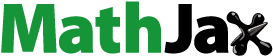
Abstract
We present conditions for Hölder calmness and upper Hölder continuity of optimal solution sets to perturbed optimization problems in finite dimensions. Studies on Hölder type stability were a popular subject in variational analysis already in the 1980s and 1990s, and have become a revived interest in the last decade. In this paper, we focus on conditions for Hölder calmness of the argmin mapping in the case of non-isolated minima. We recall known ideas and results in this context for general as well as special parametric programs, refine them and discuss particular settings, including nonlinear programs and convex semi-infinite optimization problems.
1. Introduction
Given a metric space , a function
, a multifunction
and a reference point
, we study in this paper the parametric optimization problem
(1)
(1) and special realizations of this basic model. Recall that the graph and the domain of a multifunction
are defined by
and
, respectively, and Φ is closed if
is a closed set.
We are going to present sufficient conditions for (q-order) Hölder calmness of the argmin mapping (also called optimal set mapping) of the problem (Equation1(1)
(1) ),
Recall (cf. [Citation1,Citation2]) that for
, a multifunction
is called q-order calm at
if for some
,
(2)
(2) where
for
is possible. Here
and
denote the closed ε- and δ-neighbourhood of
and
, respectively, and
is the closed unit ball in
. In the case q = 1 we say that Φ is calm or proper calm at
. If in (Equation2
(2)
(2) ) the restriction of
to
is omitted, Φ is called upper Hölder continuous of order q at
. Though our approaches and results will include the case q = 1, we are mainly interested in the q-order for
.
The study of Hölder calmness and upper Hölder continuity of minimizing sets was rather popular in the 1980s and 1990s, see, e.g. [Citation3–12]. Sufficient conditions were given by assuming constraint qualifications (or related regularity assumptions), combined with the second-order analysis of the data, including 2nd- or higher-order growth conditions. Under assumptions ensuring that the initial solution is locally isolated, a complete theory was developed at that time, see the book by Bonnans and Shapiro [Citation12]. For the general case
, we refer e.g. to Sect. 4.4 and Sect. 4.9 in [Citation12] and to the papers [Citation6,Citation9–11].
In the last decade, there is a revived interest in studying Hölder calmness and closely related properties (q-order metric subregularity, q-order local error bounds) in applications to optimization, we refer e.g. to the papers [Citation1,Citation2,Citation13–17].
However, Hölder calmness of the argmin mapping has been recovered only in a few papers. In [Citation17, Thm. 4], weak constraint qualifications and a (refined) strong quadratic growth condition were assumed to obtain -order upper Lipschitz behaviour of stationary solutions and minimizing sets for a class of parametric problems which include perturbed mathematical programs with equilibrium or vanishing constraints (MPECs or MPVCs). This result is not covered by classical ones, though, the assumptions imply that the initial stationary solution to
is locally isolated. The authors of [Citation2, Sect. 4] use an approach via Hölder error bounds to get q-order calmness of the argmin mapping of a parametric convex semi-infinite program and of an associated inequality system.
In our paper, we present two types of sufficient conditions for Hölder calmness of the argmin mapping of the parametric problem (Equation1(1)
(1) ), where we focus on the general case that
is not a singleton. By the first approach, we refine a result obtained in Theorem 2.2 of [Citation6]. In contrast to a stronger regularity requirement in [Citation6], we assume now only calmness and Lipschitz lower semicontinuity at the initial pair
, and the applied growth condition is supposed to hold only locally. The second approach is borrowed from the idea in [Citation2] to use q-order calmness of an auxiliary multifunction (which is in special cases the solution set mapping of an inequality system) as a sufficient condition for the q-calmness of the argmin mapping, see also [Citation18,Citation19] for the case q = 1. This allows the application of conditions for the Hölder calmness of level set mappings, which are given e.g. in [Citation1,Citation2,Citation14,Citation20].
The paper is organized as follows. After presenting some notation and prerequisites in Section 2, we show in Section 3 that relatively mild regularity assumptions and a growth condition of order ensure calmness of order
for the argmin mapping of (Equation1
(1)
(1) ). The statement and its assumptions are discussed for special settings and results known from the literature. In Section 4, we prove that q-order calmness of minimizing sets can be checked by known conditions for q-order calmness of a related auxiliary mapping.
