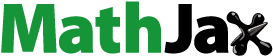
Abstract
It has been suggested for many years that students who are able to judge their own performance should do well in academic assessments. Despite the increasing number of empirical studies investigating the effect of self-assessment on academic performance, there has not been a recent synthesis of findings in the higher education context. The current meta-analysis aims to synthesise the effects of self-assessment on academic performance. In particular, it examines the difference between situations in which the process of self-assessment is revealed or observable (explicit) or not revealed or unobservable (implicit). A total of 98 effect sizes from 26 studies either reported a comparison between a group with self-assessment interventions and a control group (n = 20, k = 88) or a pre-post comparison (n = 6, k = 10). The overall effect of such interventions was significant (g = .455). Self-assessment interventions involving explicit feedback from others on students’ performance had a significantly larger effect size (g = .664) than those without explicit feedback (g = .213). There were no other significant moderators identified for either the overall effect or the effect of interventions involving explicit feedback.
Supplemental data for this article is available online at http://dx.doi.org/10.1080/02602938.2021.2012644 .
Introduction
A fundamental goal of higher education is to develop students’ capacity for life-long learning (Boud and Falchikov Citation2006), which is also one of the United Nation’s Sustainable Development Goals. Student self-assessment plays a pivotal role in achieving this goal because, from a pedagogical perspective, effective self-regulated or life-long learning is more likely to happen when students have a realistic sense of their own performance so that they can direct their further learning (Boud, Lawson, and Thompson Citation2013; Baas et al. Citation2015). Indeed, scholars advocate that self-assessment should be embedded in the curriculum so that students can develop skills and strategies for meaningful self-assessment that can lead to desirable short-term and long-term learning outcomes (Brown and Harris Citation2014; Yan Citation2020).
As self-assessment has great potential in enhancing learning outcomes when implemented for formative purposes, it has received considerable attention in higher education (Dochy, Segers, and Sluijsmans Citation1999; Sitzmann et al. Citation2010). An increasing number of empirical studies investigating the effect of self-assessment on academic performance have emerged. However, there is a lack of a current meta-analytical synthesis of the findings; most recent meta-analyses of the effect of self-assessment on academic performance were all in the K-12 context (e.g. Brown and Harris Citation2013; Sanchez et al. Citation2017; Youde Citation2019). In addition, there is a significant gap in understanding how to design effective self-assessment interventions. Self-assessment could be, and in many cases is, an implicit process without observable external evidence. A theoretical claim is that self-assessment should be made explicit, i.e. self-assessment actions should be observable or traceable, in order to enact their potential pedagogical and practical advantages (e.g. Panadero, Lipnevich, and Broadbent Citation2019; Nicol and McCallum Citation2021), because it is only with traceable actions that teachers can monitor the process and design interventions to improve their quality. However, no attempt has been made to draw together evidence about whether the explicit/implicit dimension of self-assessment influences academic performance. Thus, this paper aims to synthesise the effects of self-assessment and the difference between explicit and implicit self-assessment actions.
Self-assessment
Self-assessment has been used in many different ways (Andrade Citation2019). It can be conceptualised either as a personal ability/skill for evaluating one’s own knowledge, skills or performance, or as an instructional and learning process/practice (Yan Citation2016). Self-assessment can also serve both summative and formative purposes (Boud and Falchikov Citation1989; Panadero, Brown, and Strijbos Citation2016), and these different purposes shape how self-assessment is defined (Andrade Citation2019). The use of self-assessment for summative purposes is extremely demanding (Boud Citation1989) and the general consensus is that keeping self-assessment formative is more promising in enacting its merits in supporting student learning (Brown, Andrade, and Chen Citation2015; Yan and Brown Citation2017). In a state-of-the-art review, Panadero, Brown, and Strijbos (Citation2016) found 20 different categories of self-assessment implementations. These range from simply awarding a grade/mark to one’s own work (i.e. self-grading or self-marking) (Boud and Falchikov Citation1989) to more complex forms in which students seek and use feedback from various sources, evaluate and reflect on their own work against selected criteria to identify their own strengths and weaknesses (Yan and Brown Citation2017). In this paper we included in the meta-analysis empirical studies that used self-assessment, in simple or complex forms, for formative purposes.
The benefits of self-assessment on academic performance may be largely due to its intertwined relationship with self-regulated learning. Self-assessment requires students to judge the quality of their own work against selected criteria, identify the gap between their current and the desired performance standard and take actions to close that gap (Butler and Winne Citation1995; Andrade Citation2010). At each stage of self-regulated learning, the self-assessment process not only makes learning more goal-oriented and effective, but also equips students with plenty of learning opportunities (Yan and Boud Citation2022). For example, self-assessment facilitates goal setting by identifying personal and environmental resources and helps students search for appropriate learning strategies; it can be used to monitor the learning process, promote self-correction, and guide learning towards the learning goals; and it is also helpful for reflection on the learning outcomes and pointing out direction for future learning (Harris and Brown Citation2018; Yan Citation2020).
Making self-assessment explicit
As self-assessment requires students to evaluate their own work, it could be an implicit process, i.e. it happens in one’s head without observable evidence. This implicit, internal process can become explicit if the self-assessment actions are made observable, such as through discussing assessment criteria, seeking external feedback from others (e.g. teachers or peers), and writing down or voicing reflections on their performance. Explicit self-assessment with observable behaviours is desirable for two major reasons. Firstly, the merit of self-assessment resides in its role as an instructional and learning practice for the purpose of improving learners’ performance and directing their future learning (Boud and Falchikov Citation1989; Yan and Brown Citation2017). The problem with an implicit, internal process is that the absence of traceable evidence (Nicol and McCallum Citation2021) about what actions students actually take makes it difficult to monitor the process and to design interventions to improve its quality. Explicit self-assessment makes the internal process observable and, therefore, facilitates intentional instruction, support and intervention. Thus, to harness its pedagogical power, implicit self-assessment processes need to be made explicit. Through explicit processes, self-assessment strategies can be demonstrated and taught, and students’ self-assessment capacity is more likely to be developed, monitored and transferred to new contexts.
