Abstract
The problem of identifying and reproducing the hydrological behaviour of groundwater systems can often be set in terms of ordinary differential equations relating the inputs and outputs of their physical components under simplifying assumptions. Conceptual linear and nonlinear models described as ordinary differential equations are widely used in hydrology and can be found in several studies. Groundwater systems can be described conceptually as an interlinked reservoir model structured as a series of nonlinear tanks, so that the groundwater table can be schematized as the water level in one of the interconnected tanks. In this work, we propose a methodology for inferring the dynamics of a groundwater system response to rainfall, based on recorded time series data. The use of evolutionary techniques to infer differential equations from data in order to obtain their intrinsic phenomenological dynamics has been investigated recently by a few authors and is referred to as evolutionary modelling. A strategy named Evolutionary Polynomial Regression (EPR) has been applied to a real hydrogeological system, the shallow unconfined aquifer of Brindisi, southern Italy, for which 528 recorded monthly data over a 44-year period are available. The EPR returns a set of non-dominated models, as ordinary differential equations, reproducing the system dynamics. The choice of the representative model can be made both on the basis of its performance against a test data set and based on its incorporation of terms that actually entail physical meaning with respect to the conceptualization of the system.
Citation Doglioni, A., Mancarella, D., Simeone, V. & Giustolisi, O. (2010) Inferring groundwater system dynamics from hydrological time-series data. Hydrol. Sci. J. 55(4), 593–608.
Résumé Le problème de l'identification et de la reproduction du comportement hydrologique des systèmes hydrogéologiques peut souvent être posé en termes d'équations différentielles ordinaires relatives aux entrées et aux sorties de leurs composantes physiques, avec des hypothèses simplificatrices. Des modèles conceptuels linéaires et non-linéaires décrits sous forme d'équations différentielles ordinaires sont largement utilisés en hydrologie et peuvent être trouvés dans plusieurs études. Les systémes hydrogéologiques peuvent être décrits sur le plan conceptuel par un modèle de réservoirs interdépendants structuré comme une série de réservoirs non-linéaires, de sorte que le niveau de la nappe peut être schématisé comme étant le niveau d'eau dans léun des réservoirs interconnectés. Dans ce travail, nous proposons une méthodologie permettant d'inférer la dynamique de la réponse d'un systéme hydrogéologique aux précipitations, sur la base de données temporelles enregistrées. L'utilisation de techniques évolutives pour déduire les équations différentielles à partir de données afin d'obtenir leur dynamique phénoménologique intrinséque a été étudiée récemment par quelques auteurs et est appelée modélisation évolutive. Une stratégie nommée régression polynômiale évolutive (EPR) a été appliquée à un système hydrogéologique réel, l'aquifère peu profond libre de Brindisi, au sud de l'Italie, pour lequel 528 données enregistrées mensuellement sur une période de 44 ans sont disponibles. L'EPR retourne un ensemble de modèles non-dominés, sous forme d'équations différentielles ordinaires, reproduisant la dynamique du système. Le choix du modèle représentatif peut être fait sur la base de sa performance vis-à-vis d'un jeu de données test et de son incorporation de termes ayant réellement un sens physique par rapport à la conceptualisation du systéme.
INTRODUCTION
The problem of identifying and reproducing the hydrological behaviour of groundwater systems can often be set in terms of ordinary differential equations (ODEs) relating the inputs and outputs of their physical components under simplifying assumptions (Wanakule & Anaya, Citation1993). State–space representation (Lijung, Citation1999; Hinrichsen & Pritchard, 2004; Nise, Citation2004) of natural systems is a common approach in many hydrological applications. Conceptual linear and nonlinear models described as ODEs are widely used and can be found in several studies (Chow & Kulandaiswamy, Citation1971; Kundzewicz & Napiôrkowski, Citation1986; Knotters & Bierkens, Citation2000; Li & Simonovic, Citation2002). The use of evolutionary techniques to infer differential equations from data in order to extract their intrinsic phenomenological dynamics has recently been investigated by Cao et al. (Citation2000) and is referred to as evolutionary modelling. Some attempts to comply with this objectively difficult task can be found in the literature, in particular using genetic programming (GP) (Koza, Citation1992) or hybrid GP techniques (Babovic & Keijzer, Citation2000a; Iba & Sakamoto, Citation2002; Cao et al., Citation2003). In many cases, studies have been conducted with fairly good results on synthetic time series data generated by well-established mathematical laws, but few authors have attempted to apply the approach to a real case study, by using measurement data. From a practical point of view, conceptual state–space modelling of real groundwater system dynamics can potentially be applied to a series of environmental issues, such as springs and groundwater resources management, wetlands and surface runoff by saturation excess, desertification and drought studies, interaction between surface runoff and aquifer discharge for catchment modelling, hydrological analysis and prediction of landslide triggering (Harper Citation1975; Van Asch et al., Citation1996; Blijenberg, Citation1998; Angeli et al., Citation1998; Dehn et al., Citation2000).
The hydrological response of aquifers to meteorological conditions is often studied for operational purposes by means of time series analysis and modelling, or physically-based numerical models that are nowadays commonly available, advanced and widely tested. The latter appear more desirable since physical insight is properly taken into account. However, modelling hydrogeological systems is a challenging problem, often complicated by a poor knowledge of basic assumptions, such as actual hydraulic conductivity distribution, aquifer geometry, leakage and evapotranspiration rates. A better estimate of model parameters usually requires quite substantial investment and prolonged measurement campaigns. If good knowledge of geometry is possible, model parameters can be calibrated, but if unknown inputs concur to influence the system's response, or the representation of the actual system is too gross, results from physically-based models might not be adequate, even for relatively simple systems, as shown in previous studies (see for example Gasmo et al., Citation2000). Collecting reliable data on general and specific aquifer parameters from different sources and public corporations is not always an easy task. Conversely, piezometric head and rainfall intensity time-series data in long records are more easily available. Therefore, in some cases, data-driven approaches in groundwater hydrology are attractive, because they reconstruct a representation of the system from its “history” alone, and can therefore be complementary or even alternative to physically-based numerical models when the latter have numerous parameters to be guessed, or are calibrated on few data. As an example, landslides triggered by rainfall are rarely predicted by hydrogeomechanical models, even when the system is relatively simple (Gasmo et al., Citation2000). The approach proposed here, based on system identification from recorded time-series data, can also be a confrontation tool for other simplified models, often used in practical applications, which represent aquifers as being homogeneous or characterized by simple interpolation methods.
