ABSTRACT
Mathematics is one of the core subjects in engineering education and forms the foundation of all disciplines in engineering. In Hong Kong, a significant number of students are admitted to engineering programmes without sufficient mathematical background. Engineering mathematics is essential for teaching and learning many fundamental and advanced engineering subjects. Due to this reason, a mobile application (app) is developed as one of the core components of engineering mathematics education by offering students flexible and self-initiated learning. In this study, a hypothesis model is proposed to investigate the effectiveness of student’s learning in engineering mathematics with the developed mobile app. The results showed that the ease and convenience of using the mobile app have a positive effect on students’ autonomous learning, and motivation and have a positive relationship with the learning effectiveness of the mobile learning (M-learning) app. It was found that the average marks of the assessment tests were significantly better than the screening test by using the app and notes. The current study has important implications in that the M-learning app should be used as a supportive role to supplement traditional teaching approaches.
Introduction
Under the education revolution in Hong Kong, students’ admission to bachelor’s degree programmes at universities in Hong Kong has been based on the Hong Kong Diploma of Secondary Education (HKDSE) public examination results. Which, the university’s 3-years undergraduate curriculum was switched to 4 years school year in order to implement the new “3+3+4” academic structure in the higher education sector in Hong Kong since 2012 (Shek & Yu, Citation2017). Under this academic structure, students are required to take three years of junior secondary school, three years of senior secondary school, and four years of university education (University Grant Committees UGC, Citation2013). These curriculum and assessment changes provide opportunities for students to receive six-year secondary education and four-year higher education. The extra year in the 4-year university curriculum is devoted to general education study in order to cater to the diversified learning needs of students with different aptitudes, interests, and competencies to excel. The new structure is also intended to provide a better connection with other major education systems around the world (Education and Manpower Bureau EDB, Citation2005).
In the new academic structure, students are required to meet the general entrance requirements of the specific university, including the English Language, Chinese Language, Mathematics, and Liberal Studies, while there is no compulsory subject requirement in most universities. In other words, admission does not filter out students who do not have sufficient background in the underpinning subjects. As a result, a number of students were admitted to the engineering programmes without sufficient mathematical backgrounds. According to the recent admission statistics of a typical engineering programme, less than half of the students studied the mathematics extended part module 1 (M1) or 2 (M2) in secondary school, which is considered to be crucial to those who study engineering programmes at university. In response, Chan and Cheung (Citation2018) highlighted that only almost 50% of students are likely to take M1 and M2 during their studies in secondary school. The enrolment rate of HKDSE students in M1 and M2 has significantly dropped from 22.9% in 2011/12 to 14.4% in 2018–19 (Legislative Council Secretariat, Citation2020). Currently, only The Chinese University of Hong Kong requires 5 programmes and The University of Hong Kong needs 5 programmes including M1/M2, respectively (Education Bureau, Citation2022).
Although freshmen are required to take several fundamental subjects in their first year, it is still a great challenge for them to learn or enhance difficult engineering mathematical knowledge in only one year. Engineering mathematics is also challenging to the students even transferred from the community college (Lau et al., Citation2022). Engineering mathematics is fundamental to the learning of engineering subjects from years 2 to 4. The fundamental mathematical knowledge directly affects the study of future engineering subjects at advanced levels (Trigwell, Ashwin, & Millan, Citation2013). Therefore, various technologies and teaching approaches such as mobile application, virtual reality, problem-based learning, etc. are implemented in trying to influence students’ study in engineering mathematics. Despite various approaches being adopted, the key concerns of students’ studies are mainly on the motivation and efforts they have paid into their studies. Mobile learning (M-learning) enables students to study anytime and anywhere which provides more opportunities for student’s study and review the necessary content and knowledge.
Bano, Zowghi, Kearney, Schuck, and Aubusson (Citation2018) conducted a systematic review of M-learning in mathematics education research papers from 2003 to 2016. 60 papers (49 empirical studies) highlighted that designing and implementing appropriate mobile technologies in mathematics education are essential to foster student learning facilitation and motivation. The researchers pointed out that various countries have increased learning and teaching in mathematics education to establish the capability of the future’s workforce for the job markets of tomorrow and to underpin innovation in boosting economic growth. To this end, the introduction of M-learning in mathematics offers students flexible and self-initiated learning and diversifies mathematics pedagogical methods.
This project aims to design and develop an M-learning app for engineering mathematics based on research from the teacher’s and student’s perspectives. The study also proposes a hypothesis model to determine the learning effectiveness of the M-learning app. Several factors, including user’s motivation, ease of use, the convenience of use, and autonomy were used for measuring the learning effectiveness. Finally, the study used student performance in the screening and assessment tests for comparison. Based on the investigation results, we provide the key perspectives to determine the roles of M-learning. This paper is divided into five main sections. After the introduction in Section 1, we provide a literature review in Section 2 including the mathematical education for engineering students, mobile technologies in teaching and learning, and the review of key features in the M-learning app. In Section 3, we describe the project relevant to the development of the M-learning app, the hypothesis model, and the data analysis of this study. Before the discussion and conclusions in Section 5, we elaborate on data collection and analysis in Section 4.
Literature review
Mathematics education for engineering students
Mathematics education for engineering students is a topic among professional associations, university mathematics teachers, and mathematics education researchers studied for many years (Bingolbali, Monaghan, & Roper, Citation2007; Harris, Black, Hernandez-Martinez, Pepin, & Williams, Citation2015). In general, mathematics educators have pointed out that engineering students encounter various mathematics education issues including barriers to understanding mathematical concepts, the methods and views of mathematics, their inclinations to and their interpretation of mathematical concepts, and their adjustments to the mathematical background on admission to engineering departments for the periods and issues emerging from this. All of these rationales mean that many students have insufficient abilities to perform elementary mathematical concepts and that their mathematical skills are declining. Clearly, it brings a negative impact on their mathematical achievements in engineering programmes (Bingolbali et al., Citation2007; Gill, Citation1999). Surprisingly, Sazhin (Citation1998) identified that engineering students learn that mathematical concepts are more difficult than physical concepts. Such students perceive that physical concepts are more closely associated with real-life experiences. Thus, it is important is to investigate how to use mobile technologies in Mathematics education to foster student learning motivation and facilitation (Vitasari, Wahab, Othman, Herawan, & Sinnadurai, Citation2010).
