Abstract
The method of fragments is applied to the generation of synthetic monthly streamflow series using streamflow data from 34 gauging stations in mainland Portugal. A generation model based on the random sampling of the log-Pearson Type III distribution was applied to each sample to generate 1200 synthetic series of annual streamflow with an equal length to that of the sample. The synthetic annual streamflow series were then disaggregated into monthly streamflows using the method of fragments, by three approaches that differed in terms of the establishment of classes and the selection of fragments. The results of the application of such approaches were compared in terms of the capacity of the method to preserve the main monthly statistical parameters of the historical samples.
Editor D. Koutsoyiannis; Associate editor C. Onof
Citation Silva, A.T. and Portela, M.M., 2012. Disaggregation modelling of monthly streamflows using a new approach of the method of fragments. Hydrological Sciences Journal, 57 (5), 942–955.
Résume
La méthode des fragments est appliquée à la génération de séries synthétiques de débits mensuels en utilisant les données de 34 stations de jaugeage au Portugal continental. Le modèle de génération basé sur l'échantillonnage aléatoire de la loi log-Pearson III a été appliqué à chaque échantillon, pour générer 1200 séries synthétiques de débits annuels de même longueur que l'échantillon de base. Les débits annuels synthétiques ont ensuite été désagrégés en débits mensuels par la méthode des fragments, suivant trois approches différentes concernant la répartition en classes et la sélection des fragments. Les résultats de l'application de ces approches ont été comparés en termes de capacité de la méthode à préserver les principaux paramètres statistiques mensuels des échantillons historiques.
1 INTRODUCTION
Stochastic generation of hydrological data is widely applied in the planning and management of water resources systems (Salas et al. Citation1980, p. 16, Santos Citation1983, p. 1) The modelling of monthly hydrological data, in general, and monthly streamflow data, in particular, can adopt two general approaches: (i) the generation of monthly flows directly using an appropriate monthly model; or (ii) the generation of annual flows using an appropriate annual model, and the subsequent disaggregation of those annual flows into monthly flows. The second approach has the advantage of allowing the preservation of the statistical properties of a historical sample at both the annual and monthly time scales (Santos and Salas Citation1992). The method of fragments, proposed by Svanidze (Citation1964), is one of the disaggregation models presented by the literature on stochastic streamflow generation (Srikanthan and McMahon Citation1980a, 1980b, Citation1982, Svanidze Citation1980, Santos Citation1983, Maheepala and Perera Citation1996, McMahon and Adeloye Citation2005, Ndiritu Citation2011).
The method of fragments uses a purely deterministic approach to disaggregate annual flows into monthly flows: given a historical streamflow sample of N years, the observed monthly flows in each year of the historical sample are divided by the corresponding annual streamflow. The resulting set of 12 standardized monthly flows constitutes a fragment. Each value of the generated annual streamflow series is disaggregated into 12 generated monthly flows by a fragment. According to Santos (Citation1983, pp. 19–20), the way in which the fragments are assigned to each generated annual value is the most important feature of the method.
After the fragments are obtained, they can be assigned randomly to the generated annual flows. Srikanthan and McMahon (Citation1980a, 1980b, 1982) found that this approach did not preserve the monthly parameters of the historical sample; hence, they attempted to improve the method by introducing a relationship between the fragments and the annual flows. This was done by sorting the N historical annual flows from smallest to largest and forming N classes of fragments. The first class has the lower limit zero and the last class has no upper limit. The intermediate class limits were obtained by averaging two successive flows of the sorted series of annual flows. The corresponding fragments were assigned to each class. The generated annual flows are then checked one-by-one for the class to which they belong, and disaggregated using the corresponding fragment. By selecting an appropriate fragment for each synthetic streamflow, the preservation of the historical monthly statistics improved remarkably. Santos (Citation1983, p. 20) presented an explanation for such improvement: when there is a relationship between the within-year distribution of monthly flows of a given year and the total streamflow of that year, it must be taken into account when selecting the fragments to disaggregate the synthetic annual streamflows.
