Abstract
This study aims to assess the potential impact of climate change on flood risk for the city of Dayton, which lies at the outlet of the Upper Great Miami River Watershed, Ohio, USA. First the probability mapping method was used to downscale annual precipitation output from 14 global climate models (GCMs). We then built a statistical model based on regression and frequency analysis of random variables to simulate annual mean and peak streamflow from precipitation input. The model performed well in simulating quantile values for annual mean and peak streamflow for the 20th century. The correlation coefficients between simulated and observed quantile values for these variables exceed 0.99. Applying this model with the downscaled precipitation output from 14 GCMs, we project that the future 100-year flood for the study area is most likely to increase by 10–20%, with a mean increase of 13% from all 14 models. 79% of the models project increase in annual peak flow.
Citation Wu, S.-Y. (2010) Potential impact of climate change on flooding in the Upper Great Miami River Watershed, Ohio, USA: a simulation-based approach. Hydrol. Sci. J. 55(8), 1251–1263.
Résumé
Cette étude vise à évaluer l'impact potentiel du changement climatique sur les risques d'inondation pour la ville de Dayton, qui se trouve à l'exutoire du bassin supérieur de la Rivière Great Miami, Ohio, Etats-Unis. La méthode de cartographie des probabilités a tout d'abord été utilisé pour réduire l'échelle des simulations de précipitation annuelle de 14 modèles climatiques globaux (MCG). Nous avons ensuite construit un modèle statistique basé sur la régression et l'analyse fréquentielle de variables aléatoires pour simuler les débits annuels moyens et de pointe, à partir des précipitations. Le modèle fonctionne bien pour simuler les quantiles des débits annuels moyens et de pointe pour le 20ème siècle. Les coefficients de corrélation entre les quantiles simulés et observés pour ces variables dépassent 0.99. En appliquant ce modèle aux simulations de précipitations à échelle réduite de 14 MCG, nous prévoyons que la future crue centennale pour la zone d'étude va très vraisemblablement augmenter de 10–20%, avec une augmentation moyenne de 13% pour l'ensemble des 14 modèles. Soixante-dix-neuf pour cent des modèles projettent une augmentation du débit de pointe annuel.
INTRODUCTION
This research aims to assess the potential impact of climate change on flood risk for the city of Dayton, which lies at the outlet of the Upper Great Miami River Watershed, Ohio, USA. The objectives are: (a) to establish how precipitation will change for the area, using the downscaled results from global climate models (GCMs), and (b) to use this change to project the magnitude of a future 100-year flood, which is often used as a benchmark to evaluate flood risk.
Climate change has the potential to intensify the water cycle, causing more severe flooding events (Lettenmaier et al., Citation2008). This could have significant implications for water resource management (Kundzewicz et al., Citation2008). Milly et al. (Citation2005) projected changes in runoff globally based on 24 GCM runs for 2041–2060 relative to 1901–1970. In addition, they calculated fractions of model runs projecting the same direction as the median for all models to estimate the level of model agreement. Their results show that the Midwest region of the USA, where the study area of this research lies, is expected to have 10–25% increase in runoff (with 62% model agreement). Many studies have examined the impacts of climate change on regional or local hydrology (e.g. McCabe & Wolock, Citation2002; Hayhoe et al., Citation2006; Christensen & Lettenmaier, Citation2007; Maurer Citation2007; Rosenzweig et al., Citation2007). These studies typically use downscaling methods to produce forcings (usually precipitation and temperature) for a land hydrology model. Studies focusing on the Midwest region of the USA largely project an increase in streamflow. Jha et al. (Citation2004) used a regional climate model to downscale a mid-21st century global simulation of the HadCM2 global climate model to the upper Mississippi River basin. Their simulations showed that a 21% increase in future precipitation leads to a 50% net increase in surface water yield in the region. Takle et al. (Citation2006), using an ensemble of seven models used by the Intergovernmental Panel on Climate Change (IPCC) in its fourth assessment report (AR4), showed a more moderate increase of 5% in mean runoff. Such changes in runoff will have direct impact on the risk of flooding. Cameron (Citation2006) explored the impact of climate change on flood frequency for Lossie catchment in the northeast of Scotland, UK, using a continuous simulation methodology. Using HadCM3 and HadRM3 climate model output under various UKCIP02 scenarios, he found that the magnitude and direction of change in flood frequency are dependent on the scenarios. Fu et al. (Citation2007) extended the single-parameter precipitation elasticity of streamflow index into a two-parameter climate elasticity index, as a function of both precipitation and temperature, in order to assess climatic effects on annual streamflow. Applying this methodology to Spokane River basin, they found that the climate elasticity of streamflow index varies from 2.4 to 0.2, for a precipitation increase of 20%, as temperature varies from 1°C lower to 1.8°C higher than the long-term mean. That means a 20% precipitation increase may result in a streamflow increase of 48% if the temperature is 1°C lower, but only a 4% increase if the temperature is 1.8°C higher than the long-term mean.
