Abstract
To explore the spatial and temporal variations of the reference evapotranspiration (ETref) is helpful to understand the response of hydrological processes to climate changes. In this study, ETref was calculated by the Penman-Monteith method (P-M method) using air temperature, wind speed, relative humidity and sunshine hours at 89 meteorological stations during 1961–2006 in the Yellow River Basin (YRB), China. The spatial distribution and temporal variations of ETref were explored by means of the kriging method, the Mann-Kendall (M-K) method and the linear regression model, and the causes for the variations discussed. The contribution of main meteorological variables to the variations of ETref was explored. From the results we found that: (1) the spatial distributions of ETref display seasonal variation, with similar spatial patterns in spring, summer and autumn; (2) temporal trends for ETref showed large variation in the upper, middle and lower regions of the basin, most of the significant trends (P = 0.05) were detected in the middle and lower regions, and, in particular, the upward and downward trends were mainly detected in the middle region and lower region of the basin, respectively; and (3) sensitivity analysis identified the most sensitive variable for ETref as relative humidity, followed by air temperature, sunshine hours and wind speed at the basin scale.
Citation Yang, Zhifeng, Liu, Qiang & Cui, Baoshan (2011) Spatial distribution and temporal variation of reference evapotranspiration during 1961–2006 in the Yellow River Basin, China. Hydrol. Sci. J. 56(6), 1015–1026.
Résumé
Pour explorer les variations spatiales et temporelles de l'évapotranspiration de référence (ETref), il est utile de comprendre la réponse des processus hydrologiques aux changements climatiques. Dans cette étude, ETref a été calculée par la méthode de Penman-Monteith (méthode P-M) en utilisant les données de température de l'air, de vitesse du vent, d'humidité relative et de durée d'ensoleillement de 89 stations météorologiques du bassin du Fleuve Jaune, en Chine, couvrant la période 1961–2006. La distribution spatiale et les variations temporelles de ETref ont été explorées au moyen de la méthode de krigeage, de la méthode de Mann-Kendall (M-K) et du modèle de régression linéaire, et les causes des variations discutées. La contribution des principales variables météorologiques aux variations de ETref a été explorée. D'après les résultats nous avons constaté que: (1) la distribution spatiale de ETref présente une variation saisonnière, avec des configurations spatiales similaires au printemps, en été et en automne; (2) les tendances temporelles de ETref présentent de grandes variations dans les régions supérieures, moyennes et inférieures du bassin, la plupart des tendances significatives ayant été détectées dans les régions moyennes et inférieures, en particulier les tendances à la hausse et à la baisse principalement détectées respectivement dans les régions moyenne et inférieure du bassin; et (3) l'analyse de sensibilité a identifié que la variable la plus sensible pour ETref est l'humidité relative, suivie par la température de l'air, la durée d'ensoleillement et la vitesse du vent à l'échelle du bassin.
INTRODUCTION
In face of increasing water stress on the global scale, it is important to improve understanding of hydrological mechanisms underlying ecological patterns and processes (Rodriguez-Iturbe Citation2000, Zalewski Citation2000, Porporato et al. Citation2002). On the one hand, climate and soil control the vegetation dynamics; while on the other hand, vegetation exerts important control on the overall water balance and is responsible for many feedbacks with the atmospheric system (Rodriguez-Iturbe et al. Citation2001). Precipitation and evapotranspiration are regarded as the most important variables to diagnose climate change and reveal the environmental response to climate change on the basin scale (Yao et al. Citation2005, Cannarozzo et al. Citation2006, Liu et al. Citation2008). The temporal–spatial patterns of precipitation and evapotranspiration influence the eco-hydrological processes, which control the evolution of surface ecosystems (Cannarozzo et al. Citation2006, Oguntunde et al. Citation2006, McVicar et al. Citation2007, Zhan et al. Citation2008). This type of inquiry is fundamental to understand the coupling existing between ecosystem dynamics and the water cycle (Porporato and Rodriguez-Iturbe Citation2002, Rodriguez-Iturbe and Porporato Citation2005), which is important to explore the water needs of ecosystems and to optimize water resources allocation and management (Yang et al. Citation2005). Estimation of water needs must be adjusted to the atmospheric demand, which is related to the climatic conditions.
To deal with these issues, ETref was proposed by the United Nations Food and Agriculture Organization (FAO) for computing crop evapotranspiration, combined with crop coefficients (Allen et al. Citation1998, Jabloun and Sahli Citation2008). The ETref is defined as “the rate of evapotranspiration from a hypothetical reference crop with an assumed crop height of 0.12 m, a fixed surface resistance of 70 s m-1 and an albedo of 0.23, closely resembling the evapotranspiration from an extensive surface of green grass of uniform height, actively growing, well-watered, and completely shading the ground” (Allen et al. Citation1998). Now, ETref is regarded as one of the most important hydrological variables for scheduling irrigation systems, preparing input data to hydrological water-balance models, and calculating actual evapotranspiration for a region and/or a basin (Xu and Singh Citation2002, Xu and Li Citation2003, Gong et al. Citation2006, Kannan et al. Citation2007, Yin et al. Citation2008, Zhou et al. Citation2009). Furthermore, to obtain useful information for water resource regulation and management, accurate spatial and temporal patterns of ETref are also needed (Xu et al. Citation2006a). Xu et al. (Citation2006a, Citation2006b) investigated the spatial distribution and temporal trends of ETref in the Changjiang (Yangtze River) Basin. The research showed that the highest and lowest values for ETref occurred in the upper region and in the middle region of the basin, respectively, and a significant decreasing trend was detected in ETref for the whole catchment because of a significant decreasing trend in net total radiation and, to a lesser extent, a significant decrease in the wind speed.