Although the method of fragments is capable of generating synthetic series of monthly flows, while generally preserving the monthly and annual statistics of the sample and the additive property, it has been known not to preserve the monthly serial correlation between the first month of a year and the last month of the previous year (Srikanthan and McMahon Citation1980a, Citation2001)
Porter and Pink (Citation1991) reported that the use of the method of fragments resulted in the generation of monthly flows with a noticeable repetitive and cyclical pattern. Those authors proposed a method of synthetic fragments where the fragments were obtained from a generated monthly flow sequence. However, this method did not overcome the problem of the non-preservation of the serial correlation between the first month of a given year and the last month of the previous one (Srikanthan and McMahon 2010). Maheepala and Perera (Citation1996) proposed a modification to the method of synthetic fragments and compared it to both the Porter and Pink (Citation1991) and the Srikanthan and McMahon (Citation1980a, 1982) variations of the method. The results showed that the modification preserved annual and monthly parameters satisfactorily, as well as monthly correlations across consecutive years.
Regarding the methods of synthetic fragments, Srikanthan and McMahon (Citation2001) commented that Porter and Pink (Citation1991) and Maheepala and Perera (Citation1996) are not clear as to how the synthetic monthly data were generated and that, furthermore, it is not clear how they handle the problem of generating the right amount of zero monthly flows, which is one of the major problems in applying data generation models to ephemeral streams, according to Srikanthan and McMahon (Citation1980a). This is an important aspect of the streamflow regime in mainland Portugal, particularly in the southern region.
Santos (Citation1983) suggests that the fragments should be grouped into classes that may have more than one fragment. When disaggregating a given generated annual streamflow, a fragment is randomly selected from the class to which that annual flow belongs. Arsénio (Citation2003) adopted this technique and suggests that, when disaggregating a synthetic annual streamflow series, the random selection of fragments from a given class is carried out without replacement, until the class becomes empty. At that point, all the fragments of that class are reintroduced into it. In order to study the effect that the number and amplitude of the classes of fragments have on the performance of the method, Arsénio (Citation2003) used the method of fragments for disaggregating monthly flows considering different criteria regarding the number and size of the classes. Arsénio (Citation2003) concluded that using classes of different sizes yielded better results, and that using a small number of classes (each one comprising a large number of fragments) produced the worst results. Arsénio (Citation2003) also suggested that the definition of the classes of fragments be made by trial and error until an assembly of fragments is found that leads to a more effective preservation of the statistics of the samples. This approach constitutes a source of ambiguity to the application of this method.
Recently, Silva (Citation2010) and Silva and Portela (Citation2010, Citation2011) applied the method of fragments to generate a large number of synthetic monthly flow series for 53 gauging stations in Portugal and obtained good results. Those authors defined the classes of fragments as probability intervals and thus avoided the trial-and-error approach proposed by Arsénio (Citation2003). The objective of this study is to evaluate the performance of the method of fragments using different criteria for the definition of classes and the selection of fragments.
Following the brief theory review of the method of fragments presented in this Introduction, a methodology is proposed for disaggregating annual flows into monthly flows using the method of fragments, in which three criteria for the selection of fragments have been used. Next, the streamflow data are presented and an attempt is made to characterize relationship between the fragments and the historical annual flows. The methodology is applied and an overall evaluation and discussion of the obtained results is made. Finally, some relevant conclusions are drawn.
2 METHODOLOGY
2.1 General considerations
The methodology used to generate synthetic monthly streamflow series consists of a two-level approach, comprising a generation model for the annual synthetic series and, at the monthly level, three approaches of the method of fragments that differ in their definition of the classes and selection of fragments. The synthetic series were generated with a length equal to that of the corresponding sample.
The generation of synthetic streamflow series is a widely-used technique in the framework of studies on reservoir storage–yield design (McMahon and Adeloye Citation2005, p. 123). Using streamflow data from four Portuguese rivers, Guimarães (Citation2005) and Guimarães and Santos (Citation2011) studied the number of synthetic series, M, to generate from a given streamflow sample, in the context of water-supply reservoir design studies, and concluded that it should be 1200. This number was adopted in the current analyses.
According to Quintela (Citation1967), the annual streamflow series of Portuguese rivers is an independent time series if the hydrological year (starting on 1 October) is adopted as the time step, provided that an analysis of the correlation structure of the series does not invalidate this assumption. Hence, the modelling of the annual streamflow series may use a non-deterministic probabilistic model.
2.2 Generation of annual streamflows
The model for generating annual flows consists of a random sampling of the log-Pearson Type III distribution. Because that distribution can only take values between 0 and , the generation of negative flows is naturally avoided. Furthermore, the log-Pearson III law has a flexible distribution, assuming a number of different shapes depending on the mean, variance and skewness of the sample, and, hence, the treatment of skewed data is assured (Chow et al. Citation1988, p. 375).
