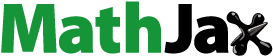
Abstract
Quantile regression predictions are considered for generalized order statistics which extend results previously established for record values and order statistics. In order to derive the results, some (univariate and bivariate) distortion representations of generalized order statistics are established. In particular, prediction of the sth generalized order statistic based on F given a single (generalized) order statistic
with r<s will be addressed. The presentation includes results for both known and unknown baseline distributions. In the latter case, we consider exponential distributions with unknown mean as well as the proportional hazards model. Using unimodality properties of the marginal density functions (pdf) of generalized order statistics, we find a simple representation of the maximum likelihood prediction of
given
.
1. Introduction
The prediction of future failure times has been widely discussed in the literature assuming various scenarios for the data model. In particular, order statistics have been extensively used when the first r failure times are available in order to predict the value of a larger order statistic. Some basic ideas and concepts as well as references can be found in the monograph by David and Nagaraja [Citation1, Section 8.7] (see also Arnold et al. [Citation2, Section 7.6] and, for prediction intervals, Hahn et al. [Citation3]). Various prediction concepts (like (best) linear prediction, (best) unbiased prediction, likelihood prediction and Bayesian prediction) have been used in this area. A brief review on point prediction methods applied under progressive censoring can be found in Balakrishnan and Cramer [Citation4, Chapter 16] which also includes references to related results in terms of generalized order statistics. Further references dealing with this problem can be found in, e.g. Navarro [Citation5], Navarro and Buono [Citation6], Volovskiy [Citation7], and Volovskiy and Kamps [Citation8].
In this paper, we consider quantile regression predictions which have been discussed in Navarro [Citation5] for record values and in Navarro and Buono [Citation6] for order statistics, respectively. We extend this approach to generalized order statistics which covers progressive censoring as a particular case. In order to establish the corresponding representations, we provide the necessary extensions to generalized order statistics in Section 2 (for details on generalized order statistics and related results, see Kamps [Citation9], Cramer and Kamps [Citation10]). In Section 3, we establish some (bivariate) distortion representation of generalized order statistics which will be utilized in Section 4 to obtain quantile regression predictions. We consider prediction of the sth (generalized) order statistic based on F and some parameters
given a single (generalized) order statistic
(r<s). First, we study the case of a known baseline distribution. Then, we consider the case of exponential distributions with unknown mean. Using unimodality properties of the density functions (pdf) of generalized order statistics, we find a simple representation of the maximum likelihood prediction (according to Kaminsky and Rhodin [Citation11]) of
given
. In particular, we show that the point predictor can be written as
where
is the mode of a function related to the density function of the
th generalized order statistic based on a uniform distribution and parameters
(see Equation (Equation7
(7)
(7) )) and
denotes the MLE based on the single observation
. Furthermore, we consider the particular cases of order statistics, ℓ-generalized order statistics, and record values and discuss the value of
in these models. Using the representation of the MLE based on a single generalized order statistic, we conclude from the above representation that the maximum likelihood predictor can be written in the form
where
depends only on the model parameters of the generalized order statistics (see Sections 4.2.1 and 4.2.2).
An extension to proportional hazard rate models is also presented. Finally, the results are illustrated by a couple of examples and simulations in Section 5.
Throughout, we use the following parametric representations for the pdf of
an exponential distribution with parameter
(for short
-distribution):
,
,
a gamma distribution with parameters
(for short
-distribution):
,
,
a beta distribution with parameters
(for short
-distribution):
,
,
2. Preliminaries
For , let
,
. According to Cramer and Kamps [Citation10] generalized order statistics
based on a cdf F and parameters
, can be defined by the stochastic representation
(1)
(1) where
defines the quantile function of F, that is,
and
denotes the (pseudo) inverse function of the reliability function
. On the other hand, such representations like (Equation1
(1)
(1) ) hold for order statistics, record values, and progressively Type-II censored order statistics as well as sequential order statistics by choosing appropriate values for the parameters
(see Cramer and Kamps [Citation12], Balakrishnan and Cramer [Citation4]). For order statistics, this result has also been derived by Malmquist [Citation13]. Clearly, simulation of
(and
) is easily possible using the product representation in (Equation1
(1)
(1) ).
In the following, we denote by the cdf of a product
as in (Equation1
(1)
(1) ) with parameters
. Notice that
is continuous and strictly increasing on
. Then, for
, we get
(2)
(2) so that we have
It should be noted that the above considerations show that the identity
(3)
(3) holds where
and
are independent random variables. This illustrates the Markovian property and shows that
can be seen as a somewhat (randomly) scaled version of
. The connection given in (Equation3
(3)
(3) ) is a key point for the results obtained in the present paper. A graphical illustration of the iterative construction can be found in Cramer [Citation14].