2. Notation and preliminaries
To define further continuity concepts besides that of q-order calmness (Equation2(2)
(2) ), let
be a multifunction with some given
. Φ is said to have the Aubin property at
Footnote1 iff there are
such that
(3)
(3)
Defining (
,
), Φ is called Lipschitz lower semicontinuous (Lipschitz l.s.c.) at
Footnote2 iff there are
such that
(4)
(4) Obviously, the Aubin property implies both calmness and Lipschitz lower semicontinuity. Note that the opposite direction fails, let e.g.
with
for
,
and
. If
holds in the definition (Equation2
(2)
(2) ), we speak of isolated q-order calmness.
We say that Φ is lower semicontinuous (l.s.c.) at iff
(5)
(5) Note that (Equation5
(5)
(5) ) (and hence (Equation4
(4)
(4) )) implies for each
,
if t is sufficiently close to
.
Φ is called upper semicontinuous in Berge's sense (B-u.s.c.) at iff for any open set
there is some neighbourhood
of
such that
for all
.
Given the parametric program (Equation1(1)
(1) ), M will be called its feasible set mapping, while
denotes its infimum value function. Given
and a closed set
, we define
(6)
(6)
Lemma 2.1
Consider the parametric optimization problem (Equation1(1)
(1) ) under the assumptions imposed there. Given
and
, let
and suppose that M is l.s.c. at
. Then
is B-u.s.c. at
.
Proof.
As assumed in (Equation1(1)
(1) ), M is closed at
, and so
is closed at
, too. Since V is compact,
is B-u.s.c. at
, by [Citation28, Lemma 2.2.3]. Further, M is l.s.c. at
, i.e.
as
. Hence, there is some
such that
for all
, and so
as
, i.e.
is l.s.c. at
. Since f is continuous at
and for every
,
is – by the Weierstrass theorem – a nonempty subset of the compact set
, then both Theorem 4.2.2(1') and Theorem 4.2.1(4) in [Citation28] apply, and ensure that
is B-u.s.c. at
.
We conclude the section by some notation. denotes any norm in
, and
is the open unit ball in this norm. Given a compact subset I of a metric space,
denotes the linear space of continuous functions
equipped with the norm
. By
(or
) we abbreviate the property that h is a k-times continuously differentiable function (or a
function with locally Lipschitzian derivative Dh). Let
be the set of nonnegative real numbers, and
. For brevity, we write
.
3. Hölder calmness under a growth condition
In this section, we present sufficient conditions for Hölder calmness of minimizing sets under certain regularity properties for the constraints and a growth condition for the objective function. Consider the parametric model (Equation1(1)
(1) ).
As usual in the literature (see e.g. [Citation12, Def. 3.1, Def. 3.141]), we say that for a given nonempty closed set and a constant
,
satisfies the local κ-order growth condition at
with respect to S if for some open neighbourhood
of
and a constant c>0,
(7)
(7) holds true. Obviously,
for all
, and
is a set of local minimizers of
. If (Equation7
(7)
(7) ) even holds for some open set
, we say that
satisfies
at S. In this case, S is called a set of weak sharp minima of order κ, see [Citation29]. If
satisfies
at a compact set S, then S is a complete local minimizing set (CLM set) in the sense of Robinson [Citation30], i.e.
holds for some open set
. Without loss of generality we will work with
, the analysis could be similarly done for CLM sets.
Theorem 3.1
Consider the parametric model (Equation1(1)
(1) ). Let
be an optimal solution of
. Suppose that M is calm and Lipschitz l.s.c. at
, and
satisfies the local κ-order growth condition (Equation7
(7)
(7) ) at
with respect to
. Then the argmin mapping Ψ is
-order calm at
.
Proof.
First we make some preparations. By the assumptions, is a closed set. Further, M is calm and Lipschitz l.s.c. at
, hence there are
such that
(8)
(8)
(9)
(9) Note that we have taken the same constant
both in (Equation8
(8)
(8) ) and (Equation9
(9)
(9) ) without losing generality, similarly for
. Moreover, the Lipschitz property of f particularly means that with some positive constants
,
and
, the condition
(10)
(10) is fulfilled. As assumed, there exists a neighbourhood
of
such that, with some c>0, the growth condition (Equation7
(7)
(7) ) holds true. Now, choose
so that
(11)
(11) Let
and
,
, be defined according to (Equation6
(6)
(6) ).