In this work, we propose a methodology for inferring the dynamics of the main physical phenomena leading to the transformation of the average rainfall of each month into groundwater-level variations. The process is applied to a real hydrogeological system and a strategy named Evolutionary Polynomial Regression (EPR) (Giustolisi & Savic, Citation2006; Doglioni et al., Citation2008) has been adopted. Evolutionary modelling methods have the advantage of requiring only recorded time series to reconstruct the system dynamics, besides having a symbolic structure which can be the object of further judgement and allow for possible rendering of the system's dynamics. The approach has been applied on monthly data recorded over a 44-year period, from a groundwater system – the shallow unconfined aquifer of Brindisi – located in the northern part of the Salento Peninsula (Apulia, southern Italy). The evolutionary search for models has been performed by means of a multi-objective strategy, which compares the structural complexity of models with their goodness of fit to data. In the case study considered here, the input data have been pre-processed to reconstruct the time derivatives that naturally emerge in the differential equation.
Some important advantages derive from this evolutionary strategy based on multi-objective symbolic regression:
-
It fosters the structural parsimony of models, thus excluding those which show structural complexity, which is likely to be overdescriptive of the data noise, rather than of physical information.
-
The models are chosen to preserve a good fit to data.
-
The availability of a set of non-dominated models (Van Veldhuizen & Lamont, Citation2000) in the space of possible structures defined by EPR, allows the analysis of the differences among them, thus bringing into prominence the main differences as well as the most recurring terms. The latter are more likely to be associated with the underlying physical information rather than reproducing only short-term effects (Giustolisi & Simeone, Citation2006; Giustolisi & Savic, Citation2009).
Therefore, the choice of representative model, as well as the analysis of returned non-dominated models and their recurrent terms, offers the opportunity to introduce physical insight into the process, which is always desirable. Evolutionary models are calibrated on segments of the hydrological time series and subsequently tested against the remaining and previously unused data. This process leads to the construction and validation of models strongly aimed at fitting the data, hence learning the phenomenon's dynamics from its realization. This general approach might be advantageous and complementary to other methods, especially when detailed knowledge of the system is not possible. Among the numerous well-fitting structures on the Pareto front, determined by the evolutionary approach, the model can be selected by choosing the equations that better represent the dynamics of the system, according to the modeller's physical expertise. A model with lower fitting standards might be preferred over a better performing one, if its symbolic representation is judged to be more realistic, and thus the physical knowledge of the modeller can be incorporated in the modelling process. The possibility to attempt conceptualization of the system's dynamics and, at the same time, select the model which is physically sound from among a set of well-fitting models can thus be an important capability of the proposed methodology. Other studies can be found in the literature that focus on the physical interpretation and conceptualization of regressive models (Knotters & Bierkens, Citation2000).
HYDROGEOLOGICAL FRAMEWORK AND BACKGROUND INFORMATION
The studied groundwater system is the shallow unconfined aquifer of Brindisi (Ricchetti & Polemio, Citation1996; Spizzico et al., Citation2006; Giustolisi & Simeone, Citation2006, Giustolisi et al., Citation2008), located in the northern part of the Salento Peninsula (Apulia, southern Italy). The aquifer is situated in the wide structural tectonic depression between two large calcareous blocks of the Apulian foreland calcareous platform: Murge and Salento. During the Pliocene this depressed area was subject to an important phase of sea transgression with subsequent regression. Over the uninterrupted thick Calcareous basement, the sedimentation process led to a stratigraphic sequence characterized, from bottom to top, by: calcareous sandstone related to Gravina calcareous Sandstone (Upper Pliocene, Lower Middle Pleistocene); then Subappenine Clays (Lower Middle Pleistocene); Quaternary deposits, constituted by sands, sandstone, conglomerates, terraced sands; and alluvial and colluvial deposits (Upper Pleistocene, Holocene). The aquifer is situated in the relatively permeable Pleistocene terraced sandy deposits. The Subappenine Clay layer forms a nearly impermeable lower boundary of the shallow surficial aquifer, but is irregularly thick and locally discontinuous. Permeability in the superficial aquifer is quite low, ranging from 8 × 10-6 to 1.4 × 10-4 m/s, but higher than the permeability of the Subappenine Clay, that ranges from 2 × 10-6 to 1 × 10-7 m/s. The shallow aquifer of Brindisi () thus represents an open system, limited in extent and supplied only by direct rainfall, discharging into the sea, but leaking downwards into the calcareous basement rocks that form a second and regional aquifer in the system (Cotecchia, Citation1977).
Fig. 1 (a) Location of Brindisi, southern Italy, (b) schematic sketch of the aquifer, and (c) schematic stratigraphy of the area.
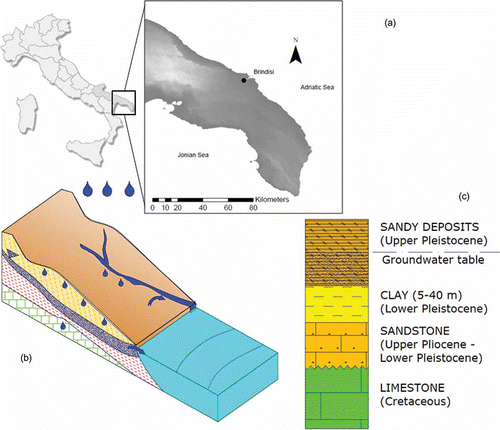
Another component of this system is represented by the well-developed hydrographic network with only a small number of deeper pathways (Cillarese channel, Fiume Grande, Siedi channel). Time series of the discharge of superficial channels in the area or rough estimates of their monthly averages are not available. However, in reconstructing the piezometry from fragmentary data collected in local wells, Ricchetti & Polemio (Citation1996) state that the drainage effect of the superficial streams is significant even if it cannot be estimated accurately.
Data available for the present study cover a period of 44 years (528 monthly data) and consist of time series of phreatic levels, H, and rainfall, P, from a well and a raingauge located close to the city of Brindisi ( and ).