Secondly, self-assessment has to be carried out explicitly if it involves interactions with others (e.g. discussing criteria, seeking external feedback). Although self-assessment can be done without external inputs, meaningful self-assessment is usually not an isolated, individualised action (Boud Citation1999). The presence of ‘others’, in addition to ‘self’, can be crucial in self-assessment for improvement purposes (Brown and Harris Citation2013; Yan and Brown Citation2017). The power of self-assessment as an instructional and learning practice could be maximised if external feedback from teachers or peers were involved. This is because external feedback can reveal biases, which might have a negative impact on academic achievement, and help students to correct them (Panadero, Lipnevich, and Broadbent Citation2019).
To facilitate the shift from implicit self-assessment processes into explicit ones, teachers can use different scaffolding mechanisms (Panadero, Lipnevich, and Broadbent Citation2019). For example, teachers can make self-assessment criteria explicit, teach students how to apply them, provide feedback on students’ self-assessment, and facilitate re-calibration of self-assessment results (Boud, Lawson, and Thompson Citation2013; Panadero, Jonsson, and Botella Citation2017). These scaffolds could be used at different steps of the self-assessment process.
Prior Meta-analyses of the effect of self-assessment on academic performance
Meta-analytical reviews of the effect of self-assessment on academic performance are scarce in higher education. Most relevant meta-analyses, whether conducted decades ago (e.g. Boud and Falchikov Citation1989) or more recently (e.g. Sitzmann et al. Citation2010; Blanch-Hartigan Citation2011; Li and Zhang Citation2021), focus on the validity or accuracy of self-assessment by examining the relationship between self-assessment results and external performance criteria (e.g. teacher marks or examination scores). Meta-analyses on the effect of self-assessment on academic performance (e.g. Brown and Harris Citation2013; Sanchez et al. Citation2017; Youde Citation2019) are all from the K-12 setting, not higher education.
An additional limitation of past empirical studies and meta-analyses on this topic is that none have investigated whether making self-assessment explicit influences its effect on academic performance. This research gap needs to be filled to understand how to improve its impact on learning.
Moderators of the influences of self-assessment on academic performance
Despite the generally positive influence of self-assessment on academic performance, the effect sizes vary across contexts (Brown and Harris Citation2013). Thus, it is vital to explore the factors that may moderate the influence of self-assessment interventions, in particular, gender, student training, the use of online technology, assessment mode, research design and research quality.
Some studies (e.g. Andrade and Boulay Citation2003; Yan Citation2018) report that male and female students had different responses to self-assessment, while other studies found no gender differences (e.g. Andrade and Du Citation2007). It is, therefore, worthwhile to test again whether its effect varies across gender. In addition, the design and implementation of self-assessment may substantially influence its effect. For example, self-assessment may bring greater learning gains for students who receive training (Brown and Harris Citation2013), perhaps because those receiving training develop a better understanding of self-assessment and are more familiar with the process. The use of online technology has become increasingly popular in self-assessment, especially since the outbreak of COVID-19. However, there is still a lack of synthesis of empirical evidence regarding whether online technology can facilitate the implementation of self-assessment and lead to learning gains. Self-assessment may be conducted in a quantitative (e.g. scores and grades) or qualitative mode (e.g. comments). Feedback research has favoured qualitative comments over quantitative scores in influencing learning because the former is more supportive to learning, while the latter can be seen by students to be summative in character and, therefore, may inhibit further learning (Lipnevich, Berg, and Smith Citation2016). However, whether the same rationale can be applied in self-assessment contexts is unclear. The methodological characteristics of studies may also influence the effects of self-assessment interventions. Specifically, we examined whether the effect differs across research design (control group design and repeated measures design) and research quality.
Research aim and questions
The aim of the current meta-analytic review is twofold. Firstly, we examined the overall effect of self-assessment interventions on improving students’ academic performance, as well as the factors that moderate this effect. Secondly, we investigated the difference in the effect between explicit and implicit self-assessment on academic performance. The specific research questions (RQs) are:
RQ1: What is the overall effect of self-assessment interventions on students’ academic performance? What factors moderate this effect?
RQ2: Does explicit self-assessment have a greater effect on students’ academic performance compared with implicit self-assessment? What factors moderate the effect of explicit self-assessment?
Methods
Search strategies
The literature search was conducted in June 2021 using ERIC and PsycInfo. No limit was set on the publication date so as to be comprehensive. The subject term ‘effect’ (i.e. ‘effect OR impact OR influence OR result OR outcome OR consequence OR contribution’) was paired with the following subject terms one by one: ‘self-assessment’, ‘self-evaluation’, ‘self-monitoring’, ‘self-reflection’, ‘self-rating’, ‘self-grading’, ‘self-review’ and ‘self-feedback’. Following this, the second round of search was conducted using the same databases and subject terms together. This yielded a total of 1,038 records. In addition, six studies were further identified via expert recommendations. After removing duplicates, 675 studies were left to be assessed for eligibility based on predefined selection criteria.
