza (2003) attributed to public investment in infrastructure, an important role as a brake on regional inequality. They find that in the period of convergence 1980–1985, the infrastructure Social status represents an important factor in reducing regional differences, not so in the case of the economic infrastructure. In the period of 1985–1998 divergence, find that the infrastructure variables are not significant, which is consistent with the change in the functions of the State, which now it encourages more the element of private investment.
Scientific and technological knowledge and the ability to innovate are elements that contribute to increase the productivity and the standard of living of nations. International experience shows that developing countries rely increasingly on their ability to generate, absorb and transfer knowledge because that way goods and services with a higher added value enrich their development capabilities and position the nation in a global environment that is increasingly interconnected and competitive (CONACyT, Citation2014).
The government’s role in economic development is decisive; Guisan (Citation2017) appoints that R + D are important for economic development and quality of life, both in Humanities and Social Sciences (H + S) and in Natural Sciences and Engineering (NSE).
In the Latin American region, some countries have not achieved a global integration, for example, Mexico’s exports dependency on the United States market and the relative importance of such exports in Mexico’s overall economic performance makes it highly susceptible to U.S. economy fluctuations (Villareal, Citation2010).
2.1. A. An outline of innovation in the Latin American countries
Today, Latin America regions face important challenges to foster sustained economic growth, reduce poverty and improve the living standards of their population. In this context, promoting progress of innovation in the regions becomes a key priority (Olavarrieta & Villena, Citation2014).
The Latin American economic integration process has been developed throughout the twentieth century making use of the following strategies: regional economic research, financing agencies, and trade agreements. It is important to note that research on regional economic issues and financing agencies are mainly funded by the United States, and that even though most of the countries are part of a trade agreement, the intraregional trade is not significant in any case (Olarte, Citation2016).
According to Olavarrieta and Villena (Citation2014), Latin America lags behind the more advanced economies in terms of innovative activities. This is not only at the output level, patent applications, high-technology exports (percentage of manufactured exports) and scientific and technical journal articles, including business research, but also at the input level, R&D expenditure (as percentage of the GDP) and researchers in R&D (per million people). Hence, it is not expected that this scenario will dramatically change at least in the short run.
Previous works have addressed innovation–growth issues in two ways: The Regional disparities of innovation activities and economic growth in countries and the causality between both issues (see Maradana 2017; Pradhan, Arvin, Hall, & Nair, Citation2016).
The six variables of innovation we use are as follows:
PAR: Number of patents filed by residents measured per thousand of population;
PAN: Number of patents filed by non-residents measured per thousand of population;
RDE: Research and development expenditure measured as a percentage of real GDP;
RRD: Researchers in research and development activities measured per thousand population;
HTE: High-technology exports measured as percentage of real domestic product; and
STJ: Scientific and technical journals articles measured per thousand population.
shows the definition of the variables. and provide a general status of innovation indicators in the Latin American countries. The status of innovation regarding each indicator (PAR, PAN, RDE, RRD, HTE, and STJ) in the Latin American countries are examined in three different time periods from 1996 to 2015. These three periods are P1: 1996–2007, P2: 2007–2013 and P3: 1996–2013. In order to have an initial understanding of the variable, in we present the descriptive statistics of these innovation variables, data for PAR, PAN, RDE, RRD, HTE, and STJ from World Development Indicators of World Bank 2016.
Table 1. Definition of variables.
Table 2. Trend of innovation (per thousand population) in Latin American countries.
Table 3. Trend of innovation (per thousand population) in Latin American countries.
4. Methods of study
The methodology chosen in this study is based on Granger (Citation1969). Other causality testing methods reported in the literature include the test proposed by Sim (Citation1972) and the procedure suggested by Pierce and Haugh (Citation1977). We empirically test the relationship between innovation and per capita economic growth. Specifically, the causality between innovation and per capita economic growth can be addressed in four different ways: supply-leading hypothesis of innovation–growth nexus, demand-following hypothesis of innovation–growth nexus, feedback hypothesis of innovation–growth nexus, and neutrality hypothesis of innovation–growth nexus.
We intend to test the following hypotheses:
H°₁A: Innovation activities do not Granger-cause per capita economic growth.