Remark 2.1
In case of order statistics, the gammas are given by ,
. Then,
has a
-distribution which can be seen from a result of Rao [Citation15] since
(see also Cramer [Citation16], Bdair and Raqab [Citation17]). For record values, that is,
for all j, one has that
. Hence, the distribution of
equals that of a product of s−r independent standard uniform random variables. In particular,
has a gamma distribution (see Navarro [Citation5]).
Remark 2.2
For illustration, we provide an expression for when
.
The case s = r + 1 is trivial and we have
for
(i.e., it is a power (or beta) distribution).
In the case s = r + 2, we can also get explicit expressions. If
, then a straightforward calculation leads to
for
(i.e., it is a negative mixture of two power distributions). If
, then
for
(i.e., it is a distorted distribution of a power distribution with the dual distortion function of a minimal repair process or an upper record).
Generally, the distribution of can be obtained easily from a log-transformation, that is,
where
. Hence, the distribution of
equals the distribution of a generalized order statistic based on parameters
and a standard exponential distribution (or the sum of independent but possibly not identically exponentially distributed random variables). Explicit representations for the pdf and cdf can be found in Kamps and Cramer [Citation18] and Cramer and Kamps [Citation10] (see also Springer and Thompson [Citation19], Springer [Citation20], Botta et al. [Citation21], Mathai [Citation22], Akkouchi [Citation23], and Levy [Citation24]). They are particularly simple in case that
γ's are all equal to γ which means that
;
γ's are all different. In this case, the cdf of
is given by
(4)
(4)
In general, the cdf can also be presented in terms of divided differences as has been pointed out in Cramer [Citation25] and Levy [Citation24].
Remark 2.3
Notice that, for parameters , the pdf of the log-transformed random variable
is given by
(5)
(5) and is log-concave and, thus, unimodal (see Cramer [Citation26] as well as Cramer et al. [Citation27]). Moreover, as pointed out in Cramer [Citation26],
is multiplicative strongly unimodal (see Cuculescu and Theodorescu [Citation28]) which also implies unimodality.
From Cramer et al. [Citation27], the limits
(6)
(6) can be obtained which show that, for s>r + 1, the maximum of
is attained at an inner point of the interval
. For r + 1 = s, the maximum is attained at zero. The latter identity in (Equation6
(6)
(6) ) can be obtained using expressions for pdfs of uniform generalized order statistics in terms of Meijer's G-functions (see Cramer et al. [Citation27], Lemma 2.1 (iv)), that is,
(7)
(7) as well as the limits for
in Lemma 2.2 (v) of Cramer et al. [Citation27]. This property will be used when considering predictions with unknown baseline distribution.
Log-concavity of the pdf of holds when, e.g., the ordered parameters
satisfy
and the difference of successive
's is at least 1. The latter one is true, for instance, for order statistics and progressively Type-II censored order statistics but not for record values. Furthermore, the moments of
can be easily obtained from (Equation2
(2)
(2) ). In particular, for
,
(8)
(8) which yields directly
.
3. Distortion representations
The key result for the predictions could be presented as follows. It will be used to establish the conditional predictive likelihood function () of
given
. An expression of the respective pdf can be found in, e.g., Cramer [Citation16, Theorem 3.3.2] (see also (Equation10
(10)
(10) )).
Theorem 3.1
Let F be a continuous cdf. With the notation introduced above, the conditional reliability function of is
(9)
(9) for
and x and y such that
and
.
Proof.
The result follows directly from the structure of generalized order statistics (see Cramer [Citation29]). First we note that, from (Equation2(2)
(2) ) and (Equation3
(3)
(3) ), we get
where
and
are independent. Therefore, for
and
,
This proves the representation in (Equation9
(9)
(9) ).
The representation in (Equation9(9)
(9) ) can be written as a distortion representation for the spacing
whose reliability function can be written as
for
, where
is a distortion function (i.e., a continuous distribution function with support included in
) and
,
, is the reliability function of the residual lifetime at age x. So all the results for distortions can be directly applied to it (see, e.g., Navarro [Citation30]).
We can also obtain a bivariate distortion representation for the distribution of the bivariate random vector ,
. For the definition and properties of bivariate distortions see [Citation31].
Theorem 3.2
Let F be continuous. With the notation introduced above, the joint reliability function of is given by
for
, where D is a bivariate distortion function which depends on
. Moreover,
where
and
is the pdf of
. In particular, we get the corresponding pdf
and zero elsewhere.
Proof.
Let D be the joint distribution function of and
. As its support is
it is a bivariate distortion function (see Navarro et al. [Citation31]). Therefore,
Moreover, for
, we have for
and
For
, we get
since V<U. The expression for the pdf d is directly obtained from the presented representation of the cdf D by, e.g., differentiating D with respect to u and v.
Remark 3.3
If we are able to get an explicit expression for D (which is not easy in general), then we can apply all the results included in Navarro et al. [Citation31]. In particular, we could predict from
for
. This kind of predictions is not as common as that considered in Theorem 3.1 since, if we work with lifetimes, we usually have information about early failures
and we want to predict future failures
for s>r. In this case, we have information about a current failure
at time x and we want to predict a past failure
.