Selection of studies
A study had to meet the following criteria to be included. Firstly, it investigated the effect of self-assessment on academic performance. Secondly, only non-self-reported measures of academic performance could be included as quality control. Thirdly, a study needed to provide sufficient information for the calculation of effect size. The first author was contacted if insufficient data was available in the paper. Fourthly, various forms of self-assessment were accepted (e.g. quantitative, qualitative, using technology), but the content of the assessment had to be restricted to achievement (e.g. not effort). Fifthly, two types of research design were included (i.e. experimental/quasi-experimental design with control groups and repeated measures design without control groups) in order to synthesise more comprehensive empirical evidence. Sixthly, only studies conducted in the higher education context were included. Lastly, a study needed to be written in English, published in a peer-reviewed journal or as a dissertation or thesis.
To ensure screening quality, 50 studies were randomly selected from the identified records. Two coders independently screened them by title and abstract for relevance. Inter-rater reliability (kappa) between the two coders was .81, above the minimum recommendation (Fleiss Citation1971). Any disagreement was discussed, and a consensus was reached before moving on to further screening the rest of the studies as well as filtering them based on the full text of the articles. After two rounds of screening, 26 articles with 98 effect sizes meeting the selection criteria were included in the subsequent meta-analysis. shows a summary of the search and selection process.
Data extraction
Three types of information were extracted from each study. The first was the methodological characteristics of the study, including sampling method (random, systematic or convenient), study design (experimental/quasi-experimental or pre-post design), sample assignment (random assignment or not), confounder report (without or with), confounder control (without or with), data source (assessed or self-reported), instrument source (standardised test, published in journal papers or self-developed), attrition rate and gender (percentage of male). To represent the overall quality of each study, a surrogate index (research quality) was synthesised based on the above-mentioned characteristics except for gender, categorised into three levels (strong, moderator, and weak). Five methodological dimensions were evaluated , including study design, participant selection bias, confounders, data collection and withdrawals (Thomas et al. Citation2004). For the first dimension, random sampling was considered as strong, systematic sampling as moderate, and convenient as weak. For the second dimension, experimental/quasi-experimental design with a control group plus random assignment was considered as strong, pre-post design (with or without random assignment) as moderate, and no control group plus no random assignment as weak. For the third dimension, when potential confounders were reported and controlled, it was considered as strong; when potential confounders were only reported but not controlled, it was considered as moderate; when neither were reported nor controlled, it was considered as weak. For the fourth dimension, a strong data source would be from a standardised test assessed by teachers or researchers; a moderate one from a published instrument in journal papers and self-reported from students; a weak source from a self-reported instrument. For the fifth dimension, an attrition rate below .20 was considered as strong, between .20 and .40 as moderate, and above .40 as weak. The study quality was a synthesised index based on the five dimensions. A study with none or one weak dimension was considered as strong overall in study quality, with two weak dimensions as moderate, and with three or more as weak.
The second type of information extracted was the characteristic of assessment design, including online technology (without or with), student training on self-assessment (without or with), and assessment mode (qualitative, quantitative, or both).
The third type was outcome variables and estimates of effect size (i.e. sample size, mean, standard deviation and correlation).
Coding explicit and implicit self-assessment
According to Yan and Brown (Citation2017) self-assessment process model, we unfolded the self-assessment process into three steps plus a calibration of self-assessment judgement. For each step, we coded the process as explicit or implicit based on the descriptions of self-assessment actions in the included studies. The distinction between explicit and implicit self-assessment is whether the process involves observable behaviours or not. For example, in the first step of determining assessment criteria, self-assessment was regarded as explicit if the assessment criteria were provided or constructed by teachers or students. If students did self-assessment without explicit criteria (although students might still have their own criteria in mind) or the included study did not provide any information regarding the criteria, the self-assessment was treated as implicit.
In the second step, external feedback, self-assessment was explicit if feedback information on students’ performance (not feedback on self-assessment judgement) was provided by external parties (e.g. teachers, peers, etc.). Otherwise, self-assessment was implicit. It should be noted that, in the original model of Yan and Brown (Citation2017), the second step was self-directed feedback seeking which highlights that students should take an active role in initiating and implementing feedback seeking behaviours, and that feedback may come from both external and internal sources. For our analysis here, however, we used external feedback instead for two reasons. Firstly, whether students used internal feedback or not was often ignored or not reported by researchers. In contrast, the provision of external feedback in self-assessment interventions is traceable and was usually reported in studies. Secondly, external feedback could be helpful to self-assessment whether it is actively sought or passively received by the student. Although self-directed feedback seeking may be preferred from the perspective of self-regulated and life-long learning, its absence in reports means that this aspect of the Yan and Brown model cannot be addressed in this study.
In the step of self-reflection, self-assessment was regarded as explicit if the self-reflection process and/or results were made explicit through writing (e.g. writing a reflective worksheet or answering reflective questions) or other methods (e.g. discussing with others). It was regarded as implicit if the self-reflection was a totally inwards process.
In the calibration of self-assessment judgement, if students were explicitly required or provided opportunities to calibrate their self-assessment judgement (e.g. revising the self-assessment grades/comments), it was explicit. Otherwise, it was implicit.
Effect size calculation
Cohen’s d (also known as the standardised mean difference) (Cohen Citation1988) was used to calculate the effect size for the studies comparing self-assessment interventions with control groups. For repeated measures design studies without control groups, the effect size was calculated using the formula of Becker (Citation1988). As this calculation requires the pre-post correlation of the outcome variables, which is rarely ever reported in published studies, we imputed a correlation of .50, following a regular practice applied in many meta-analyses. As Cohen’s d effect size has been found to have an upward bias when the study sample size is small, all Cohen’s d were converted into Hedges’ g (Hedges Citation1981). The data analysis procedure can be found in the Supplementary Materials.