2.3 Monthly disaggregation of streamflows using the method of fragments
As mentioned in the Introduction, the method of fragments uses a purely deterministic approach to disaggregate synthetic annual flows into synthetic monthly flows. In this method, for a given year, k, the observed monthly flows are divided by the corresponding annual flow volume, Xk , and the resulting set of 12 standardized monthly flows constitutes the fragment pertaining to the year k, φ k :
where Xk ,j are the monthly flows (j = 1, 2, … , 12).
The application of the method of fragments requires that the fragments are formed and assembled into classes beforehand. For the constitution of the array of fragments, [φ], the annual flows must previously be ranked from smallest to largest:
where Xk
* represents the kth annual flow such that , and
represents the monthly flows of that year.
After the array of fragments is formed, one has to select a fragment, φ
i
, to disaggregate each synthetic annual flow, into 12 monthly flows,
, as follows:
where i = 1, 2, … , N and j = 1, 2, … , 12.
According to Santos (Citation1983, pp. 19–20), the way in which the fragments are selected for each synthetic annual flow is the most important feature of the method. In the state of the art involving the method of fragments, reviewed in the introduction, a number of different procedures are available for carrying out that selection, and this generally involves forming classes of fragments. In this paper, the method of fragments was implemented using three different criteria to define the classes of fragments and the selection of fragments. A description of each criterion follows (if pertinent, such description assumes that the annual flows are already ranked).
• |
Criterion 1 This criterion, which constitutes the initial concept of the method of fragments by Svanidze (Citation1964) (Srikanthan and McMahon Citation1980a, 1980b), considers a single class ranging from 0 to | ||||
• | Criterion 2 The second criterion adopts the modification proposed by Srikanthan and McMahon (Citation1980a, 1980b, 1982), in which they introduced a relationship between the fragments and the annual streamflow. This procedure considers one class per fragment, the lower limit of the first class being zero, the last class having no upper limit, and the remaining class limits being obtained by averaging each two consecutive values of the ranked series of annual streamflows. For each year of the synthetic series, the generated annual flow is checked to determine to which class it belongs. The selected fragment is the one associated with that class. | ||||
• | Criterion 3 This procedure, used by Silva (Citation2010) and Silva and Portela (Citation2010, 2011), defines the classes of fragments as probability intervals with uniform amplitude of 10%. It considers classes with one or more fragments and proposes a robust and well-defined methodology for the definition of these classes, thus avoiding the ambiguous and time-consuming trial-and-error approach used by Arsénio (Citation2003). Though additional research is still required regarding the effect of the amplitude of the intervals in the performance of the criterion, the procedure implemented is as follows. |
First, a series of nine equally-spaced non-exceedence probabilities of the annual streamflow, with an increment of 10%, is considered (10%, 20%, … , 90%). Next, one estimates the annual flows, , with the aforementioned non-exceedence probabilities, by inverting the probability distribution function of the log-Pearson III law. This results in 10 equally probable classes with limits represented by [
[, [
[, [
[, … , [
[, [
[.
The fragments are distributed among the successive classes, while simultaneously verifying if there are any empty classes. Any empty classes that occur must be redefined. If the first class is empty, it is included in the next class; if the last class is empty, it is included in the previous class; if an intermediate class is empty, half of the probability step that defines it is attributed to each of the two bordering classes.
The fragments are randomly selected, without replacement. If any class runs out of fragments, it is refilled with the respective fragments.
It should be stressed that, while criteria 2 and 3 consider that there is a relationship between the annual streamflow and the within-year distribution of monthly flows, Criterion 1 considers that both features are independent.
Following the generation of the synthetic annual and monthly streamflow series, the results of the application of the method of fragments in accordance with each criterion were compared in terms of its ability to preserve the main seasonal statistical parameters of the historical samples, namely the mean, standard deviation, skewness coefficient and lag-one serial correlation of the monthly streamflow series.
3 STREAMFLOW DATA
The method of fragments, with the class definition and the fragment selection given by each criterion, was applied to obtain synthetic monthly flow series based on the streamflow samples from 34 gauging stations in unregulated Portuguese rivers. The names of the gauging stations and the main characteristics of the samples are presented in . The stations were selected from the set of 53 used by Silva (Citation2010) and by Silva and Portela (Citation2010, 2011), according to the following two rules: longest samples; even geographical distribution. By applying the above rules, 31 stations with at least 30 years of records were selected. This data set was complemented with three additional stations (numbers 10, 23 and 26) that have considerably shorter samples, but that cover areas where there is little or no information. The location of the 34 stations is presented in . As is shown in , the mean annual flow depth of the samples ranges from 127 to 2058 mm, thus representing a wide variety of hydrological regimes.
