Abstract
Time series of soil moisture-related parameters provide important insights into the functioning of soil water systems. Analysis of patterns within such time series has been used in several studies. The objective of this work was to compare patterns in observed and simulated soil moisture contents to understand whether modelling leads to a substantial loss of information or complexity. The time series were observed at four plots in sandy soils within the USDA-ARS OPE3 experimental watershed, for a year; precipitation and evapotranspiration (ET) were measured and estimated, respectively, and used for soil water flow simulation with the HYDRUS-1D software. The information content measures are the metric entropy and the mean information gain, and complexity measures are the fluctuation complexity and the effective measure complexity. These measures were computed based on the binary encoding of soil moisture time series, and used probabilities of patterns, i.e. probabilities of joint or sequential appearance of symbol sequences. The information content of daily soil moisture time series was much smaller than that of rainfall data, and had higher complexity, indicating that soil worked essentially as an information filter. Information content and complexity decreased and increased with depth, respectively, demonstrating the increase in the information filtering action of soil. The information measures of simulated soil moisture content were close to those of the measurements, indicating the successful simulation of patterns in the data. The spatial variability of the information measures for simulated soil moisture content at all depths was less pronounced than the one of measured time series. Compared with precipitation and estimated ET, soil moisture time series had more structure and less randomness in this work. The information measures can provide useful complementary knowledge about model performance and patterns in observation and modelling results.
Citation Pan, F., Pachepsky, Y. A., Guber, A. K., & Hill, R. L. (2011) Information and complexity measures applied to observed and simulated soil moisture time series. Hydrol. Sci. J. 56(6), 1027–1039.
Résumé
Les séries chronologiques de paramètres liés à l'humidité du sol fournissent des indications importantes sur le fonctionnement des systèmes d'eau dans le sol. L'analyse des structures au sein de telles séries chronologiques a été utilisée dans plusieurs études. L'objectif de ce travail était de comparer les structures observées et simulées dans les teneurs en eau du sol afin de comprendre si la modélisation conduit à une perte substantielle d'information ou de complexité. Les séries chronologiques ont été observées pendant une année sur quatre parcelles ayant des sols sableux dans le bassin versant expérimental OPE3 de l'USDA-ARS. Les précipitations et les évapotranspirations (ET) ont été mesurées et estimées, respectivement, et utilisées pour la simulation des écoulements d'eau dans le sol avec le logiciel HYDRUS-1D. Les mesures d'information sont l'entropie métrique et le gain d'information moyen, et les mesures de complexité sont la complexité de fluctuation et la complexité de mesure efficace. Ces mesures ont été calculés sur la base d'un codage binaire des séries temporelles d'humidité du sol, et des probabilités de structures utilisées, c'est-à-dire des probabilités d'apparition conjointe ou séquentielle de séquences de symboles. Le contenu d'information des séries quotidiennes d'humidité était beaucoup plus faible que celui des données de précipitations, et avait une complexité plus grande, ce qui indique que le sol agit essentiellement comme un filtre d'information. Le contenu d'information se réduit et la complexité augmente avec la profondeur, ce qui démontre l'augmentation du filtrage de l'information par le sol. Les mesures d'information de la teneur en eau du sol simulée étaient proches de celles des mesures, indiquant la bonne simulation des structures dans les données. La variabilité spatiale des mesures d'information pour la teneur en eau du sol simulée à toutes les profondeurs était moins prononcée que celle des séries mesurées. En comparaison aux précipitations et ET estimées, les séries d'humidité du sol ont montré plus de structure et moins de caractère aléatoire dans ce travail. Les mesures d'information peuvent fournir des connaissances utiles et complémentaires sur la performance du modèle et sur les structures dans les observations et les résultats de modélisation.
1 INTRODUCTION
The flow and retention of water in field soils are notoriously complex phenomena. Soil heterogeneity makes this complexity easy to perceive but difficult to characterize in models. As a result, soil water flow and retention models include strong simplifying assumptions. The extent to which these assumptions affect the correspondence between measured and simulated water contents is usually defined by analysing residuals on a set of measurement time series. This comparison characterizes the ability of the model to reproduce temporal trends.