By Lemma 2.1, is B-u.s.c. at
. Hence, for some
one has
(12)
(12) Suppose that δ is a constant satisfying
(13)
(13) which finishes our preparations. Now we are going to prove that for some
,
(14)
(14) This immediately implies that Ψ is
-order calm at
, since for
,
(15)
(15) Indeed, if
then
holds for some
and (especially) for all
so
and
To show (Equation14(14)
(14) ), consider any
and
, where ε, δ and V are given according to (Equation11
(11)
(11) ) and (Equation13
(13)
(13) ), respectively. Next we apply the existence of auxiliary points
and
such that (by calmness and Lipschitz l.s.c.) Lipschitz estimates for
and
hold in terms of
. Along with the growth condition, the latter will be used for estimates in terms of the locally Lipschitzian function f.
Using (by
), (Equation8
(8)
(8) ) and (Equation13
(13)
(13) ), we get
(16)
(16) where
, and so, according to (Equation7
(7)
(7) ) and
,
(17)
(17) Because of (Equation9
(9)
(9) ) and (Equation13
(13)
(13) ), we have
(18)
(18) Note that
,
and
. By defining
(Equation10
(10)
(10) ), (Equation16
(16)
(16) ) and (Equation18
(18)
(18) ) then give
Using these estimates, one has
Therefore, together with (Equation17
(17)
(17) ),
which entails
(19)
(19) To finish the proof, let now
Using (Equation12
(12)
(12) ) and (Equation16
(16)
(16) ), we then have
Hence, again with (Equation16
(16)
(16) ),
and so, by applying the mean value theorem to the function
, for
,
This implies
(20)
(20) while we have from (Equation19
(19)
(19) ),
and
(Equation18
(18)
(18) ), by definition of
,
Combining this with (Equation20
(20)
(20) ), we thus obtain
By setting
, (Equation14
(14)
(14) ) is shown. This completes the proof.
Note. According to (Equation15(15)
(15) ),
only holds if
. Under the assumptions of the theorem,
is not automatically nonempty for t near
. Consider for
the example of minimizing the function
(or some smoothed modification) subject to
at
.
Corollary 3.1
Consider the parametric model (Equation1(1)
(1) ). Suppose that for some
,
is nonempty and compact, M is calm and Lipschitz l.s.c. at
for each
, and for some
,
satisfies
at S. Then there exist some open bounded set
and some constant
such that for t near
,
(21)
(21)
Proof.
The assumptions here ensure that the assumptions of Theorem 3.1 are fulfilled for each (x now replaces
). By this theorem, we find that for each
, there are positive constants
,
and
such that if
, then
(22)
(22) The sets
,
, define a covering of S by open balls. Now, standard compactness arguments apply. Indeed, since S is compact, there are finitely many points
such that
, where Q is open. Putting
, and
, we see that (Equation22
(22)
(22) ), applied to
, implies
provided that
. This completes the proof.
Let us now discuss specializations and the assumptions of the preceding theorem and its corollary, where we focus on the case of non-isolated initial solutions (i.e. is not a singleton) and q-order calmness for
.
Note that Hölder stability for isolated solutions of is almost completely handled for nonlinear programs and further classes of optimization problems in Chapters 4 and 5 of [Citation12]. Further, note that the assertion of Corollary 3.1 can be strengthened for parametric nonlinear programs with
or
data. For such problems even calmness (i.e.
in (Equation21
(21)
(21) )) holds provided that the quadratic growth condition
at
and the linear independence constraint qualification are satisfied, see e.g. [Citation6, Thm. 3.3] or similar results in [Citation7] and [Citation12, § 4.9.3]. Calmness of minimizing sets also holds for certain convex parametric programs with fixed constraint set under a so-called strong quadratic growth condition, see [Citation9,Citation10].