Teaching and learning technologies
It is obvious that the pre-requisite of fundamental mathematical knowledge and students’ understanding of prior mathematical knowledge appears to be necessary for the success of students’ studies (Rach & Ufer, Citation2020). Thus, to avoid the need of reviewing basic mathematical concepts again at the beginning of the advanced level of engineering subjects due to the insufficient engineering mathematical background of the students during class. In this way, various teaching and learning approaches include online learning (Trenholm & Peschke, Citation2020), M-learning, virtual reality/ mixed reality (Fong, Tang, Sie, Yu, Lo & Ma, Citation2021; Tang, Chau, Kwok, Zhu, & Ma, Citation2022), project-based learning (Mo & Tang, Citation2017), etc. are proposed to deal with the emerging challenges by giving higher teaching and learning autonomy for the students and educators. This is essential not only to reduce the distraction of the teaching progress and pedagogy but also to reduce the influence of the study progress of other students who have a stronger mathematical background.
Recently, the advanced technological tools integrated with modern learning platforms (Chau, Law, & Tang, Citation2021; Lau et al., Citation2021) generate great potential to enhance the learning process. Technological advancement has also enhanced the exponential growth of M-learning during the digital era. M-learning is considered to be the future trend in teaching and learning (Sarrab, AI-Shihi, & Rehman, Citation2013). A number of researchers addressed M-learning to overcome the pitfalls of traditional learning systems. Peng, Su, Chou, and Tsai (Citation2009) reported that ubiquitous mobile technologies can help students to learn expediently, appropriately, and immediately. Beth and Kathryn (Citation2012) claimed that mobile devices are consumer-friendly because of their flexibility and high efficiency. Subramanya and Farahani (Citation2012) pointed out that in terms of computing power, portability, and ease of use, mobile devices are clearly superior to carrying a laptop.
The maturity of mobile technology has resulted in the successful implementation of mobile learning (M-learning). The combination of wireless networks and mobile devices indicates that there is considerable potential to enhance and encourage the M-learning process. In general, the employment of network technology and mobile devices in the learning process has been transformed from E-learning to M-learning (Sarrab et al., Citation2013). M-learning is a growing and adaptive area where a number of conference proceedings and journal papers have been published. The number of mobile subscriptions has demonstrated remarkable growth (Bano et al., Citation2018). According to the World Bank Group (Citation2018), the mobile cellular subscription was over 106% globally in 2018, which indicated that each people may have more than one mobile phone. According to the statistical report on Internet Development in China released by China Internet Network Information Center (CNNIC) (Citation2019), the number of mobile internet users had reached 847 million and is continuously growing. Nevertheless, the digital divide is still serious across various regions and countries which makes a difference in the digital literacy of students.
The use of smartphones at reasonable prices has created an increase in the adoption of applications (apps) for numerous learning activities (Sarrab et al., Citation2013). A similar study was conducted by (Tang & Yu, Citation2018), in which a mobile platform was designed and developed to provide a convenient and attractive tool for students to review and study a computer-aided design (CAD) subject. Students have shown significant improvement by using the mobile platform in their studies. Despite the M-learning app can be used to facilitate students learning, educators reinforce the motion that we need to consider the challenges and obstacles of M-learning like financial constraints, insufficient effective educational policies for M-learning, a shortage of expertise in the implementation of mobile pedagogies, scarce hardware resources (e.g., bandwidth, infrastructure) and the technology acceptance relevant to the adoption of mobile devices by students (Bano et al., Citation2018). Thus, some researchers have pointed out that M-learning is problematic (Traxler, Citation2009).
The critical features of M-learning
Broadly speaking, numerous researchers have identified different characteristics of M-learning. Klopfer, Squire, and Jenkins (Citation2002) gave insights into M-learning related to five main characteristics: social interactivity, portability, connectivity, individuality, and context sensitivity. Koole (Citation2009) illustrated the FRAME model to recognize personal and social learning processes as well as the technical features of mobile devices. M-learning fosters collaboration, extended contextualization of learning, and obtaining key information. Kearney, Schuck, Burden, and Aubusson (Citation2012) further extended Koole’s model to highlight authenticity, personalization, and collaboration as the three unique features of the M-learning experience under time and space boundaries. M-learning can contribute to learner-centric conceptualization where knowledge can be continuously and seamlessly enriching (2012). The seamlessness of mobile learning (MSL) has been characterized by Wong and Looi (Citation2011) with a number of features. Wong (Citation2012) has further interpreted those features into an ultimate objective of MSL or M-learning which is to achieve knowledge synthesis. M-learning enables a learner to explore, identify, and grasp the potential opportunities in daily life, where learning and daily activities can be integrated with the help of mobile technology.
Research model
The effectiveness of M-learning has been investigated in many studies. Adalia (Citation2017) determined effectiveness factors based on the design of the functions in the M-learning app. In this study, we refer to the models in Kilis (Citation2013) and Rochma and Triyono (Citation2019), as well as taking the performance of the students into account, as suggested in Mileva (Citation2011). As such, the aforementioned corresponding studies provide a reasonable rationale for the selected model for the current study. In this study, we use three major factors (independent variable): students’ study motivation, ease, and convenience of use of the mobile app. Autonomous learning is the belief variable derived from the ease and convenience of use. We hypothesize that the ease and convenience of use of the mobile app have a positive relationship to students’ autonomous learning, and the dependent variable has positive effects on the learning effectiveness together with students’ study motivation. The hypothesized model refers to the student’s acceptance model of M-learning in Li (Citation2017) and is summarized in .