Table 1 Pluviometric and piezometric data
Both the measurement stations belong to the Apulian Regional Hydrographic Service. The data used to construct the models, the training data set, consist of 300 monthly measurements, while the remaining 228 months of data were used only for model testing, being completely unseen during the model construction stage. Time series of derivatives of P and H were estimated as central differences, as suggested in Mathews & Fink (Citation2004) up to the fourth order in the pre-processing, and were provided as input to the modelling process. Using these measurement data represents a challenging task for EPR, since their quality is partially compromised by background noise and unknown extra inputs. In natural groundwater systems, the water table response to precipitation results from multiple effects including: rainfall intensity distribution, pumping, evapotranspiration, atmosphere pressure, sea and earth tide, and other irregular noises (Van der Kamp & Gale, Citation1983; Wittenberg, Citation2003). No reliable data are available on pumping rates at local wells in the area throughout the entire period, or on evapotranspiration rates that could be appraised only from temperature data based on empirical methods. This disturbs EPR in the process of seeking those terms which actually give a physical insight, rather than describing short-term effects related to the particular realization of the phenomenon under investigation. Moreover, monthly data are probably not entirely adequate to capture infiltration effects related to existing differences in precipitation intensity. Actually, different distributions of the same amount of rainfall can produce different effects in terms of recharge. However, as a consequence of their relatively low permeability, the aquifer's soils act as a low-pass filter and there is an observable delay in the data between peak rainfall and discharge that is usually higher than one month (Ricchetti & Polemio, Citation1996; Mancarella & Simeone, Citation2008).
SEEKING A CONCEPTUAL MODEL FOR THE SHALLOW BRINDISI AQUIFER
The conceptual model shown in is based on hydrogeological considerations and physical insight of the system.
Fig. 3 A multiple-tank conceptual nonlinear model for the aquifer of Brindisi. The variables p and h represent, respectively, inflow (rainfall) and water level in reservoirs. The groundwater table oscillations are represented by water-level variations in Reservoir 2. Tanks are progressively numbered and variable subscripts refer to flow origin and delivery. Water losses from the system are embodied by the surface runoff, q 10, and the leakage to a deeper regional aquifer, q 2D .
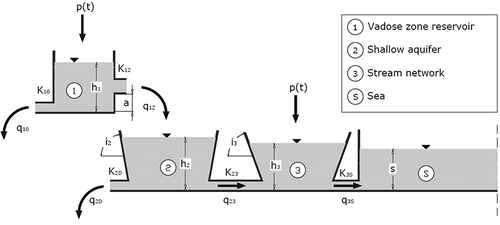
If the groundwater system is conceptually described as a multiple reservoir model structured as series of interconnected tanks, groundwater level, H, can be seen as the water level in one of them. Groundwater-level dynamics result from the exchange of water between different components of the overall system. In hydrology, it is common to translate the perception of a groundwater system into a multiple-reservoir model (Arikan, Citation1988; Barrett & Charbeneau, Citation1997; Knotters & Bierkens, Citation2000; Zhang & Savenije, Citation2005) representing a lumped state–space model. The reader is referred to for the following discussion and notation.
The water table in the shallow aquifer can be represented by H = h 2(t), with t = 1, …, N, where N is the number of data available. Hydraulic head differences between the interconnected tanks control flow exchange among the components of the system. In such a system, Tank 1, discharging into the groundwater Reservoir 2, simulates rainfall interception and storage in the vadose zone. The tank represents the damping effect of the vadose zone, which allows for downward flux only when the field capacity of the soil is already occupied by filtrated water. The unsaturated zone is part of the most superficial aquifer soils, poorly permeable. A loss of rainfall actually intervenes before water can reach the shallow aquifer due to surface runoff and evapotranspiration. Infiltration q 12 flows to the shallow aquifer when the minimum threshold level a, representing the soil retention capacity, is exceeded. Leakage towards a deeper aquifer is regarded as an orifice outflow controlled by the water head (q 2D ).
A channel network interacting with the groundwater system can be represented as one or more downstream serial tanks forced by rainfall and acting on groundwater reservoir through a load effect (Tank 2).
If the groundwater reservoir is considered to follow a linear relationship between tank level, h, and outflow, q out, the following expression can be assumed:
where k out is a coefficient of proportionality accounting for the orifice width, and h is the difference between water level in the reservoir and the orifice height. In the modelling of groundwater flow phenomena, especially at higher scales, this assumption appears to be justifiable, since the analogy with Darcy's law, which rules the flow hydraulics of saturated soils, is evident. However, the discussion on the linearity of groundwater reservoirs is still open (Fenicia et al., Citation2005).
An exchange flow, q ij between two interconnected reservoirs, i and j, is simulated by the following equation:
In this type of system, a continuity equation can be written for each tank, as follows:
Following all these considerations, and in agreement with the preceding equations, if we assume, for simplicity, that the tanks are prismatic (VI = Aihi , with Ai constant in time), then the ordinary differential equations ruling the dynamics of the system represented in the figure are:
As the system is linear in the variables (h 1 , h 2 , h 3), it is possible, by using Laplace transformations, to derive an analytical expression relating the water level hi (t) (i = 1, 2, 3) in any of the interconnected tanks to the forcing terms p(t) and s(t) in the form:
It is noteworthy that derivatives of p(t) appear in the differential equation relating p(t) and h 2(t). A look at coefficient M 21 reveals that this term is due to indirect forcing of p(t) in tanks upstream and downstream of the shallow aquifer. Similar observations might be drawn for the other variables.