The availability of sensors capable of measuring soil moisture-related soil parameters with a high temporal resolution has led to the documentation of soil moisture fluctuations at various frequencies to the same extent as the availability of high frequency rainfall measurements led to the discovery of fluctuations in rainfall intensity. Temporal fluctuations of soil moisture are critical for characterizing the dynamics of hydrological processes (Kim and Entekhabi Citation1998, Oevelen Citation1998, Haider et al. Citation2004, Casper et al. Citation2007, Al-Hamdan and Cruise Citation2010, Zehe et al. Citation2010). These fluctuations were viewed as the manifestation of intrinsic properties of the soil-water system, and various approaches were applied to soil water content time series to gain knowledge about this system. The mechanistic interpretation of the fluctuations in some measurements was successful. Or and Ghezzehei (Citation2000) showed that the dripping rates in caves can provide information about the fracture surface properties. Or and Moebius (Citation2009) analysed acoustic emission measurements resulting from water front displacement in Hele-Shaw cells packed with glass beads of different sizes, and showed marked differences between the acoustically-rich drainage process relative to low activity during imbibition under similar conditions. These authors were able to link the acoustic emission activity and pressure jumps in the liquid phase with amplitudes of air entry pressures and the width of pore throats.
Another line of research demonstrated that the fluctuations in soil moisture content can provide insight into scaling properties in soil-water systems. Delworth and Manabe (Citation1989) interpreted soil moisture temporal variation as a first-order Markov process with additive white noise. One of the parameters of this model was interpreted as the scale of temporal autocorrelation. Entin et al. (Citation2000) demonstrated such scaling of soil moisture measurements in different parts of the world. Nie et al. (Citation2008) analysed the large national data set from China and found this scale to decrease with depth of the measurements. Usowicz (Citation1999) demonstrated fractal scaling in time series of soil moisture measured under several crop types and noted the decrease in fractal dimension with depth. Anomaly analysis has provided yet another useful approach to analysing soil moisture fluctuations. Kuenzer et al. (Citation2008) processed the global data set of soil moisture time series with respect to global anomaly derivation; an anomaly in the soil moisture data set depicts “wetter than normal” or “drier than normal” conditions with respect to the long-term mean. Findings indicated that extreme events such as confirmed floods and droughts were clearly represented in the data set. Anomaly analyses in months prior to known extreme events indicated that the time series held a strong potential for flood early warning activities.
Pattern analysis provides yet another approach to extracting knowledge about hydrological systems from fluctuating time series. Analysis of occurrences of values larger or smaller than normal ones was suggested as the source of the fluctuation measures based on information theory. For example, Lange (Citation1999a) suggested replacing the original time series with symbolic strings using binary symbols, where values below (respectively, above) the median were assigned the symbol 0 (or 1). Then the patterns within those symbol series were characterized using Shannon entropy and other measures from information theory. Lange (Citation1999a) has shown that catchments work as filters of the information contained in precipitation time series. His analysis of data on 30 catchments showed that runoff had smaller information content and larger complexity than precipitation, and that the land cover controlled this information filtering. The pattern characterization proposed by Lange (Citation1999a) was applied to time series of soil moisture contents by Pachepsky et al. (Citation2006) to compare the performance of different soil water flow models, by Engelhardt et al. (Citation2009) to compare hydrological time series in mountain forest catchments, and by Wang et al. (Citation2009) for characterizing heterogeneous water flow and solute transport in soils.
One application of the analysis of fluctuations in time series is the evaluation of model ability to reproduce the information content and complexity in measurements. An information theory-based method for such comparisons was proposed and applied to precipitation data by Moss (Citation1992). Soil moisture modelling results have not been analysed toward that end. The objective of this work was to apply the information content and complexity measures to compare HYDRUS-1D simulations and measurements of soil water content time series to see whether substantial differences can be found between simulated and measured time series of soil moisture.
2 MATERIALS AND METHODS
2.1 Study site and experimental data
The study site was the USDA-ARS OPE3 (Optimizing Production Inputs for Economic and Environmental Enhancement) experimental watershed (area 22 ha) in Beltsville, Maryland (). The site is located in the Atlantic Coastal Plain, with a climate characterized as humid, temperate and semi-continental. The average annual precipitation rate is 1055 mm (1949–1993). The shallow groundwater is usually found at depths between 0.5 m and 3 m. The vadose zone of the OPE3 site is formed of fluvial deposits. A typical soil profile includes a coarse sandy loam surface horizon (0–25cm), a sandy clay loam horizon (25–100cm), and a loam horizon below 140 cm, with loamy sand and fine textured clay loam lenses between 120 and 250 cm (Guber et al. Citation2010). Multisensor capacitance probes (MCP, EnviroSCAN, SENTEK Pty Ltd, South Australia) were installed in the four plots (each 1 m2 and 10 m apart) of a square 10 × 10 m experimental site in field B of the OPE3 site () to monitor soil moisture content at depths from 0.1 to 1.0 m below the land surface with 0.1 m interval and a 15-min measurement frequency. The field correction was developed and applied to the MCP readings (Guber et al. Citation2010). The meteorological data including rainfall, relative humidity, wind speed and direction were measured as 30-min averages at a meteorological station located about 80 m from the experimental site. The daily evapotranspiration (ET) rates were computed from mean wind speed, relative humidity, net radiation, air humidity and temperature using the Penman-Monteith method as documented by FAO (Allen et al. Citation1998). Groundwater depth was also monitored at the four plots with a time interval of 30 min using a Cera-Diver (Van Essen Instruments, Delft, The Netherlands). Observations for the year 2007 were used in this work.