Considering the influence of topography and land-surface conditions on the forcing variables, McVicar et al. (Citation2007) used an “interpolate-then-calculate” approach to obtain spatially-distributed ETref, and their results demonstrated that the distribution of monthly ETref with high spatial resolution can be spatially modelled while taking into account the influence of topography. In order to explore the spatial distribution of temporal trends on the basin scale, it is most important to understand the mechanism of interaction between climate, vegetation and hydrological processes, and to forecast the hydrological processes using hydrological models (Yao and Creed Citation2005). This can provide useful information to optimize the water resource management and maintain the ecosystem integrity. However, investigating the spatial distribution of temporal trends in ETref time series still remains a difficult task due to their complex and nonlinear nature in different regions, and relatively little research has been conducted on it (Cohen et al. Citation2002, Xu et al. Citation2006a, McVicar et al. Citation2007).
The objectives of this study are: (a) to calculate ETref with the Penman-Monteith (P-M) method using air temperature, wind speed, relative humidity and sunshine hours, and to analyse the spatial distribution of ETref in different seasons; (b) to explore temporal trends and their spatial distribution by the non-parametric Mann-Kendall (M-K) method and a linear regression model; and (c) to conduct sensitivity analysis to investigate the relative change in annual ETref due to relative change in the four meteorological variables: air temperature, wind speed, relative humidity and sunshine hours. All tests were based on ETref series calculated at 89 meteorological stations from the period 1961–2006 in the Yellow River Basin (YRB), China.
STUDY AREA
The Yellow River is about 5400 km long with a drainage area of 7.95 × 105 km2 (). It directly supports a population of 107 million people (McVicar et al. Citation2007). Originating from the Tibetan Plateau, the river flows through the Loess Plateau and North China Plain, and runs into the Bohai Sea. From the headstream to the estuary of the Yellow River, the watershed characteristics vary with the changes in topography and elevation. The area from upstream to Lanzhou, well covered by vegetation, is the main source of water resources, and about 54% of the river runoff is from this region (Jia et al. Citation2006). The headwater part of the Yellow River Basin, in particular, is covered by snow and frozen soil throughout the whole year. As the river flows through the Loess Plateau, most of the erosion is produced by the rainstorms that frequently occur in the flood season, combined with the loose soil texture and sparse vegetation in this region (Jia et al. Citation2006). As a result of high erosion rates from the Loess Plateau and the continuous presence of levees and dykes, the well-known suspended river has formed in the lower reach of the YRB—the bottom of the river bed is, in places, 20 m above the surrounding land surface (Li Citation2003). As most of the basin is located in arid and semi-arid regions, precipitation is concentrated in intense, but infrequent, storms (Yu Citation2002). The increase in agricultural and industrial water use, combined with a decrease in precipitation, has contributed to the decrease in runoff and drying-up in the YRB (Liu and Cheng Citation2000, McVicar et al. Citation2002, Liu Citation2004, Yang et al. Citation2004, Cong et al. Citation2009). According to Liu et al. (Citation2008), precipitation in the YRB presented decreasing trends at most stations during 1961–2006. Yang et al. (Citation2004) investigated the causes of drying up of the Yellow River and found that this can be attributed to the processes of precipitation and evaporation, as well as irrigation. Cong et al. (Citation2009) found the simulated natural runoff follows a similar trend to the precipitation in the YRB during the last half century, and this implies that changes in the natural runoff are mainly controlled by the climate variability and change rather than land-use change. Chen et al. (Citation2006) explored the variations of P-M ETref and its causes on the Tibetan Plateau. They detected negative trends in P-M ETref for the Tibetan Plateau. Changes in wind speed and, to a lesser degree, in relative humidity were found to be the most important meteorological variables affecting P-M ETref trends on the Tibetan Plateau while changes in sunshine duration played an insignificant role.
DATA AND METHOD
Data
The time series of the monthly records of air temperature, wind speed, sunshine hours and relative humidity for the period 1961–2006 at 89 meteorological stations in the YRB, China were used in this study. The data were provided by the National Climate Centre (NCC) of the China Meteorological Administration (CMA). The location of the stations in YRB is shown in . The annual values of ETref were obtained by summing monthly ETref at each of the 89 station for 1961–2006, calculated from the mean monthly data of air temperature, wind speed, sunshine hours and relative humidity by the P-M method.
In order to have a rough idea on the climate of the study region, the basin was divided into three parts, mainly according to the topography and elevation (). The dividing line for the upper and middle regions is mainly along the border of the Tibetan Plateau and the Loess Plateau, at about 2000 m above sea level (m a.s.l.), while that between the middle and lower regions is mainly along the dividing line of the Loess Plateau and the plains, at about 1000 m a.s.l. The mean elevations for the upper, middle and lower regions are 3693, 1403 and 677 m a.s.l., respectively.