Assuming that some tanks are non-prismatic, e.g. reverse truncated cones having rk
for minor radius and ik
for slope ratio (k = 1, 2, 3), the set of differential equations (
Equation1(1)) and (
Equation2
(2)) ruling the dynamics of the system, under the hypothesis that Darcy's law is valid, can be written again considering water balance (3) in each reservoir. Thus, nonlinearity is introduced into the model, because differentiating the storage volume over time leads to the products of water levels by their derivatives. Further nonlinearity in the system may be introduced by assuming a nonlinear relationship between the tank water level and its outflow, for those cases in which Darcy's law cannot hold (Şen, Citation2000), as well as assuming variable-dependent parameters (Kundzewicz & Napiórkowski, Citation1986). It is usually not possible for nonlinear systems to analytically draw an explicit relationship between p(t) and h
2(t) without linearizing the system, starting from:
AN APPROACH FOR INFERRING GROUNDWATER SYSTEM DYNAMICS
This paragraph introduces a brief overview of Evolutionary Polynomial Regression (EPR) (Giustolisi & Savic, Citation2006, Citation2009), the numerical technique adopted in the proposed approach for inferring groundwater system dynamics from time-series data. Following a general explanation, the peculiar application of the technique to the current problem will be clarified.
Evolutionary Polynomial Regression is a data-driven hybrid technique based on evolutionary computing; its paradigm can be classified as Genetic Programming (GP) (Koza, Citation1992). GP proved effective for several modelling applications (Babovic & Kejzer, Citation2000b), but suffers from certain limitations, such as code bloating, difficulty in dealing with constant parameter, code nesting, etc. (Soule & Heckendorn, Citation2002). Davidson et al. (Citation2003) offer an interesting demonstration of the potential of a hybrid evolutionary strategy based on rules for rendering GP more effective. The EPR approach is similar to GP in terms of the class of results generated (symbolic formulas), but it circumvents some of GP's shortcomings by integrating a Genetic Algorithm (GA) (Goldberg, Citation1989) with a Least Squares (LS) approach. Similar to other evolutionary techniques, EPR provides a “transparent” and structured system identification, (Savic et al., Citation1999; Giustolisi & Savic, Citation2006). EPR is a two-stage method that (1) searches model structures based on a GA, and (2) estimates their parameters based on the linear optimization.
The EPR searches models having symbolic pseudo-polynomial structures, which are reported in Giustolisi & Savic (Citation2006). The general structure adopted for the evolutionary search in the present case study is given in the following equation:
Although the Single Objective approach of EPR has proven effective in several applications (Giustolisi & Savic, Citation2006; Giustolisi et al., Citation2004b,Citationc; Mancarella & Simeone, Citation2008), a Multi Objective (MO) strategy (Giustolisi & Savic, Citation2009) is implemented and integrated into EPR. The model structure search can thus be guided by model ranking according to both the goodness of fit of models to data and their structural complexity (i.e. the number of parameters and total number of inputs in the symbolic expression). This is expected to improve the identification of the system because more criteria are taken into account during the evolutionary search. The procedure will try to achieve a higher value of statistical fitness to data while forcing the model structure to be parsimonious (Giustolisi & Savic, Citation2009). An advantage is that, as a product of the MO approach, a set of models will be constructed and available for further judgement.
The MO optimization problem consists in finding a vector of decision variables, which satisfies constraints and optimizes a vector function whose elements represent the objective functions (Coello, Citation1999). These objective functions are representative of multiple performance criteria. Therefore, the goal of such optimization is to find a set of solutions which are acceptable for the analyst. This formally corresponds to finding the vector of decision variables:
Let
x
be a whatever vector of decision variables, not related to the aforementioned input vector
X
i
, the vector
x
* is said to be Pareto optimal, if there exists no different vector
x′
which would decrease some criterion indicator without causing a simultaneous increase in at least one other criterion (Coello, Citation1999; Van Veldhuizen & Lamont, Citation2000). Moreover, in Equationequation (9)(6), g and r are generic functions which represent the optimization constraints. These are user-assumed functions, which analytically describe the mathematical constraints bounding the dominion of decision variables. Pareto optimality almost never implies a single solution, but rather a set of solutions that constitute the so-called non-dominated set. The Pareto front is a surface of optimal solutions bounding a multi-dimensional domain C defined by possible values of the objective functions, whose expressions are generally given as Ck
().
Fig. 4 Representation of the Pareto front for a design space C of a dual-objective optimization problem. The solid line in the left part of the plot is the Pareto front.
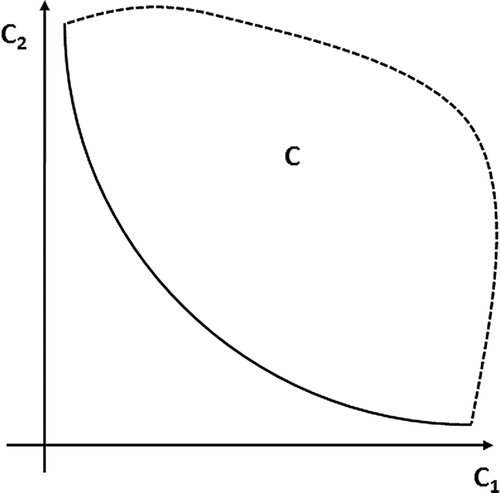
In the present work, the objective functions subject to minimization in MO EPR are: (a) a function addressing the performance of models in terms of fit to data, whose expression will be explained in the remainder, and (b) the number of parameters, aj . The latter objective function relates to the structural complexity of the models, #aj , here meant as the number of monomial groups constituting the equation returned by EPR and can be simply expressed as:
Therefore, EPR seeks the best non-dominated models with respect to both structural complexity and goodness of fit. Symbolic expressions are automatically ranked according to both their goodness of fit and complexity.
The GA used for the evolutionary stage of EPR is OPTIMOGA (Giustolisi et al., Citation2004a), which is employed to select the set of independent variables ( X k) that must form the model structure. Further details on OPTIMOGA can be found in Giustolisi et al. (2004), and Giustolisi & Savic (Citation2006) clearly describe how OPTIMOGA is applied in EPR in order to conduct the structural identification of models.
The data used in this study were pre-processed in order to set the proper input space to allow EPR to construct a differential equation. The input to the modelling process has been arranged so that the structures which are investigated by EPR have the general form reported in the following vector notation:
Following the structure given in equation, the outcomes of the modelling process are symbolic ordinary differential equations which are consistent with the specific dynamical response of the groundwater table to precipitation. The overall process will then represent an approach to infer groundwater system dynamics.
MODELLING RESULTS
The present study explores the possibility of employing data-driven techniques to support the modeller in building a model of a real groundwater system, by discovering the ODEs that describe its dynamics. This is one of the very first attempts that can be found in literature, especially on a real groundwater system; therefore, the presented results may be subject to future enhancements.