2.2 Information content and complexity measures for soil moisture time series
To apply information theory-based measures, time series of hydrological variables (soil moisture, rainfall and ET in this study) were replaced with symbolic strings as suggested by Lange (Citation1999a) and Wolf (Citation1999). The symbol 1 was assigned to measurements that exceeded the median values of the variables and the symbol 0 was assigned to measurements that were below or at the median values. Simulated time series of hydrological variables were encoded analogously. The word of length L was defined as a group of L consecutive symbols, and the string of symbols had 2 L possible words. If the word length was two, then possible words were 00, 01, 10, and 11. Each word represented the state of the system. The transition from one state to another was defined as the change in the words starting from two consecutive observations. For example, if the string was 00110 and the word length was two, then the shift from the first word 00 to the second word 01 represented the transition from the 00 state to the 01 state, the shift from the second word 01 to the third word 11 represented the transition from the 01 state to 11 state, etc. Three sets of empirical probabilities were defined: (a) state probabilities pL ,i for the word i to appear in the symbolic string, i = 1, 2, …, 2 L ; (b) transition probabilities pL ,ij for the sequence of words “i” and “j” to appear, i = 1, 2, …, 2 L , j = 1, 2, …, 2 L ; and (c) pL ,i→j the conditional probability of the occurrence of the jth word after the ith word, i = 1, 2, …, 2 L , j = 1, 2, …, 2 L (Wolf Citation1999). The subscript L indicates that words of length L were considered.
The information content was quantified with the metric entropy and the mean information gain (as these terms are defined in Wolf Citation1999). The Shannon entropy (Shannon Citation1948) H(L) for words of length L was defined as a measure of information in the time series after it has been encoded with symbols:
Shannon's entropy measures the information contained in a message as opposed to the portion of the message that is determined or predictable (e.g. Chang et al. Citation2009). The metric entropy, Hu , is essentially the Shannon's entropy divided by the word length, and this normalization results in the value of information being independent of the word length. The metric entropy (also called the Kolmogorov entropy) represents the extent of the disorder in the sequence of symbols. The entropy vanishes for constant sequences, increases monotonically when the disorder increases, and reaches its maximum of one for uniformly distributed random sequences of words with complete randomness.
The mean information gain, HG , as yet another measure of the information content, quantifies the additional information that can be gained on average for the whole symbol sequence from knowing the next symbol. It is defined as:
The mean information gain (also called the conditional entropy) includes the probabilities of state changes in a time series. The larger values of the mean information gain indicate greater possibility of state changes from one to another, and higher randomness in, or less predictability of a time series.
The complexity in this work reflects the presence of patterns, or internal structure in a time series. Two measures of such complexity—the fluctuation complexity and effective measure complexity—were used in this study to quantify the internal structure in symbolic strings. The fluctuation complexity is the mean square deviation of the net information gain (i.e. the differences between information gain and loss) defined as:
The more the net information gain is fluctuating in the investigated string, the more complex is the string in the sense of the fluctuation complexity (Bates and Shepard Citation1993). The fluctuation complexity characterizes fluctuations in the system transitions from one state to another.
The effective measure complexity (C EM) evaluates the minimum total amount of information that has to be stored at any time for an optimal prediction of the next symbol. This measure can be approximately calculated as (Grassberger Citation1986):
The effective measure complexity describes the minimum information required for an optimal prediction of the next symbol.
Values of the complexity measure (fluctuation and effective measure complexity) are small for time series that are easy to describe, such as constant or periodic sequences, or completely random data. Larger values of the complexity measures are observed in time series that are not amenable to an easy description involving only a few parameters (Pachepsky et al. Citation2006, Wolf Citation1999).
All information theory-based measures were computed in this study using the SYMDYN software (Wolf Citation1999). The length of words L was set equal to two according to the length of the data set (Pachepsky et al. Citation2006).