4. Quantile regression predictions
4.1. Predictions when F is supposed known
Theorem 3.1 allows us to predict from
by using the median regression curve m which is obtained by solving the equation (in y)
Denoting by
the unique median of this cdf, the median regression curve is given by
which for order statistics leads to the result given in Navarro and Buono [Citation6].
This prediction can be reinforced with the following prediction bands. If we want to get
for some
, then
where
and
are the unique α and β quantiles of
. For example, the
centred prediction band is obtained as
In other cases, we could prefer bottom prediction bands obtained as
Numerical methods can be used to determine these quantiles. Here we could also plot the level curves
for, e.g., p = 0.05, 0.25, 0.5, 0.75, 0.95.
Another option is to consider the mode, that is, the maximum likelihood predictor (MLP). The conditional pdf can be obtained from Theorem 3.1 as
(10)
(10) where f and
are the pdf of F and
. To get the MLP we must look for the maximum of this function for fixed values of x and the gamma parameters.
Another reasonable option could be to consider the unique mode of
which leads to the predictor
In this regard, knowledge about the shapes of the density functions could be helpful. These shapes have been completely characterized by Bieniek [Citation32] for uniform generalized order statistics, that is, for arbitrary (positive) γ's. For progressively Type-II censored order statistics, the possible shapes reduce significantly (see Balakrishnan and Cramer [Citation4, Theorem 2.7.5]). These results are helpful in finding the mode. However, the maximum of the density function has to be computed numerically by standard methods like Newton–Raphson, Nelder–Mead procedures etc.
The third option is to consider the mean of (see (Equation8
(8)
(8) )) so that the corresponding predictor is given by
(11)
(11) This method is equivalent to consider the regression curve of
given by
Of course, in general,
is different from the real regression curve
.
4.2. Inferential approaches when F contains unknown parameters
If forms a parametric family of absolutely continuous distributions with unknown parameters
, the likelihood function of the first r generalized order statistics
and data
is given by
Assuming a proportional hazard rate (PHR) model with known baseline cdf
and parameter
, that is,
,
Here all the results on inference (estimation, prediction) for exponential generalized order statistics can be applied. This yields explicit results as given on pp. 7–8 in Navarro and Buono [Citation6] for order statistics. In particular, the MLE of ϑ is given by
(12)
(12) where
with
. In case of exponential distributions,
equals the total time on test. Related results can be found in Cramer and Kamps [Citation33].
Kaminsky and Rhodin [Citation11] initiated the discussion of maximum likelihood prediction. A comprehensive treatment of maximum likelihood prediction of future generalized order statistics from exponential distributions has been presented by Volovskiy [Citation7] in a general framework. The results are based on representations of the joint density functions of generalized order statistics in terms of so-called Meijer's G-functions (see, e.g., Mathai [Citation22]). However, using the density function of
in (Equation10
(10)
(10) ), we get the predictive likelihood function (
) of
and ϑ given
from Theorem 3.1 or Theorem 3.3.2 in [Citation16]. It is given by
In case of exponential distributions, the
is given by
with
and
as in (Equation5
(5)
(5) ). Now, we find a universal upper bound for the first factor which is attained for any given
by choosing
appropriately. In fact, we get
where
denotes the mode of the function
. Note that, according to Remark 2.3,
when r + 1 = s and
is an inner point of the interval
when r + 1<s.
Then, we obtain an upper bound for the as
(13)
(13) with equality iff
for any given
. Therefore, we get an upper bound w.r.t.
which is independent of ϑ but it can be attained for any given value of ϑ by defining
as above. The maximum has usually to be obtained numerically but, for selected cases, an explicit representation of
is available (see Section 4.2.1).
The remaining part of the upper bound depending on ϑ corresponds to the likelihood function given a single observation , that is,
(14)
(14) In this case, the MLE
of ϑ uniquely exists (see Hermanns et al. [Citation34]) so that the maximum likelihood predictor is given by
(15)
(15) Computational methods to compute the MLE (like the EM-algorithm) can be found in Hermanns et al. [Citation34] (see also Glen [Citation35]).
For some submodels of generalized order statistics like order statistics and record values, the weight and the MLE of ϑ can be obtained as closed form expressions.
Remark 4.1
Both maximization problems, i.e., maximizing the function w.r.t.
in (Equation13
(13)
(13) ) and the likelihood w.r.t. ϑ in (Equation14
(14)
(14) ), are related to equations of the type
where α is the elasticity function of
and
, respectively. The elasticity function of a function h is defined as
.