The EPR approach returned a set of four non-dominated equations, in the two-objective space represented by fitness and model structure complexity. The returned models belong to the Pareto front of the space of possible solutions allowed by EPR structures. Here for brevity, two models from the EPR-returned Pareto front are shown. These are characterized by different structural complexity:
The equations have clearly readable structures, and the differences as well as the similarities among the equations can be seen immediately. In particular, the main difference between the two equations is the presence of the 12-month moving average contribution. This has an opposite sign to the six-month contribution, thus providing a sort of counterbalancing effect for the rainfall.
reports the coefficient of determination (CoD) of the first-order derivative estimated by the model with respect to the first-order derivative obtained as central differences from measured data. The CoD is evaluated as follows:
Table 2 Coefficient of determination (CoD) values of the selected models on training and test data sets
Note that the closer CoD is to 1, the better the fit of model output to measured values. However, when CoD tends to zero, assuming that N is large enough, SSE divided by N is comparable to the estimate of the variance. This implies that the model is comparable to the simplest model, i.e. the mean value of the measured values. During the construction of the models, the EPR approach used the SSE as an objective function to describe the goodness of fit of the models to the data.
ANALYSIS AND DISCUSSION OF RESULTS
Comments on the results obtained by the modelling process, and on the capability of the proposed approach to infer ordinary differential equations related to the dynamics of the groundwater system studied, are made based on the similarity and differences in the terms composing the models. Some interesting considerations can be outlined from these equations. However, it is noteworthy that these are not aimed at emphasizing the superiority of EPR to conceptual models. This discussion is about how a data-driven technique might elicit the physical information contained in data. Nonetheless, this kind of approach is biased by the presence in data of non-useful information and of noise in general. Therefore, the identified differential equations cannot replace a classical conceptual model. At most, they can help modellers, even in the use of conceptual models, whereas part of the system's dynamics can be perceived by a data-driven differential equation. On this premise, it is possible to argue that:
-
EquationEquations (14)
(14) and ( Equation15
(15)) are of third order, which somehow reflects the three-tank conceptual model. Higher derivatives would occur if the system could be schematized with additional tanks. The space of possible solutions of the structural search included also higher-order derivatives that have been anyhow discarded by EPR in the model search. If higher-order interactions exist, they are not captured by the modelling process in the case study presented here. If the interaction between some components of the system produces low contributions to the modelled output, it can hardly be identified by data-driven approaches when their magnitude is comparable to the data noise.
-
The third derivative of h, which may be related to the interactions with downstream and upstream tanks, is present in all the models found with a magnitude comparable to the other terms, interactions with other tanks of the system appear strong. Idealized reservoirs upstream and downstream play an important role in determining water-level increase in the tank schematizing the aquifer. Since upstream and downstream tanks are assumed to represent respectively the vadose zone and hydrographic network, then evapotranspiration and drainage effects play an important role in determining water accumulation in the aquifer. Moreover, the term rules the decay of groundwater level, h when precipitation is absent (p = 0), i.e. the first-order derivative decays proportionally to this term with an additional negative bias.
-
A constant threshold term is always present in all models assuming a value in the selected models that is comprised in a narrow range. This term may be associated with the general mean outflow from the system and might somehow represent leakage rates (q 2D in ). This term is also relevant in conditioning the decay of water level, h, as we could expect on the basis of the groundwater system knowledge.
-
In both the models it is possible to observe that the 6-month moving average of rainfall is always selected, while the 12-month moving average is just selected in model (15). It is noteworthy that a 3-month moving average was never selected.
Both the presented equations were chosen for integration in order to further investigate their actual capability of describing the dynamics of the aquifer. In particular, numerical integration by ODE45 (Shampine, Citation1994; Shampine & Reichelt, Citation1997) of the selected model was attempted. Before the integration, a pre-processing procedure was undertaken. In fact, the structure of the differential equation is the one that has been identified by EPR in the evolutionary search. However, the coefficients were re-evaluated, since EPR was used on a central difference derivative scheme, while ODE45 does not assume the use of that differential approach (Shampine, Citation1994; Shampine & Reichelt, Citation1997). In particular, the coefficients were optimized using a single-objective GA by Giustolisi et al. (Citation2004a). The objective function was the minimization of the SSE between the measured groundwater table data and those returned by the integration of EPR models based on ODE45. This post-processing of the models was aimed at identifying a proper differential equation, which could be meaningful with respect to groundwater data, as well as coherent with the integration strategy.
After the post-processing of the coefficients, Equationequations (14)(14) and (
Equation15
(15)) became:
EquationEquations (17)(17) and (18) were integrated using the first four values of groundwater levels as initial condition, in order to evaluate up to the second-order derivative of h; moreover, the boundary conditions were represented by rainfall values p
6 and p
12.
Although the full dynamics of the water table variations cannot be fully captured by the model from a predictive point of view, a reasonable description of trend and cyclic components is obtained up to 250 months of simulation from assignment of initial condition. Integration results are shown in for Equationequation (17)(17) and in for Equationequation (18)
(18). Note that shows the results over a 20-year period for the training set, while for the test set the last 13 years are shown, omitting the early 6 years of data, i.e. 72 months. In particular, the last decision emphasizes that Equationequation (17)
(17) is able to simulate the phenomenon on a period which was not used for identification, and how the simulation quality is dependent on the initial conditions given to the ODEs. In fact, the integration over the test set is made assuming new initial conditions. Besides, looking at , it is evident how the choice of the initial condition can influence the results of the integration. Indeed, shows how the integration of Equationequation (17)
(17) on the entire test set, starting from January 1977 and over a period of 19 years, returns a description of the groundwater profile which gets worse than that in (b), as it gets further from the initial condition.
Fig. 5 Simulation results from the integration of Equationequation (17)(17): (a) training set and (b) test set.