A central issue in assessing the potential impact of climate change on flooding is that such study at local level often requires data of temporal and spatial resolutions that are not currently available from the output of global climate models (GCMs). There are two basic approaches to downscaling global climate models to the local scale: dynamic and statistical approaches. Even output from dynamic downscaling using regional climate models (RCMs), often with a grid size of 50 km or larger, does not provide sufficient resolution for relatively small watersheds. Therefore, statistical downscaling is usually the only choice that remains for climate impact assessments for small watershed hydrology (Wood et al., Citation2004). In addition, there is controversy on the general utility and reliability of using climate model output directly for hydrological studies (Prudhomme et al., Citation2002; Koutsoyiannis et al., Citation2008). Often local observation data are used in a variety of ways to correct the bias of climate models before they can be used for assessing the impacts on hydrological processes (Wood et al., Citation2004).
The general approach of statistical downscaling is to establish the empirical relationships between patterns in large-scale atmosphere–ocean circulation and smaller-scale climate (and related to hydrological) variables (Katz et al., Citation2002), as deterministic numerical models of the climate systems predict large-scale patterns (e.g. of “smooth” variables such as atmospheric pressure) much better than they do regional or local weather or climate variables (especially, “erratic” variables like precipitation) (Lettenmaier, Citation1995; Wilby et al., Citation1998). Most often, such empirical relationships are established by using least squares regression, and then used to interpolate future climate conditions. However, because of the difference in scales and the “smooth” vs “erratic” natures of dependent and independent variables, the correlation is usually low with a coefficient of determination (R 2) rarely over 0.20. As a result, this statistical downscaling approach is often found to be inadequate for hydrological studies (such as Souvignet et al., Citation2010). This paper proposes a different approach based on the statistics of extremes.
Basic methods in the statistics of extremes involve fitting a theoretical probability distribution model to the observed data series of extreme values (such as annual peak flow in flood frequency analysis). The derived models are then used to relate the magnitude of extreme events to their probability of occurrence. In this paper, this basic approach is used in two steps of the research. First, we use the probability distributions of the observed data to correct the GCM output, effectively downscaling the GCM results to the local scale based on the relationship between magnitude and probability of occurrence of any particular climate variable. Second, we establish a frequency-based statistical model to simulate present and future magnitude of a 100-year flood.
In order to account for uncertainty due to model choice in climate modelling, output from a suite of 14 GCM models is used to present the range of future projections. These models were utilized heavily in the reports of Working Groups I and II of the fourth IPCC assessment (IPCC, Citation2007a,Citationb), and have received unprecedented scrutiny by experts in a variety of fields. Based on this, we look for the agreement of these models. The multi-model approach has been used widely in climate impact assessments (e.g. Winkler et al., Citation2002, Citation2007; Milly et al., Citation2005; Hayhoe et al., Citation2006; Takle et al., Citation2006).
Four sections follow this introduction: the next section gives a brief account of the study area, followed by details of the methodology used, presentation of the results and their discussion, and finally, a brief conclusion.
STUDY AREA
This study focuses on the Great Miami River (GMR) located in southern Ohio State, USA, near the city of Dayton. The GMR is a tributary of the Ohio River, which runs along the southern border of Ohio. One of the Great Miami's source watersheds, and the focus of this study, is the Upper Great Miami Watershed (UGMW) (), which is composed of streams and tributaries from 11 different counties, including one in Indiana, and covers 6420 km2 of the Great Miami River Basin. The city of Dayton lies at the outlet of the UGMW, where the GMR meets its two major tributaries: the Stillwater and Mad River. It is highly vulnerable to flooding. The Great Dayton Flood of 1913 flooded the city and the surrounding area with water from the Great Miami River, causing the greatest natural disaster in Ohio history. The flood was created by a series of three winter storms that hit the region in March 1913. Within 3 days, 250–300 mm of rain fell throughout the Great Miami River watershed on frozen ground, resulting in more than 90% runoff, which caused the river and its tributaries to overflow. The existing series of levees failed, and downtown Dayton experienced flooding up to 6 m deep. This flood is still the flood of record for the Great Miami River watershed. As a result, flooding is a highly salient issue in this area.