The mean monthly values of the major meteorological variables were plotted () for the upper, middle, and lower regions, and the whole basin. The variations in the meteorological variables indicate that: (a) the monthly variation of air temperature in the lower region is greatest, followed by that in middle and upper regions; (b) the relative humidity is higher in the lower region than in other regions in all months except for June, and the maximum relative humidity occurs in the period July–September in the basin; (c) the maximum wind speed occurs in April for all regions, and wind speed is higher in the lower region than that in other regions; and (d) sunshine hours present a similar pattern in the middle and lower regions with a maximum value occuring in June, but the value is relatively higher in April, August and November in the upper region of the YRB.
METHOD
The Penman-Monteith method
The P-M method is a physically-based model for estimating ETref, which explicitly incorporates both radiative and aerodynamic parameters (Xu et al.
Citation2006a, McVicar et al.
Citation2007). It has been accepted as the most accurate method to estimate the ETref for its better results when compared with other equations in various regions of the world (López-Urrea et al.
Citation2006), and recommended by the FAO as the best method to determine ETref (Allen et al.
Citation1998). The P-M method (Equationequation (1)(1)) was selected to estimate ETref in this study:
where ETref is the reference evapotranspiration (mm d-1), Rn is the net radiation at surface (MJm-2 d-1), G is the soil heat flux density (MJm-2 d-1), T is the mean daily air temperature at 2 m height (°C), u 2 is the wind speed at 2 m height (m s-1), es is the saturation vapour pressure (kPa), ea is the actual vapour pressure (kPa), es – ea is the saturation vapour pressure deficit (kPa), Δ is the slope of the vapour pressure curve (kPa °C-1), γ is the psychrometric constant (kPa °C-1). If the time-step of all data is monthly then the resultant ETref is provided with units of mm month-1 (Allen et al. Citation1998, McVicar et al. Citation2007). One can calculate Δ and Rn from the following equations:
where, n is the actual duration of sunshine hours; N is the potential sunshine hours; and Ra is the extra-terrestrial solar radiation (MJ m-2 d-1). The values of N and Ra can be obtained from standard tables (Allen et al. Citation1998) for different latitudes and months. Further, R nl is the net outgoing longwave radiation (MJm-2 d-1); σ is the Stefan-Boltzmann constant (4.903 × 10-9 MJK-4 m-2 d-1); T max, K and T max, K are the maximum and minimum absolute temperature during a 24-h period (K = °C + 273.16); and as and bs are regression constants.
Spatial interpolation
There are many algorithms available to interpolate spatially-distributed hydrological and climatic data (McVicar et al. Citation2007). In this study, kriging with spherical variogram (kriging method), inverse distance weighted (IDW) and spline method were selected to test annual ETref. These methods have been frequently used to interpolate data in hydrology (Yao and Creed Citation2005, Daly Citation2006). According to the spatial cross-validation, the correlation coefficients between the interpolated and original values of ETref were 0.96, 0.74 and 0.93 for the kriging method, IDW and spline method, respectively. So the kriging method was selected in the study for interpolating the mean seasonal and mean annual values at 89 stations at 30-km resolution.
The kriging method is a technique of making optimal, unbiased estimations of regionalized variables at unsampled locations, and has been preferred by researchers particularly when applied to data obtained from a low-density and irregularly spaced network (Goovaerts Citation2000, Campling et al. Citation2001, Xu et al. Citation2006a, Basistha et al. Citation2008). The description of kriging theory and its application can be found in Journel and Huijbregts (Citation1978). The general equation of kriging estimator is:
where x denotes the set of spatial coordinates (x, y); and λi is the weight associated with the ith observation point, xi .
Temporal trends
In order to test the significance of the trend existing in ETref series, both the non-parametric M-K method and the linear regression model were performed. The non-parametric M-K method is used to detect abrupt climate change against trend (Mann Citation1945, Kendall Citation1948), and has been widely used and tested as an effective method to evaluate the presence of a statistically significant trend in hydrological and climatological time series (e.g. Douglas et al. Citation2000, Birsan et al. Citation2005). The linear regression model is the most common technique of statistical diagnosis and forecasting in modern climate, and has been used to detect patterns in meteorological variables (Hameed et al. Citation1997, Wei Citation1999, da Silva Citation2004). In our study, the linear trends in the series of ETref were calculated by the least squares regression, and the estimated slopes were tested against the hypothesis of null slope by means of a two-tailed T test at the 95% confidence level (Serrano et al. Citation1999). The M-K method is expressed as described below.
Under the null hypothesis of no-trend, the time series of a variable has no change; the time series could be regarded as x 1, x 2, … , xn . For each term, mi is computed as the number of xi in the series whose values exceeded xj (1 ≤ j ≤ i). The test statistic is calculated as follows:
Assuming that the series is random and independent, the expected value E(dk
) and variance of may be shown as follows:
We define:
The terms of u(dk ) (1 ≤ k ≤ n) constitute curve C1.
The null hypothesis of no trend will be rejected at a confidence level of α if the standard normal probability Pr(|u| < |u(dk )|) > α.