2.3 Soil moisture content simulation
Soil moisture content was simulated in this study using the HYDRUS-1D model, a finite element model for simulating the one-dimensional movement of water, heat and multiple solutes in variably saturated media (Simunek et al. Citation2008). The water flow in variably saturated soils is governed by the Richards equation:
The HYDRUS-1D software was used here to simulate soil moisture content along the vertical soil profiles using the data on rainfall, ET, soil properties and groundwater level. The vertical soil material distribution was obtained from soil cores. The hydraulic conductivity was estimated based on the soil pore size distribution using the ROSETTA software (Schaap et al. Citation2001). Saturated and residual soil moisture contents, and van Genuchten parameters were obtained by fitting Equationequation (6)(6) to measured water retention curves. The atmospheric boundary with daily rainfall and ET was set as the top boundary condition, and the variable pressure head from the measured groundwater level was set as the bottom boundary condition. The measured soil moisture content was set as the initial condition. The soil moisture content was measured and simulated for 267 days in 2007 at plots P1, P3, and P4. The soil hydraulic conductivity and van Genuchten parameters were calibrated using a series of trial-and-error runs based on simulations of 30-day soil moisture content data obtained in 2006.
3 RESULTS AND DISCUSSION
3.1 Rainfall and soil moisture content
The information content and complexity measures of rainfall, ET and soil moisture content based on daily time series are plotted in . The metric entropy and mean information gain of daily rainfall data were larger than 0.8, indicating the extremely high randomness of the daily rainfall time series and a relatively uniform distribution of the states. The state and transition probabilities of daily rainfall time series for the states 00, 01, 10, 11 are listed in the . The corresponding state probabilities were 0.492, 0.170, 0.170 and 0.168, respectively. The state probability of two consecutive days being both less than median (state 00) was much greater than the probability of both being larger than median (state 11), or the probability of switching from less than median to the larger than median (state 01) and vice versa (state 10). The total number of days without rainfall was 241 in the year of 2007, and thus the median value was set to zero and the rainfall for 241 days in the 365-day rainfall time series was less than the median with a state of 00. Correspondingly, the probability of the state 00 was close to 0.5. The metric entropy from Equationequation (1)(1) had a relatively large value of 0.893, because the other three state probabilities were significantly smaller than 0.5. The mean information gain was 0.868 for daily rainfall time series based on Equationequation (2)
(2) and the transition probabilities listed in .
Fig. 3 Information theory-based measures of daily rainfall, evaporation, and soil moisture content time series.
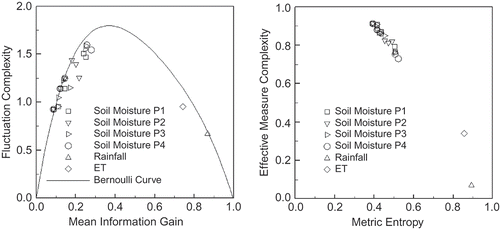
Table 1 State and transition probabilities of the time series of rainfall, ET, and measured soil moisture content at 20 cm below the surface of plot P1
The fluctuation complexity and the effective measure complexity were 0.671 and 0.071 for daily rainfall time series, respectively (Δ symbols in ). The fluctuation complexity represents the extent of fluctuation of information gain and loss in a time series. Because of the high randomness in daily rainfall time series, the fluctuation complexity was relatively small. The effective measure complexity describes the minimum information required for an optimal prediction of the next symbol and is calculated based on the ratio of the transition probability and state probability of a time series as given by Equationequation (4)(4). The value was extremely small for daily rainfall time series due to the small ratio of transition probability and state probability listed in . The results with high information content and low complexity of daily rainfall data identified the fact that meteorological controls of rainfall did not impose the structures on the precipitation time series. The information theory-based measures of daily ET time series are shown in and its state and transition probabilities listed in were similar to those for daily rainfall data. The differences were that the ET time series generally had less information content and a higher complexity than the rainfall data. This can also be explained because the solar radiation as the major ET control had less randomness and imposed more structure in the ET time series. The information measures of the rainfall and ET time series fall into the right end of the Bernoulli curve (), corroborating previous information theory-based studies of patterns in daily rainfall time series (Lange Citation1999a, Citation1999b, Pachepsky et al. Citation2006).
The information content and complexity measures of daily soil moisture time series at the observation depths are presented in . The metric entropy values were around 0.4–0.5 for all depths and the mean information gain varied from 0.08 to 0.3. The state and transition probabilities of daily soil moisture time series at 20 cm below the land surface are shown in for plot P1. The probabilities at other depths in the plots were similar (data not shown). The probabilities of states 00, and 11 were 0.481, and 0.475, much larger than those of states 01 (0.022) and 10 (0.022). Thus, the highly non-uniformly distributed soil moisture time series led to the small metric entropy. As compared with the daily rainfall and ET time series, the information content measures for soil moisture time series were much smaller than those of daily rainfall and ET data, indicating that soil moisture time series were much less random than the rainfall data. This is because the information contained in rainfall time series was only partially transferred to the soil moisture content in variably saturated soil. The soils provided substantial signal-filtering and de-noising during the information transfer from rainfall to the soil moisture content.