Theorem 3.1 and its corollary modify and refine the result of Theorem 2.2 in [Citation6]. In particular, they are given in the up-to-date language of Hölder calmness and under weaker regularity properties of the constraints. Note that some ideas in the above proof go already back to [Citation3] where a standard nonlinear program with smooth constraints for was studied.
In the literature on Hölder stability of isolated minimizers or minimizing sets, the constraint set mapping M is usually supposed to have the Aubin property at (cf. e.g. [Citation2,Citation3,Citation7,Citation12]) which implies (Equation8
(8)
(8) ) and (Equation9
(9)
(9) ), or the Lipschitz l.s.c. assumption (Equation9
(9)
(9) ) at
is replaced by the stronger requirement
(23)
(23) cf. [Citation5,Citation6].
Example 3.1
[Citation32, Example 1]
Property (Equation23(23)
(23) ) and hence the Aubin property are already violated in the very simple example
,
On the other hand, Γ is calm and Lipschitz l.s.c. at
.
A typical realization of Corollary 3.1 concerns the parameterized optimization problem
(24)
(24) where
, T is a Banach space,
is locally Lipschitz,
is a
function, and
is a closed convex set. Let M and Ψ be the feasible and optimal set mapping, respectively, of (Equation24
(24)
(24) ). Robinson's constraint qualification (RCQ) [Citation33] holds at
if
which becomes the Mangasarian-Fromovitz constraint qualification (MFCQ) in the case
considered later.
Corollary 3.2
Consider the parametric problem (Equation24(24)
(24) ). Suppose that
is nonempty and compact, f satisfies
at S, and RCQ holds at every point
. Then there exist some open bounded set
and some
such that for t near
,
Proof.
The result immediately follows from Corollary 3.1, since M has the Aubin property at provided that RCQ holds at
, see [Citation33] or [Citation12, Prop. 2.89].
If f is assumed to be a function, the result of the preceding corollary is a special case of Proposition 4.41 in [Citation12] which was given in a Banach space setting for the functions f and G and the set K, and which, in addition, permits approximate solutions of
.
In Theorem 3.1 and its corollaries, the growth condition is a crucial (but abstract) requirement. Sufficient conditions or characterizations for
in the case of non-isolated minima are rare. For general
one finds such conditions in terms of suitable higher-order directional derivatives in [Citation34] (see also the references therein); however, it is not easy to verify them. This is possible in particular for
under smoothness or convexity assumptions, cf. e.g. [Citation12,Citation29,Citation34]. If f is the point-wise maximum of finitely many
functions, characterizations of
for non-isolated minima can be found in [Citation10,Citation11]. Concerning quadratic growth in programs of the type (Equation24
(24)
(24) ) with
data f and G, we refer to [Citation12, Sect. 3.5] for criteria in terms of the given problem. In particular, the local quadratic growth condition holds under some local 2nd-order sufficient optimality condition, see for example [Citation12, Thm. 3.150] or [Citation35].
Calmness for various types of constraint systems has been studied extensively, see e.g. [Citation1,Citation36–42]. As mentioned above, M is calm and Lipschitz l.s.c. at provided that M has the Aubin property at this point. Characterizations and applications of the Aubin property are well-studied for many classes of multifunctions and variational problems, we refer here exemplarily to the books [Citation12,Citation22–25,Citation43] for details. Therefore, Theorem 3.1 has potential applications to different types of models.
can represent not only standard constraint systems, but also, for example, solutions of a complementarity problem, of a generalized equation, or of a (lower level) optimization problem under perturbations.
For special constraint set mappings, the differences between Aubin property, Lipschitz lower semicontinuity and property (Equation23(23)
(23) ) are marginal, but do exist. An interesting study of this phenomenon and of the related moduli in the context of (finite and semi-infinite) linear inequality systems has been recently published in [Citation32]. Further, the mapping in Example 3.1 is a special polyhedral multifunction which does not satisfy the Aubin property, but is Lipschitz l.s.c. and calm. On the other hand, it is well-known that in some standard situations, the feasible set mapping M of problem (Equation1
(1)
(1) ) is calm and Lipschitz l.s.c. if and only if it has the Aubin property. This is recalled in the next remark.