Applying the method to the inverse series, is computed as the number of xi
in the series whose values exceeds xj
(i ≤ j≤ N). When
and
we could obtain the series of
as follows:
The terms of (1 ≤ k ≤ n) constitute curve C2.
If C1 exceeds the confidence line ±1.96 (P = 0.05), it means that there is a significant upward or downward trend in the series. Based on this, if the intersection point of curves C1 and C2 is between the two confidence lines, we can consider that abrupt climate change takes place at that point (Fu and Wang Citation1992).
Sensitivity analysis
As a synthetic climatic variable, ETref, reflects the combined effects of many climatological factors. Sensitivity analysis is important to understand the relative importance of climatic variables to the variation of ETref (Xu et al. Citation2006a). A simple but practical way of sensitivity analysis is to calculate and plot the relative changes of an input variable against the resultant relative change of the output variable as a curve (Goyal Citation2004, Gong et al. Citation2006, Xu et al. Citation2006a). Then the corresponding relative change of the outcome can easily be read from the sensitivity curve for a certain relative change of the variable.
In this study, seven scenarios are generated for each meteorological variable using Equationequation (11)(11):
where X is the meteorological variable and t is the time in day.
RESULTS AND DISCUSSION
Spatial distribution of seasonal and annual reference evapotranspiration
The spatial distributions of seasonal and annual ETref are plotted in , which reflects the combined effects of meteorological variables in different regions. The results are as follows:
a. | ETref, influenced by the seasonal variations of meteorological variables, demonstrated different spatial patterns in all seasons and the year. The lowest seasonal and annual values for ETref were found in the upper region of the YRB. The relatively low air temperature and wind speed caused low ETref values in the upper region during the whole year. | ||||
b. | The distributions of ETref in spring, summer and autumn presented similar patterns in the whole basin. The relatively high values of ETref extend in the northeast portion of the middle region. As seen from , the relatively high air temperature, wind speed, sunshine hours and low relative humidity were the main causes for high ETref values in the middle region in spring, summer and autumn. | ||||
c. | In winter, the centre of high values of ETref moved to the lower region, while that of low ETref moved to the northern part of the middle region, compared to the other seasons. As air temperature presented similar temporal variations, it contributed little to the difference in spatial pattern in the three regions during different seasons. The higher wind speed and relative humidity mainly contributed to higher ETref in the lower region and lower ETref in the middle region, respectively, in the winter. |
The spatial distribution of ETref, combined with land cover and soil water content, has been used to estimate the ecological water requirement (Yang et al. Citation2005), which can provide more valuable information for water resources planning and management at the basin scale (Xu et al. Citation2006a, Liu et al. Citation2010).
Trend analysis of annual reference evapotranspiration
The M-K method with a nominal rejection rate of 5% was applied to 89 annual ETref series. The Yangcheng station (113°53′E, 35°19′N) was selected as an example to demonstrate the abrupt change occurring in the time series of ETref. It revealed that the downward abrupt change occurred in 1986 ().
Fig. 4 The abrupt change tested by the Mann-Kendall method for annual ETref series of Yangcheng station for 1961–2006. Here, as the line C1 is over the confidence line (P = 0.05), the cross-over point of C1 and C2 is the start point of abrupt change in this series.
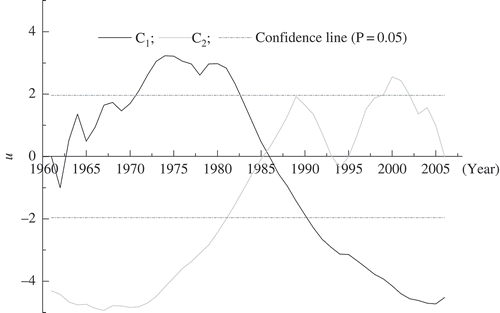
Significant trends The significant trends for different stations are shown in : 79 of the 89 stations showed a significant trend (P = 0.05). Among these 79 stations with significant trend, 51 showed an upward trend, and 28 a downward trend. The distribution of temporal trend for ETref indicated that:
a. | Among 24 stations in the upper region, six showed a downward trend, 12 exhibited an upward trend, and the other six stations showed no significant trend (60% of all stations with no significant trend in the basin). | ||||
b. | Thirty-one (31) of 41 stations (61%) exhibited a significant upward trend in the middle region, only seven stations showed a downward trend, and three stations presented no trend | ||||
c. | In the lower region, 15 of 24 stations displayed a downward trend, only eight stations and one station showed an upward trend and no trend, respectively. |
Time and frequency of abrupt changes The time and frequency of the abrupt changes in ETref tested by the M-K method are shown in . The results show that:
Fig. 6 The time and frequency of the abrupt changes in ETref detected by the M-K method in the YRB in the 1961–2006 period, with the years when abrupt changes occurred (where two abrupt changes were detected at one station, the years are in brackets).