Table 2 Information measures of daily soil moisture content time series along the soil profile
The effective measure complexity and fluctuation complexity for soil moisture content at all depths were larger than 0.9 and much larger than those for daily rainfall time series. The transition probabilities from states 00 to 00, and 11 to 11 for soil moisture content time series in were 0.466, and 0.455, but those from one state to another state were very small, indicating that the state changes occurred rarely in the soil moisture content time series and that soil moisture remained in either state (00) or in state (11) for long time periods. This led to the large values of complexity measures according to Equationequations (3)(3) and Equation(4)
(4), which indicates that the soil moisture time series had more intrinsic structure than the precipitation time series. Rainfall conversion to soil moisture content was controlled by physical processes of canopy interception, ET, runoff, and infiltration. These controls probably imposed additional structure on the soil moisture time series as compared with the rainfall time series.
Information theory-based measures of daily soil moisture time series in soil profiles at the four plots are shown in . The metric entropy and the mean information gain of soil moisture content decreased with depth, demonstrating the increase in the information filtering action of soil. The effective measure complexity had a tendency to increase with depth. The slight increase in probabilities of dominant states 00, and 11 and the increased transition probabilities along the soil profile led to the decrease in information content measures and to the increase in effective measure complexity along the depths. The fluctuation complexity had a tendency to decrease with depth. Although the ratio of two state probabilities (e.g. two states 00 and 01) increased with depth, the transition probability decreased (e.g. from state 00 to 01) as the depth increased. Thus, small transition probabilities caused the decrease in fluctuation complexity along the soil profile according to Equationequation (3)(3).
3.2 Simulated soil moisture content
shows measured and simulated water contents from 20 cm to 80 cm below the land surface at 10 cm increments, for plots P1, P3, and P4. The root-mean-square errors and mean-absolute errors between the measured and simulated soil moisture content are listed in . In general, the root-mean-square errors were in the range 0.0112–0.0465 at these depths for plots P1, P3, and P4 and the mean-absolute errors were smaller than the root-mean square errors for the corresponding depths. Relatively small root-mean-square and mean-absolute error values indicate a good agreement between the simulated and measured soil moisture content. The information theory-based measures of simulated soil moisture time series were very close to those of the measurements () indicating that the simulated soil moisture time series reproduced the information content and complexity of the measurements. also shows that the range of information theory-based measures of simulated time series at different depths was narrower than that of the measurements. For example, standard deviations of metric entropy, mean information gain, fluctuation complexity and effective measure complexity for measured soil moisture time series were 0.042, 0.059, 0.052 and 0.212 for Plot P1, respectively. The corresponding standard deviations of information theory-based measures for simulated time series were 0.021, 0.029, 0.031 and 0.104. The spatial variability of the information and complexity measures for simulated soil moisture time series at all depths was less pronounced than for the measured time series. Reasons for this could be the use of the same values for boundary conditions or using pedotransfer functions that smoothed the structures of the time series.
Table 3 Root-mean-square-error (RMSE) and mean-absolute-error (MAE) of measured and simulated soil moisture content along the soil profile
Fig. 5 Measured (solid line) and simulated (dashed line) soil moisture contents down the soil profile.
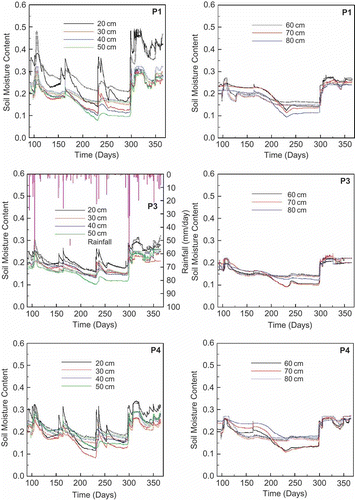
Fig. 6 Information theory-based measures of measured and simulated soil moisture content time series.
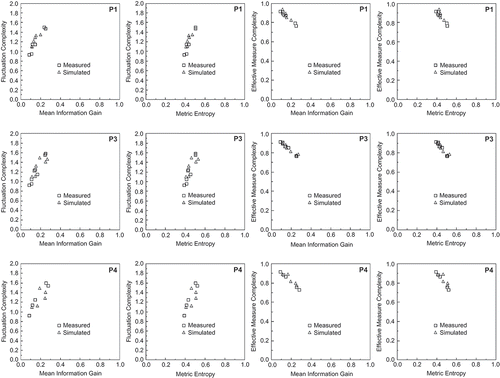
In a previous study, Pachepsky et al. (Citation2006) applied the information theory-based measures used in this study to discriminate the model performance of soil water flux simulations by HYDRUS-1D and MWBUS models. The conclusions were that the randomness and complexity of the simulated output may depend on the conceptual complexity of the models. The average values of metric entropy and mean information gain of the HYDRUS-1D simulated soil flux time series in Pachepsky et al. (Citation2006) were 0.73 and 0.44 ( in Pachepsky et al. Citation2006), larger than the results of this study (0.44 and 0.16, in this study). This study has analysed the data, which exhibited less randomness and relative non-uniform time series of the simulations. The simulated soil moisture time series here contained more information required for predictions than the ones of Pachepsky et al. (Citation2006) due to the smaller effective measure complexity in this study. Although we used the same model HYDRUS-1D to simulate the soil water redistribution in the two studies, the output variables were different, the soil water flux in Pachepsky et al. (Citation2006) and soil moisture content in this study. The cumulative soil water flux was calculated based on the simulated soil moisture content in HYDRUS-1D and the conceptual simple assumptions. This is the reason why the soil moisture time series in this study had more complexity and less information content than the cumulative soil water flux time series in Pachepsky et al. (Citation2006).