METHODOLOGY
Downscaling climate model outputs through probability mapping
The objective of probability mapping is to obtain the probability distribution, rather than monthly/annual time series, of a climate variable, in this case annual mean precipitation. It involves the following steps. Let P obs be the observed annual mean precipitation, P GCM20 be the global climate model (GCM) output of simulated annual mean precipitation of the same period, and P GCM21 be the de-trended GCM simulated annual mean precipitation of the 21st century.
-
Fit a probability distribution function, F, for P obs, P GCM20, and P GCM21, respectively to get F obs(P obs), F GCM20(P GCM20) and F GCM21(P GCM21).
-
Map the GCM value to the observed distribution to correct its bias:
(1)
Correct the future GCM output with the scale of corrected/raw GCM results:
Developing a statistical model to simulate future peak flow
We first used the observed data series to establish: (a) a relationship between annual precipitation (P) and annual mean streamflow (Q mean); and (b) a relationship between mean streamflow (Q mean) and annual peak flow (Q peak). After fitting various linear and nonlinear models, we found that a linear model fits best for (a), and a power function gives the highest R 2 value for (b) (for details, see ). Therefore, we can specify the relationships in two steps as:
The ordinary least-square method was used to estimate the parameters α1, β1, α2, β2. We treated precipitation (P) and the residuals (E 1 and E 2) as random variables that follow certain distributions, and applied the frequency analysis technique to establish their respective probability distributions. We first used Monte Carlo simulation to simulate the 100-year (1% probability) flood for the present to validate our model, using the present annual precipitation as input. Then by using downscaled future precipitation data from GCMs as input for P, we could use the model to simulate and project future 100-year peak flows.
Frequency analysis
Frequency analysis was used to relate the quantity of a random variable to its frequency (probability) of occurrence through the use of probability distributions (Chow et al., Citation1998). In this study, the following distributions commonly used in studying extreme events were fitted to each of the data series in order to find the best fit:
– normal and related distributions: normal, lognormal, inverse Gaussian, generalized Gaussian; | |||||
– gamma family distributions: gamma, generalized gamma, Pearson III; | |||||
– extreme value distributions: generalized extreme value (GEV), Weibull; | |||||
– the logistic distributions: logistic, log-logistic; and | |||||
– beta distribution. |
The maximum likelihood (ML) method is used to estimate the parameters for each distribution. The ML method is considered the most efficient method, since it provides the smallest sampling variance of the estimated parameters and, hence, of the estimated quantiles, compared to other methods. The method of moments (MOM) is a natural and relatively easy parameter estimation method. However, MOM estimates are usually inferior in quality and generally are not as efficient as the ML estimates, especially for distributions with a large number of parameters (three or more), because higher-order moments are more likely to be highly biased in relatively small samples (Rao & Hamed, Citation2000). The probability weighted moments method (PWM) gives parameter estimates comparable to the ML estimates (Rao & Hamed, Citation2000) in most cases. The χ2 test is used as the main method to evaluate the goodness of fit for these distributions in order to choose the best one for the data series. The χ2 test is the most common test for goodness of fit, although it has a major disadvantage in that the test result is dependent on the binning of the data. For this study, we calculated the number of bins (k) from sample size (N) through the following commonly-used formula:
As additional methods to evaluate distribution, statistics such as mean, variance, skewness etc., as well as quantile values derived from fitted distributions, are compared with those from the observed data series (Q–Q plot). In this way, we can not only evaluate the fitting of the models over the entire data range, but also examine the model fitting in particular regions, such as tails where most extreme events occur. The Kolmogorov-Smirnov (K-S) and Anderson-Darling (A-D) tests are sometimes used to evaluate the goodness of fit of distributions. They have the advantage that the test results are not dependent on binning of data. However, they have their own limitations. The most serious limitation of the K-S test is that the distribution must be fully specified. That is, if location, scale and shape parameters are estimated from the data (as is the case in our study), the critical region of the K-S test is no longer valid. It must typically be determined by simulation (NIST/SEMATECH, Citation2006). The A-D test is a modification of the K-S test that makes use of the specific distribution in calculating critical values. This has the advantage of allowing a more sensitive test and the disadvantage that critical values must be calculated for each distribution. As a result, the A-D test is only available for a few specific distributions. For many distributions used in this study, the critical values for A-D test are not established. Because of these limitations, K-S and A-D tests are not used to evaluate distributions in this study.