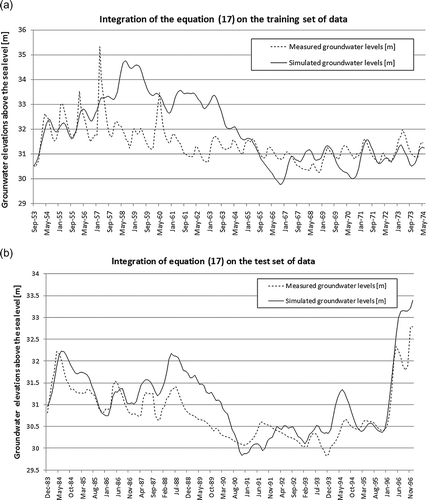
Fig. 6 Simulation results from the integration of Equationequation (17)(17) on the entire test set of 19 years.
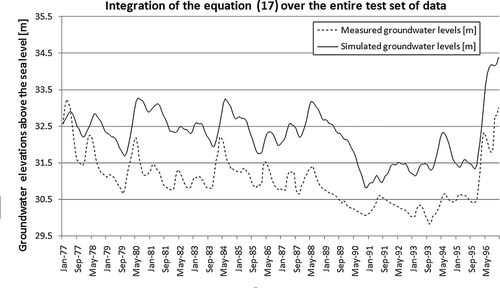
Fig. 7 Simulation results from the integration of Equationequation (18)(15) on the last 13 years of the test set.
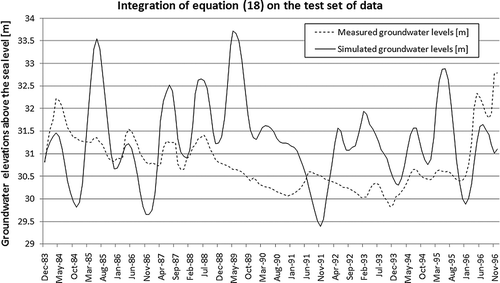
This shortcoming is probably related to the rainfall terms, but also to the use of a single differential equation over long periods, without changing either the initial conditions or the constant values. Clearly, changing the initial condition yearly should improve the simulation results, as would periodical re-evaluation of the constant values, given that the phenomenon is time varying at a time scale of several years. In particular, it seems that the simulated groundwater levels have higher inertia than the measured one. This is quite evident in the early part of the simulations, which is shown in the left part of the profiles of and . It is noteworthy that Equationequation (18)(18) presents two rainfall-related terms having opposite signs. This implies a sort of counterbalancing effect, in which the short-term effects related to the 6-month moving average are compensated by the 12-month components. Moreover, this makes the inertia to rainfall events lower than in Equationequation (17)
(17). However, this did not necessary imply better results, as shown in , where Equationequation (18)
(18) is integrated over the last 13 years of the test set, returning quite a poor profile. Nevertheless, measured groundwater table data probably contain information and effects related to other phenomena which are not accounted for. For instance, due to the lack of specific data, pumping effects resulting from the exploitation of the aquifer for irrigation are not considered. These are known to be non-secondary; moreover, the use of groundwater increased massively during the 44 years of measurement. In particular, the second part of the time series of groundwater levels covers a period in which the monitored aquifer was increasingly exploited for irrigation purposes. Irrigation typically corresponds to dry periods and, in the last 10 years of observation, a severe drought was experienced that considerably lowered the water table. These variations, due to both natural and human factors, made the identification of a data-driven differential equation even more challenging. For this reason, further investigations are required in order to properly explore the effects of long- and short-term components. The latter can probably help in accounting for those seasonal natural events which presently still seem to be underestimated. As far as the human effects are concerned, it is now difficult to estimate their entity and influence, since no relevant data are available.
As mentioned above, the very left part of the profiles in both and shows that the closer the values are to the initial condition, the higher the fitting of the simulation to the measured data. In Fig. , the values corresponding to the training and test sets are shown in parts (a) and (b), respectively. Here, the training and test sets correspond to the sets used for EPR. In particular, for both the equations, the simulation over the test set was performed assuming as initial conditions the first four values of groundwater levels belonging to the aforementioned test set.
Looking at the individual equations, Equationequation (17)(17) seems to overestimate groundwater levels after high peaks, as is evident in (a). However, it tends to return a good description of levels, where no important peaks are met. This can probably be related to the presence of a single rainfall term, which gives a high contribution where there are highly contributing rainfall periods. EquationEquation (18)
(18) seems to behave slightly differently to Equationequation (18)
(18), since it does not tend to constantly overestimate groundwater levels after a severely rainy period; however, it locally overestimates peaks, both high and low, as presented in . However, due to the aforementioned factors, it was envisaged that good reproduction of the groundwater profile by such equations was not possible.
The results can be considered encouraging, given that the ordinary differential equations describing the system have been identified solely from time-series data. The model broadly explains the general dynamics in the phreatic variation of the groundwater system considered. However, the dynamical response of the present hydrogeological system to precipitation seems to be strongly conditioned by the type of rainfall distribution over time, since there is a term penalizing water table increase after intense rainfall, and by saturation of the aquifer. The latter influences the way precipitation is accounted for, depending on the water level found in the aquifer during infiltration. Indeed, retention phenomena are expected to be lower at higher degrees of saturation and infiltration is more effective for aquifer recharge. The vadose zone behaves as a low-pass filter, since the model produces a higher increase of groundwater level when rainfall is frequent but not intense. Looking at the terms in the models, as previously outlined, the influence of water-level variations in the channel network, as well as accumulation in the vadose zone, appears relevant for the aquifer recharge, as a consequence of significant evapotranspiration and drainage effects. On the basis of field surveys in a limited number of wells, Ricchetti & Polemio (Citation1996) showed that the reconstructed piezometric surface denotes a significant drainage effect of the superficial hydrographic network. This close relationship is captured by the models induced by EPR. The methodology proposed here can potentially be applied to infer the dynamics of distributed systems, once reliable time-series data are provided in other locations. Within this work, a lumped parameter system was idealized and modelled by using only a piezometer and a rainfall gauge. The aim of the approach is to investigate and discover the general system dynamics of the shallow aquifer of Brindisi, rather than produce accurate forecasts.