4 GENERAL REMARKS
In this study, the information content measures (i.e. metric entropy and mean information gain) and complexity measures (i.e. fluctuation complexity and effective measure complexity) were selected to study the information content and complexity of simulated and measured soil moisture time series. A variety of other measures in information theory could have also been used to measure the information and complexity of the time series, e.g. mean mutual information, Rényi entropy, information entropy, relative entropy, principle of maximum entropy, etc. (Wolf Citation1999, Mays et al. Citation2002, Al-Hamdan and Cruise Citation2010, Brunsell Citation2010, Singh Citation2010). The selection of information measures depends on the type of study, the model and the type of the model output used in the analysis (Pachepsky et al. Citation2006).
Using more than one measure of the information content and complexity may be beneficial for the comprehensive analysis of patterns in time series. Metric entropy represents the extent of a time series close to uniform distribution, and mean information gain describes how much information is to be gained by knowing the next symbol. The metric entropy can present the information of randomness and time series distribution, but cannot give the information of the state changes of a time series, which can be presented by mean information gain. The two measures are necessary to describe the characteristics of a time series including its randomness, distribution information, and state changes within the sequences. Fluctuation complexity is a measure of fluctuation between the information gain and loss, and effective measure complexity evaluates the minimum information required for an optimal prediction of the next symbol. To better understand the complexity of a time series and its prediction, it is important to have the complexity measures, fluctuation complexity and effective measure complexity. If only one of the information content and complexity measures is adopted, it is not enough to characterize the distribution and fluctuations in a time series.
Recent studies (Engelhardt et al. Citation2009, Pachepsky et al. Citation2006, Wang et al. Citation2009) have adopted the two-letter system (i.e. binary symbols) to represent a time series with two states 0 and 1. Since the measured and simulated soil moisture content relatively rarely switched from larger than median to lower than median values in this study, the three- or four-letter alphabet might better capture the changes between states and more accurately analyse the randomness and complexity of the time series. We note, however, that the number of symbols and the size of the alphabet affect the applicability of complexity measures for the given time series lengths. The accuracy of information theory-based measure estimation is dependent on the estimation of probability distributions of words and transitions in symbol strings. The relative frequency is used to estimate the probability and its accuracy is based on the availability of data (Pachepsky et al. Citation2006). Wolf (Citation1999) presented the length of a time series required to estimate the information theory-based measures with 5% relative error or better accuracy. For example, at least 146 and 262 symbols are required to estimate fluctuation complexity for two-symbol and three-symbol alphabets, respectively. The length of a time series is an important factor in selecting the number of symbols.
Complexity is a term having multiple connotations and subject-specific theories of complexity are developed, for example, in system dynamics and industrial engineering. This work focused on the specific type of complexity in time series manifested by the presence of patterns and deviations from randomness; in other words, on structure within seemingly random time series. This definition of complexity affects the interpretation of fluctuations in time series. Fluctuations are characterized by state and transition probabilities rather than by absolute values of soil water contents. Both complexity measures (fluctuation and effective measure complexity) evaluate the dissimilarity between the groups of consecutive measurements rather than fluctuations from one measurement to the next. The binary coding provides a very coarse partition of the whole range of measurements. Therefore, only very substantial fluctuations in water contents, that result in transition from the top 50% to the bottom 50% or vice versa, are reflected in state and transition probabilities, and affect complexity measures. Using a larger number of symbols for coding will lead to the introduction of more detail into fluctuation description. That however, will require longer time series to evaluate the complexity measures in a reliable manner, as discussed above.
5 CONCLUSIONS
This study applied information theory-based measures to compare the patterns in observed and measured soil moisture time series, and to use the information content and complexity measures to evaluate the ability of the model to reproduce the temporal trends of soil moisture content in variably saturated soils. The results demonstrated that the soil worked essentially as an information filter during the information transfer from the rainfall through the variably saturated soils, and that the modelling reproduced the information content and complexity of the soil moisture time series.