H1₁A: Innovation activities Granger-cause per capita economic growth.
H°₁B: Per capita economic growth does not Granger-cause innovation activities.
H1₁B: Per capita economic growth Granger-causes innovation activities.
This study considers 12 of the largest Latin American economies according to the World Bank: Argentina, Brazil, Chile, Colombia, Costa Rica, Ecuador, Guatemala, Mexico, Panama, Paraguay, Peru, and Uruguay; we have omitted Venezuela due to the lack of data available. We use the GDP as a reference for our variables. The empirical investigation considers annual data over the period 1996 to 2015 which was obtained from the World Development Indicators of the World Bank. The results presented in for every Latin American country chosen, indicate that according to the unit root test innovation and per capita economic growth some countries are completely non-stationary, but only at the first difference following the results of previous works.
In the causality between innovation and per capita economic growth, GDP per capita economic growth and innovation are used as a proxy for PAR, PAN, RDE, RRD, HTE, and STJ.
Model 1: For individual country analysis
The testable hypotheses are
The testable hypotheses are
where ECT is the error correction term, which is derived from the long-run co-integration equation; p and q are the lag lengths for the estimation; Δ is the first difference operator; and ε1t and ε2t are the independent and normally distributed random error with a zero mean and a finite heterogeneous variance.
Model 2: For panel data analysis
The testable hypotheses are
The testable hypotheses are
where i = 1,2, …,N represents a country in the panel, t = 1,2, …, and T represents the year in the panel.
The choice of a particular model depends upon the order of integration and the co-integrating relationship between innovation and per capita economic growth. Therefore, we follow Maradana (2017) and we first deploy unit root test and co-integration test, both at individual country and the panel setting, for knowing the order of integration and the presence of co-integrating relationship between innovation and per capita economic growth.
The Augmented Dickey Fuller (ADF) unit root test (Dickey and Fuller Citation1981) are used for individual country analysis, while the ADF-fisher chi-square panel unit root test is used for the panel setting. On the other hand, Johansen co-integration test is used for individual country analysis, while Fisher/Maddala co-integration test is used in the panel setting.
5. Results and discussion
5.1. Case 1: between patents-residents (PAR) and per capita economic growth (GDP)
In Brazil, we find unidirectional causality from per capita economic growth to innovation (PAR≤GDP). For countries like Colombia, Costa Rica, Ecuador, Mexico, Panama, Paraguay and Uruguay, we find bidirectional causality between innovation and per capita economic growth (PAR≤>GDP). While in Argentina, Chile, Guatemala and Peru, per capita economic growth does not Granger-cause innovation (PAR<#>GDP)
5.2. Case 2: between patents-non-residents (PAN) and per capita economic growth (GDP)
For countries like Argentina, Costa Rica, Ecuador, Guatemala, Mexico, Panama, Paraguay, Peru and Uruguay, we find bidirectional causality between innovation and per capita economic growth (PAN≤>GDP). Chile shows a unidirectional causality from per capita economic growth to innovation (GDP≤PAN). In countries like Brazil and Colombia, we find per capita economic growth does not Granger-cause innovation (GDP<#>PAN)
5.3. Case 3: between R&D expenditure (RDE) and per capita economic growth (GDP)
Brazil shows a unidirectional causality from innovation to per capita economic growth (RDE≤GDP), whereas in Chile we find the unidirectional causality from per capita economic growth to innovation (GDP≤RDE). Additionally, in Colombia we find the existence of bidirectional causality between innovation and per capita economic growth (RDE≤>GDP), while in the rest of the countries per capita economic growth does not Granger-cause innovation (RDE<#>GDP).
5.4. Case 4: between researcher in R&D activities (RRD) and per capita economic growth (GDP)
In Brazil, we find per capita economic growth Granger-causes innovation (RRD≤GDP). For countries like Colombia, Costa Rica, Ecuador, Mexico, Panama, Paraguay, Peru and Uruguay there is a bidirectional causality between innovation and per capita economic growth (RRD≤>GDP), while in the context of Argentina, Chile, Guatemala and Peru, per capita economic growth does not granger-cause innovation (RRD<#>GDP).