Remark 3.1
It is known that for a canonically perturbed constraint system
(25)
(25) with
, the implication
(and so the equivalence) holds for two standard situations, namely
if all
,
, are
functions,
or if
are convex and
(
,
),
.
For (i), the implication was given in [Citation41, Lemma 1], by showing that the Lipschitz l.s.c. property implies the MFCQ which is equivalent with the Aubin property [Citation33]. For (ii) one even has: if is l.s.c. at
then
for small
, which implies the generalized Slater CQ (i.e.
holds for some
, and
are linearly independent) and hence (see [Citation44]) the Aubin property.
We recall an example from [Citation45] to illustrate that the independent perturbation of all parameters in the system (Equation25(25)
(25) ) is crucial to get the equivalence (i) discussed in Remark 3.1.
Example 3.2
[Citation45, Example 2.5]
Define the multifunction ,
Obviously,
Hence,
for all
and
for all t, and so Ω is in particular calm and Lipschitz l.s.c. at
. On the other hand, Ω has not the Aubin property, since for any t>0, one has
and (for the Euclidean norm
)
It is easy to see that MFCQ does not hold at
.
Let us continue with a brief reference to conditions in [Citation17], which guarantee calmness and Lipschitz lower semicontinuity for a solution mapping defined by disjunctive constraints, but do not imply that the Aubin property is satisfied.
Remark 3.2
Consider the constraint set mapping M of the parametric problem (Equation24(24)
(24) ), but assume that K is the union of finitely many convex polyhedra, and G is a
function. Let
. In [Citation17], the so-called first order sufficient condition for metric subregularity (FOSCMS) of the mapping
at
(i.e. its inverse is calm at
) is used as constraint qualification.FOSCMS is based on Gfrerer's concept [Citation46] of a limiting normal cone to a subset K in direction u and guarantees that M is Lipschitz l.s.c. and calm, see [Citation17, Prop. 1]. In special settings, this constraint qualification can be rewritten in terms of the problem data. This has been done e.g. for MPECs, see [Citation17, Sect. 5].
It is worth noting that FOSCMS, in general, does not imply metric regularity of , which equivalently means that the Aubin property for
does not follow automatically. The latter is demonstrated by [Citation17, Example 3] for a special MPEC. This is in contrast to standard nonlinear programs (see Remark 3.1).
We conclude this section by a remark concerning the case that an argmin mapping acts as the constraint set mapping M in the problem (Equation1(1)
(1) ). Then it may happen that the Lipschitz l.s.c. assumption on M leads to a special (and less interesting) setting.
Remark 3.3
[Citation25, Sect. 4.2]
Consider the optimization problem
where X is a nonempty subset of a real Hilbert space T, f is any function from X to
, and t varies near
. Let
Then there holds, by Theorem 4.8 in [Citation25]: If
and
is Lipschitz l.s.c. at
, then
and
is isolated calm at
.
Thus, if is Lipschitz l.s.c., then both M and Ψ in (Equation1
(1)
(1) ) are isolated calm at
. Note that
is even locally single-valued near
if
has the Aubin property at this point [Citation25, Cor. 4.7].
4. Hölder calmness via associated inequalities
Following the ideas in [Citation18,Citation19], we show that q-order calmness of minimizing sets for the basic model (Equation1(1)
(1) ) is implied by the corresponding calmness property for the solution set of some associated inequality system. This extends partially Theorem 4.7 in [Citation2] which was proved in the context of convex semi-infinite programs under canonical perturbations.
First we recall an auxiliary result on (proper) calmness of optimal values.
Lemma 4.1
[Citation19, Lemma 3.1]
Consider the problem (Equation1(1)
(1) ). Given
, suppose that M is calm and Lipschitz l.s.c. at
. Then there exists some
such that, with
, the function
is calm at
, i.e.
holds for some neighbourhood U of
, some constant
and all
.
The q-order calmness of the optimal set mapping Ψ of (Equation1(1)
(1) ) will be related to the multifunction
(26)
(26) To prove the next theorem, one can argue the same way as in the case q = 1, which is handled in [Citation19, Thm. 3.1]. For completeness, we give the simple proof.