The information content of daily rainfall data was larger than 0.8, indicating the extremely high randomness and close to uniform distribution of daily rainfall time series. The relatively uniform distribution and small ratio of state and transition probabilities led to the low complexity of daily rainfall time series. These results were similar to results of previous studies (Lange Citation1999a, Citation1999b, Pachepsky et al. Citation2006) obtained in different geographic regions. The comparisons of the information measures of daily rainfall and soil moisture content data showed the relatively smaller information content and greater complexity in soil moisture time series than in rainfall data. The results indicated that the information of rainfall time series was only partly transferred to the soil moisture time series through the variably saturated soils and that the soils played a critical role in signal-filtering during the information transferring processes. There was more structure in the soil moisture time series due to their higher complexity. The information content of soil moisture time series decreased with the increase of complexity down the soil profile, demonstrating the increase in the information filtering action of soil.
The soil moisture time series was simulated using the HYDRUS-1D software based on the observed rainfall, ET, groundwater level, and calibrated soil properties. The good agreement and small values of root-mean-square errors between the measured and simulated soil moisture content indicated the successful model simulations. The information measures were used as non-residual-based criteria to evaluate the model's ability to determine whether the modelling led to a substantial loss of information or complexity. The range of information measures of the simulated results was narrower than that of the measurements, demonstrating that the modelling led to some loss of information or complexity. However, overall, the information measures of the simulated and observed soil moisture time series were close, indicating that the model was able to reproduce patterns in observed time series.
Acknowledgements
This study was supported by US Department of Agriculture and US Nuclear Regulatory Commission Interagency Agreement IAA-NRC-05-005 on “Model Abstraction Techniques to Simulate Transport in Soils”.
REFERENCES
- Al-Hamdan , O. Z. and Cruise , J. F. 2010 . Soil moisture profile development from surface observations by principle of maximum entropy . Journal of Hydrologic Engineering , 15 ( 5 ) : 327 – 337 .
- Allen, G., Pereira, L. S., Raes, D. and Smith, M. (1998) Crop evapotranspiration—guidelines for computing crop water requirements. FAO Irrigation and Drainage Paper 56. Available at http://www.fao.org/docrep/X0490E/x0490e00.htm#Contents (http://www.fao.org/docrep/X0490E/x0490e00.htm#Contents)
- Bates , J. E. and Shepard , H. K. 1993 . Measuring complexity using information fluctuation . Physics Letters A , 172 ( 6 ) : 416 – 425 .
- Brunsell , N. A. 2010 . A multiscale information theory approach to assess spatial-temporal variability of daily precipitation . Journal of Hydrology , 385 : 165 – 172 .
- Casper , M. , Gemmar , P. , Gronz , O. , Johst , M. and Stüber , M. 2007 . Fuzzy logic-based rainfall–runoff modeling using soil moisture measurements to represent system state . Hydrological Sciences Journal , 52 ( 3 ) : 478 – 490 .
- Chang , W. , Fang , B. , Yun , X. and Wang , S. 2009 . The Block Lossless Data Compression Algorithm . International Journal of Computer Science and Network Security , 9 ( 10 ) : 116 – 123 .
- Delworth , T. and Manabe , S. 1989 . The influence of soil wetness on near-surface atmospheric variability . Journal of Climate , 2 : 1447 – 1462 .
- Engelhardt , S. , Matyssek , R. and Huwe , B. 2009 . Complexity and information propagation in hydrological time series of mountain forest catchments . European Journal of Forest Research , 128 : 621 – 631 .
- Entin , J. K. , Robock , A. , Vinnikov , K. Y. , Hollinger , S. E. , Liu , S. and Namkhai , A. 2000 . Temporal and spatial scales of observed soil moisture variations in the extratropics . Journal of Geophysical Research – Atmosphere , 105 ( D9 ) : 11865 – 11877 .
- Grassberger , P. 1986 . Toward a quantitative theory of self-generated complexity . International Journal of Theoretical Physics , 25 : 907 – 938 .
- Guber , A. K. , Pachepsky , Y. A. , Rowland , R. and Gish , T. J. 2010 . Field correction of the multisensor capacitance probe calibration . International Agrophysics , 24 : 43 – 49 .
- Haider , S. S. , Said , S. , Kothyari , U. C. and Arora , M. K. 2004 . Soil moisture estimation using ERS 2 SAR data: a case study in the Solani River catchment . Hydrological Sciences Journal , 49 ( 2 ) : 323 – 334 .
- Kim , C. P. and Entekhabi , D. 1998 . Impact of soil heterogeneity in a mixed-layer model of the planetary boundary layer . Hydrological Sciences Journal , 43 ( 4 ) : 633 – 658 .