5.5. Case 5: between high-technology exports (HTE) and per capita economic growth (GDP)
Colombia shows a presence of unidirectional causality from innovation to per capita economic growth (HTE≤GDP), whereas for Paraguay we find the presence of unidirectional causality from per capita economic growth to innovation (GDP≤HTE). Moreover for countries like Brazil, Chile, Ecuador, Guatemala, Peru and Uruguay there is a bidirectional causality between innovation and per capita economic growth (HTE≤>GDP), while in the context of Argentina, Costa Rica, Mexico and Panama, per capita economic growth does not Granger-cause innovation (HTE<#>GDP).
5.6. Case 6: Between scientific and technical journals articles (STJ) and per capita economic growth (GDP)
For Brazil, we find the presence of unidirectional causality from per capita economic growth to innovation (GDP≤STJ), whereas for countries like Argentina, Chile, Colombia, Costa Rica, Ecuador, Mexico, Panama, Paraguay, Peru and Uruguay we find bidirectional causality between innovation and per capita economic growth (STJ≤>GDP), while in the context of Guatemala, we find that per capita economic growth does not Granger cause innovation (STJ<#>GDP).
Case 1: co-integration between PAR and GDP; case 2: co-integration between PAN and GDP; case 3: co-integration between RDE and GDP; case 4 co-integration between RRD and GDP; case 4: co-integration between HTE and GDP; case 6: co-integration between STJ and GDP. PAR is number of patents by residents, PAN is number of patents by non-residents, and RDE is research and development expenditure, RRD is research and development activities, HTE is high-technology exports, and STJ is scientific and technical journal articles, and GDP is per capita economic growth. 0 stands for absence of co-integration between innovation (PAR/PAN/RDE/RRD/HTE/STJ) and per capita economic growth, 1 stands for presence of co-integrating vector between innovation (PAR/PAN/RDE/RRD/HTE/STJ) and per capita economic growth. Parentheses indicate the number of co-integrating vectors (s). Results are derived based on and results.
Table 4. Descriptive statistics of the variables.
Table 5. Results of unit root test.
Table 6. Results of Johansen–Juselius co-integration test (Max test).
Table 7. Results of Johansen–Juselius co-integration test (trace test).
Table 8. Summary of co-integration test results.
Case 1: co-integration between PAR and GDP; case 2: co-integration between PAN and GDP; case 3: co-integration between RDE and GDP; case 4 co-integration between RRD and GDP; case 4: co-integration between HTE and GDP; case 6: co-integration between STJ and GDP. PAR is number of patents by residents, PAN is number of patents by non-residents, and RDE is research and development expenditure, RRD is research and development activities, HTE is high-technology exports, and STJ is scientific and technical journal articles, and GDP is per capita economic growth. SLH indicates the unidirectional causality from innovation to economic growth, DFH indicates the unidirectional causality from economic growth to innovation, FBH indicates the bidirectional causality between innovation and economic growth, and NEH indicates no causal flows between innovation and economic growth. Results are derived based on and results.
Table 9. Results of test from error correction model.
Table 10. Results of test from error correction model.
In , we observe that per capita economic growth in some cases leads to innovation, lending support to demand-following hypothesis of innovation–growth nexus. In other cases, it is innovation that regulates the level of per capita economic growth, lending support to supply-leading hypothesis of innovation–growth nexus. In some cases innovation and per capita economic growth are mutually interdependent. This supports feedback hypothesis of innovation–growth nexus. Additionally, there are also cases where innovation and per capita economic growth are independent of each other. That is the situation where both are neutral and offer support to neutrality hypothesis of innovation–growth nexus.
Table 11. Summary of granger casualty test.
Table 12. Summary of granger causality test results.
Table 13. Summary of Granger causality test results.
Case 1: co-integration between PAR and GDP; case 2: co-integration between PAN and GDP; case 3: co-integration between RDE and GDP. PAN is number of patents by non-residents, and RDE is research and development expenditure, RRD is research and development activities and GDP is per capita economic growth. Results are derived on the basis of results.
Case 4 co-integration between RRD and GDP; case 4: co-integration between HTE and GDP; case 6: co-integration between STJ and GDP. RRD is research and development activities, HTE are high-technology exports, and STJ is scientific and technical journal articles, and GDP is per capita economic growth. Results are derived on the basis of results.