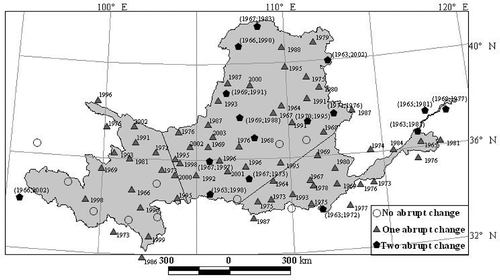
a. | Of 79 stations, two abrupt changes occurred in 16 stations and one abrupt change in the other stations. The timing of the abrupt changes in ETref series was between 1963 and 2003. | ||||
b. | There were 95 abrupt changes in the 79 stations with significant trend: the number of these occurring during 1961–1970, 1971–1980, 1981–1990, 1991–2000 and 2001–2006 were 26, 24, 14, 25 and 6, respectively. | ||||
c. | Four of 24 (16.67%) and 11 of 41 (26.83%) stations were detected with two abrupt changes in the lower and middle regions, respectively, while there was only one station with two abrupt changes in the upper region. | ||||
d. | Between 1961 and 2006, the abrupt changes in the lower and middle regions were relatively earlier than those in the upper region of the YRB. |
The reasons for temporal variation of ETref result mainly from the complicated watershed characteristics which influence the response of ETref to the climate changes in the different regions of the YRB. From east to west, the mean elevation in the lower, middle and upper regions is 677, 1403 and 3693 m a.s.l., respectively, which may influence the variations in regional climate; furthermore, the variation in the vegetation, presenting obvious regional characteristics, has an important influence on the regional climate (Li and Yang Citation2004). In addition, the Asian monsoon influences the meteorological conditions in different regions of the YRB (Ye and Gao Citation1979, Cui et al. Citation2006), and the Tibetan Plateau, in particular, plays an important role in forming and inducing the variations in regional weather and climate in the YRB (Liu and Yin Citation2002). Xu and Ma (Citation2009) noted that, influenced by the global climate changes, the East Asian summer monsoon intensity had changed significantly in the past 50 years, leading to a response by the water cycle of the YRB. All these factors can result in regional characteristics in the meteorological variables, e.g. decreasing trends from east to west presented by precipitation in the YRB (Liu et al. Citation2008).
Fitted data to linear regression model
shows the results of the least-squares analysis. Thirty-eight stations showed a negative trend of ETref, with an average annual increase of –1.7 mm year-2, while 51 stations had a positive trend, with an average annual change of 1.2 mm year-2. About 47% of stations (42 of 89) showed significant trend at the 95% confidence level on a basin scale, and most trends revealed by the linear regression model were consistent with those obtained by the M-K method. As influenced by meteorological variables, the trends in ETref revealed by the linear regression model also showed different characteristics in different regions:
Fig. 7 Trends (mm year-2) for the annual ETref from 1961 to 2006 in the YRB, China. Circles placed around the dots denote significant trend (0.05 level).
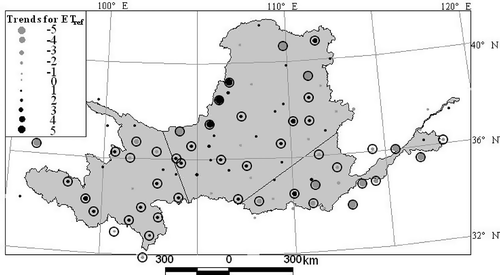
a. | In the upper region, the average trend of ETref was –1.7 and 1.0 mm year-2 for the seven negative and 17 positive trend stations, respectively. Only one station displayed negative trend among the eight significant trend stations, but its abrupt change was upward. One among the other seven stations with significant positive trends showed an abrupt downward change. | ||||
b. | The negative and positive trends were detected in 12 and 29 stations, respectively, in the middle region, with an average slope of –1.38 and 1.52 mm year-2 average change, which are larger than those in the upper region. Fourteen of the 23 significant trend stations displayed positive trend, with upward trend detected by the M-K method. Among the nine negative significant trend stations, one station showed no abrupt changes and one showed two upward changes (in 1963 and 1998). | ||||
c. | In the lower region, about 79% of stations (19 of 24) showed negative trends, with the average change of –1.9 mm year-2, and the remaining five stations displayed positive trend with an average increase of 0.9 mm year-2. Seven stations showed significant negative trend and four showed significant positive trend. | ||||
d. | The average slope for ETref is 0.19, 0.67 and –1.34 mm year-2 during 1961–2006 in the upper, middle and lower regions of the YRB, respectively. |
Significant increasing trends (P = 0.05) in air temperature have been reported by Liu et al. (Citation2010). The causes for the decreasing trends in pan evaporation and ETref with the increases in air temperature have been explored by several researchers (e.g. Roderick and Farquhar Citation2002, Citation2004, Roderick et al. Citation2007, Citation2009a, Citationb). In China, some studies also have been performed to reveal this problem (e.g. Xu et al. Citation2006b, Cong and Yang Citation2008, Liu et al. Citation2010). Cong and Yang (Citation2008) investigated causes for the changes in pan evaporation from 1956 to 2005; their results showed that pan evaporation decrease is caused by decreasing radiation and wind speed before 1985 and pan evaporation increase is caused by decreasing vapour pressure deficit due to strong warming after 1986. According to Liu et al. (Citation2010), the decreasing trends in radiation and wind speed caused ETref to decrease, and significant decreasing trends (P = 0.05) in wind speed is the main cause for decreasing trends in ETref in the lower region of the YRB.