Figure 1. Snapshots of the developed mobile application. (a) Content page with collapsible index. (b) Descriptions and examples of an engineering mathematics topic.
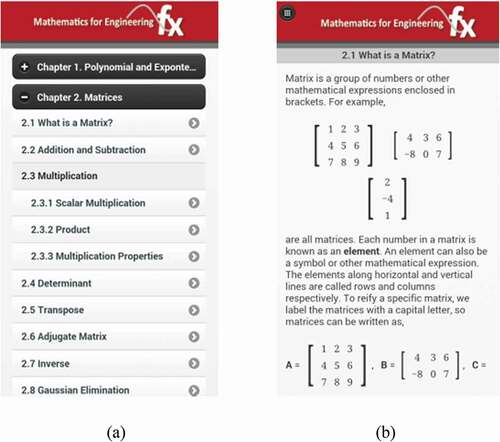
Study motivation
Study motivation is a psychological force that modifies or directs behaviour to facilitate effective student learning (Chau et al., Citation2021). Student motivation can be caused by various psychological factors and is usually related to students’ effective learning (Shabeena, Noshaba, & Sufiana, Citation2013). Therefore, in this study, we hypothesize that:
H1: Study Motivation has a positive effect on students’ learning effectiveness in mobile learning
Ease of use
Perceived ease of use is shown to affect behavioural intention (Ong, Lai, & Wang, Citation2004). Ease of use is particularly important and is related to the perceived usefulness and the attitudes towards using technological learning according to the general technology acceptance model (TAM) (Davis, Bagozzi, & Warshaw, Citation1989). The ease of use in the mobile app is believed to be important to students’ intention of using mobile technology. Therefore, we hypothesize that:
H2: There is a positive relationship between ease of use and students’ autonomous learning
Convenience of use
The convenience of use refers to whether the mobile device and the learning app are immediately available for the students to access information quickly and conveniently for their study (Alomary, Woollard, & Evans, Citation2016). Almasri (Citation2014) and Yu, Ally, and Tsinakos (Citation2018) proposed that convenience of use is one of the factors that have a positive effect on users’ intention and self-regulated autonomous learning. Therefore, we hypothesize that:
H3: There is a positive relationship between convenience of use and students’ autonomous learning
Autonomous learning
Autonomous learning by a student through the M-learning app refers to the ability to self-learn and set up directions in the planning, pacing, monitoring, and evaluation of the learning process (Little, Citation1994). Autonomy supportive teaching is believed to be linked with improved student performance (Furtak & Kunter, Citation2012). This study investigates the link between students’ autonomous learning and their learning effectiveness. Therefore, we hypothesize that:
H4: Students’ autonomous learning has a positive effect on learning effectiveness in mobile learning
Learning effectiveness
Student’s learning effectiveness refers to student perception of the ability of the M-learning app to provide support and sufficient content to enrich their knowledge for study. It also refers to their perceptions of the learning support to improve their study performance in a subject (Xi, 2017; Gore, Citation2017). The hypothesis model in this study was limited to investigating the perceptions of the target students who are studying fundamental engineering mathematics. In general, engineering relies remarkably on proficiency in mathematics. Engineering mathematics learning concentrates particularly on the learning of applied mathematical skills concerning solving engineering problems. Engineers require to identify the main functions of mathematical formulae with the aim that they can apply them in fixing engineering problems in association with their field understanding of engineering concepts (Fhloinn & Carr, Citation2017; Khiat, Citation2010). Indeed, Masouros and Alpay (Citation2010, p. 71) indicated that engineering mathematics learning can “reflect the hierarchical structure of mathematics and the way in which mathematics can be linked to real applications of ever-greater sophistication as the student progresses through the engineering degree”. To the best of the authors’ knowledge, the concept of engineering mathematics learning has inclined towards primary and secondary mathematics students. Also, research in Hong Kong relating to understanding engineering mathematics learning is seriously overlooked (Khiat, Citation2010).
Methodology
Recalling the objectives of this project, the aims are to encourage self-learning of the students and provide a convenient channel for the students to review and study fundamental mathematical concepts and equations that are needed in engineering courses. Therefore, a hypothesis model is proposed to evaluate the key items regarding the effectiveness of students learning with mobile technologies. All procedures performed in the studies were in accordance with the ethical standards of the university research committee and informed consent was obtained by participants before enrolling in the study.
Development of the M-learning application
The proposed engineering mathematics application not only encourages students’ self-learning, but also helps students to review mathematical concepts and equations to facilitate them to learn other engineering subjects easily. The development of the mobile app is divided into two major phases: the content and mobile app design.
In the design of the mobile app, we have adopted the model suggested by Pelton & Francis (Citation2011). We adopted several key principles in designing the app including, simple, meaningful content and models, and user-friendly design. These design principles are important, particularly for students learning to enhance their motivation, to ensure all students, particularly those who are not familiar with technology-based learning can handle the app and find the related content easily and quickly. This is particularly important as most of the students may not spend much time learning materials that they may feel boring. The design based on the key principles enables students to find out the necessary learning content quickly and easily, and without spending much time searching for the information. As such, we are focusing on several features of the M-learning app including:
The design of the education app is simple.
Distractions like background music and flashing effects are minimized.
The App provides useful models or manipulations such as examples and descriptions, for a more comprehensive understanding.
The App is user-friendly, less stress and success-oriented, which can build up the students’ confidence and increase their study motivation.
shows snapshots of the designed mobile application (Tang & Yu, Citation2018).