Data
Observed data (P obs)
US National Climatic Data Center (NCDC) annual mean precipitation data are spatially interpolated and aggregated within the watershed boundary. A total of 123 stations within and surrounding the watershed were chosen to interpolate the spatial distribution of precipitation (). Three spatial interpolation methods were tried on the data set: inverse distance weighted (IDW), tension spline and kriging. For cross-validation, we removed one data location at a time and predict the associated data value using the remaining data points. We then calculated the root mean squared error for all data locations. We decided to use kriging as the best method for the data set based on the cross-validation results (), and used it for spatial interpolation of annual mean precipitation of each year (). The interpolated values were then aggregated by the watershed in order to generate an annual mean precipitation data series for the whole watershed.
Table 1 Cross-validation results for annual precipitation depth (mm)
GCM data (P GCM20 and P GCM21)
We used the output from 14 GCM models used in the IPCC Fourth Assessment Report (AR4) under the A2 emissions scenario. We extracted data of the nearest grid cell on: (a) simulated 20th century annual mean precipitation (P GCM20), and (b) simulated 21st century annual mean precipitation (P GCM21). The exact location of the grid cell varies depending on the model, but they all contain the majority of the watershed. illustrates a typical location of the GCM grid cell for which output data is extracted, in relation to the study area.
Stationarity
The basic assumption for frequency analysis is that there is no significant trend in the data. In other words, the data have to be stationary. This is necessary because frequency analysis attempts to establish a distribution of an ensemble of a variable at any given time step. Often a single time slice does not give us a sufficient amount of data to characterize the distribution of a variable. However, when there is no significant trend in the data series, we can use the time series distribution to approximate the ensemble distribution at a particular time. The observed data for the Upper Great Miami Watershed show no significant trend. The standard W–W test gives the W statistic the value of 0.5, far smaller than the 95 percentile value of 1.96. Therefore the stationarity assumption is satisfied.
Most GCM output of annual precipitation series for the 20th century also shows no significant trends for this location. However, the GCM output of annual precipitation series for the 21st century usually shows some trends. This is to be expected, because the GCMs have a built-in climate shift driven by increasing atmospheric concentrations of greenhouse gases. Most of the models project increasing precipitation, whereas a few of them project no change or decreasing trends for the study area. Therefore, we needed first to de-trend the 21st century data by adding the estimated trend to all data points for the 21st century (). By doing so, we established an ensemble of annual precipitation data for the time step of 2100. We could then compare it with the ensemble of 20th century (i.e. present) using frequency analysis techniques.
Streamflow data (Q mean and Q peak) Annual mean streamflow (Q mean) and annual peak flow (Q peak) were collected from the National Water Information System (NWIS) maintained by the US Geological Survey (USGS) for the gauge station near Dayton at the outlet of the watershed. The data sets ran from 1931 to 1997, and show no significant trends during this time period.
RESULTS
Downscaling of GCM precipitation output
Probability mapping works reasonably well to downscale the GCM output. After fitting all the distributions listed in the methodology section and running the goodness-of-fit tests, the best distribution was chosen for: the observed annual mean precipitation, the GCM simulated 20th century annual mean precipitation and the de-trended GCM output for 21st century annual mean precipitation from 14 GCMs. A distribution was evaluated based on the following criteria: (a) χ2 test results; (b) comparison of shape statistics of fitted distributions with observed values; and (c) comparison of quantile values derived from fitted distributions with those from observation. Using annual precipitation as an example, shows the χ2 test results and distribution shape statistics for the observed data series and various fitted distributions. displays the observed and modelled quantile values of annual mean precipitation for fitted normal distribution. presents the RMS difference between observed and modelled quantile values for mean precipitation for various fitted distributions. Based on these results, normal distribution is selected as the best approximation for the observed annual precipitation data series. A similar procedure was carried out for all GCM 20th and 21st century model output data for the nearest grid to our watershed. summarizes the fitting results, showing the selected distribution, the χ2 test results and the RMSE of quantile values for each of these model output data series. Based on the best distributions, we corrected the bias in the GCMs using the probability mapping method outlined in the Methodology Section. shows the percentile values for the raw and corrected GCM output from the PMI ECHAM5 model. It can be seen that ECHAM5 model has a consistent bias of about 300 mm for all percentiles. The output of other GCMs has biases of similar magnitude. This amount is about one third of the annual mean precipitation of the area, and twice the projected increase in annual mean precipitation. Bias of this magnitude makes it inappropriate to use GCM output directly for local hydrological studies.