Theorem 4.1
Consider the problem (Equation1(1)
(1) ). Suppose that for the reference point
,
(i) | the feasible set mapping M is calm and Lipschitz l.s.c. at | ||||
(ii) | for |
Then the argmin mapping Ψ is q-order calm at .
Proof.
The assumptions of the theorem imply that for some constants , with
,
and
,
(27)
(27)
(28)
(28) Since the assumptions of Lemma 4.1 are satisfied, we conclude that for some modulus
and some neighbourhood
of
,
(29)
(29) Now let
be a neighbourhood of
such that for all
and all
both
(30)
(30) hold true, where (Equation28
(28)
(28) ) was used. By definition and (Equation15
(15)
(15) ), one has
,
,
and
Consider any
and suppose
, otherwise q-order calmness is trivially satisfied. Hence, we obtain
and
from (Equation15
(15)
(15) ), and by (Equation29
(29)
(29) ) and (Equation30
(30)
(30) ),
So, we can apply (Equation27
(27)
(27) ) (recall
and
), and it follows
Then
yields
This completes the proof.
Let us continue with a discussion of the above result for semi-infinite or finite parametric optimization problems of the type
(31)
(31) where I is any compact set in a metric space,
(
) are locally Lipschitz,
and
are continuous, and
varies in the parameter space
near some given
. If
, this reduces to a standard nonlinear program.
In the analysis of the model (Equation31(31)
(31) ), let
,
and
symbolize the constraint set mapping, the optimal set mapping and the optimal value function. The auxiliary mapping L in (Equation26
(26)
(26) ) now becomes
(32)
(32) where b varies near
and μ varies near
. Given
and the optimal value
, we define the auxiliary function,
Corollary 4.1
Consider the problem (Equation31(31)
(31) ). Suppose that the feasible set mapping M is calm and Lipschitz l.s.c. at
. Then the argmin mapping Ψ is q-order calm at
with
if the multifunction L (Equation32
(32)
(32) ) is q-order calm at
, or, equivalently, if the level set map
(33)
(33) is q-order calm at
.
Proof.
Obvious from Theorem 4.1 and the definitions of L (Equation32(32)
(32) ) and H.
For level set mappings, the following characterization of q-order calmness has been given by the second author in [Citation1, Prop. 3.4] (see also [Citation14, Cor. 4.3]):
Let X be a complete metric space, be lower semicontinuous and
. The level set map
is q-order calm at
if and only if there are
such that
(34)
(34) This can be reformulated in certain procedures to check q-order calmness, see [Citation1] for details. An application to standard nonlinear programs in the case
is discussed below.
Consider now the model (Equation31(31)
(31) ) under the assumptions
(35)
(35) In this case, Corollary 4.1 covers the implications (iii) ⇒ (i) ⇒ (ii) of Theorem 4.7 in [Citation2], which was given under the assumption that
satisfies the Slater CQ (i.e.
). It is known (cf. [Citation44]) that the Slater CQ implies the Aubin property, and vice versa, hence M is Lipschitz l.s.c. and calm.
Remark 4.1
Theorem 4.7 in [Citation2] is proved by showing and utilizing that q-order calmness of L is equivalent to the property for H to have a q-order error bound. The latter holds if and only if
see [Citation2, Prop. 4.3], where
means the subdifferential of convex analysis.
An important additional result of [Citation2, Thm. 4.7] is that under Slater CQ the opposite direction of Corollary 4.1 holds if h and are linear.
For a standard nonlinear program with data, a sufficient condition for the
-order Hölder calmness of inequality systems, given in [Citation1], can be immediately used. Consider the model (Equation31
(31)
(31) ) and assume that
(36)
(36) Then Theorem 4.11 in [Citation1] gives that
(Equation33
(33)
(33) ) is
-order calm at
if
or if (otherwise) there exists some
such that
(37)
(37) where
denotes Clarke' subdifferential [Citation47]. Note that (Equation37
(37)
(37) ) is equivalent to some injectivity condition on the contingent derivative of
at
, for details of this characterization we refer to [Citation1, Sect. 4.2].