The key learning outcome of using mobile learning is to enhance students’ knowledge of fundamental mathematics to support the study of the latter engineering subjects. To achieve the learning outcome, a pilot study was performed to investigate the essential mathematics topics that may be useful to support the 4-years curriculum to study underpinning subjects. The study collected qualitative feedback and quantitative information from teachers and students to determine the specific engineering mathematics topics that may be useful for supporting students’ studies. Eight different engineering subjects, corresponding to six fundamental mathematics topics, were investigated. Different engineering subjects in the following areas were investigated: computer-aided design integrated design, mechatronics, engineering modelling, design for the environment, and design for manufacture. Another questionnaire was also distributed to determine the mathematical level of the engineering students. In the questionnaire, students can identify the mathematics techniques that they may feel difficult from their perspective and select more than one option. The mathematics techniques such as matrix, statistics, coordinate systems, differentiation and integration (Tang & Yu, Citation2018).
Data collection and analysis
In this data collection and analysis, the participants and data collection method is first described. The instruments used in the investigation include 2 tests and a questionnaire which is elaborated on next. Finally, the data analysis methods are also elaborated.
Participants and data collection
In order to collect data, the M-learning app was introduced to the target engineering students in the first teaching week at the commencement of the teaching semester. To ensure fairness to each student were participated in the tests, they were allowed to study using traditional notes and the developed mobile app according to their preference. In the beginning, a screening test was conducted to measure the mathematical capability of the students. A period of about two weeks was allocated to the target students to review and study the required mathematical knowledge using the developed app. About one and a half months later, an assessment test was conducted to assess students’ capability on the required mathematical knowledge. Students were required to participate in both screening and assessment tests and declare what kind of teaching materials they used to support their studies. In this study, the mobile app was developed in 2018. The study was conducted across two semesters from two classes of students studying the same subject. The subject was instructed by the same experience teacher.
Questionnaires for content design
In order to decide on the content of the learning platform, we have designed two sets of questionnaires from teachers’ and students’ perspectives. Both questionnaires are divided into two parts. In the first part, respondents are required to determine which fundamental mathematical techniques are required in various engineering subjects and what are the levels of difficulties of the students according to their perspectives. In the second part, respondents are required to give their opinions and suggestions on the types of content expected to be provided by the M-learning app, including exercises, quizzes, videos, notes, reference materials, etc. M-learning app has shifted from desktop to laptop and from laptop to palmtop devices like tablets and mobile phones (Marzuki, Majid, Shukri, Zawawi, & Bakar, Citation2020). As suggested by Du (Citation2015), education app fosters students to access information conveniently without using a computer. Broadly speaking, traditional classroom and online learning intend to deliver thorough, in-depth information or teach particular skills in a particular discipline or subject. However, the education app aims to provide quick information distribution for supporting a continuous learning process. As such, the duration of lessons normally will not last long. Furthermore, Fan and Wang (Citation2020) pointed out that education app conducts different gamified interactions through particular learning tasks like team interaction, social interaction, and competitive interaction in the virtual space.
Evaluation instruments
The evaluation instruments used in this study include screening and assessment tests, and a questionnaire. The questions in the screening and assessment tests were totally different but at the same level of difficulty. The questions in the tests are reviewed by 3 experienced local teachers in order to ensure a different set of question papers are at a similar level of difficulty to minimize the potential bias in the tests, as well as to justify the difficulty and time allowed for the tests are reasonably designed. On the other hand, we made use of the screening test to evaluate the levels of students between the test and control groups at the beginning to reduce the potential bias. The tests were graded and used to measure the number of students that could satisfy a certain level of mathematical knowledge for various engineering subjects. The results from the screening and assessment tests were compared to evaluate the effectiveness of the developed app.
Immediately after the assessment test, students were also required to complete a questionnaire. The questionnaire consisted of two sections in order to collect qualitative and quantitative information. The qualitative data included students’ comments and opinions on the platform and teaching materials. Quantitative data, including ease and convenience of using the app, autonomous learning and motivation, and learning effectiveness, were collected and measured, so as to verify the hypothesis learning effectiveness model of the proposed M-learning app in this study. The 5-point Likert scale was used to measure the degree of agreement from strongly disagree and strongly agree, in order to judge the students’ level of agreement for each set of factors including the ease of use, convenience of use, autonomous and study motivation of the developed app, etc., 3–4 questions were used for each set of determining factors. For each measurement factor, we calculated the average score of all participants in order to measure the level of agreement for each set of quantitative data.
Data analysis
A commercially available statistical analysis package SPSS V25.0 was used for the data analysis. The paired t-test with a 95% confidential level was used to compare the results between pre-and post-tests. A P-value of < 0.05 was considered statistically significant. The determining factors including the dependent, belief, and independent variables were validated using Cronbach’s alpha value, and we have followed the rules from George and Maller (George Citation2003) where an alpha value > 0.7 is acceptable, alpha value > 0.8 is good and alpha value > 0.9 is excellent. The validity of the survey study was performed using the Kaiser-Meyer-Olkin (KMO) test, and KMO values between 0.8 and 1 indicate the sampling is adequate. Lastly, the partial least squares (PLS) modelling and bootstrapping analysis were used to validate the proposed hypothesis model.
Results
Design of M-learning app
At the beginning of M-learning app development, an investigation was conducted to determine the content design of the mobile app from the teachers’ and students’ perspectives. illustrates the number of subjects required for different engineering mathematics topics. It was found that basic mathematics (including logarithmic functions, exponential functions, quadratic functions, polynomial Equations, etc.) were the most commonly adopted in the investigated subjects and were also the weakest parts for the students.
In the determination of the mathematical level of the students, a total of 70 feedbacks were collected (). In the questionnaire, it was found that around half of the students agree that matrix is the most difficult mathematical technique (including determinants, matrix inverse functions, and system equations or Gaussian elimination). It was also found that basic mathematics (logarithmic functions, exponential functions, quadratic functions, polynomial Equations, etc.) and statistics were difficult for most of the students. Besides, 41% of the students suggested that exercises and notes were important in the design of the mobile learning platform. Therefore, it was reviewed that matrix algebra is the most useful for supporting students’ study in more advanced engineering subjects. The specific content of matrix algebra includes Gaussian elimination, matrix operations, matrix inverse, sub-matrices problems, etc.