Table 2 Summary statistics and χ2 tests for fitted distributions for observed annual mean precipitation (mm)
Table 3 The RMS difference between observed and modelled quantile values for annual mean precipitation
Table 4 Summary of fitting results for 20th and 21st century precipitation output from GCMs
Estimation of model parameters
With an R 2 value of 0.72, the linear regression model seems to fit well for the relationship between the observed precipitation data of GMW and the annual mean streamflow at the Dayton station (). The values of α1, and β1 are estimated using the least-squares method. To estimate the relationship between the mean streamflow and mean peak flow, we fitted linear, exponential, power and logarithmic regression models (). With an R 2 of 0.51, the power function seems to perform the best. Similarly, α2, and β2 are estimated using the least-squares method.
Fig. 6 Linear regression between annual mean streamflow (Q mean) at Dayton station and annual precipitation (P) of the watershed.
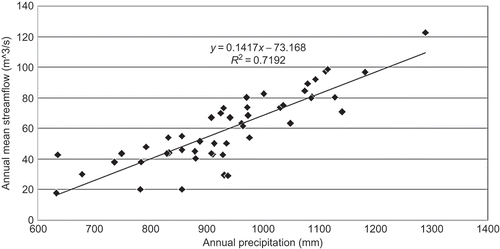
Various distribution functions are fitted to the random variables P, E 1 and E 2. The best distributions are chosen based on the χ2 goodness-of-fit test and other methods outlined in the Methodology Section. When evaluating distributions, particular attention is given to the fitness of distribution at the tails, where extreme events occur. The best distributions are presented in Figs , together with their Q–Q plots to visualize the goodness of fit of the models.
The final model is expressed as:
-
P ∼ Normal (μ = 952.21, σ = 147.21)
-
E 1 ∼ Weibull (k = –0.079, μ = –5.45, σ = 10.78)
-
E 2 ∼ Log-logistic (α = 5.99, β = 746.29, γ = –750.89)
Validation of models
In order to validate the models, we used Monte Carlo simulation from the distribution of observed precipitation data to get the percentile values for mean streamflow and peak flow values and compare them with observations. It can be seen from and that we can model the distribution of annual mean streamflow (Q mean) and the annual peak flow (Q peak) very well. The root mean squared (RMS) difference between observed and modelled quantile values is 61 mm for precipitation (or 6% of average annual mean precipitation), and 35 m3/s for annual peak flow (or 4% of average annual peak flow).
Future 100-year peak flow
After model validation, we used the bias-corrected precipitation output from the 14 GCMs for the 21st century as our model input, and ran the same model to get the magnitude of 100-year (1% probability) peak flow for the end of the 21st century. The results are presented in . The mean change from all models is a 13% increase from the present 100-year peak flow. In terms of model agreement, 11 out of 14 models (79%) projected an increase in 100-year peak flow. Eight out of those 11 models (73%, or 57% of the total number of models) projected an increase of between 10 and 20% in 100-year peak flow from present.
CONCLUSIONS
The probability mapping approach works well to downscale GCM output to the scale of the study area. The method produces from GCM output data quantile values very close to observations. The statistical model performs very well in simulating observed annual mean and peak streamflow values. By applying this model to output from 14 GCMs, we could project the future 100-year peak flow for the study area as most likely to increase between 10 and 20%, with the mean increase of 13% from all models. This will have social and economic implications for the Dayton area, as more of the heavily developed riparian area would be exposed to increased flood risk. In order to quantify such impacts, it is necessary to map the present and future flood plain areas and overlay this with land use, population distribution, and infrastructure data. Further study will be conducted in that direction.