Results
The overall effect of self-assessment interventions
A total number of 98 effect sizes from 26 studies either reported a comparison between a group with self-assessment interventions and a control group (n = 20, k = 88) or a pre-post comparison (n = 6, k = 10). The full references of included studies can be found in the Supplementary Materials. shows that there were more positive effect sizes than negative ones and there was no outlier (g > 2.343 or g < −1.698). The overall effect of self-assessment interventions was .455, that was statistically different from zero (SE = .106, 95%CI, .243-.666; t = 4.273; p <.0001, k = 98 in 26 studies). The estimated variance components were τ2Level 3 = .193 and τ2Level 2 = .107. It means that I2level3 = 54.33% of the total variation can be attributed to the between-cluster heterogeneity, and I2level2 = 30.14% of the total variation go to the within-cluster heterogeneity. The three-level model provided a significantly better fit compared to a two-level model with level three heterogeneity constrained to zero (between studies: LRT: , p <.0001; within studies: LRT:
, p <.0001). Funnel plots and the three-level Egger regression test indicate the absence of publication bias (see the Supplementary Materials for details).
Moderator analysis for the overall effect of self-assessment interventions
Four aspects of the moderating effect of explicit self-assessment were examined: determining assessment criteria, external feedback, self-reflection and calibration of self-assessment judgement. The results () showed that only one aspect, i.e. external feedback, had a significant moderating effect. Self-assessment interventions involving explicit feedback on students’ performance had significantly larger effect sizes (g = .664, p <.05) than those without explicit feedback (g = .213, p > .05). The other three aspects of the moderating effects of explicitness were observable, but not statistically significant. The mean effect size of interventions with explicit criteria (e.g. rubrics) (g = .503, p >.05) was larger than those without explicit criteria (g = −.154, p > .05). It should be noted, however, that there were only three effect sizes from two studies with implicit assessment criteria. Interventions involving explicit calibration of self-assessment judgement demonstrated larger mean effect size (g = .594, p >.05) than those without explicit calibration (g =.304, p <.05). However, interventions with explicit self-reflection had slightly smaller mean effect size (g = .432, p > .05) than those without explicit self-reflection (g = .474, p < .01).
Table 1. Differences in effect sizes for moderators (all self-assessment interventions).
There were no other significant moderators identified, although some differences were observable. For example, the percentage of male participants in the sample was examined as a moderator. The regression coefficient was negative (-.819, p > .05), implying that there was a lesser effect size for males than females. When students did self-assessment without online technology, the mean effect size (g = .510, p < .001) appeared to be larger than that using online technology (g = .210, p > .05). There was also a slight difference between the mean effect size of studies with self-assessment training for students (g = .477, p > .05) and those without training (g = .446, p < .01). Regarding the assessment mode, the mean effect size of studies with quantitative evaluations (g = .559, p > .05) was larger than studies with qualitative evaluations (g = .374, p > .05) and studies with both of the two evaluations (g = .383, p > .05). The mean effect size of the studies with repeated measures design (g = .571, p < .05) was larger than that of the studies with control group design (g = .425, p > .05). In terms of study quality, the mean effect size of studies with strong quality (g = .893, p > .05) was larger than the mean effect sizes of studies with moderate (g = .435, p < .05) or weak quality (g = .438, p > .05). However, in all these differences, the effect sizes were not statistically significant. We did not employ multiple regression because only one moderator, i.e. explicit feedback, was significant.
Moderator analysis for the effect of self-assessment interventions with explicit feedback
As presented above, self-assessment interventions involving explicit feedback on students’ performance had a significantly larger effect size than those without explicit feedback. We, therefore, grouped all studies using self-assessment interventions with explicit feedback together (n = 15, k = 40) and did a further moderator analysis. That is, we examined what factors influenced the effect of self-assessment interventions with explicit feedback on academic performance. The difference between this analysis and that in the above section is that here we focused on self-assessment interventions with explicit feedback. By doing so we can have a better understanding of the conditions in which self-assessment interventions with explicit feedback can bring more learning gains.
Although there were some observable differences of effect sizes, none of moderators were statistically significant (see for details). The negative regression coefficients of percentage of male participants (-.219, p > .05) implies that the effect of explicit self-assessment was smaller for male than female students. Using online technology was not analysed as a moderator as there was only one effect size without online technology. The mean effect size of studies with student training (g = .610, p > .05) was slightly smaller than that of studies without training (g = .791, p < .01). For assessment mode, the mean effect size of studies with qualitative evaluations (g = .884, p > .05) was larger than studies with quantitative evaluations (g = .578, p > .05) and studies with both methods (g = .552, p > .05). There was little difference between the effect sizes for studies with control group design (g = .687, p > .05) and those with repeated measures design (g = .702, p < .05). With regard to the study quality, the mean effect size of strong-quality studies was highest (g = .937, p > .05), followed by moderate-quality studies (g = .566, p < .05), and weak-quality studies (g = .188, p > .05).
Table 2. Differences in effect sizes for moderators (only self-assessment interventions with explicit feedback).
Discussion
Self-assessment is believed to benefit students’ academic performance if it is used in a formative fashion (Andrade Citation2019; Panadero, Lipnevich, and Broadbent Citation2019). This meta-analytical review aimed to test this effect by synthesising empirical evidence in higher education. A total number of 98 effect sizes from 26 studies reporting either an experimental-control comparison or a pre-post comparison were included in the synthesis.