4.2.1. Order statistics
For order statistics and
in a sample of size n, we have
,
. Then, we find from (Equation7
(7)
(7) ) that
is the pdf of a
-distribution (see Remark 2.1). Thus, its maximum is attained at the mode of the
-distribution which is given by
(see, e.g., Marshall and Olkin [Citation36, p. 480]). Then, solving the equation
yields the expression
Therefore, we get the predictor
This result is given in Navarro and Buono [Citation6]. Note that the factor is zero when s = r + 1 so that we obtain the prediction
in this case.
It remains to compute the MLE of ϑ which means to obtain the MLE for the parameter ϑ based on the single order statistic from an exponential distribution. This problem has been addressed in Glen [Citation35]. Then, the MLE has the form
(16)
(16) where
is the unique solution of the equation
(17)
(17) in z>0. Note that the function on the left is strictly increasing from
(
) to n (
). As a result, we can write the maximum likelihood predictor as
If r = 1, then
so that
(as expected).
4.2.2. ℓ-Generalized order statistics
A similar argument can be applied for ℓ-generalized order statistics with , that is, for generalized order statistics with parameters
,
, with k>0. As pointed out in Cramer [Citation16, Example 3.1.4],
with a random variable
. Thus,
which is the pdf of a special beta power distribution (see Zografos and Balakrishnan [Citation37], Cordeiro and dos Santos Brito [Citation38]). From (Equation5
(5)
(5) ), we get
which has the pdf
Using similar arguments as for order statistics in Section 4.2.1, we find that
attains its maximum at the value
Note that the representation is quite similar to the order statistics' case. As above, we get the predictor
Note that the factor is zero when s = r + 1 so that we obtain the prediction
in this case. In order to compute the MLE
, we can proceed as for order statistics. As above, we get the likelihood function as
Then, we get the unique maximum by choosing
as the unique solution of the equation (cf. (Equation17
(17)
(17) ))
(18)
(18) which reduces to (Equation17
(17)
(17) ) when
(and k = 1). Note that the function on the left is strictly increasing from
(
) to
(
). Thus, as for order statistics, the maximum likelihood estimator is given by
(see (Equation16
(16)
(16) )) with
as unique solution of the equation (Equation18
(18)
(18) ). Again, as above, the maximum likelihood predictor can be written in the form
4.2.3. Record values
For record values, is the pdf of a gamma distribution with parameters s−r and 1. It attains its maximum value at the mode (see, e.g. Marshall and Olkin [Citation36, p. 314])
Then, we arrive at the predictor
By analogy with (ℓ-generalized) order statistics, the best prediction for s = r + 1 is given by the rth record, that is, by
. This fact was already mentioned in Volovskiy and Kamps [Citation8, p. 854].
The MLE is obtained from
so that
. Thus, we get
A similar result has been established in Kaminsky and Rhodin [Citation11, Example 5.2] and Volovskiy [Citation7, Proposition 5.8] assuming that the first r record values have been observed (see also Remark 4.2); note also that it is important to take into account the counting of the records values, that is, whether the counting of the records starts with one and zero, respectively; see also Arnold et al. [Citation39, Section 5.6]).
4.2.4. PHR model
A similar representation holds in the PHR model with baseline cdf F where has to be replaced by
so that the maximum likelihood predictor of
given
is given by
Remark 4.2
If the problem is discussed given the right censored sample then the likelihood equations can be explicitly solved, that is, the MLE is given by (Equation12
(12)
(12) ). Thus, the corresponding prediction is given by
The result for record values can be found in Volovskiy and Kamps [Citation8]. For the case of multiply censored samples from generalized order statistics, we refer to Volovskiy [Citation7].
5. Illustration
In this section, some examples are given to illustrate the results presented in the previous section. In the first example, assuming a parent exponential distribution, predictions related to order statistics are analyzed.
Example 5.1
Let us consider a sample of size m = 20 whose parent distribution is exponential with parameter . Consider the corresponding generalized order statistics
based on the parameters
(order statistics),
, which are obtained as
where
. By randomly generating m = 20 uniform numbers in
, we obtain the simulated generalized order statistics as
We want to give median predictions for this sample. In the case s = r + 1, we have
, from which the median is given by
. Hence, the median regression curve is given by
(19)
(19) By proceeding in this way, we obtain the median prediction for
. Moreover, we study the mean predictions for this case, which are obtained by
(20)
(20) By replacing ϑ with
in Equations (Equation19
(19)
(19) ) and (Equation20
(20)
(20) ), where
is given by (Equation12
(12)
(12) ), the predictions with unknown parameter ϑ are obtained. In Figure (left), we plot the difference in absolute value between exact values and predictions based on the median and the mean assuming both known and unknown value of the parameter. Note that with unknown ϑ some predictions based on the median and on the mean are not given in the figure in order to preserve its readability. We observe that, under the assumption of known ϑ, the predictions based on the median are better than the predictions based on the mean in 10 over 19 cases, while in one case (r = 19) they give the same value. Similarly, with unknown ϑ, the predictions based on the median are better than the predictions based on the mean in 11 over 19 cases and they are equal for r = 19.