Finally, we recall from [Citation19, Prop. 4.2] a refined version of Theorem 4.1 for the parametric problem (Equation1(1)
(1) ) in the case of proper calmness (i.e. q = 1). There it was shown that the multifunction L (Equation26
(26)
(26) ) is calm at
provided that
(i) M is calm and Lipschitz l.s.c. at ,
(ii) the level set map is calm at
, and
(iii) the restricted level set map is calm at
.
In the proof of this statement, an intersection theorem for calm multifunctions is applied (cf. [Citation25, Thm. 2.5]), and it is used that for locally Lipschitzian f the inverse of the level set mapping
has the Aubin property at
.
For a detailed discussion of further specializations to the settings (Equation35(35)
(35) ) and (Equation36
(36)
(36) ) in the case q = 1, we refer to [Citation19, Sect. 4].
KK-class-style-files.rar
Download (27.2 KB)Acknowledgments
The authors are indebted to the referees and the associate editor for the very careful reading of the original manuscript and several constructive remarks that improved the presentation.
Disclosure statement
No potential conflict of interest was reported by the author(s).
Notes
1 This concept was first studied in [Citation21,Citation22] and originally designated pseudo-Lipschitz continuity; the name Aubin property goes back to [Citation23]. Further, in [Citation24], the term Lipschitz-like was introduced for this property.
2 The terminology Lipschitz l.s.c. is taken from the authors' book [Citation25]. In the more recent literature, one finds also names like recession with linear rate [Citation26] or inner calm [Citation27] for this concept.
3 In [Citation5] the name pseudo-Lipschitzian* was suggested for a multifunction which is calm and satisfies~(23), see also [Citation6,Citation31] for its application in stability analysis. If only~(23) holds, the name Lipschitz-l.s.c.* was recently introduced in [Citation32].
References
- Kummer B. Inclusions in general spaces: Hoelder stability, solution schemes and Ekeland's principle. J Math Anal Appl. 2009;358:327–344.
- Kruger AY, López MA, Yang XQ, et al. Hölder error bounds and Hölder calmness with applications to convex semi-infinite optimization. Set-Valued Var Anal. 2019;27:995–1023.
- Alt W. Lipschitzian perturbations of infinite optimization problems. In: Fiacco AV, editor. Mathematical programming with data perturbations. New York: Marcel Dekker; 1983. p. 7–21
- Auslender A. Stability in mathematical programming with nondifferentiable data. SIAM J Control Optim. 1984;22:239–254.
- Klatte D. A note on quantitative stability results in nonlinear programming. Berlin: Sektion Mathematik, Humboldt-Universität Berlin; 1987. p. 77–86 (Seminarbericht Nr. 90).
- Klatte D. On quantitative stability for non-isolated minima. Control Cybernet. 1994;23:183–200.
- Shapiro A. Perturbation theory of nonlinear programs when the set of solutions is not a singleton. Appl Math Optim. 1988;18:215–229.
- Shapiro A. Perturbation analysis of optimization problems in Banach spaces. Numer Funct Anal Optim. 1992;13:97–116.
- Ioffe AD. On sensitivity analysis of nonlinear programs in Banach spaces. The approach via unconstrained optimization. SIAM J Optim. 1994;4:1–43.
- Bonnans JF, Ioffe AD. Quadratic growth and stability in convex programming problems with multiple solution. J Convex Anal. 1995;2:41–57.
- Bonnans JF, Ioffe AD. Second-order sufficiency and quadratic growth for nonisolated minima. Math Oper Res. 1995;20:801–817.
- Bonnans JF, Shapiro A. Perturbation analysis of optimization problems. New York: Springer; 2000.
- Gaydu M, Geoffroy MH, Jean-Alexis C. Metric subregularity of order q and the solving of inclusions. Cent Eur J Math. 2011;9:147–161.
- Klatte D, Kruger A, Kummer B. From convergence principles to stability and optimality conditions. J Convex Anal. 2012;19:1043–1072.
- Li G, Mordukhovich BS. Hölder metric subregularity with applications to proximal point method. SIAM J Optim. 2012;22(4):1655–1684.
- Kruger AY. Error bounds and Hölder metric subregularity. Set-Valued Var Anal. 2015;23(4):705–736.