The methodology used in this paper is largely based on frequency analysis, which assumes stationarity of data series. This basic assumption is not satisfied with watersheds where long-term trends exist for annual mean precipitation or other hydro-climatic variables. However this methodology can be adjusted to accommodate such trends. Instead of examining the trend in the whole time series, we can compare two specific time steps. To generate an ensemble distribution for one specific time step, we can first de-trend the time-series data, and use the local mean of the specific time step to adjust the distribution of the de-trended data to get an approximation of the ensemble distribution of that particular time step. We can use a similar method to establish the distribution for different points in time, and use these distributions to simulate peak flow magnitudes at these time steps in order to examine the consequent trends that might exist in peak flow. Getting an ensemble distribution through de-trending assumes that the shape of distribution of the variable is constant. If there is reason to suspect that the shape of the distribution (such as variance, skewness and kurtosis) also changes during the time period, we should only use a limited neighbourhood around a specific point in time we are interested in, in order to establish a local distribution. Bootstrapping techniques may be necessary to estimate the distribution. Alternatively, a time-series model might be considered if ergodicity of the process can be established.
REFERENCES
- Cameron , D. 2006 . An application of the UKCIP02 climate change scenarios to flood estimation by continuous simulation for a gauged catchment in the northeast of Scotland, UK (with uncertainty) . J. Hydrol , 328 : 212 – 226 .
- Chow , V. T. , Maidment , D. R. and Mays , L. W. 1988 . Applied Hydrology , New York : McGraw-Hill .
- Christensen , N. S. and Lettenmaier , D. P. 2007 . A multimodel emsemble approach to assessment of cliamte change impacts on the hydrology and water resources of the Colorado River basin . Hydrol. Earth System Sci , 11 : 1417 – 1434 .
- Fu , G. , Charles , S. P. and Chiew , H. S. 2007 . A two-parameter climate elasticity of streamflow index to assess climate change effects on annual streamflow . Water Resour. Res , 43 doi:10.1029/2007WR005890
- Hayhoe , K. , Wake , C. , Huntington , T. G. , Luo , L. , Schwartz , M. D. , Sheffield , J. , Wood , E. F. , Anderson , B. , Bradbury , J. , DeGaetano , T. T. and Wol , D. 2006 . Past and future changes in climate and hydrological indicators in the US Northeast . Clim. Dyn. , 28 ( 4 ) : 381 – 407 .
- IPCC (2007a) Climate Change 2007: The Physical Science Basis. Contribution of Working Group I to the Fourth Assessment Report of the Intergovernmental Panel on Climate Change (S. Solomon, D. Qin, M. Manning, Z. Chen, M. Marquis, K. B. Averyt, M. Tignor & H. L. Miller, eds). Cambridge: Cambridge University Press. http://www.ipcc.ch/pdf/assessment-report/ar4/wg1/ar4-wg1-spm.pdf (http://www.ipcc.ch/pdf/assessment-report/ar4/wg1/ar4-wg1-spm.pdf) (Accessed: 5 October 2007 ).
- IPCC (2007b) Climate Change 2007: Impacts, Adaptation and Vulnerability. Contribution of Working Group II to the Fourth Assessment Report of the Intergovernmental Panel on Climate Change (M. L. Parry, O. F. Canziani, J. P. Palutikof, P. J. van der Linden & C. E. Hanson, eds). Cambridge: Cambridge University Press. http://www.ipcc.ch/pdf/assessment-report/ar4/wg1/ar4-wg1-spm.pdf (http://www.ipcc.ch/pdf/assessment-report/ar4/wg1/ar4-wg1-spm.pdf) (Accessed: 5 October 2007 ).
- Jha , M. , Pan , Z. , Takle , E. S. and Gu , R. 2004 . Impacts of climate change on streamflow in the upper Mississippi River basin: a regional climate model perspective . J. Geophys. Res , 109 doi:10.1029/2003JD003686
- Katz , R. W. , Parlange , M. B. and Naveau , P. 2002 . Statistics of extremes in hydrology . Adv. Water Resour , 25 : 1287 – 1304 .
- Koutsoyiannis , D. , Efstratiadis , A. , Mamassis , N. and Christofides , A. 2008 . On the credibility of climate predictions . Hydrol. Sci. J , 53 ( 4 ) : 671 – 684 .