Figure 1. Absolute value differences between generalized order statistics and predictions with known parameter ϑ based on the median (black circles) or the mean (red squares) and with unknown parameter ϑ based on the median (blue triangles) or the mean (green stars) from the simulated sample in Example 5.1 with m = 20, and s−r = 1 (left). Predictions (red) for from
for m = 20, s−r = 1, for the exponential distribution in Example 5.1. The black points are the observed values and the blue lines are the limits for the
(continuous lines) and the
(dashed lines) prediction intervals (right).
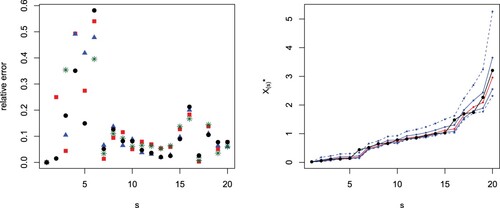
Similarly, by replacing the median with
,
,
and
we obtain the 50% and
quantile prediction intervals. The results are plotted in Figure (right). There we can observe that 2-out-of-19 exact points do not belong to the
prediction interval and 8-out-of-19 do not belong to the
prediction interval.
Now, we turn on considering the case s = r + 2. In this case, under the parameter and the model assumptions, the predictions and the prediction intervals are obtained by the quantiles of
By analogy, we can obtain point predictions based on the mean by using
By analogy with the case s = r + 1, predictions with unknown ϑ can be obtained as well. In Figure (left) we plot the difference in absolute value between exact values and predictions obtained with the median and the mean assuming both known and unknown value of the parameter, by leaving out the predictions with unknown ϑ for
and 3 as they are much bigger compared to the others. In the figure, we can observe that the predictions based on the median are better than the ones based on the mean in 15 over 18 cases assuming known ϑ, while they are better in 9 over 18 cases and equal for r = 17 assuming unknown ϑ.
Figure 2. Absolute value differences between generalized order statistics and predictions with known parameter ϑ based on the median (black circles) or the mean (red squares) and with unknown parameter ϑ based on the median (blue triangles) or the mean (green stars) from the simulated sample in Example 5.1 with m = 20, and s−r = 2 (left). Predictions (red) for from
for m = 20, s−r = 2, for the exponential distribution in Example 5.1. The black points are the observed values and the blue lines are the limits for the
(continuous lines) and the
(dashed lines) prediction intervals (right).
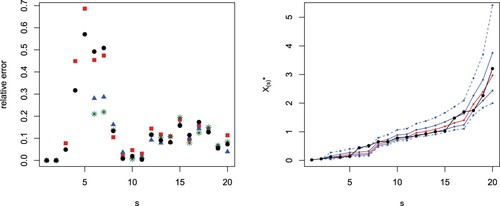
In Figure (right), we plot the predictions for (red line), s = r + 2 jointly with the limits of the
(dashed blue lines) and
(continuous blue line) prediction intervals in the simulated sample. In this case, we can observe that 2-out-of-18 exact points do not belong to the
prediction interval and 8-out-of-18 do not belong to the
prediction interval.
As the parameters are all different, it is possible to use (Equation4
(4)
(4) ) to determine the predictions and the prediction bands for the cases in which
. Assuming known ϑ, the results are presented for some selected choices of r and s in Table . Then, in Table , we present the number of exact values which do not belong to the 50% and
prediction intervals. We obtain also the point predictions based on the mean with known ϑ and on the median and the mean with unknown ϑ, and the results are given in Tables , respectively. From the tables, we can observe that the predictions based on both the median and the mean assuming unknown ϑ are really bad for small values of r, while they are comparable with the predictions assuming known ϑ for higher values of r.
Table 1. Predicted values based on the median from
assuming known ϑ in Example 5.1 for some choices of r and s such that
. In the bottom line we provide the exact values.
Table 2. Number of exact values out of the 50% and prediction intervals with fixed r in the sample of size 20 considered in Example 5.1.
Table 3. Predicted values based on the mean from
assuming known ϑ in Example 5.1 for some choices of r and s such that
. In the bottom line we provide the exact values.
Table 4. Predicted values based on the median from
assuming unknown ϑ in Example 5.1 for some choices of r and s such that
. In the bottom line we provide the exact values.
Table 5. Predicted values based on the mean from
assuming unknown ϑ in Example 5.1 for some choices of r and s such that
. In the bottom line we provide the exact values.
In the following example, we study the performance of our predictions for a different model of generalized order statistics in a sample with parent exponential distribution.