Table 1 Main characteristics of the annual streamflow samples
The correlation structure of the historical samples of annual streamflow was analysed by computing the lag-one autocorrelation coefficients of the annual flows. It was found that, at the analysed gauging stations, the annual streamflow values constitute an independent time series, provided that the hydrological year (starting on 1 October) is adopted.
A visual analysis was made for each sample to determine whether there was a relationship between the annual flow and the within-year distribution of monthly flows. An example of such analysis is given in for sample no. 2 (Amieira). In , for each year of the historical annual series (47 years for this sample), there is a set of 12 markers, displayed horizontally, representing the values that constitute the fragment for the annual streamflow indicated in the vertical axis. The colour of each marker indicates the ratio, R, between that monthly flow and the corresponding annual flow. If there was a relationship between the annual flow and the within-year distribution of monthly flows, one would expect the colours of the markers to vary at the same rate at which the annual flow increases or decreases, which does not happen: the marker colours for the different months do not exhibit discernible patterns.
Fig. 2 Visual analysis of the relationship between the fragments and the annual streamflows of sample no. 2 (Amieira).
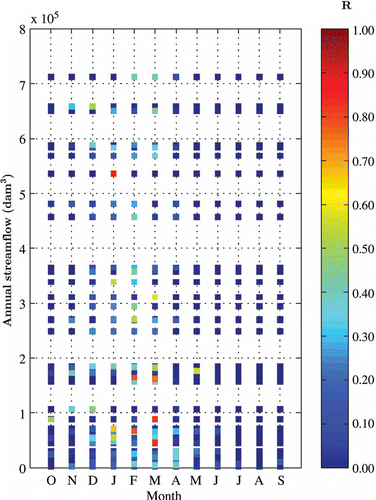
For the set of 34 gauging stations, the visual analyses based on diagrams such as that shown in were not conclusive as to whether or not there is any relationship between the annual flow and the within-year distribution of monthly flows. Furthermore, it is an ambiguous analysis, since it relies on subjective interpretation. Santos (Citation1983, p. 20) presents a more objective approach for evaluating whether or not there is a relationship between the within-year distribution of monthly flows and the annual flows of the streamflow sample. The approach is exemplified in . For a given sample, it consists of dividing the monthly flows of each year, Xi
,j
, by the mean monthly flow of that year, . Considering that the total time is one, i.e. each month is 1/12 in annual time units, the within-year irregularity factor, d, is the area above
. The area below the line is a natural flow regulation factor, ψ. Naturally, ψ + d = 1.
For each streamflow sample, the d values were plotted against the annual flows and the correlation coefficient, r, between the two variables is determined. shows the analysis for sample no. 2 (on the horizontal axis, the annual flows were divided by the mean annual flow of the sample). shows the correlation coefficient, r, obtained for each sample.
Table 2 Correlation coefficients, r, between the d values and the historical annual flows
The procedure proposed by Yevjevich (Citation1972, pp. 237–242) was used to determine whether a given value of r differs significantly from zero. A significance level of α = 5% was considered. It was found that in 11 of the samples, the correlation coefficients are significantly different from zero (indicated in bold in ).
Notwithstanding the prior analyses, the authors believe that the relationship between annual streamflow and the within-year distribution of monthly streamflow is not particularly strong in Portuguese rivers. This conclusion seems to be in accordance with the results presented in , as even for the cases where the correlation differs significantly from zero, the correlation coefficients are relatively small.
4 RESULTS AND DISCUSSION
The quality of the method of fragments, based on the three criteria presented in Section 2.3, was assessed by comparing monthly statistical parameters, namely the mean, standard deviation, skewness coefficient and lag-one serial correlation coefficient. Each statistical parameter evaluated based on a given sample was compared to the average of 1200 values of that parameter obtained from an equal number of synthetic flow series, each of equal length to that of the corresponding sample, as previously mentioned. Three sets of 1200 synthetic flow series were considered: one for each of the three different criteria used in implementing the method of fragments.
