Abstract
Facial expression recognition (FER) plays a vital role in image processing according to the widespread development of human interactive applications. In the past few years, various researchers have focused on FER for implementing it in different applications. The existing system suffers from various complexities such as low accuracy, computational cost, and poor recognition performances. In this article, we proposed a novel concept to recognize facial expressions. The proposed work comprises three sections that are pre-processing, feature extraction, and classification. The pre-processing techniques remove the unwanted data from the original image and enhance the crucial details for further processing. The Convolutional Neural Network (CNN) is used for feature extraction. But, it yields lower performance in terms of feature extraction due to the shortage of hyperparameter tuning. Hence, the Multi-strategy Gravitational Search Algorithm (M-GSA) is utilized to extract the facial expression features from the eyebrow movement, nose, chin, and lip corner of the facial images. The facial expressions are classified via the Support Vector Machine (SVM) classifier. In this work, the top five facial expressions such as surprise, sad, happy, fear, and angry with three facial expression datasets such as FER-2013 dataset, CK+ dataset, and JAFFE dataset. Ultimately, the proposed method demonstrates better classification accuracy and recognition rates than different kinds of state-of-art methods.
Acknowledgment
The Authors extend their warm thanks to Deanship of Scientific Research (DSR), King Saud University, Riyadh, Saudi Arabia for permitting us to carry out the research and also for acknowledging the financial aid from DSR through the Project Group No. RG-1441-343.
Correction Statement
This article has been republished with minor changes. These changes do not impact the academic content of the article.
Additional information
Notes on contributors
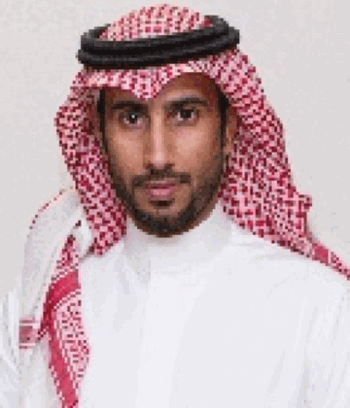
Wael Mohammad Alenazy
Wael Mohammad Alenazy is currently working as an assistant professor and chair of Self-Development Skills at Common First Year Deanship, King Saud University, Riyadh, Saudi Arabia. He received his PhD and master's degree from Faculty of Engineering and Information Technology, University of Technology, Sydney (UTS), Australia. His area of specialization includes advanced ICT solutions on smart environments and smart classroom. His current area of research interests includes smart learning, enhanced smart education, image processing and augmented reality.
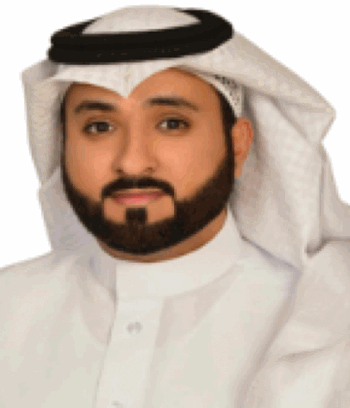
Abdullah Saleh Alqahtani
Abdullah Saleh Alqahtani is currently working as an assistant professor and coordinator- IT in Computer Science at Common First Year Deanship, Department of Self-Development Skills, King Saud University, Riyadh, Saudi Arabia. He received his PhD and master's degree from School of Computer Science, Engineering and Mathematics under the Faculty of Science and Engineering at Flinders University, Adelaide, Australia. His area of specializations includes data analytics on e-commerce, e-Business and data optimization by structural equation modeling. His current area of research interests includes optimization, computational algorithms, data science, deep learning and artificial intelligence. Email: [email protected]