- Kundzewicz , Z. W. , Mata , L. J. , Arnell , N. W. , Döll , P. , Jimenez , B. , Miller , K. , Oki , T. , Şen , Z. and Shiklomanov , I. 2008 . The implications of projected climate change for freshwater resources and their management . Hydrol. Sci. J , 53 ( 1 ) : 3 – 10 .
- Lettenmaier , D. 1995 . “ Stochastic modeling of precipitation with applications to climate model downscaling ” . In Analysis of Climate Variability: Applications of Statistical Techniques , Edited by: von Storch , H. and Navarra , A. 197 – 212 . Berlin : Springer Verlag .
- Lettenmaier , D. P. , Major , D. , Poff , L. and Running , S. 2008 . “ Water resources. In: U. C. Research ” . In The Effects of Climate Change on Agriculture, Land Resources, Water Resources, and Biodiversity in the United States , Edited by: Bucklon , P. , Janetos , A. and Schimel , O. 121 – 150 . Washington, DC : US Climate Change Science Program .
- Maurer , E. P. 2007 . Uncertainty in hydrologic impacts of climate change in the Sierra Nevada, California under two emissions scenarios . Clim. Change , 82 : 309 – 325 .
- McCabe , G. J. and Wolock , D. M. 2002 . Trends and temperature sensitivity of moisture conditions int he conterminous United States . Clim. Res , 20 : 19 – 29 .
- Milly , P. C. , Dunne , K. A. and Vecchia , A. V. 2005 . Global pattern of trends in streamflow an water availability in a changing climate . Nature , 438 : 347 – 350 .
- NIST/SEMATECH. (2006) e-Handbook of Statistical Methods. Available fromLast updated: 18 July 2006 http://www.itl.nist.gov/div898/handbook/ (http://www.itl.nist.gov/div898/handbook/) (Accessed: 31 July 2009 ).
- Prudhomme , C. , Reynard , N. and Crooks , S. 2002 . Downscaling of global climate models for flood frequency analysis: where are we now? . Hydrol. Processes , 16 ( 6 ) : 1137 – 1150 .
- Rao , A. R. and Hamed , K. H. 2000 . Flood Frequency Analysis , Boca Raton, FL : CRC Press .
- Rosenzweig , C. , Major , D. C. , Demong , K. , Stanton , C. , Horton , R. and Stults , M. 2007 . Managing climate change risks in New York City's water system: assessment and adaptation planning . Mitigation and Adaptation Strategies for Global Change , 12 ( 8 ) : 1391 – 1409 .
- Souvignet , M. , Gaese , H. , Ribbe , L. , Kretschmer , N. and Oyarzún , R. 2010 . Statistical downscaling of precipitation and temperature in north-central Chile: an assessment of possible climate change impacts in an arid Andean watershed . Hydrol. Sci. J , 55 ( 1 ) : 41 – 57 .
- Takle , E. S. , Anderson , C. , Jha , M. and Gassman , P. W. 2006 . “ Upper Mississippi River basin modeling system Part 4: climate change impacts on flow and water quality ” . In Coastal Hydrology and Processes , Edited by: Singh , V. P. and Xu , Y. J. 135 – 142 . Littleton, CO : Water Resrouces Publications .
- Wilby , R. L. , Wigley , T. M. , Conway , D. , Jones , P. D. , Hewitson , B. C. , Main , J. and Wilks , D. S. 1998 . Statistical downscaling of general circulation model output: a comparison of methods . Water Resour. Res , 34 : 2995 – 3008 .
- Winkler , J. A. , Andresen , J. A. and Guentchev , G. 2002 . Possible impacts of projected temperature change on commercial fruit production in the Great Lakes region . J. Great Lakes Res. , 28 ( 4 ) : 608 – 625 .
- Winkler , J. A. , Potter , B. E. and Wilhelm , D. F. 2007 . Climatological and statistical characteristics of the Haines Index for North America . Int. J. Wildland Fire , 16 ( 2 ) : 139 – 152 .
- Wood , A. W. , Leung , L. R. and Lettenmaier , D. P. 2004 . Hydrological implications of dynamical and statistical approaches to downscaling climate model outputs . Clim. Change , 62 ( 1‐3 ) : 189 – 216 .