Overall effect of self-assessment
The mean effect size of self-assessment interventions in this synthesis was .455. For educational interventions, an effect size of .40 or above is regarded as meaningful and an effect size of .60 or above is considered large (Hattie Citation2008). Thus, self-assessment deserves more pedagogical attention because it is not only theoretically compatible with the fundamental goals of higher education, such as self-regulated and life-long learning, but also can effectively enhance students’ academic performance. The mean effect size revealed in this synthesis is similar to the findings in meta-analyses in the school context. For example, Brown and Harris (Citation2013) review of 23 studies revealed a positive effect (median effect between .40 and .45) across year levels and subject areas. Similarly, the review by Youde (Citation2019) including 19 studies published between 1991 and 2017 reported a mean effect size of .46. The mean effect size in this synthesis is larger than that reported in Sanchez et al. (Citation2017) review (.34). This is probably because we included all types of self-assessment, while Sanchez et al. (Citation2017) only covered self-grading. This finding is congruent with previous arguments that self-grading, or grade guessing (Boud and Falchikov Citation1989), is less effective because it may not involve eliciting and using criteria and making meaningful evaluative judgments (Yan and Boud Citation2022).
Like past meta-analyses in the school context, despite the positive mean effect size, the magnitude of learning gains varied across studies, with 26 (26.5%) studies in this meta-analysis reporting negative effects. It suggests that the use of self-assessment per se does not guarantee better academic performance: it depends on how it is used. The implementation of self-assessment is complicated and may be influenced by both personal and contextual factors, as are other types of formative assessment (Yan et al. Citation2021). To enact and maximise the positive impact of self-assessment, teachers need to consider both these factors when designing and implementing self-assessment in courses.
Difference between the effect of explicit and implicit self-assessment
This meta-analysis is the first to compare the effect of explicit and implicit self-assessment interventions. Theoretically, making self-assessment explicit is likely to facilitate intentional instruction of self-assessment strategies which, in turn, may increase the effectiveness of self-assessment. Explicit self-assessment with observable behaviours (e.g. discussion of assessment criteria, external feedback and written reflection) provides teachers with opportunities to monitor, intervene and scaffold students’ self-assessment processes, especially for students with limited self-assessment experience and strategies. This may be especially important for weaker students who have demonstrated less capacity to judge their own performance (Falchikov and Boud Citation1989).
The results showed that self-assessment interventions with explicit feedback on students’ performance had significantly larger effect sizes than those without explicit feedback. This finding highlights the crucial role of other persons (e.g. teachers and peers) in the self-assessment process (Boud Citation1999; Brown and Harris Citation2013). The feedback provided by others serves as important reference information against which students compare their own performance (Nicol Citation2021). External feedback can reveal biases, which are quite common in self-assessment, and help students correct them. In the long term, the interaction with external feedback during self-assessment can enhance students’ ability to generate self-feedback (Panadero, Lipnevich, and Broadbent Citation2019). In contrast, self-assessment without explicit feedback is problematic not only because it is difficult to notice its influence, but also because it is subject to biases that never get revealed and openly examined.
This aspect, external feedback, is somewhat different from the Yan and Brown (Citation2017) original model where the equivalent step is entitled self-directed feedback seeking. In line with the self-regulation perspective, Yan and Brown (Citation2017) highlighted the active and reflective role of students in their self-assessment model and focused on students’ feedback seeking behaviour. In practice, however, it is not easy to differentiate whether external feedback is actively sought or passively received by students. Although it is desirable for students to have the capacity and willingness to actively seek external feedback, this meta-analysis shows that the existence of external feedback, no matter whether it is student-initiated or not, can increase the effectiveness of self-assessment. Thus, the provision of external feedback should be fostered in self-assessment processes.
The other three aspects of the explicit/implicit dimension (i.e. determining assessment criteria, self-reflection and calibration of self-assessment judgement) did not influence the effectiveness of self-assessment interventions. This non-significant result might be attributable to the insufficient number of studies in some comparisons. For example, there are only three effect sizes from two studies without explicit assessment criteria. The low number, on the one hand, decreases the statistical power and, on the other hand, indicates that making self-assessment criteria explicit has become a norm in practice. Nevertheless, caution should be taken in interpreting these results. Our coding was based on the description of self-assessment interventions in the included studies. Thus, the trustworthiness of the description is crucial. It is not surprising that some studies did not provide sufficient details regarding the interventions, which might hamper the precision of the coding. It is also possible that students might have done explicit self-assessment (e.g. discuss with peers on assessment criteria, getting feedback from others, etc.), but the researchers did not know or did not report it. To facilitate better communication and synthesis, we urge researchers to provide a precise account of the self-assessment process in their interventions in the future.
Moderators of self-assessment
Apart from external feedback, there were no other significant moderators identified in this meta-analysis. To some extent, this result aligns with past meta-analyses on similar topics. For instance, Sanchez et al. (Citation2017) conducted analyses exploring five moderators for the effect of self-grading on test performance, including educational level, subject area, training to students, frequency of self-grading and the use of rubrics. All these moderators showed no significant effect. Youde’s (Citation2019) review identified only one significant moderator: self-assessment had a stronger effect for middle school learners than high school learners. This finding, however, should be interpreted with caution. Some studies directly compared the effects of self-assessment interventions with different designs and found significant differences. For example, Panadero and Romero (Citation2014) reported that the rubric condition led to significantly better academic performance than the non-rubric condition. Unfortunately, such kinds of studies with multiple self-assessment conditions are extremely rare. In addition, it is not unusual that multiple moderators are nested within the same intervention, such as in Birjandi and Tamjid (Citation2012) study: students in one condition conducted qualitative self-assessment and received no training, while those in the other condition did quantitative self-assessment but received training. Such collinearity of moderators (Murano, Sawyer, and Lipnevich Citation2020) may make the real impact of individual moderators murky. Future experimental studies should consider including multiple conditions that represent different designs of self-assessment to identify the features of effective self-assessment interventions.