Sensitivity analysis of reference evapotranspiration
The relative change in annual ETref due to relative change in meteorological variables is presented in . This shows that the two most sensitive variables are relative humidity and air temperature, followed by sunshine hours and wind speed. In response to the change in temperature by ±30%, ETref varies between ±13%. The ETref is less sensitive to decreasing temperature in comparison to increasing temperature, e.g. ETref reduces by –12.72% when air temperature decreases by –30%, while it increases by 13.01% with an increase of 30% in air temperature. This result is consistent with that addressed by Goyal (Citation2004). The ETref showed an increasing trend with the decrease in relative humidity (e.g. ETref increases by 14.52% with a decrease of 30% in relative humidity). Wind speed and sunshine hours affect ETref in a similar pattern, despite a little higher magnitude for sunshine hours. The ETref is more sensitive to the decreased wind speed and increasing sunshine hours in comparison to increasing wind speed and decreasing sunshine hours. The ETref increases by 4.09% and 7.02% with an increase of 30% in wind speed and sunshine hours; while it reduces by 4.62% and 6.71% with a decrease of 30% in wind speed and sunshine hours, respectively. All of these results indicate that, influenced by radiometric and aerodynamic variables, the changes of ETref with increasing or decreasing different meteorological variables presents a nonlinear relationship (McVicar et al. Citation2007, Liu et al. Citation2010). Furthermore, the contribution of changes in the meteorological variables to the change in ETref in the basin should be considered with the trends of ETref and meteorological variables. This will be explored in the future to obtain more useful information to regulate water allocation and management for the YRB.
CONCLUSIONS
The ETref was calculated by the Penman-Monteith (P-M) method using monthly air temperature, wind speed, relative humidity and sunshine hours at 89 standard meteorological stations from 1961 to 2006 in the YRB and then regionally mapped. Using the Mann-Kendall (M-K) method and linear regression model, temporal trends and the spatial distribution of the ETref were explored, and then the sensitivity of ETref to the main meteorological variables was investigated. The following conclusions can be drawn:
1. | As affected by seasonal variations in the meteorological variables, the spatial distribution of ETref presented seasonal variations. Similar spatial distribution patterns of ETref were found in the spring, summer and autumn. The ETref, influenced by the changes in meteorological variables, presented different spatial patterns in winter: the centres of the high and low values of ETref moved to the lower region and the northern part of the middle region of the basin, respectively. | ||||
2. | The results of the M-K method indicated that 79 stations experienced abrupt changes in the last 46 years, 51 with upward trends and 28 with downward trends. The distribution of temporal trend in ETref also displayed spatial variation in different regions of the basin. The time and frequency of the abrupt change in ETref demonstrated that stations featured two abrupt changes mainly in the middle and lower regions of the YRB, and the abrupt changes in the lower and middle regions were relatively earlier than those in the upper region of the YRB. The trends of ETref revealed by the linear regression model exhibited almost the same spatial distribution pattern as those detected by the M-K method, and 42 among the 89 stations got through the T test at the 95%. confidence level | ||||
3. | Sensitivity analysis showed that the most sensitive variable for ETref in the YRB is relative humidity, followed by air temperature, sunshine hours and wind speed. The ETref showed an increasing trend with the decrease in relative humidity and vice versa, and it was less sensitive to the decreasing temperature in comparison to increasing temperature. Wind speed and sunshine hours affect ETref in a similar pattern, despite a slightly higher magnitude for sunshine hours. The ETref was more sensitive to decreasing wind speed and increasing sunshine hours in comparison to increasing wind speed and decreasing sunshine hours. |
Acknowledgments
This research is supported by the National Science Foundation for Distinguished Young Scholars of China (no. 50625926), the Major State Basic Research Development Programme of China (973 Program) (no. 2010CB951104 and no. 2006CB403303) and the National Natural Science Foundation of China (no. 40701189). The authors are also grateful to the National Meteorological Information Center, China Meteorological Administration for providing the meteorological data. The authors thank two anonymous reviewers and the editors for their detailed and constructive comments.
REFERENCES
- Allen , R.G. 1998 . Crop evapotranspiration—guidelines for computing crop water requirements , Rome : FAO, FAO Irrigation and Drainage Paper 56 .
- Basistha , A. , Arya , D.S. and Goel , N.K. 2008 . Spatial distribution of rainfall in Indian Himalayas—a case study of Uttarakhand region . Water Resources Management , 22 : 1325 – 1346 .
- Birsan , M.V. 2005 . Streamflow trends in Switzerland . Journal of Hydrology , 314 : 312 – 329 .
- Campling , P. , Gobin , A. and Feyen , J. 2001 . Temporal and spatial rainfall analysis across a humid tropical catchment . Hydrological Processes , 15 : 359 – 375 .
- Cannarozzo , M. , Noto , L.V. and Viola , F. 2006 . Spatial distribution of rainfall trends in Sicily (1921–2000) . Physics and Chemistry of the Earth , 31 : 1201 – 1211 .
- Chen , S.B. , Liu , Y.F. and Thomas , A. 2006 . Climatic change on the Tibetan Plateau: potential evapotranspiration trends from 1961–2000 . Climatic Change , 76 : 291 – 319 .
- Cohen , S. , Ianetz , A. and Stanhill , G. 2002 . Evaporative climate changes at Bet Dagan, Israel, 1964–1998 . Agricultural and Forest Meteorology , 111 : 83 – 91 .