The comparison was made by plotting the relative error (%) of the mean and standard deviation (SD) estimates, and the absolute error (-) of the skewness and serial correlation estimates, against the months. It should be noted that this analysis takes into account the hydrological year in Portugal, and so it starts in October.
The absolute and relative errors were calculated as follows, where Gen denotes the mean of the 1200 statistics estimated from the generated synthetic series and Hist the same statistic estimated from the corresponding historical sample:
shows an example of the results obtained for sample no. 11 (Cunhas). Since it is not feasible to represent the analogous figures for the remaining samples here, the sums of the absolute values of the relative and absolute errors were computed and are shown in .
Table 3 Sum of the absolute values of the errors of the monthly parameters–relative errors for the means and standard deviations (SD), and absolute errors for the skewness coefficients and lag-1 serial correlations*
Fig. 5 Relative and absolute errors in the monthly parameters of sample no. 11 (Cunhas) (Hist. denotes the results obtained from the historic sample and Criterion 1 to 3, those from the method of fragments based on the different criteria).
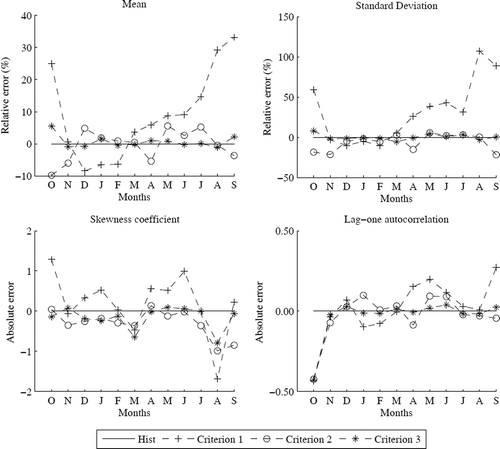
The results of and show that, although the analyses carried out, as exemplified in and , were not conclusive as to whether there is a relationship between the annual streamflow and the within-year distribution of monthly flows, the consideration of such a relationship when selecting the fragments greatly improves the performance of the method of fragments; that is, criteria 2 and 3 perform much better than Criterion 1. They also show that, generally, Criterion 3 performs better than Criterion 2. In fact, as highlighted in , from the 34 samples and depending on the monthly parameter, the best results (smallest sum of errors) were obtained for Criterion 1 in zero (SD and lag-1 serial corr.) to three (mean) samples, for Criterion 2 in three (SD) to eight (lag-1 serial corr.) samples, and for Criterion 3 in 26 (lag-1 serial corr.) to 31 (SD) samples. Furthermore, when Criterion 3 does not show the best performance, it is never the least well-performing, presenting error measures very close to those of the best criterion.
In order to further analyse the difference in performance of criteria 2 and 3, box plots were drawn of the generated values of the analysed monthly parameters and these were compared with the historical parameters. In those plots, the whiskers have a maximum length of 1.5 × IQR (interquartile range) and the values outside the whiskers are considered to be outliers (Robbins Citation2005, p. 91). Figures show the comparison between the performance of the two methods for samples 2, 9 and 30. The figures pertaining to the remaining samples, though omitted here, showed similar results: in general, Criterion 3 slightly, but consistently, outperforms Criterion 2.
Fig. 6 Comparison of the monthly parameters of the monthly historical and generated synthetic series of sample no. 2 (Amieira) (results obtained by using criteria 2 and 3 in the method of fragments).
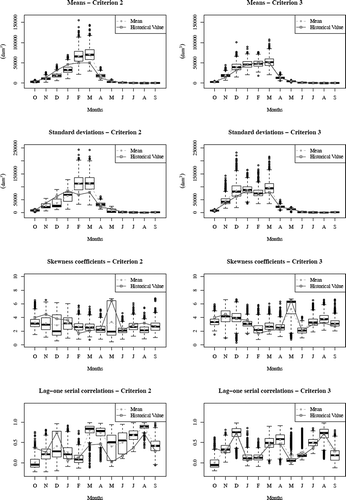
Fig. 7 Comparison of the monthly parameters of the monthly historical and generated synthetic series of sample no. 9 (Cidadelhe) (results obtained by using criteria 2 and 3 in the method of fragments).