Conclusion
The present meta-analysis provides an updated synthesis on the effect of self-assessment on academic performance. Overall, self-assessment interventions had positive and meaningful effects on students’ academic performance. This supports theoretical claims about the benefits of self-assessment in improving student learning. Self-assessment interventions with explicit feedback on students’ performance showed a significantly larger effect than those without explicit feedback, indicating that the availability of external feedback provides important scaffolding for successful self-assessment. No other significant moderator was identified in this meta-analysis, which calls for more studies to gauge in more depth the features of effective self-assessment interventions. The findings of this synthesis highlight the importance of self-assessment in teaching in higher education and inform researchers and practitioners about the design and implementation of self-assessment interventions.
Supplemental Material
Download MS Word (38.4 KB)Acknowledgments
We would like to acknowledge Ernesto Panadero’s contribution to the creation of the database (e.g., establishing the search in terms of the engines and keywords to use, selection of articles, and inter-judges agreement).
Disclosure statement
No potential conflict of interest was reported by the authors.
Funding
The work described in this paper was supported by a General Research Fund from the Research Grants Council of the Hong Kong Special Administrative Region, China (Project No. EDUHK 18600019).
Notes on contributors
Zi Yan is an Associate Professor in the Department of Curriculum and Instruction at The Education University of Hong Kong. His research interests focus on two related areas, i.e., educational assessment in the school and higher education contexts with an emphasis on student self-assessment; and Rasch measurement, in particular its application in educational and psychological research. A recent book co-edited with Lan Yang is entitled, Assessment as learning: Maximising opportunities for student learning and achievement.
Xiang Wang is a Research Assistant in the Department of Curriculum and Instruction at The Education University of Hong Kong. His publications and research interests focus on two areas, i.e., meta-analysis in educational and psychological research; and sports psychology with an emphasis on promoting athlete mental health.
David Boud is Alfred Deakin Professor and Foundation Director of the Centre for Research in Assessment and Digital Learning at Deakin University, Melbourne. He is also Emeritus Professor at the University of Technology Sydney and Professor in the Work and Learning Research Centre at Middlesex University, London. He has published extensively on teaching, learning and assessment in higher and professional education. His current work focuses on assessment-for-learning in higher education, academic formation and workplace learning.
Hongling Lao is a Post-doctoral Fellow in the Department of Curriculum and Instruction at The Education University of Hong Kong. She majored in educational measurement at graduate school, specialized in diagnostic classification models. Her current research interest is the integration of educational measurement and assessment to enhance learning and teaching in K-12 context, with a focus on teacher and student assessment literacy.
References
- Acuna, E., and C. Rodriguez. 2004. “A Meta-Analysis Study of Outlier Detection Methods in Classification.” Technical Paper, Department of Mathematics, University of Puerto Rico at Mayaguez. https://academic.uprm.edu/∼eacuna/paperout.pdf
- Andrade, H. L. 2010. “Students as the Definitive Source of Formative Assessment: Academic Self-Assessment and the Self-Regulation of Learning.” In Handbook of Formative Assessment, edited by H. L. Andrade, and G. J. Cizek, 90–105. New York: Routledge.
- Andrade, H. L. 2019. “A Critical Review of Research on Student Self-Assessment.” Frontiers in Education 4: 4. doi:10.3389/feduc.2019.00087.
- Andrade, H. L., and B. Boulay. 2003. “Gender and the Role of Rubric-Referenced Self-Assessment in Learning to Write.” The Journal of Educational Research 97 (1): 21–34. doi:10.1080/00220670309596625.
- Andrade, H. L., and Y. Du. 2007. “Student Responses to Criteria-Referenced Self-Assessment.” Assessment & Evaluation in Higher Education 32 (2): 159–181. doi:10.1080/02602930600801928.
- Assink, M., and C. J. Wibbelink. 2016. “Fitting Three-Level Metanalytic Models in R: A Step-by-Step Tutorial.” The Quantitative Methods for Psychology 12 (3): 154–174. doi:10.20982/tqmp.12.3.p154.
- Baas, D., J. Castelijns, M. Vermeulen, R. Martens, and M. Segers. 2015. “The Relation between Assessment for Learning and Elementary Students’ Cognitive and Metacognitive Strategy Use.” The British Journal of Educational Psychology 85 (1): 33–46. doi:10.1111/bjep.12058.
- Becker, B. J. 1988. “Synthesizing Standardized Mean-Change Measures.” British Journal of Mathematical and Statistical Psychology 41 (2): 257–278. doi:10.1111/j.2044-8317.1988.tb00901.x.
- Birjandi, P., and N. H. Tamjid. 2012. “The Role of Self-, Peer and Teacher Assessment in Promoting Iranian EFL Learners’ Writing Performance.” Assessment & Evaluation in Higher Education 37 (5): 513–533. doi:10.1080/02602938.2010.549204.
- Blanch-Hartigan, D. 2011. “Medical Students’ Self-Assessment of Performance: Results from Three Meta-Analyses .” Patient Education and Counseling 84 (1): 3–9. doi:10.1016/j.pec.2010.06.037.
- Boud, D. 1989. “The Role of Self Assessment in Student Grading.” Assessment & Evaluation in Higher Education 14 (1): 20–30. doi:10.1080/0260293890140103.