Example 5.2
Now, by analogy with Example 5.1, we consider again a standard exponential distribution and a sample of size m = 20, but with parameters defined by
, that is, we consider 2-generalized order statistics with parameter k = 1 (see Cramer [Citation16, p. 5]). The simulated generalized order statistics
, are given by
By proceeding as described in Example 5.1, we obtain the median and the mean predictions for
both in the case of known and unknown parameter ϑ. In Figure (left) we plot the difference in absolute value between exact values and predictions based on the median or the mean assuming known and unknown value of ϑ. There we observe that, assuming known ϑ, the predictions based on the median are better than the predictions based on the mean in 10 over 19 cases, while in one case (r = 19) they give the same value. Similarly, assuming unknown ϑ, the predictions based on the median are better than the predictions based on the mean in 12 over 19 cases and they are equal for r = 19. In this case, the predictions based on the assumption of known ϑ perform much better than the predictions based on unknown ϑ (as expected). Furthermore, we obtain the 50% and
quantile prediction intervals. The results are plotted in Figure (right). There we can observe that 2-out-of-19 exact points do not belong to the
prediction interval and 8-out-of-19 do not belong to the
prediction interval.
Figure 3. Absolute value differences between generalized order statistics and predictions with known parameter ϑ based on the median (black circles) or the mean (red squares) and with unknown parameter ϑ based on the median (blue triangles) or the mean (green stars) from the simulated sample in Example 5.2 with m = 20, and s−r = 1 (left). Predictions (red) for from
for m = 20, s−r = 1, for the exponential distribution in Example 5.2. The black points are the observed values and the blue lines are the limits for the
(continuous lines) and the
(dashed lines) prediction intervals (right).
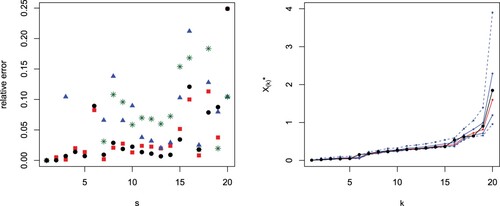
In the case s = r + 2, with the parameters and the model assumptions stated above, the predictions and the prediction intervals are obtained by the quantiles of
Analogously, we obtain the predictions based on the mean and the predictions with unknown ϑ.
In Figure (left) we plot the difference in absolute value between exact values and predictions based on the median or the mean both assuming known and unknown parameter ϑ. There, we can observe that, assuming known ϑ, the predictions based on the median are better than the ones based on the mean in 15 over 18 cases. In the case of unknown parameter ϑ, the predictions based on the median are better than the ones based on the mean in 11 over 18 cases. In Figure (right), we plot the predictions for (red line), s = r + 2 jointly with the limits of the
(dashed blue lines) and
(continuous blue line) prediction intervals in the simulated sample. In this case, we can observe that 2-out-of-18 exact points do not belong to the
prediction interval and 8-out-of-18 do not belong to the
prediction interval.
Figure 4. Absolute value differences between generalized order statistics and predictions with known parameter ϑ based on the median (black circles) or the mean (red squares) and with unknown parameter ϑ based on the median (blue triangles) or the mean (green stars) from the simulated sample in Example 5.2 with m = 20, and s−r = 2 (left). Predictions (red) for from
for m = 20, s−r = 2, for the exponential distribution in Example 5.2. The black points are the observed values and the blue lines are the limits for the
(continuous lines) and the
(dashed lines) prediction intervals (right).
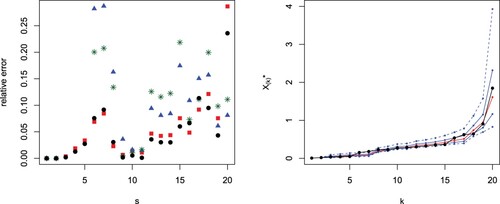
As the parameters are all different, it is possible to use (Equation4
(4)
(4) ) to determine the predictions and the prediction bands for the cases in which
. In Table the results are presented for some selected choices of r and s assuming known ϑ. In Table , we present the number of exact values which do not belong to the 50% and
prediction intervals. We obtain also the point predictions based on the mean with known ϑ and on the median and the mean with unknown ϑ, and the results are given in Tables . From the tables, we can observe that the predictions based on both the median and the mean assuming unknown ϑ are really bad for small values of r, while they are comparable with the predictions assuming known ϑ for higher values of r, and on average even better in the case of the predictions based on the mean.
Table 6. Predicted values based on the median from
assuming known ϑ in Example 5.2 for some choices of r and s such that
. In the bottom line we provide the exact values.
Table 7. Number of exact values out of the 50% and prediction intervals with fixed r in the sample of size 20 considered in Example 5.2.
Table 8. Predicted values based on the mean from
assuming known ϑ in Example 5.2 for some choices of r and s such that
. In the bottom line we provide the exact values.
Table 9. Predicted values based on the median from
assuming unknown ϑ in Example 5.2 for some choices of r and s such that
. In the bottom line we provide the exact values.
Table 10. Predicted values based on the mean from
assuming unknown ϑ in Example 5.2 for some choices of r and s such that
. In the bottom line we provide the exact values.