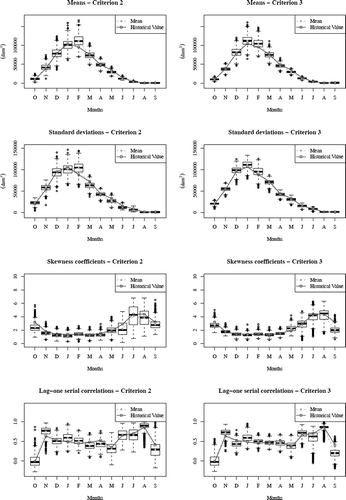
Fig. 8 Comparison of the monthly parameters of the monthly historical and generated synthetic series of sample no. 30 (Qta das Laranjeiras) (results obtained by using criteria 2 and 3 in the method of fragments).
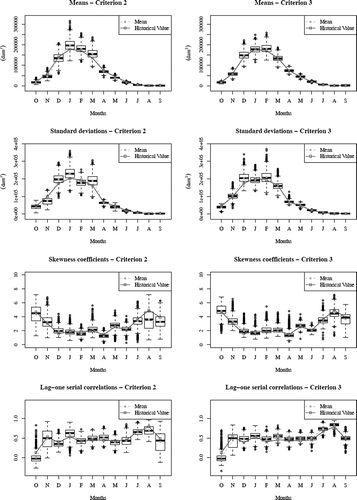
The latter results show that the consideration of fewer, wider classes (with more than one fragment), and the introduction of randomness when selecting a fragment from a class—as implemented by Criterion 3—improves the performance of the method of fragments, as generally denoted by the smaller amplitudes of the boxes and whiskers. The higher variability of the results produced by Criterion 2, though not yet totally understood, may be related to the fact that using N classes reduces the probability of applying some of the fragments, especially those with very narrow classes, resulting from annual flows that are very close together.
The results also confirm a well-known limitation of the method of fragments: the non-preservation of the serial correlation between the first month of one year and the last month of the previous year (Srikanthan and McMahon Citation1980a, 2001). In fact, Figs show that, for October, this coefficient is consistently close to zero in the synthetic series. We believe that the method of fragments (under the formulations used herein) can only preserve the first lag-one serial correlation if the historical one is close to zero itself. This can be verified for sample no. 9 (Cidadelhe) in .
5 CONCLUSIONS
The method of fragments was used to disaggregate synthetic annual streamflow values into synthetic monthly streamflow series using three different criteria to define the classes of fragments and to select the fragments:
1. | a single class with fragments selected randomly and without replacement; accordingly all the fragments are used (Svanidze Citation1964); | ||||
2. | as many classes as the number of fragments, with automatic replacement of each used fragment (Srikanthan and McMahon Citation1980b, Citation1980a, 1982); and | ||||
3. | classes defined based on the assumption of equally probable width; the fragments are selected randomly and the replacement only occurs when the class to which they belong becomes empty; not necessarily all the fragments are used (Silva Citation2010, Silva and Portela Citation2010, 2011). |
The different approaches were applied to 34 streamflow samples from gauging stations geographically spread throughout mainland Portugal. The performance of each approach was evaluated in terms of the capacity of the synthetic monthly series to preserve the main monthly statistical parameters of the historical samples. The results show that while criteria 2 and 3 perform much better than Criterion 1, Criterion 3 generally, outperforms Criterion 2. In fact, for most of the 34 samples (between 26 and 31 samples, depending on the monthly parameter), Criterion 3 ensures the smallest error measures. We stress that the results confirmed a well-known limitation of the method of fragments: the non-preservation of the serial correlation between the last month of one year and the first month of the following one.
Given the results described above, we recommend the method of fragments, integrated with he procedure developed by Silva (Citation2010) and Silva and Portela (Citation2010, 2011) for defining the classes and the selection of fragments (i.e. Criterion 3) for the disaggregation modelling of monthly streamflows in mainland Portugal.
The new approach of the method of fragments (Criterion 3) still provides opportunities for further development, such as: analysis of the effect of the amplitude of the probability intervals that define the classes of fragments in its performance; investigating the reason why it generally reduces the variability of the seasonal parameters of the generated series, compared to another approach (Criterion 2); the development of procedures for improving the preservation of the serial correlation between the last month of one year and the first month of the following one; and the possibility of its application to other time steps, namely daily.
REFERENCES
- Arsénio , J.J.G. 2003 . Gestão de Albufeiras: avaliação do potencial dos sistemas com recurso a séries sintéticas de escoamentos (in Portuguese) , Lisbon , , Portugal : Thesis (Master), Instituto Superior Técnico .