- Gfrerer H, Klatte D. Lipschitz and Hölder stability of optimization problems and generalized equations. Math Program Ser A. 2016;158:35–75.
- Cánovas MJ, Hantoute A, Parra J, et al. Calmness of the argmin mapping in linear semi-infinite optimization. J Optim Theory Appl. 2014;160:111–126.
- Klatte D, Kummer B. On calmness of the argmin mapping in parametric optimization problems. J Optim Theory Appl. 2015;165:708–719.
- Yao JC, Zheng XY. Error bound and well-posedness with respect to an admissible function. Appl Anal. 2016;95(5):1070–1087.
- Aubin J-P. Lipschitz behavior of solutions to convex minimization problems. Math Oper Res. 1984;9:87–111.
- Aubin J-P, Ekeland I. Applied nonlinear analysis. New York: Wiley; 1984.
- Rockafellar RT, Wets RJ-B. Variational analysis. Berlin: Springer; 1998.
- Mordukhovich BS. Variational analysis and generalized differentiation I II. Berlin: Springer; 2006.
- Klatte D, Kummer B. Nonsmooth equations in optimization – regularity, calculus, methods and applications. Dordrecht: Kluwer Academic; 2002.
- Ioffe AD. Variational analysis of regular mappings: theory and applications. Cham: Springer; 2017.
- Benko M, Gfrerer H, Outrata JV. Calculus for directional limiting normal cones and subdifferentials. Set-Valued Var Anal. 2019;27:713–745.
- Bank B, Guddat J, Klatte D, et al. Non-linear parametric optimization. Berlin: Akademie; 1982. Basel: Birkhäuser; 1983.
- Burke JV, Ferris MC. Weak sharp minima in mathematical prgramming. SIAM J Control Optim. 1993;31:1340–1359.
- Robinson SM. Local epi-continuity and local optimization. Math Program. 1987;37:208–222.
- Römisch W, Schultz R. Distribution sensitivity in stochastic programming. Math Program. 1991;50:197–226.
- Cánovas MJ, Gisbert MJ, Henrion R, et al. Lipschitz lower semicontinuity moduli for linear inequality systems. J Math Anal Appl. 2020;490(2):124313.
- Robinson SM. Stability theorems for systems of inequalities. Part II: differentiable nonlinear systems. SIAM J Numer Anal. 1976;13:497–513.
- Studniarski M, Ward DE. Weak sharp minima: characterizations and sufficient conditions. SIAM J Control Optim. 1999;38:219–236.
- Bonnans JF, Cominetti R, Shapiro A. Second order optimality conditions based on parabolic second order tangent sets. SIAM J Optim. 1999;9:466–492.
- Robinson SM. Some continuity properties of polyhedral multifunctions. Math Program Study. 1981;14:206–214.
- Li W. Abadie's constraint qualification, metric regularity, and error bounds for differentiable convex inequalities. SIAM J Optim. 1997;7:966–978.
- Pang J-S. Error bounds in mathematical programming. Math Program. 1997;79:299–332.
- F. Facchinei F, Pang J-S. Finite-dimensional variational inequalities and complementary problems, I, II. New York: Springer; 2003.
- Henrion R, Outrata J. Calmness of constraint systems with applications. Math Program Ser B. 2005;104:437–464.
- Klatte D, Kummer B. Optimization methods and stability of inclusions in Banach spaces. Math Program Ser B. 2009;117:305–330.
- Gfrerer H. First order and second order characterizations of metric subregularity and calmness of constraint set mappings. SIAM J Optim. 2011;21:1439–1474.
- Dontchev AL, Rockafellar RT. Implicit functions and solution mappings. A view from variational analysis. 2nd ed. 2014. Dortrecht: Springer; 2009.
- Robinson SM. Regularity and stability for convex multivalued functions. Math Oper Res. 1976;1:130–143.
- Fusek P, Klatte D, Kummer B. Examples and counterexamples in Lipschitz analysis. Control Cybernet. 2002;31(3):471–492.
- Gfrerer H. On directional metric regularity, subregularity and optimality conditions for nonsmooth mathematical programs. Set-Valued Var Anal. 2013;21:151–176.
- Clarke FH. Optimization and nonsmooth analysis. New York: Wiley; 1983.