- Cong , Z.T. and Yang , D. 2008 . Does evapotranspiration paradox exist in China? . Hydrology and Earth System Sciences Discussions , 5 : 2111 – 2131 .
- Cong , Z.T. 2009 . Hydrological trend analysis in the Yellow river basin using a distributed hydrological model . Water Resources Research , 45 : W00A13 doi: doi:10.1029/2008WR006852
- Cui , X.F. 2006 . Climate impacts of anthropogenic land use changes on the Tibetan Plateau . Global Planetary Change , 54 : 33 – 56 .
- Daly , C. 2006 . Guidelines for assessing the suitability of spatial climate data sets . International Journal of Climatology , 26 : 707 – 721 .
- da Silva , V.P.R. 2004 . On climate variability in Northeast of Brazil . Journal of Arid Environments , 58 : 575 – 596 .
- Douglas , E.M. , Vogel , R.M. and Kroll , C. N. 2000 . Trends in floods and low flows in the United States: impact of spatial correlation . Journal of Hydrology , 240 : 90 – 105 .
- Fu , C.B. and Wang , Q. 1992 . The definition and detection of the abrupt climatic change . Scientia Atmospherica Sinica , 16 : 482 – 493 . in Chinese
- Gong , L. B. 2006 . Sensitivity of the Penman-Monteith reference evapotranspiration to key climatic variables in the Changjiang (Yangtze river) basin . Journal of Hydrology , 329 : 620 – 629 .
- Goovaerts , P. 2000 . Geostatistical approaches for incorporating elevation into the spatial interpolation of rainfall . Journal of Hydrology , 228 : 113 – 129 .
- Goyal , R.K. 2004 . Sensitivity of evapotranspiration to global warming: a case study of arid zone of Rajasthan (India) . Agricultural Water Management , 69 : 1 – 11 .
- Hameed , T. 1997 . Method for trend detection in climatological variables . Journal of Hydrologic Engineering , 2 : 154 – 160 .
- Jabloun , M. and Sahli , A. 2008 . Evaluation of FAO-56 methodology for estimating reference evapotranspiration using limited climatic data application to Tunisia . Agricultural Water Management , 95 : 707 – 715 .
- Jia , Y.W. 2006 . Development of the WEP-L distributed hydrological model and dynamic assessment of water resources in the Yellow River Bain . Journal of Hydrology , 331 : 606 – 629 .
- Journel , A.G. and Huijbregts , C.J. 1978 . Mining geostatistics , London : Academic Press .
- Kannan , N. 2007 . Sensitivity analysis and identification of the best evapotranspiration and runoff options for hydrological modeling in SWAT-2000 . Journal of Hydrology , 332 : 456 – 466 .
- Kendall , M.G. 1948 . Rank correlation methods , New York : Hafner .
- Li , G.Y. 2003 . Ponderation and practice of the Yellow river control , Zhengzhou : Yellow River Conservancy Press .
- Liu , C.M. 2004 . Study of some problems in water cycle changes of the Yellow River Basin . Advances in Water Science , 15 : 608 – 614 . in Chinese
- Liu , C.M. and Cheng , L. 2000 . Analysis on runoff series with special reference to drying up courses of lower Huanghe river . Acta Geographica Sinica , 55 : 257 – 265 . in Chinese
- Li , C.H. and Yang , Z.F. 2004 . Spatial-temporal changes of NDVI and their relations with precipitation and runoff in the Yellow River Basin . Geographical Research , 23 : 753 – 759 . in Chinese
- Liu , Q. , Yang , Z.F. and Cui , B.S. 2008 . Spatial and temporal variability of annual precipitation during 1961–2006 in Yellow River Basin. China . Journal of Hydrology , 361 : 330 – 338 .
- Liu , Q. 2010 . The temporal trends of reference evapotranspiration and its sensitivity to key meteorological variables in the Yellow River Basin, China . Hydrological Processes , 24 : 2171 – 2181 .
- Liu , X.D. and Yin , Z.Y. 2002 . Sensitivity of East Asia monsoon climate to the uplift of Tibetan Plateau . Palaeogeography, Palaeoclimatology, Palaeoecology , 183 : 223 – 245 .
- López-Urrea , R. 2006 . An evaluation of two hourly reference evapotranspiration equations for semiarid conditions . Agricultural Water Management , 86 : 277 – 282 .
- Mann , H.B. 1945 . Non-parametric test against trend . Econometrika , 13 : 245 – 259 .
- McVicar , T.R. 2007 . Spatially distributing monthly reference evapotranspiration and pan evaporation considering topographic influences . Journal of Hydrology , 338 : 196 – 220 .
- McVicar , T.R. 2002 . Monitoring regional agricultural water use efficiency for Hebei Province on the North China Plain . Australian Journal of Agricultural Research , 53 : 55 – 76 .
- Oguntunde , P.G. 2006 . Hydroclimatology of Volta River Basin in West Africa: trends and variability from 1901 to 2002 . Physics and Chemistry of the Earth , 31 : 1180 – 1188 .
- Porporato , A. 2002 . Ecohydrology of water-controlled ecosystems . Advances in Water Resources , 25 : 1335 – 1348 .
