ABSTRACT
Social bots are computer software designed for content production and interaction with humans. With the popularity of images in social networks, social bots need to have visual awareness of image content while only understanding texts is far from enough to be active in social networks. We introduce a novel task, Visual Social Comment (VSC), in which social bots should generate relevant and informative comments on social contents of both images and texts. In this task of multimodal context, our work focuses on how to extract and fuse the information of vision and text to improve the quality of generated comments, and how to deal with the problem that neural dialog models trained with maximum likelihood estimation (MLE) criteria tend to generate generic responses. In order to fuse visual and textual context features closely through the relationship between them, we adopt joint attention of multimodal context to modify the standard sequence-to-sequence (Seq2Seq) framework. We also leverage the topic information transferred from a topic classification model to build a perceptual loss function, which encourages the generative comment model to generate more informative and diverse comments with the topic corresponding to context. The experimental results of models trained with data from Sina Weibo show that comments generated by our proposed models achieve better performance in both relevance and informativeness than those generated by other baseline models.
Disclosure statement
No potential conflict of interest was reported by the author(s).
ORCID
Additional information
Funding
Notes on contributors
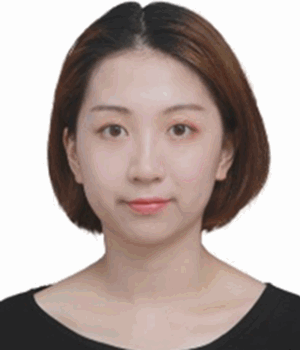
Yue Yin
Yue Yin received the B.S. degree in Electronics and Information Engineering from Shanghai University, China, in 2017, where she is currently pursuing the M.S. degree. Her research interest is natural language processing. E-mail: [email protected]
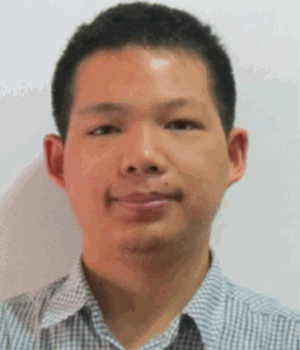
Hanzhou Wu
Hanzhou Wu received both B.S. and Ph.D. from Southwest Jiaotong University, Chengdu, China, in 2011 and 2017. From 2014 to 2016, he was a visiting scholar in New Jersey Institute of Technology, New Jersey, United States. He was a researcher in Institute of Automation, Chinese Academy of Sciences, from 2017 to 2019. Currently, he is an Assistant Professor in Shanghai University, China. His research interests include information hiding, graph theory and game theory. He has published around 20 papers in peer journals and conferences such as IEEE TDSC, IEEE TCSVT, IEEE WIFS, ACM IH&MMSec, and IS&T Electronic Imaging, Media Watermarking, Security and Forensics. E-mail: [email protected]
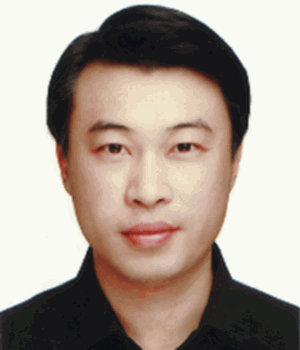
Xinpeng Zhang
Xinpeng Zhang received the B.S. degree in computational mathematics from Jilin University, China, in 1995, and the M.E. and Ph.D. degrees in communication and information system from Shanghai University, China, in 2001 and 2004, respectively. Since 2004, he has been with the faculty of the School of Communication and Information Engineering, Shanghai University, where he is currently a professor. His research interests include information hiding, image processing, and digital forensics. He has published over 200 papers in these areas.