Among the common mobile learning platforms such as apps, websites, and Blackboard (the university’s internal learning platform), most students prefer to use mobile Apps. Regarding the suggestions on the design of the mobile learning platform, most students prefer a simple, user-friendly well-organized structured App. Finally, over 58% and 25% of the students are Android and Apple iOS users respectively. In this project, an application was developed to support both the Apple iOS and Android Operating systems (OS).
Data analysis
In the data analysis, the demographic information of the participants is first given. The reliability and validity analysis is performed to ensure the data are suitable for further analysis. Lastly, tests are performed to determine whether the hypothesis in the proposed research model is supported.
Participants
The investigation was conducted at a university in Hong Kong, where 70 undergraduate students participated. All of the survey participants were engineering students. illustrates the demographic information of the students who participated in the investigation. There were 43 (61.43%) males and 27 (38.57%) females in the study, and the average age of the participants was 22.34, with a standard deviation (SD) of 1.006.
Table 1. Demographic characteristics of students who participated in both the screening and assessment tests.
Reliability and validity analysis
We adopted a theoretical approach to validate the model by using factor analysis in this study. It was found that Cronbach’s alpha values for ease of use, the convenience of use, and study motivation were 0.842, 0.893, and 0.902 respectively which indicated that all the dependent variables showed a good or excellent level of acceptance. The Cronbach’s alpha value for the belief variable in autonomous learning was 0.724 which was acceptable in terms of reliability, while the dependent variable in learning effectiveness also demonstrated a good level of reliability (α = 0.862). The hypothesis model showed good fitting the model with the KMO value of 0.867 > 0.8. summarizes Cronbach’s alpha for the dependent, belief, and independent variables.
Table 2. Summary of the Cronbach’s alpha and variances explained of all indicator variables.
Hypothesis model
To test the proposed hypothesis model, Pearson’s correlation coefficient (Schober, Boer, & Schwarte, Citation2018) was used to determine the correlation of each factor. The results are summarized in . All factors showed a positive correlation of the coefficients ranging from 0.528 to 0.756. Partial least squares (PLS) modelling (Pirouz, Citation2006) was also carried out to investigate the relationship between the measured variables, and bootstrapping analysis was performed to investigate the significance of the hypothesis model. The results are presented in . All the connected factors in the proposed hypothesis model were significant in explaining the relationships. The path coefficients between the convenience of use and ease of use for students autonomous were 0.319 and 0.482, which demonstrated statistical significance at the level of 0.05 (p = 0.002 < 0.05) and 0.001 (p = 0.000 < 0.001) respectively, as hypothesized in H2 and H3. On the other hand, the student’s motivation and autonomy were also positively related to learning effectiveness, with path coefficients of 0.498 and 0.628 respectively. The level of significance was 0.001 (p = 0.000 < 0.001), supporting hypotheses H1 and H4.
Table 3. Pearson’s correlation coefficient of all measured factors.
Table 4. Bootstrapping analysis between each pair of connected factors.
Students test performance
In this study, most of the students (N = 44) chose to study using the mobile app, while the rest of the students (N = 26) used traditional notes in their study. shows the average marks for the screening test and assessment test between students using notes and apps. It was found that those students who selected teaching notes for learning had higher scores in the screening (µ = 56) and assessment (µ = 75.96) tests compared to the students using the app for the screening (µ = 46.15) and assessment (µ = 66.59) tests respectively. However, the difference in the mean score between both tests for the students using the mobile app was slightly higher (0.48) than for the students using teaching notes. It was revealed that the mean difference between the teaching supports is insignificant (p = 0.083). It was also interesting that the students have better results were preferred to use notes in their studies. Further investigation of students’ technological acceptance can be conducted in future studies. On the other hand, the difference in mean scores between the screening and assessment tests was also compared. It was revealed that the average mark in the assessment test was significantly higher (p = 0.000 < 0.001) than in the screening test, whether the student’s used notes or apps in their study. Nevertheless, for students without a strong mathematical background. There was no significant improvement by using the notes (p = 0.934 > 0.05), while the improvement was significant by using the mobile app (p = 0.004 < 0.01). This indicates that the mobile app can make significant apps, particularly for those students without strong underpinning backgrounds in mathematics.
Table 5. Average marks of the tests between students using notes and app.
Discussion
In this study, an M-learning app was designed that aimed to support enhanced student learning in engineering mathematics. We proposed a hypothesis model to determine the learning effectiveness based on students’ motivation and autonomous learning, as well as comparing students’ performance based on the test results.
Accounting for the hypothesis research model, the hypothesis was determined by using factor analysis and partial least squares (PLS) modelling. As stated in the hypothesis that motivation and student autonomous learning have a positive effect on learning effectiveness with M-learning, the hypothesis was supported by the statistical analysis. The results of this study align with the findings from other literature as suggested by Hannah and Josh (Citation2017), Hamid (Citation2012), Shadiev, Hwang, and Liu (Citation2018), and Ramamuruthy and Rao (Citation2015). We believe that students’ self-learning motivation and their autonomy are important factors for students to review the study so as to enhance their learning outcomes ultimately. On the other hand, we also hypothesis that the belief variable in students’ autonomous learning is positively affected by the ease and convenience of the M-learning app. The results aligned with the study by Adalia (Citation2017), and that M-learning offers a flexible and convenient platform for students learning assisted by the recently developed information technology (IT) platform (Kumar & Suji, Citation2019). We argue that the findings agree with the principles of mobile app design that ease of use and user-friendly design are important for students to search for necessary information for students learn autonomously without the need to seek other support irrespective of the backgrounds of the students.