- Porporato , A. and Rodriguez-Iturbe , I. 2002 . Ecohydrology—a challenging multidisciplinary research perspective . Hydrological Sciences Journal , 47 : 811 – 821 .
- Roderick , M.L. and Farquhar , G.D. 2002 . The cause of decreased pan evaporation over the past 50 years . Science , 298 : 1410 – 1411 .
- Roderick , M.L. and Farquhar , G.D. 2004 . Changes in Australian pan evaporation from 1970 to 2002 . International Journal of Climatology , 24 : 1077 – 1090 .
- Roderick , M.L. , Hobbins , M.T. and Farquhar , G.D. 2009a . Pan evaporation trends and the terrestrial water balance. I. Principles and observations . Geography Compass , 3 : 746 – 760 .
- Roderick , M.L. , Hobbins , M.T. and Farquhar , G.D. 2009b . Pan evaporation trends and the terrestrial water balance. II. Energy balance and interpretation . Geography Compass , 3 : 761 – 780 .
- Roderick , M.L. 2007 . On the attribution of changing pan evaporation . Geophysical Research Letters , 34 : L17403 – 031166 . doi: doi:10.1029/2007GL
- Rodriguez-Iturbe , I. 2000 . Ecohydrology: a hydrologic perspective of climate-soil-vegetation dynamics . Water Resources Research , 36 : 3 – 9 .
- Rodriguez-Iturbe , I. and Porporato , A. 2005 . Ecohydrology of water-controlled ecosystems: soil moisture and plant dynamics , Cambridge : Cambridge University Press .
- Rodriguez-Iturbe , I. 2001 . Plants in water-controlled ecosystems: active role in hydrologic processes and response to water stress I. Scope and general outline . Advances in Water Resources , 24 : 695 – 705 .
- Serrano , A. , Mateos , V.L. and García , J.A. 1999 . Trend analysis of monthly precipitation over the Iberian Peninsula for the period 1921–1995 . Physics and Chemistry of the Earth (B) , 24 : 85 – 90 .
- Wei , F. Y. 1999 . The techniques of statistical diagnosis and forecasting in modern climate , Beijing : Meteorological Press . in Chinese
- Xu , C.Y. 2006b . Decreasing reference evapotranspiration in a warming climate—a case of Changjiang (Yangtze) River Catchment during 1970–2000 . Advances in Atmospheric Sciences , 23 : 513 – 520 .
- Xu , C.Y. 2006a . Analysis of spatial distribution and temporal trend of reference evapotranspiration and pan evaporation in Changjiang (Yangtze River) catchment . Journal of Hydrology , 327 : 81 – 93 .
- Xu , Z.X. and Li , J.Y. 2003 . A distributed approach for estimating basin evapotranspiration: comparison of the combination equation and the complementary relationship approaches . Hydrological Processes , 17 : 1509 – 1523 .
- Xu , J.X. and Ma , Y.X. 2009 . Response of the hydrological regime of the Yellow River to the changing monsoon intensity and human activity . Hydrological Sciences Journal , 54 : 90 – 100 .
- Xu , C.Y. and Singh , V.P. 2002 . Cross-comparison of empirical equations for calculating potential evapotranspiration with data from Switzerland . Water Resources Management , 16 : 197 – 219 .
- Yang , Z.F. , Cui , B.S. and Liu , J.L. 2005 . Estimation methods of eco-environmental water requirements: case study . Science in China (D): Earth Sciences , 48 : 1280 – 1292 .
- Yang , W.D. 2004 . Analysis of water resources variability in the Yellow River of China during the last half century using historical data . Water Resources Research , 40 : W06502 doi: doi:10.1029/2003WR002763.
- Yao , H. and Creed , I. 2005 . Determining spatially distributed annual water balances for ungauged locations on Shikoku Island, Japan: a comparison of two interpolators . Hydrological Sciences Journal , 50 : 245 – 263 .
- Yao , H. , Hashino , M. and Yuan , Z. 2005 . “ Simulating effects of climate change on evapotranspiration of major crops in China ” . In Regional hydrological impacts of climatic change—impacts assessment and decision making , Edited by: Franks , S. Vol. 295 , 189 – 197 . Wallingford : IAHS Press, IAHS Publication .
- Ye , D. and Gao , Y. 1979 . The meteorology of the Qinhai-Xizang (Tibet) Plateau , Beijing : Sciences Press . in Chinese
- Yin , Y.H. 2008 . Radiation calibration of FAO56 Penman-Monteith model to estimate reference crop evapotranspiration in China . Agricultural Water Management , 95 : 77 – 84 .
- Yu , L. 2002 . The Huanghe (Yellow) River: a review of its development, characteristics, and future management issues . Continental Shelf Research , 22 : 389 – 403 .
- Zalewski , M. 2000 . Ecohydrology—the scientific background to use ecosystem properties as management tools toward sustainability of water resources . Ecological engineering , 16 : 1 – 8 .
- Zhan , C.S. 2008 . An integrated hydrological and meteorological approach for the simulation of terrestrial evapotranspiration . Hydrological Sciences Journal , 53 : 1151 – 1164 .
- Zhou , M. C. 2009 . Evapotranspiration in the Mekong and Yellow River basins . Hydrological Sciences Journal , 54 : 623 – 638 .