6. Conclusion
Although the relationship between innovation and growth could be considered complicated to identify, this article provides a view of the importance of the 6 variables by analyzing the Granger causal nexus in a sample of 12 Latin American countries, using time series data from 1996 to 2015.
In general for countries such as Colombia, Costa Rica, Ecuador Mexico, Panama, Paraguay and Uruguay we found bidirectional causality between innovation and per capita economic growth in most of the variables; these results are aligned with the results of Mendoza (Citation2017).
Regarding patents filed by non-residents we detected for Colombia, Mexico, Costa Rica, Panama and Paraguay and Uruguay bidirectional causality between innovation and per capita economic growth. While Argentina, Chile, and Guatemala and Peru show unidirectional causality from per capita economic growth to innovation. In both cases innovation comes from abroad rather than from nationals.
In Brazil three variables (PAR, RRD AND STJ) indicate a unidirectional causality from economic growth to innovation. Between high-technology exports and per capita economic growth, for Chile, there is bidirectional causality between innovation and per capita economic growth, while in the context of Mexico per capita economic growth does not Granger-cause innovation.
Latin American countries have the challenge of improving environment to attract the sufficient FDI (foreign direct investment). In addition, governments must evaluate the results in order to reduce the risk of wasting money and have no impact on innovation.
Policy makers and academics interested in this matter should know that bidirectional relationship between innovation and per capita economic growth does not necessarily reflects the complete situation, more variables such as education and continuity of policies should be studied. This study analyzed the Granger causal nexus between innovation and per capita economic growth for 12 Latin American countries using time series data from 1996 to 2015. Policy makers and academics interested in this matter should know that the implications drawn from research on per capita economic growth that disregard the dynamic interrelation of the two variables will be imperfect. It is the conjoined bidirectional relationship between innovation and per capita economic growth the foundation of our research and the premise for future research.
shows that in regard of case 1 (PAR and GDP) 7 out of the 12 Latin American countries have a bidirectional causality between innovation and economic growth, and only Brazil indicates the unidirectional causality from economic growth to innovation. Cases 2, 4 and 6 also have a bidirectional causality between innovation and economic growth in most of the countries.
According to previous studies mostly focus on indicating the supply-driven approach of innovation-growth nexus, in we show that only Colombia have this nexus and particularly in case 5. In the Demand-following hypothesis innovation–growth nexus we found only Brazil and in particular in cases 4 and 6.
In order to achieve innovation, it is necessary to implement regular evaluations regarding policy design and financing needs; imitate practices that in other countries are increasingly implemented to promote innovations; the governmental agencies responsible for the funding of S&T (Science and Technology) and innovation projects should develop monitoring and assessment systems based on qualitative and quantitative information and indicators; support programs as well as the expected outputs and outcomes should be highlighted at the outset.
Since enterprises also foster and develop innovations, it is important for the government to promote effective loans among them. In order to create interest and facilitate loans, governments need to:
Promote low interest loans
Reduce bureaucracy and times
Constant evaluation of loans
Government also has an important role in attracting capital from foreign firms. Attracting foreign investors could be a difficult task since politics, economics and society are involved.
A country with a bad political environment or a bad economic situation would not attract the sufficient FDI (foreign direct investment); and, a society that is not well-educated will lack of opportunities. In addition, governments must evaluate the results in order to reduce the risk of wasting money and have no impact on innovation. Many governments provide incentives to attract more enterprises, such as tax holidays, suppression of trade union activity, and an accelerated depreciation allowances.
Disclosure statement
No potential conflict of interest was reported by the authors.
Additional information
Funding
References
- Aghion, P., & Howitt, P. (1992). A model of growth through creative destruction. Econometrica, 60(2), 323–351.
- Beneki, C., Giannias, D., & Moustakas, G. (2012). Innovation and economic performance: The case of Greek SMEs. Regional and Sectorial Economic Studies, 12(1), 31–42.
- CONACyT (2014). DECRETO por el que se aprueba el Programa Especial de Ciencia, Tecnología e Innovación 2014-2018. Decree to approve the Special Program of Science, Technology and Innovation 2014-2018. DOF, Wednesday, July 30th.