Finally, test results were adopted to measure students’ performance. The results have demonstrated a significant improvement in the mean score on the test by using both mobile apps and notes. Despite there being no statistically significant difference when comparing teaching notes and the mobile app, the mobile app can make a significant improvement for those students without a strong underpinning mathematics knowledge than using the traditional notes. We argue that the proposed hypothesis model was able to provide insight to justify the key factors for determining the learning effectiveness of the students in M-learning. On the other hand, the mobile device is flexible and immediately available, so M-learning can be utilized to boost the learners using personalized learning, previews, and assignments (Xu, Citation2016). The M-learning app in the current study can be used in a supportive role to supplement traditional teaching. The proposed hypothesis model in the current study contributes to the development of M-learning teaching pedagogy, as well as the design of the mobile app for improving students’ learning effectiveness. Additionally, the current research suggests that M-learning should not be used to replace the traditional teaching materials, but act in a supportive role to supplement current teaching approaches.
Conclusions
A student-oriented mobile app was designed to enhance learning motivation, and therefore effectiveness, for learning engineering mathematics. We developed a hypothesis model to investigate the dependent variables in the student’s experience based on the independent variables, including ease of use and convenience of use of the mobile app, as well as the belief variable in student’s autonomy and another independent variable in student’s motivation. It was found that the independent variables have a positive effect on the belief variables and the independent variables are positively related to the dependent variables in students’ learning effectiveness. The study results showed that the hypothesis model was reliable and valid.
On the other hand, the learning effectiveness of the developed application is justified by the average results between the two tests. The study also investigated the students’ study performance between the screening and assessment tests by using the app and traditional notes in their study. It was suggested that those students who participated in this study achieved significant improvement when using both the app and traditional notes. Despite this study being intended to investigate student performance through academic test results, however, due to the limitation of the class size, the sample size of the investigation was still small. Since the current study is a pilot investigation on the hypothesis model, the sample size should be increased by including the results of students in other academic years and other subjects in the future.
Moreover, this study determined student performance based on the general mean score of the test, and different measurements to investigate student’s mathematical abilities can be made. Further investigation should also be conducted to investigate the design of the M-learning app so that it can be better than just using the traditional notes, rather than only providing a supportive role for student’s learning. Nevertheless, the study has important implications not only for the design of the M-learning platform such as the interface, content, UX/UI, etc., but it also provides important information on the factors that contribute to the effectiveness of M-learning. Nowadays, virtual teaching is become vital as a part of teaching and learning for students, M-learning enables students to learn anytime and anywhere which is particularly important to develop related teaching pedagogy for educators in the future.
Acknowledgments
We acknowledge support by the Department of Industrial and Systems Engineering, The Hong Kong Polytechnic University and the City University of Macau.
Disclosure statement
No potential conflict of interest was reported by the author(s).
Additional information
Funding
Notes on contributors
Yuk Ming Tang
Dr Yuk Ming Tang received his B.Sc. and M.Phil. from The Chinese University of Hong Kong. He was awarded a Ph.D. degree from the same university. He worked as a postdoctoral fellow at the Faculty of Medicine at CUHK after graduation. Currently, he is teaching in the Department of Industrial and Systems Engineering, the Hong Kong Polytechnic University (PolyU). His research interests include virtual reality (VR)/ mixed reality (MR), artificial intelligence (AI), blockchain, computer-aided design (CAD), and sustainable technology in Industry 4.0 and healthcare applications.
Ka Yin Chau
Dr. Chau is currently the Associate Dean of the Faculty of Business and Director of Asian Pacific Research Centre. He has published 50 academic papers indexed in SSCI and SCI.
Yui-yip Lau
Until now, Dr Lau has published more than 276 research papers in international journals and professional magazines, contributed 10 book chapters, 2 books and presented numerous papers in international conferences. He has also secured over HK$ 10 million research grants. Recently, he has been awarded a Certificate of Appreciation by the Institute of Seatransport in recognition of his outstanding performance on research and the Best Paper Award in international leading conferences. His research interests are cruise, ferry, maritime transport, air transport, impacts of climate change, maritime education and training, transport history, sustainability issues, supply chain management, health logistics, and regional development.
G.T.S. Ho
HO To Sum, George is currently an Associate Professor in the Department of Supply Chain and Information Management from The Hang Seng University of Hong Kong (HSUHK). He received his PhD in the Department of Industrial and Systems Engineering (ISE) from the Hong Kong Polytechnic University (PolyU). His research areas focus on Big Data Analytics, Internet of Things (IoT) and Smart City Applications. During the years of research, he has published more than 70 international journal papers that are SCI/SSCI listed such as the “International Journal of Production Economics”, the “International Journal of Production Research” and “Expert Systems with Applications”. He also scores an 25 h-index for publications. He has also managed several ITF projects, collaborative projects and consultancy projects, with different commercial companies, namely MTR Corporation Limited, Pok Oi Hospital, and Sino Parking Services Limited. Besides, his specialty has made him serve as the Associate Editor for International Journal of Engineering Business Management.
References
- Adalia, M. (2017). M-learning effectiveness factors. International Journal of Engineering Associates, 6(1), 1–8.
- Almasri, A.K.M. (2014). The influence on mobile learning based on technology acceptance model (Tam), mobile readiness (Mr) and perceived interaction (Pi) for higher education students. International Journal of Technical Research and Applications, 2(1), 05–11.
- Alomary, A., Woollard, J., & Evans, C. (2016). To use or not use: Mobile learning? In Proceedings of Academics World 27th International Conference, Paris, France. (pp. 32–35).
- Bano, M., Zowghi, D., Kearney, M., Schuck, S., & Aubusson, P. (2018). Mobile learning for science and mathematics school education: A systematic review of empirical evidence. Computers & Education, 121, 30–58.
- Beth, B., & Kathryn, L. (2012). Mathematical apps and mobile learning. In Society for Information Teaching and Teacher Education International Conference, New Orleans, Louisiana, United States. (pp. 38409).
- Bingolbali, E., Monaghan, J., & Roper, T. (2007). Engineering students’ conceptions of the derivative and some implications for their mathematical education. International Journal of Mathematical Education in Science and Technology, 38(6), 763–777.