In the last example, we discuss again the model used in Example 5.2 and analyze the coverage of the 50% and prediction intervals.
Example 5.3
Consider the model already presented in Example 5.2, i.e., standard exponential distribution and a sample of size m = 20, with parameters defined by
. To better analyze the predictions, we simulate N = 100 random samples of size m. Then, by fixing the values of r and s, we get the predictions for each sample. We start with the case s = r + 1 and in particular with r = 4 and s = 5. The results are plotted in Figure (left). There, we can observe that 49 values do not belong to the
prediction intervals and 14 values do not belong to the
prediction intervals. Now, we consider the case s = r + 2, with r = 4 and s = 6. The results are plotted in Figure (right) where we can observe that 54 and 13 values do not belong to the 50% and
prediction intervals, respectively. In Figure , we consider some cases in which the difference s−r is greater than two. More precisely, in Figure (left) we have r = 4 and s = 10 with 59 and 15 values out of the 50% and
prediction intervals, respectively, and in Figure (right) we have r = 4 and s = 12 with 56 and 15 values out of the 50% and
prediction intervals, respectively.
6. Conclusion and outlook
In this paper, we have provided different tools to predict future data from present and past data in ordered data sets that can be modelled as generalized order statistics. These results extend preceding ones for order statistics and record values (that are included in the generalized order statistics' model as particular cases). The quantile regression technique allows us to provide not only point predictions but also confidence regions for those values.
The examples included in Section 5 show how to apply the established theoretical results to specific situations with different assumptions and tools. In the first example, we consider ordered data from a sample (of order statistics) with a baseline exponential distribution and we use mean and median predicted values, providing 50% and 90% confidence prediction bands as well. We do the same in the second example but with data from a 2-generalized order statistics' model. The coverage probabilities for the quantile regression bands obtained with this model are analyzed in the third example. These techniques can be used to analyze similar examples with ordered data.
There are several tasks for future research projects. As already mentioned above, we can study different models and/or different baseline distributions (Weibull, Pareto, etc.). Especially, the case of unknown parameters is of particular interest. So far, the regression approach is based on a completely known baseline distribution function F. However, one may consider estimators of the cumulative distribution function F and the quantile function . For instance, suppose
is the cumulative distribution function of an
-distribution with unknown mean
. Given an estimator
of ϑ, one can estimate
and
by the corresponding plug-in estimators. Then, from (Equation11
(11)
(11) ), we get the predictor
which has the same form as the maximum likelihood predictor given in (Equation15
(15)
(15) ). However, its computation does not involve any computational method. Hence, we get for a generalized order statistics' model alternative but always explicit predictors to those obtained in Section 4.2 for an unknown mean. In particular, for order statistics and record values, we find the predictors
Similarly, the other regression methods can be taken into account. Furthermore, if
is a consistent estimator of the mean ϑ, asymptotic confidence bands can be obtained in such a situation. It would be interesting to study these predictors and extend the method to other distributions. Another relevant task is to develop fit tests to determine whether our techniques can be applied for given data. All these problems are crucial in order to provide accurate predictions for future data values. Finally, one may consider Type-I or hybrid censored data (see Balakrishnan et al. [Citation40] for a recent review of these models and related results) and apply the regression approach to predictions based on this kind of data.
Acknowledgements
The authors are grateful to an associate editor and two reviewers for suggestions which led to an improved version of the manuscript.
Disclosure statement
No potential conflict of interest was reported by the author(s).
Additional information
Funding
References
- David HA, Nagaraja HN. Order statistics. 3rd ed. Hoboken (NJ): Wiley; 2003.
- Arnold BC, Balakrishnan N, Nagaraja HN. A first course in order statistics. Philadelphia: Society for Industrial and Applied Mathematics (SIAM); 2008.
- Hahn GJ, Meeker WQ, Escobar LA. Statistical intervals: a guide for practitioners. New York: Wiley; 2017.
- Balakrishnan N, Cramer E. The art of progressive censoring. applications to reliability and quality. New York: Birkhäuser; 2014.
- Navarro J. Prediction of record values by using quantile regression curves and distortion functions. Metrika. 2021;85:675–706. doi: 10.1007/s00184-021-00847-w
- Navarro J, Buono F. Predicting future failure times by using quantile regression. Metrika. 2023;86:543–576. doi: 10.1007/s00184-022-00884-z
- Volovskiy G. Likelihood-based prediction in models of ordered data [PhD thesis]. RWTH Aachen, Aachen; 2018.
- Volovskiy G, Kamps U. Maximum observed likelihood prediction of future record values. TEST. 2020;29:1072–1097. doi: 10.1007/s11749-020-00701-7
- Kamps U. A concept of generalized order statistics. Stuttgart: Teubner; 1995.