- Dickey, D. A, & Fuller, W. A. (1981). Likelihood ratio statistics for autoregressive time series with a unit root. Econometrica, 49(4), 1057–1072. doi:10.2307/1912517
- Freeman, C. (1995). The ‘National system of innovation in historical perspective. Cambridge Journal of Economics, 19(1), 5–24.
- Granger, C. W. J. (1969). Investigating causal relations by econometric models and cross-spectral models. Econometrica, 37, 424–438.
- Grossman, G. M., & Helpman, E. (1994). Endogenous innovation in the theory of growth. Journal of Economic Perspectives, 8(1), 23–44.
- Guisan, M. C. (2017). Rd expenditure on higher education in Spain, 1990-2015: Inequalities, among regions and fields, and comparisons with Europe and the United States. Regional and Sectoral Economic Studies, 17(1), 53–64.
- Hobday, M. (1995). Innovation in East Asia: The Challenge to Japan. Brookfield, VT: Edward Elgar.
- Hu, A. G. (2015). Innovation and economic growth in east Asia: An overview. Asian Economic Policy Review, 10(1), 19–37.
- Maradana, R. P., Pradhan, R. P., Dash, S., Gaurav, K., Jayakumar, M., & Chatterjee, D. (2017). Does innovation promote economic growth? Evidence from European countries. Journal of Innovation and Entrepreneurship, 6(1), 1.
- Martin, S., & Scott, J. T. (2000). The nature of innovation market failure and the design of public support for private innovation. Research Policy, 29(4–5), 437–447.
- Mathews, J. A. (1995). High-Technology Industrialization in East Asia: The case of the semiconductor industry in Taiwan and Korea. Taiwan: Chung-Hua Institution for Economic Research.
- Mendoza, J. E. (2017). Innovación tecnológica y crecimiento regional en México, 1995-2000. Revista Mexicana de Economía y Finanzas Nueva Época REMEF. The Mexican Journal of Economics and Finance, 1(3), 187– 201.
- Olarte, S. H. (2016). El proceso de regionalización latinoamericano ¿aceptar que la cooperación es la única manera? [Latin American regionalization process. Is cooperation the only way?] Regional and Sectorial Economic Studies, 16(1), 171–186.
- Olavarrieta, S., & Villena, M. G. (2014). Innovation and business research in Latin America: An overview. Journal of Business Research, 67(4), 489–497.
- Pierce, D. A., & Haugh, L. (1977). Causality in temporal systems: Characterization and survey. Journal of Econometrics, 5, 265–293.
- Pradhan, R. P., Arvin, M. B., Hall, J. H., & Nair, M. (2016). Innovation, financial development and economic growth in eurozone countries. Applied Economics Letters, 23(16), 1141–1144.
- Schumpeter, J. (1961). Reprint of 1934 edition, The theory of economic development. Cambridge, MA: Harvard University Press.
- Schumpeter, J. (1975). Colophon edition of 1942 edition, Capitalism, Socialism and Democracy. New York: Harper and Row.
- Segerstrom, P. S. (1991). Innovation, imitation, and economic growth. Journal of Political Economy, 99(4), 807–827.
- Sim, C. (1972). Money, income and casuality. American Economic Reviezu, 62, 54O552.
- Solow, R. M. (1979). Un modelo de Crecimiento. In A. Sen (Ed.), Economía del Crecimiento (pp. 525). México, D. F.: Fondo de Cultura Económica.
- Teece, D. J., Pisano, G., & Shuen, A. (1997). Dynamic capabilities and strategic management. Strategic Management Journal, 18(7), 509–533.
- Verspagen, B. (2005). Innovation and economic growth.The Oxford Handbook of Innovation.
- Villareal, M. (2010). The Mexican economy after the global financial crisis, congressional research service 7-5700. Retrieved from www.crs.gov.R41402
- Wong, P. K., Ho, Y. P., & Autio, E. (2005). Entrepreneurship, innovation and economic growth: Evidence from GEM data. Small Business Economics, 24(3), 335–350.
- World Economic Forum. (2008). Bridging skills and innovation gaps in Latin America: Country implementation of the competitiveness lab. Retrieved from http://www3.weforum.org/docs/WEF_Bridging_Skills_Innovation_Gaps_Latin_America.pdf