- Chan, A.K.W., & Cheung, A.K.L. (2018). Gender differences in choosing STEM subjects at Secondary School and University in Hong Kong. Hong Kong: The Women’s Foundation.
- Chau, K.Y., Law, K.M., & Tang, Y.M. (2021). Impact of self-directed learning and educational technology readiness on synchronous E-learning. Journal of Organizational and End User Computing (JOEUC), 33(6), 1–20.
- China Internet Network Information Center. (2019). Statistical Report on Internet Development in China. https://cnnic.com.cn/IDR/ReportDownloads/201911/P020191112539794960687.pdf
- Davis, F.D., Bagozzi, R.P., & Warshaw, P.R. (1989). User acceptance of computer technology: A comparison of two theoretical models. Management Science, 35(8), 982–1003.
- Du, Y. (2015). Information use and barriers on a mobile app in distance learning. Journal of Library & Information Services in Distance Learning, 9(3), 204–220.
- Education and Manpower Bureau. (2005, May). The new academic structure for senior secondary education and higher education. https://334.edb.hkedcity.net/doc/eng/report_e.pdf
- Education Bureau. (2022). Entrance requirements of UGC-funded Universities on Mathematics. Retrieved from https://www.edb.gov.hk/attachment/en/curriculum-development/kla/ma/u.pdf
- Fan, J., & Wang, Z. (2020). The impact of gamified interaction on mobile learning APP users’ learning performance: The moderating effect of users’ learning style. Behaviour & Information Technology, 1–14. doi:https://doi.org/10.1080/0144929X.2020.1787516
- Fhloinn, E.N., & Carr, M. (2017). Formative assessment in mathematics for engineering students. European Journal of Engineering Education, 42(4), 458–470.
- Fong, K.N.K., Tang, Y.M., Sie, K., Yu, A.K.H., Lo, C.C.W., Ma, Y.W.T. . (2021). Task-specific virtual reality training on hemiparetic upper extremity in patients with stroke. Virtual Reality 26, 453–464 . doi:https://doi.org/10.1007/s10055-021-00583-6.
- Furtak, E., & Kunter, M. (2012). Effects of autonomy-supportive teaching on student learning and motivation. Journal of Experimental Education, 80(3), 284–316.
- George, D., & Mallery, P. (2003). SPSS for Windows step by step: A simple guide and reference. 11.0 update (4th ed.). Boston, MA: Allyn & Bacon.
- Gill, P. (1999). Aspects of undergraduate engineering students’ understanding of mathematics. International Journal of Mathematical Education in Science and Technology, 30(4), 557–563.
- Gore, T. (2017). The relationship between levels of fidelity in simulation, traditional clinical experiences and objectives. International Journal of Nursing Education Scholarship, 14(1), /j/ijnes.2017.14.issue-1/ijnes-2017-0012/ijnes-2017–0012.xml.
- Hamid, T. (2012). The effects of motivation in education. Procedia - Social and Behavioral Sciences, 31, 820–824.
- Hannah, M., & Josh, C. (2017). Mobile learning and its effects on academic achievement and student motivation in middle grades students. International Journal for Scholarship of Technology Enhanced Learning, 1, 91–110.
- Harris, D., Black, L., Hernandez-Martinez, P., Pepin, B., & Williams, J.; with the TransMaths Team. (2015). Mathematics and its value for engineering students: What are the implications for teaching? International Journal of Mathematical Education in Science and Technology, 46(3), 321–336.
- Kearney, M., Schuck, S., Burden, K., & Aubusson, P. (2012). Viewing mobile learning from a pedagogical perspective. Research in Learning Technology, 20(1), 1–17.
- Khiat, H. (2010). A grounded theory approach: Conceptions of understanding in engineering mathematics learning. The Qualitative Report, 15(6), 1459–1488.
- Kilis, S. (2013). Impacts of mobile learning in motivation, engagement and achievement of learners: Review of literature. Gaziantep University Journal of Social Sciences, 12(2), 375–383.
- Klopfer, E., Squire, K., & Jenkins, H. (2002). Environmental detectives: PDAs as a window into a virtual simulated world. In Proceedings of IEEE International Workshop on Wireless and Mobile Technologies in Education, IEEE Computer Society, Vaxjo, Sweden: pp. 95–98.
- Koole, M.L. (2009). A model for framing mobile learning, in empowering learners and educators with mobile learning. Ed M. Ally 25–47. Canada: Athabasca University Press, Athabasca.
- Kumar, C.P.S., & Suji, D.B. (2019). Global trends of ICT for education, emerging technologies to enhance learning among slow learners. lulu.com. 137–139.
- Lau, Y.Y., Tang, Y.M., Chau, K.Y., Vyas, L., Sandoval-Hernandez, A., & Wong, S. (2021). COVID-19 crisis: Exploring community of inquiry in online learning for sub-degree students. Frontiers in Psychology, 12, 679197.
- Lau, Y.Y., Tang, Y.M., Yiu, N.S.N., Ho, C.S.W., Kwok, W.Y.Y., & Cheung, K. (2022). Perceptions and challenges of engineering and science transfer students from Community College to University in a Chinese educational context. Frontiers in Psychology, 12, 797888.
- Legislative Council Secretariat. (2020). Nurturing of Local Talent. Retrieved from https://www.legco.gov.hk/research-publications/english/1920rb03-nurturing-of-local-talent-20200601-e.pdf
- Li, X.Q. (2017). Students’ acceptance of mobile learning:: An empirical study based on blackboard mobile learn. International Journal of Interdisciplinary Telecommunications and Networking, 9(1), 52– 69. 1 0.4018/I JITN.2017010105.
- Little, D. (1994). Learner Autonomy: A theoretical construct and its practical application. Die Neueren Sprachen, 93, 430–442.
- Marzuki, M.M., Majid, W.Z.J.A., Shukri, R.S.M., Zawawi, M.Z.M., & Bakar, H.A. (2020). 4P-model of accounting learning process: The role of mobile apps technology among non-accounting students. Journal of Education for Business, 95(6), 384–392.