- Chow , V.T. , Maidment , D. and Mays , L. 1988 . Applied hydrology , Singapore : McGraw-Hill International .
- Guimarães , R.C.P.D.C. 2005 . Simulação no Dimensionamento e Gestão de Sistemas de Recursos Hídricos. Geração de Séries Sintéticas de Escoamento (in Portuguese) , Évora , , Portugal : Thesis (PhD), Universidade de Évora .
- Guimarães , R. and Santos , E.G. 2011 . Principles of stochastic generation of hydrologic time series for reservoir planning and design: a case study . Journal of Hydrologic Engineering , 1 : 237
- Maheepala , S. and Perera , B. 1996 . Monthly hydrologic data generation by disaggregation . Journal of Hydrology , 178 ( 1–4 ) : 277 – 291 .
- McMahon , T. and Adeloye , A. 2005 . Water resources yield , Littleton , CO : Water Resources Publications .
- Ndiritu , J. 2011 . A variable-length block bootstrap method for multi-site synthetic streamflow generation . Hydrological Sciences Journal , 56 ( 3 ) : 362 – 379 .
- Porter , J.W. and Pink , B.J. 1991 . “ Method of synthetic fragments for disaggregation in stochastic data generation ” . In International Hydrology and Water Resources Symposium, 2–4 October 1991, Perth, Australia , 781 – 786 . Barton, ACT : Institute of Engineers .
- Quintela , A. 1967 . Recursos de águas superficiais em Portugal Continental (in Portuguese) , Lisbon , , Portugal : Thesis (PhD), Instituto Superior Técnico .
- Robbins , N.B. 2005 . Creating more effective graphs , Hoboken , NJ : Wiley-Interscience .
- Salas , J.D. , Delleur , J.W. , Yevjevich , V. and Lane , W.L. 1980 . Applied modeling of hydrologic time series , 2nd , Littleton , CO : Water Resources Publications .
- Santos , E.G. 1983 . Disaggregation modeling of hydrologic time series , Colorado , , USA : Thesis (PhD), Colorado State University .
- Santos , E.G. and Salas , J.D. 1992 . Stepwise disaggregation scheme for synthetic hydrology . Journal of Hydraulic Engineering , 118 ( 5 ) : 765 – 784 .
- Silva , A.T.C. and de , F. 2010 . Design of the storage capacity of artificial reservoirs , Lisbon , , Portugal : Thesis (Master), Instituto Superior Técnico .
- Silva , A.T. and Portela , M.M. 2010 . Geração de séries sintéticas de escoamentos anuais e mensais: aplicação extensiva a Portugal Continental (in Portuguese) . Revista Recursos Hídricos , 31 ( 2 ) : 27 – 41 .
- Silva , A.T. and Portela , M.M. 23–24 May 2011 2011 . “ Generation of monthly synthetic streamflow series based on the method of fragments ” . In Water Resources Management 2011—6th International Conference on Sustainable Water Resources Management , 23–24 May 2011 , Riverside , CA : Wessex Institute of Technology . Hampshire
- Srinkanthan , R. and McMahon , T.A. 1980a . Stochastic generation of monthly flows for ephemeral streams . Journal of Hydrology , 47 ( 1–2 ) : 19 – 40 .
- Srinkanthan , R. and McMahon , T.A. 1980b . Stochastic time series modelling of arid zone streamflows . Hydrological Sciences Bulletin , 25 ( 4 ) : 423 – 434 .
- Srinkanthan , R. and McMahon , T.A. 1982 . Stochastic generation of monthly streamflows . Journal of the Hydraulics Division of the American Society of Civil Engineers , 108 ( 3 ) : 419 – 441 .
- Srinkanthan , R. and McMahon , T.A. 2001 . Stochastic generation of annual, monthly and daily climate data: a review . Hydrology and Earth System Sciences , 5 ( 4 ) : 653 – 670 .
- Svanidze , G.G. 1964 . “ Osnovy rascheta regulirovaniya rechnogo stoka metodom Monte-Karlo ” . In Izd. Akad. Nauk GSSR, Tbilisi (in Russian)
- Svanidze , G.G. 1980 . Mathematical modeling of hydrologic series; for hydroelectric and water resources computations , Littleton , CO : Water Resources Publications .
- Yevjevich , V. 1972 . Probability and statistics in hydrology , Fort Collins , CO : Water Resources Publications .