- Boud, D. 1999. “Avoiding the Traps: Seeking Good Practice in the Use of Self Assessment and Reflection in Professional Courses.” Social Work Education 18 (2): 121–132. doi:10.1080/02615479911220131.
- Boud, D., and N. Falchikov. 1989. “Quantitative Studies of Student Self-Assessment in Higher Education: A Critical Analysis of Findings.” Higher Education 18 (5): 529–549. doi:10.1007/BF00138746.
- Boud, D., and N. Falchikov. 2006. “Aligning Assessment with Long-Term Learning.” Assessment & Evaluation in Higher Education 31 (4): 399–413. doi:10.1080/02602930600679050.
- Boud, D., R. Lawson, and D. G. Thompson. 2013. “Does Student Engagement in Self-Assessment Calibrate Their Judgement over Time?” Assessment & Evaluation in Higher Education 38 (8): 941–956. doi:10.1080/02602938.2013.769198.
- Brown, G. T. L., H. Andrade, and F. Chen. 2015. “Accuracy in Student Self-Assessment: Directions and Cautions for Research.” Assessment in Education: Principles, Policy & Practice 22 (4): 444–457. doi:10.1080/0969594X.2014.996523.
- Brown, G. T. L., and L. R. Harris. 2013. “Student Self-Assessment.” In the SAGE Handbook of Research on Classroom Assessment, edited by J. H. McMillan, 367–393. Thousand Oaks: Sage.
- Brown, G. T. L., and L. R. Harris. 2014. “The Future of Self-Assessment in Classroom Practice: Reframing Self-Assessment as a Core Competency.” Frontline Learning Research 3: 22–30. doi:10.14786/flr.v2i1.24.
- Butler, D. L., and P. H. Winne. 1995. “Feedback and Self-Regulated Learning: A Theoretical Synthesis.” Review of Educational Research 65 (3): 245–281. doi:10.3102/00346543065003245.
- Cheung, M. W. L. 2014. “Modeling Dependent Effect Sizes with Three-Level Meta-Analyses: A Structural Equation Modeling Approach.” Psychological Methods 19 (2): 211–229. doi:10.1037/a0032968.
- Coburn, K. M., and J. K. Vevea. 2019. “Weightr: Estimating Weight-Function Models for Publication Bias.” R package version 2.0.2.
- Cohen, J. C. 1988. Statistical Power Analysis for the Behavioral Sciences. 2nd ed. NJ: Lawrence Erlbaum Erlbaum Associates.
- Dochy, F., M. Segers, and D. Sluijsmans. 1999. “The Use of Self-, Peer and Co-Assessment in Higher Education: A Review.” Studies in Higher Education 24 (3): 331–350. doi:10.1080/03075079912331379935.
- Egger, M., G. Davey-Smith, M. Schneider, and C. Minder. 1997. “Bias in Meta-Analysis Detected by a Simple, Graphical Test.” BMJ (Clinical Research ed.) 315 (7109): 629–634. doi:10.1136/bmj.315.7109.629.
- Falchikov, N., and D. Boud. 1989. “Student Self-Assessment in Higher Education: A Meta-Analysis.” Review of Educational Research 59 (4): 395–430. doi:10.3102/00346543059004395.
- Fernández-Castilla, B., L. Declercq, L. Jamshidi, S. N. Beretvas, P. Onghena, and W. van den Noortgate. 2021. “Detecting Selection Bias in Meta-Analyses with Multiple Outcomes: A Simulation Study.” The Journal of Experimental Education 89 (1): 125–144. doi:10.1080/00220973.2019.1582470.
- Fleiss, J. L. 1971. “Measuring Nominal Scale Agreement among Many Raters.” Psychological Bulletin 76 (5): 378–382. doi:10.1037/h0031619.
- Harrer, M., P. Cuijpers, T. A. Furukawa, and D. D. Ebert. 2021. Doing Meta-Analysis with R: A Hands-on Guide. Florida: CRC Press.
- Harris, L. R., and G. T. L. Brown. 2018. Using Self-Assessment to Improve Student Learning. New York: Routledge.
- Hattie, J. 2008. Visible Learning: A Synthesis of over 800 Meta-Analyses Relating to Achievement. New York: Routledge.
- Hedges, L. V. 1981. “Distribution Theory for Glass’s Estimator of Effect Size and Related Estimators.” Journal of Educational Statistics 6 (2): 107–128. doi:10.3102/10769986006002107.
- Higgins, J. P., J. Thomas, J. Chandler, M. Cumpston, T. Li, M. J. Page, and V. A. Welch. 2019. Cochrane Handbook for Systematic Reviews of Interventions. New Jersey: John Wiley & Sons.
- Li, M., and X. Zhang. 2021. “A Meta-Analysis of Self-Assessment and Language Performance in Language Testing and Assessment.” Language Testing 38 (2): 189–218. doi:10.1177/0265532220932481.
- Light, R. J., and D. B. Pillemer. 1984. Summing up: The Science of Reviewing Research. Cambridge, MA: Harvard University Press.
- Lipnevich, A. A., D. A. G. Berg, and J. K. Smith. 2016. “Toward a Model of Student Response to Feedback.” In Handbook of Human and Social Conditions in Assessment, edited by G. T. L. Brown and L. R. Harris, 169–185. New York: Routledge.
- Murano, D., J. E. Sawyer, and A. A. Lipnevich. 2020. “A Meta-Analytic Review of Preschool Social and Emotional Learning Interventions.” Review of Educational Research 90 (2): 227–263. doi:10.3102/0034654320914743.