CONCLUSIONS
We tried to model a real hydrogeological system by means of Evolutionary Polynomial Regression to infer its dynamics from pluviometric and piezometric time-series data, with some encouraging results. The aim of the proposed approach is to investigate the general system dynamics of the shallow aquifer of Brindisi, rather than to produce an accurate forecast. The study has pioneered results from one of the very first approaches that can be found in the literature, where evolutionary models are built and calibrated on the basis of time series to identify the differential equations which are consistent with the dynamics of a real groundwater system. The application presented herein is oriented to the identification of ODEs pertaining to the dynamical response of the groundwater table to precipitation. A set of equations is returned by the multi-objective evolutionary search of model structures and can thus be evaluated not only on the basis of goodness of fit to data, but also from the point of view of the modellers' physical knowledge. Some explicit terms show some similarities to those pertaining to a conceptual model of the aquifer. However, this is far from stating that their physical interpretation is possible. As this is a data-driven approach, if some components of the system interact according to a law that produces low contributions to the modelled output, some interactions might not be captured by the modelling process if their value is comparable to data noise. Indeed, the integration of the post-process identified ODEs returned results which may appear fair or even poor. However, the presented profiles represent integration over a period of 44 years, where boundary conditions are likely to have changed, even if no information about this is embedded into the model. Future work on this issue might address the investigation of these boundary effects, as well as seek a better definition of the numerical derivatives used by EPR.
Despite these limitations, EPR might provide a valid tool to approach the study of natural systems, and represent an approach which is parallel to a physically-based methodology. It can be used in order to enhance conceptual or physically-based models, especially when hydrological time series of data are available and the physical knowledge of the system is poor. Finally, the dynamical response of the studied hydrogeological system to precipitation appears strongly conditioned by the rainfall distribution over time, since in the returned equations there is a term penalizing water table increase after intense rainfall, and by saturation of the aquifer. The latter influences the way precipitation is accounted for, depending on the water level found in the aquifer during infiltration. The influence of water level variations in the hydrographic network as well as accumulation in the vadose zone appears relevant for the aquifer recharge, as a consequence of significant evapotranspiration and drainage effects.
REFERENCES
- Angeli , M. G. , Buma , J. , Gasparetto , P. , Pasuto , A. and Silvano , S. 1998 . A combined hillslope hydrology/stability model for low-gradient clay slopes in the Italian Dolomites . Engng Geol. , 49 : 1 – 13 .
- Arikan , A. 1988 . MODALP: a deterministic rainfall–runoff model for large karstic areas . Hydrol. Sci. J. , 33 : 401 – 414 .
- Babovic , V. and Keijzer , M. 2000a . “ Evolutionary algorithms approach to induction of differential equations ” . In Proc. Fourth Int. Conf. on Hydroinformatics 251 – 258 . Iowa City, , USA
- Babovic , V. and Keijzer , M. 2000b . Genetic programming as a model induction engine . J. Hydroinf. , 2 ( 1 ) : 35 – 61 .
- Barrett , M. E. and Charbeneau , R. J. 1997 . A parsimonious model for simulating flow in a karst aquifer . J. Hydrol. , 196 : 47 – 65 .
- Benjamin , J. R. and Cornell , C. A. 1960 . Probability, Statistics and Decision for Civil Engineering , New York : McGraw-Hill .
- Blijenberg , H. 1998 . The initiation of debris flows in the Bachelard Valley, France. PhD Thesis, Faculty of Geography , The Netherlands : University of Utrecht, Nederlandse Geografische Studies .
- Cao , H. , Kang , L. , Chen , Y. and Yu , J. 2000 . Evolutionary modeling of systems of ordinary differential equations with genetic programming . Genetic Programming and Evolvable Machines , 1 : 309 – 337 .
- Cao , H. , Kang , L. , Chen , Y. and Guo , T. 2003 . The dynamic evolutionary modeling of HODEs for time series prediction . Comput. Math. Appl. , 46 ( 8–9 ) : 1397 – 1411 .
- Chow , V. T. and Kulandaiswamy , V. C. 1971 . General hydrologic system model . J. Hydraul. Div. ASCE , 97 ( HY6 ) : 791 – 804 .
- Coello , C. A. C. 1999 . A comprehensive survey of evolutionary-based multiobjective optimization techniques . Knowl. Inf. Syst. , 1 ( 3 ) : 269 – 308 .
- Cotecchia , V. 1977 . Studi e ricerche sulle acque sotterranee e sull'intrusione marina in Puglia (Penisola Salentina) (Investigation on groundwater and salt intrusion phenomena of Apulia – Salento peninsula, in Italian) . Quad. Ist. Di Ricerca sulle Acque , 20 : 145 – 158 .
- Davidson , J. W. , Savic , D. A. and Walters , G. A. 2003 . Symbolic and numerical regression: experiments and applications . Inf. Sci. , 150 ( 1/2 ) : 95 – 117 .
- Dehn , M. , Gerd Bürger , G. , Buma , J. and Gasparetto , P. 2000 . Impact of climate change on slope stability using expanded downscaling . Engng Geol. , 55 : 193 – 204 .
- Doglioni , A. , Giustolisi , O. , Savic , D. A. and Webb , B. W. 2008 . An investigation on stream temperature analysis based on evolutionary computing . Hydrol. Processes , 22 ( 3 ) : 315 – 326 . doi:10.1002/hyp.6607
- Fenicia , F. , Savenije , H. H. G. , Matgen , P. and Pfister , L. 2005 . Is the groundwater reservoir linear? Learning from data in hydrological modelling . Hydrol. Earth Syst. Sci. Discuss. , 2 : 1717 – 1755. .
- Gasmo , J. M. , Rahardjo , H. and Leong , E. C. 2000 . Infiltration effects on stability of a residual soil slope . Computers and Geotechnics , 26 ( 2 ) : 145 – 165 .
- Giustolisi , O. , Doglioni , A. , Savic , D. A. and Laucelli , D. 2004a . “ A proposal for an effective multi-objective non-dominated genetic algorithm: the OPTimised Multi-Objective Genetic Algorithm: OPTIMOGA ” . In Report 2004/07, School of Engineering, Computer Science and Mathematics , UK : Centre for Water Systems, University of Exeter .
- Giustolisi , O. , Savic , D. A. and Doglioni , A. 2004b . “ Data reconstruction and forecasting by evolutionary polynomial regression ” . In 6th International Conference on Hydroinformatics , Edited by: Ljong , S. Y. , Phoon , K. K. and Babovic , V. Vol. 2 , 1245 – 1252 . Singapore : World Scientific Publishing Company .