- Cramer E, Kamps U. Marginal distributions of sequential and generalized order statistics. Metrika. 2003;58:293–310. doi: 10.1007/s001840300268
- Kaminsky KS, Rhodin L. Maximum likelihood prediction. Ann Inst Stat Math. 1985;37:507–517. doi: 10.1007/BF02481119
- Cramer E, Kamps U. Sequential k-out-of-n systems. In: Balakrishnan N, Rao CR, editors. Handbook of statistics: advances in reliability. Vol. 20, Chapter 12. Amsterdam: Elsevier; 2001. p. 301–372.
- Malmquist S. On a property of order statistics from a rectangular distribution. Skand Aktuarietidskr. 1950;33:214–222.
- Cramer E. Sequential order statistics. In: Kotz S, Balakrishnan N, Read CB, Vidakovic B, Johnson NL, editors. Encyclopedia of statistical sciences. Update Vol. 12. Hoboken (NJ): Wiley; 2006. p. 7629–7634.
- Rao CR. On some problems arising out of discrimination with multiple characters. Sankhyā. 1949;9:343–366.
- Cramer E. Contributions to generalized order statistics. Oldenburg, Germany: Habilitationsschrift, University of Oldenburg; 2003.
- Bdair OM, Raqab MZ. Prediction of future censored lifetimes from mixture exponential distribution. Metrika. 2022;85:833–857. doi: 10.1007/s00184-021-00852-z
- Kamps U, Cramer E. On distributions of generalized order statistics. Statistics. 2001;35:269–280. doi: 10.1080/02331880108802736
- Springer MD, Thompson WE. The distribution of products of beta, gamma and Gaussian random variables. SIAM J Appl Math. 1970;18:721–737. doi: 10.1137/0118065
- Springer MD. The algebra of random variables. New York: Wiley; 1979.
- Botta RF, Harris CM, Marchal WG. Characterizations of generalized hyperexponential distribution functions. Comm Stat Stoch Models. 1987;3:115–148. doi: 10.1080/15326348708807049
- Mathai AM. A handbook of generalized special functions for statistical and physical sciences. Oxford: Clarendon Press; 1993.
- Akkouchi M. On the convolution of exponential distributions. J Chungcheong Math Soc. 2008;21:501–510.
- Levy E. On the density for sums of independent exponential, Erlang and gamma variates. Stat Papers. 2022;63:693–721. doi: 10.1007/s00362-021-01256-x
- Cramer E. Hermite interpolation polynomials and distributions of ordered data. Stat Methodol. 2009;6:337–343. doi: 10.1016/j.stamet.2008.12.004
- Cramer E. Logconcavity and unimodality of progressively censored order statistics. Stat Probab Lett. 2004;68:83–90. doi: 10.1016/j.spl.2004.01.016
- Cramer E, Kamps U, Rychlik T. Unimodality of uniform generalized order statistics, with applications to mean bounds. Ann Inst Stat Math. 2004;56:183–192. doi: 10.1007/BF02530531
- Cuculescu I, Theodorescu R. Multiplicative strong unimodality. Aust N Z J Stat. 1998;40:205–214. doi: 10.1111/anzs.1998.40.issue-2
- Cramer E. Dependence structure of generalized order statistics. Statistics. 2006;40:409–413. doi: 10.1080/02331880600822291
- Navarro J. Introduction to system reliability theory. Cham: Springer; 2021.
- Navarro J, Calı C, Longobardi M, et al. Distortion representations of multivariate distributions. Stat Methods Appl. 2022;31:925–954. doi: 10.1007/s10260-021-00613-2
- Bieniek M. Variation diminishing property of densities of uniform generalized order statistics. Metrika. 2007;65:297–309. doi: 10.1007/s00184-006-0077-4
- Cramer E, Kamps U. Estimation with sequential order statistics from exponential distributions. Ann Inst Stat Math. 2001;53:307–324. doi: 10.1023/A:1012470706224
- Hermanns M, Cramer E, Ng HKT. EM algorithms for ordered and censored system lifetime data under a proportional hazard rate model. J Stat Comput Simul. 2020;90:3301–3337. doi: 10.1080/00949655.2020.1800706
- Glen AG. Accurate estimation with one order statistic. Comput Stat Data Anal. 2010;54:1434–1441. doi: 10.1016/j.csda.2010.01.012
- Marshall AW, Olkin I. Life distributions. structure of nonparametric, semiparametric, and parametric families. New York (NY): Springer; 2007.
- Zografos K, Balakrishnan N. On families of beta- and generalized gamma-generated distributions and associated inference. Stat Methodol. 2009;6:344–362. doi: 10.1016/j.stamet.2008.12.003
- Cordeiro GM, Brito RS. The beta power distribution. Braz J Probab Stat. 2012;26:88–112. doi: 10.1214/10-bjps124
- Arnold BC, Balakrishnan N, Nagaraja HN. Records. New York: Wiley; 1998.
- Balakrishnan N, Cramer E, Kundu D. Hybrid censoring know-how – designs and implementations. Cambridge: Academic; 2023.