- Nicol, D. 2021. “The Power of Internal Feedback: Exploiting Natural Comparison Processes.” Assessment & Evaluation in Higher Education 46 (5): 756–778. doi:10.1080/02602938.2020.1823314.
- Nicol, D., and S. McCallum. 2021. “Making Internal Feedback Explicit: Exploiting the Multiple Comparisons That Occur during Peer Review.” Assessment & Evaluation in Higher Education : 1–19. doi:10.1080/02602938.2021.1924620.
- Panadero, E., G. T. L. Brown, and J.-W. Strijbos. 2016. “The Future of Student Self-Assessment: A Review of Known Unknowns and Potential Directions.” Educational Psychology Review 28 (4): 803–830. doi:10.1007/s10648-015-9350-2.
- Panadero, E., A. Jonsson, and J. Botella. 2017. “Effects of Self-Assessment on Self-Regulated Learning and Self-Efficacy: Four Meta-Analyses.” Educational Research Review 22: 74–98. doi:10.1016/j.edurev.2017.08.004.
- Panadero, E., A. A. Lipnevich, and J. Broadbent. 2019. “Turning Self-Assessment into Self-Feedback.” In The Impact of Feedback in Higher Education: Improving Assessment Outcomes for Learners, edited by D. Boud, M. D. Henderson, R. Ajjawi, and E. Molloy. London: Springer Nature.
- Panadero, E., and M. Romero. 2014. “To Rubric or Not to Rubric? The Effects of Self-Assessment on Self-Regulation, Performance and Self-Efficacy.” Assessment in Education: Principles.” Policy & Practice 21 (2): 133–148. doi:10.1080/0969594X.2013.877872.
- Sanchez, C. E., K. M. Atkinson, A. C. Koenka, H. Moshontz, and H. Cooper. 2017. “Self-Grading and Peer-Grading for Formative and Summative Assessments in 3rd through 12th Grade Classrooms: A Meta-Analysis.” Journal of Educational Psychology 109 (8): 1049–1066. doi:10.1037/edu0000190.
- Schwarzer, G. 2007. “Meta: An R Package for Meta-Analysis.” R News 7 (3): 40–45.
- Sitzmann, T., K. Ely, K. G. Brown, and K. N. Bauer. 2010. “Self-Assessment of Knowledge: A Cognitive Learning or Affective Measure?” Academy of Management Learning & Education 9 (2): 169–191. doi:10.5465/amle.9.2.zqr169.
- Thomas, B. H., D. Ciliska, M. Dobbins, and S. Micucci. 2004. “A Process for Systematically Reviewing the Literature: Providing the Research Evidence for Public Health Nursing Interventions.” Worldviews on Evidence-Based Nursing 1 (3): 176–184. doi:10.1111/j.1524-475X.2004.04006.x.
- van den Noortgate, W., J. A. López-López, F. Marín-Martínez, and J. Sánchez-Meca. 2013. “Three-Level Meta-Analysis of Dependent Effect Sizes.” Behavior Research Methods 45 (2): 576–594. doi:10.3758/s13428-012-0261-6.
- Vevea, J. L., and C. M. Woods. 2005. “Publication Bias in Research Synthesis: Sensitivity Analysis Using a Priori Weight Functions.” Psychological Methods 10 (4): 428–443. doi:10.1037/1082-989X.10.4.428.
- Viechtbauer, W. 2010. “Conducting Meta-Analyses in R with the Metafor Package.” Journal of Statistical Software 36 (3): 1–48. doi:10.18637/jss.v036.i03.
- Wickham, H., M. Averick, J. Bryan, W. Chang, L. D. A. McGowan, R. François, G. Grolemund, et. al. 2019. “Welcome to the Tidyverse.” Journal of Open Source Software 4 (43): 1686. doi:10.21105/joss.01686.
- Yan, Z. 2016. “The Self-Assessment Practices of Hong Kong Secondary Students: Findings with a New Instrument.” Journal of Applied Measurement 17 (3): 1–19.
- Yan, Z. 2018. “Student Self-Assessment Practices: The Role of Gender, Year Level, and Goal Orientation.” Assessment in Education: Principles.” Policy & Practice 25 (2): 183–199. doi:10.1080/0969594X.2016.1218324.
- Yan, Z. 2020. “Self-Assessment in the Process of Self-Regulated Learning and Its Relationship with Academic Achievement.” Assessment & Evaluation in Higher Education 45 (2): 224–238. doi:10.1080/02602938.2019.1629390.
- Yan, Z., and D. Boud. 2022. “Conceptualising Assessment-as-Learning.” In Assessment as Learning: Maximising Opportunities for Student Learning and Achievement, edited by Z. Yan, and L. Yang, 11–24. New York: Routledge.
- Yan, Z., and G. T. L. Brown. 2017. “A Cyclical Self-Assessment Process: Towards a Model of How Students Engage in Self-Assessment.” Assessment & Evaluation in Higher Education 42 (8): 1247–1262. doi:10.1080/02602938.2016.1260091.
- Yan, Z., Z. Li, E. Panadero, M. Yang, L. Yang, and H. Lao. 2021. “A Systematic Review on Factors Influencing Teachers’ Intentions and Implementations regarding Formative Assessment.” Assessment in Education: Principles.” Policy & Practice 28 (3): 228–260. doi:10.1080/0969594X.2021.1884042.
- Youde, Jeffrey J. 2019. “A Meta-analysis of the Effects of Reflective Self-assessment on Academic Achievement in Primary and Secondary Populations.” PhD diss., University of Seattle Pacific, ProQuest (27542662).