- Giustolisi , O. , Savic , D. A. , Doglioni , A. and Laucelli , D. 2004c . “ Knowledge discovery by evolutionary polynomial regression ” . In 6th International Conference on Hydroinformatics , Edited by: Ljong , S. Y. , Phoon , K. K. and Babovic , V. Vol. 2 , 1647 – 1654 . Singapore : World Scientific Publishing Company .
- Giustolisi , O. and Savic , D. A. 2006 . A symbolic data-driven technique based on evolutionary polynomial regression . J. Hydroinf. , 8 ( 3 ) : 207 – 222 .
- Giustolisi , O. and Simeone , V. 2006 . Optimal design of artificial neural networks by a multi-objective strategy: groundwater level predictions . Hydrol. Sci. J. , 51 ( 3 ) : 502 – 523 .
- Giustolisi , O. , Doglioni , A. , Savic , D. A. and di Pierro , F. 2008 . An evolutionary multi-objective strategy for the effective management of groundwater resources . Water Resour. Res. , 44 : W01403 doi:10.1029/2006WR005359
- Giustolisi , O. and Savic , D. A. 2009 . Advances in data-driven analyses and modelling using EPR-MOGA . J. Hydroinf. , 11 ( 3–4 ) : 225 – 236 .
- Goldberg , D. E. 1989 . Genetic Algorithms in Search, Optimization and Machine Learning , USA : Addison Wesley .
- Harper , T. R. 1975 . The transient groundwater pressure response to rainfall and prediction of rock slope instability . Int. J. Rock Mechanics and Mining Science & Geomechanics Abstracts , 12 : 175 – 179 .
- Hinrichsen , D. and Pritchard , A. J. 2005 . Mathematical Systems Theory I, Modelling, State Space Analysis, Stability and Robustness , Berlin : Springer-Verlag .
- Iba , H. and Sakamoto , E. Inference of differential equation models by genetic programming . Proc. Genetic and Evolutionary Computation Conf. GECCO 2002 . New York, USA. pp. 788 – 795 .
- Knotters , M. and Bierkens , M. F. P. 2000 . Physical basis of time series models for water table depths . Water Resour. Res. , 36 ( 1 ) : 181 – 188 .
- Koza , J. R. 1992 . Genetic Programming: On the Programming of Computers by Natural Selection , Cambridge, Massachusetts : MIT Press .
- Kundzewicz , Z. W. and Napiórkowski , J. J. 1986 . Nonlinear models of dynamic hydrology . Hydrol. Sci. J. , 31 : 163 – 185 .
- Li , L. and Simonovic , S. P. 2002 . System dynamics model for predicting floods from snowmelt in North American prairie watersheds . Hydrol. Processes , 16 : 2645 – 2666 .
- Lijung , L. 1999 . System Identification: Theory for the User , second , Upper Saddle River, New Jersey, , USA : Prentice Hall ECS Professional .
- Mancarella , D. and Simeone , V. 2008 . Modellazione e previsione nei sistemi idrogeologici mediante EPR (Evolutionary Polynomial Regression) (EPR-based modelling and forecasting of hydrogeological systems, in Italian) . Giornale di Geologia Applicata , 8 ( 1 ) : 5 – 16 .
- Mathews , J. H. and Fink , K. K. 2004 . Numerical Methods Using Matlab , fourth , Upper Saddle River, New Jersey, , USA : Prentice-Hall Inc .
- Nise , N. S. 2004 . Control Systems Engineering , fourth , New York, , USA : John Wiley & Sons, Inc .
- Ricchetti , E. and Polemio , M. 1996 . L'acquifero superficiale del territorio di Brindisi: dati idrogeologici diretti e immagini radar da satellite . Memorie Della Società Geologica Italiana , 51 ( 2 ) (abstract in English)
- Savic , D. A. , Walters , G. A. and Davidson , J. W. 1999 . A genetic programming approach to rainfall–runoff modelling . Water Resour. Manag. , 13 : 219 – 231 .
- Şen , Z. 2000 . Non-Darcian groundwater flow in leaky aquifers . Hydrol. Sci. J. , 45 ( 4 ) : 595 – 606 .
- Shampine , L. F. 1994 . Numerical Solution of Ordinary Differential Equations , New York, , USA : Chapman & Hall .
- Shampine , L. F. and Reichelt , M. W. 1997 . The MATLAB ODE Suite . SIAM J. Scientific Computing , 18 : 1 – 22 .
- Soule , T. and Heckendorn , R. B. 2002 . An analysis of the cause of the code growth in genetic programming . Genetic Programming and Evolvable Machines , 3 : 283 – 309 .
- Spizzico , M. , Lopez , N. , Sciannamblo , D. and Tinelli , R. 2006 . La Piana di Brindisi: fenomeni di interazione fra le falde idriche sotterranee presenti nell'area . Giornale di Geologia Applicata , 3 : 17 – 24 . (abstract in English)
- Van Asch , Th. W. J , Hendriks , M. R. , Hessel , R. and Rappange , F. E. 1996 . Hydrological triggering of landslides in varved clays in the French Alps . Engng Geol. , 42 : 239 – 251 .
- Van der Kamp , G. and Gale , J. E. 1983 . Theory of earth tides and barometric effects in porous formations with compressible grains . Water Resour. Res. , 19 : 538 – 544 .
- Van Veldhuizen , D. A. and Lamont , G. B. 2000 . Multiobjective evolutionary algorithms analyzing the state-of-the-art . Evolutionary Computation , 8 ( 2 ) : 125 – 144 .
- Wanakule , N. and Anaya , R. A lumped parameter model for the Edwards aquifer. Tech . Report no. 163 . 1993 . Texas Water Resources Institute, Texas A&M University, College Station, USA
- Wittenberg , H. 2003 . Effects of season and man-made changes on baseflow and flow recession: case studies . Hydrol. Processes , 17 : 2113 – 2123 .
- Zhang , G. P. and Savenije , H. H. G. 2005 . Rainfall–runoff modelling in a catchment with a complex groundwater flow system: application of the Representative Elementary Watershed (REW) approach . Hydrol. Earth System Sci. , 9 : 243 – 261 .