- Masouros, S.D., & Alpay, E. (2010). Mathematics and online learning experiences: A gateway site for engineering students. European Journal of Engineering Education, 35(1), 59–78.
- Mileva, N. (2011). The effectiveness of mobile learning in the form of performance support system in higher education. International Journal of Interactive Mobile Technologies (Ijim), 5(4), 17–21.
- Mo, J.P.T., & Tang, Y.M. (2017). Project-based learning of systems engineering V model with the support of 3D printing. Australasian Journal of Engineering Education, 22(1), 3–13.
- Ong, C.S., Lai, J.Y., & Wang, Y.S. (2004). Factors affecting engineers’ acceptance of asynchronous e-learning systems in high-tech companies. Information and Management, 41(6), 795–804.
- Pelton, T., & Francis Pelton, L. (2011). Design principles for making meaningful mathematics apps. In M. Koehler & P. Mishra (Eds.), Proceedings of Society for Information Technology & Teacher Education International Conference 2011, 2199–2204. Chesapeake, VA: AACE
- Peng, H., Su, Y., Chou, C., & Tsai, C. (2009). Ubiquitous knowledge construction: Mobile learning re-defined and a conceptual framework. Innovations in Education & Teaching International, 46(2), 171–183.
- Pirouz, D. (2006). An overview of partial least squares. SSRN Electronic Journal, 10 1–16 . h ttp://d x.d oi.org/1 0.2139/ssrn.1631359
- Rach, S., & Ufer, S. (2020). Which prior mathematical knowledge is necessary for study success in the university study entrance phase? Results on a new model of knowledge levels based on a reanalysis of data from existing studies. International Journal of Research in Undergraduate Mathematics Education, 6(3), 375–403.
- Ramamuruthy, V., & Rao, S. (2015). Smartphones promote autonomous learning in ESL classrooms. Malaysian Online Journal of Educational Technology, 3(4), 23–35.
- Rochma, A.F., & Triyono, S. (2019). Auto-mobile language learning: Autonomous language learning based on the practicality of mobile applications. International Journal of Pedagogy and Teacher Education, 3(2). https://jurnal.uns.ac.id/ijpte/article/view/23288
- Sarrab, M., AI-Shihi, H., & Rehman, O.M.H. (2013). Exploring major challenges and benefits of M-learning adoption. British Journal of Applied Science & Technology, 3(4), 826–839.
- Sazhin, S.S. (1998). Teaching mathematics to engineering students. International Journal of Engineering Education, 14(2), 145–152.
- Schober, P., Boer, C., & Schwarte, L. (2018). Correlation coefficients: Appropriate use and interpretation. Anesthesia and Analgesia, 126(5), 1763–1768.
- Shabeena, S., Noshaba, P.N., & Sufiana, K.M. (2013). Motivational techniques for effective learning: A meta analysis. Elixir International Journal, 64, 19170–19176.
- Shadiev, R., Hwang, W., & Liu, T. (2018). Investigating the effectiveness of a learning activity supported by a mobile multimedia learning system to enhance autonomous EFL learning in authentic contexts. Educational Technology Research and Development, 66(4), 893–912.
- Shek, D.T., & Yu, L. (2017). The impact of 3-year and 4-year undergraduate programs on university students: The case of Hong Kong. International Journal of Adolescent Medicine and Health, 29(1), 49–55. PMID: 27299206.
- Subramanya, S.R., & Farahani, A. (2012). Point-of-view article on: Design of a smart phone app for learning concepts in mathematics and engineering. International Journal Of Innovation Science, 4(3), 173–184.
- Tang, Y.M., & Yu, K.M. (2018). Development and evaluation of a mobile platform for teaching mathematics of CAD subjects. Computer-Aided Design and Applications, 15(2), 164–169. doi:https://doi.org/10.1080/16864360.2017.1375665
- Tang, Y.M., Chau, K.Y., Kwok, A.P.K., Zhu, T., & Ma, X. (2022). A systematic review of immersive technology applications for medical practice and education - Trends, application areas, recipients, teaching contents, evaluation methods, and performance. Educational Research Review, 35, 100429.
- Traxler, J. (2009). Learning in a Mobile Age. International Journal of Mobile and Blended Learning, 1, 1–12.
- Trenholm, S., & Peschke, J. (2020). Teaching undergraduate mathematics fully online: A review from the perspective of communities of practice. International Journal of Educational Technology in Higher Education, 17(1), 1–18.
- Trigwell, K., Ashwin, P., & Millan, E.S. (2013). Evoked prior learning experience and approach to learning as predictors of academic achievement. British Journal of Educational Psychology, 83(3), 363–378.
- University Grant Committees. (2013). The “3+3+4” academic structure, annual report. https://www.ugc.edu.hk/doc/eng/ugc/publication/report/AnnualRpt1213/06.pdf
- Vitasari, P., Wahab, M.N.A., Othman, A., Herawan, T., & Sinnadurai, S.K. (2010). The relationship between study anxiety and academic performance among engineering students. Procedia Social and Behavioral Science, 8, 490–497.
- Wong, L.H., & Looi, C.K. (2011). What seams do we remove in mobile assisted seamless learning? A critical review of the literature. Computer & Education, 57(4), 2364–2381.
- Wong, L. (2012). A learner-centric view of mobile seamless learning. British Journal Of Educational Technology, 43(1), E19–E23.
- The World Bank Group. (2018). Mobile cellular subscriptions (per 100 people). https://data.worldbank.org/indicator/IT.CEL.SETS.P2
- Xu, Q. (2016). A brief study on English autonomous learning ability based on mobile learning in EFL curriculum. Canadian Social Science, 12(11), 114–118.
- Yu, S.Q., Ally, M., & Tsinakos, A. (2018). Mobile and ubiquitous learning: An international handbook. (Springer). doi:https://doi.org/10.1007/978-981-10-6144-8