- Kuenzer, C., Bartalis, Z., Schmidt, M., Zhao, D. and Wagner, W. (2008) Trend analyses of a global soil moisture time series derived from ERS-1/-2 scatterometer data: floods, droughts and long term changes. The International Archives of Photogrammetry, Remote Sensing and Spatial Information Sciences XXXVII (B7), 1363–1368. http://www.isprs.org/proceedings/XXXVII/congress/7_pdf/8_ICWG-VII-IV/01.pdf (http://www.isprs.org/proceedings/XXXVII/congress/7_pdf/8_ICWG-VII-IV/01.pdf)
- Lange , H. 1999a . “ Time series analysis of Ecosystem variables with complexity measures ” . In International Journal for Complex Systems , Cambridge, MA : New England Complex Systems Institute . Mauscript #250
- Lange , H. 1999b . Are ecosystems dynamical systems? . International Journal of Computing Anticipatory Systems , 3 : 169 – 186 .
- Mays , D. C. , Faybishenko , B. A. and Finsterle , S. 2002 . Information entropy to measure temporal and spatial complexity of unsaturated flow in heterogeneous media . Water Resources Research , 38 ( 12 ) : 1313 doi: doi:10.1029/2001WR001185
- Moss , M. E. 1992 . Bayesian relative information measure: a tool for analyzing the outputs of general circulation models . Journal of Geophysical Research – Atmosphere , 97 ( D3 ) : 2743 – 2755 .
- Nie , S. , Luo , Y. and Zhu , J. 2008 . Trends and scales of observed soil moisture variations in China . Advances in Atmospheric Sciences , 25 ( 1 ) : 43 – 58 .
- Oevelen , P. J. 1998 . Soil moisture variability: a comparison between detailed field measurements and remote sensing measurement techniques . Hydrological Sciences Journal , 43 ( 4 ) : 511 – 520 .
- Or , D. and Ghezzehei , T.A. 2000 . Dripping into cavities from unsaturated fractures under evaporative conditions . Water Resources Research , 36 ( 2 ) : 381 – 393 .
- Or , D. and Moebius , F. Capillary-inertial jumps and dissipation at imbibition and drainage fronts—on the difference between transient and steady unsaturated flows . American Geophysical Union (AGU) Fall Meeting . San Francisco, CA. pp. H13C – 0973 .
- Pachepsky , Y. , Guber , A. , Jacques , D. , Simunek , J. , van Genuchten , M. Th. , Nicholson , T. and Cady , R. 2006 . Information content and complexity of simulated soil water fluxes . Geoderma , 134 : 253 – 266 .
- Schaap , M. G. , Leij , F. J. and van Genuchten , M.Th. 2001 . ROSETTA: a computer program for estimating soil hydraulic parameters with hierarchical pedotransfer functions . Journal of Hydrology , 251 : 163 – 176 .
- Shannon , C. E. 1948 . A mathematical theory of communication . ATandT Technology Journal , 27 ( 379–423 ) : 623 – 656 .
- Simunek , J. , Sejna , M. , Saito , H. , Sakai , M. and van Genuchten , M.Th. 2008 . “ The HYDRUS-1D software package for simulating the one-dimensional movement of water, heat, and multiple solutes in variably-saturated media ” . In Version 4.0, HYDRUS Software Series 3 , Riverside, CA : Department of Environmental Sciences, University of California Riverside .
- Singh , V. P. 2010 . Entropy theory for derivation of infiltration equations . Water Resources Research , 46 : W03527 doi: doi:10.1029/2009WR008193
- Usowicz , B. 1999 . Application of geostatistical analysis and fractal theory for the investigation of moisture dynamics in soil profile of cultivated field (in Polish) . Acta Agrophysica , 22 : 229 – 243 .
- van Genuchten , M. Th. 1980 . A closed-form equation for predicting the hydraulic conductivity of unsaturated soils . Soil Science Society of America Journal , 44 : 892 – 898 .
- Wang , K. , Zhang , R. and Hiroshi , Y. 2009 . Characterizing heterogeneous soil water flow and solute transport using information measures . Journal of Hydrology , 370 : 109 – 121 .
- Wolf , F. 1999 . Berechnung von Information und Komplexität von Zeitreihen – Analyse des Wasserhaushaltes von bewaldeten Einzugsgebieten . Bayreuth. Forum Okologie , : 65
- Zehe , E. , Graeff , T. , Morgner , M. , Bauer , A. and Bronstert , A. 2010 . Plot and field scale soil moisture dynamics and subsurface wetness control on runoff generation in a headwater in the Ore Mountains . Hydrology and Earth System Sciences , 14 : 873 – 889 .