Abstract
Two key issues of continuous wavelet transform (CWT), the choice of wavelet basis function and the determination of analytic procedure of CWT, were studied and approaches to solve them proposed. Then, the improved CWT method was used to reveal the periodic characteristics of several typical hydrological series, including runoff and precipitation data measured at diverse sampling rates. Finally, the results of periods identified by three methods were compared, and the variation of the first main period (FMP) with length of the annual hydrological series was investigated. The results indicate that hydrological time series show both global and local characteristics. Comparatively, the latter are more complicated and difficult to describe, because of their frequent manifestations of irregular phenomena. Moreover, the variation of FMP just reflects the complicated local characteristics of the hydrological series. In summary, this study improves the understanding of complicated hydrological processes, whereas description and simulation of the local characteristics of hydrological series should be the focus of future research.
Editor D. Koutsoyiannis
Citation Sang, Y.F., Wang, D., Wu, J.-C., Zhu, Q.-P., and Wang, L., 2013. Improved continuous wavelet analysis of variation in the dominant period of hydrological time series. Hydrological Sciences Journal, 58 (1), 1–15.
Résumé
Nous avons étudié deux questions fondamentales de la transformée en ondelettes continues (TOC), à savoir le choix de la fonction de base de l'ondelette et la détermination de la procédure analytique de la TOC et proposé des approches pour leur résolution. Nous avons ensuite utilisé la TOC améliorée pour révéler les caractéristiques de périodicité de plusieurs séries hydrologiques caractéristiques, comprenant des données de précipitation et d'écoulement mesurées selon différentes fréquences d'échantillonnage. Enfin, les périodes identifiées par trois méthodes ont été comparées, et nous avons étudié la variation de la première période principale (PPP) en fonction de la longueur des séries hydrologiques annuelles. Les résultats montrent que les séries hydrologiques présentent à la fois des caractéristiques globales et locales. Comparativement, ces dernières sont plus complexes et difficiles à décrire en tant que manifestations fréquentes de phénomènes irréguliers. En outre, la variation de la PPP ne reflète que les caractéristiques complexes locales de la série hydrologique. En résumé, cette étude permet de mieux comprendre des processus hydrologiques complexes, mais la description et la simulation des caractéristiques locales des séries hydrologiques devront faire l'objet de futures recherches.
1 INTRODUCTION
Hydrological time series are the comprehensive reflections of the hydrological processes which are influenced by many and often interrelated physical factors, especially climatic conditions, land surface conditions and human activities (Blazkova and Beven Citation1997, Pelgrum et al. Citation2000, Katz Citation2002, Hanson et al. Citation2004, Seungho et al. Citation2005). Observed hydrological series usually show non-stationary and multi-temporal scale characteristics in daily, monthly, annual and multi-annual processes (Cahill Citation2002, Neupauer et al. Citation2006, Sang et al. Citation2012b). It is important to reveal the characteristics and identify components of hydrological time series, because these are the basis of understanding hydrological processes, hydrological simulation and forecasting, water resources management, as well as many other practical water activities. However, it is a difficult task in practice, because of the influences from many adverse factors. Traditional methods, such as time-series analytic techniques and Fourier transform, have their own deficiencies and thus cannot adequately meet the practical needs (Matalas Citation1967, Box et al. Citation1994, Fleming et al. Citation2002). Wavelet analysis (WA) is a prevalent and powerful method of time series analysis (Mallat Citation1989, Daubechies Citation1992, Walker Citation1999, Kwon et al. Citation2006). At present, WA has been widely applied to many fields of hydrology, including, but not limited to, the following aspects: (a) revelation of the characteristics of hydrological series under multi-temporal scales (Torrence and Compo Citation1998); (b) identification of diverse components in hydrological series, such as periods, trend and change points (Smith et al. Citation1998, Ravines et al. Citation2008); (c) description of the complexities of hydrological series by proper indexes, such as wavelet energy entropy (WEE) (Labat Citation2005) and fractal dimension (D) (Blanco et al. Citation1998), and analysis of the energy distribution of hydrological series at multiple scales by wavelet variance estimator (Coulibaly and Burn Citation2004); (d) de-noising in hydrological time series (Donoho Citation1995, Bruni and Vitulano Citation2006, Sang et al. Citation2009a); and (e) wavelet cross-correlation analysis of hydrological series (Labat Citation2005, Schaefli et al. Citation2007).
Although wavelet analysis is a complete methodology, as discussed above, its effectiveness in practice is influenced by many key issues that are still unresolved. In this paper, we mainly focus on two key issues concerning continuous wavelet transform (CWT). The first is choice of the wavelet basis function (WBF). In the process of wavelet analysis, if the WBF used is not suitable for the studied hydrological time series, the results would be inaccurate, or even completely wrong. Besides, the wavelets used for analysing those hydrological series with different composition and characteristics should also be different. Several contributions have discussed this issue, as outlined in Section 3, but no reasonable and workable approaches have been found to date. The other key issue is determination of the analytic procedure of CWT. Characteristics of hydrological time series under multi-temporal scales are obviously different (Torrence and Compo Citation1998, Cahill Citation2002, Neupauer et al. Citation2006, Sang et al. Citation2012a). Although the commonly-used two-dimensional contour map can represent the characteristics of hydrological time series, not all of them can be revealed and easily understood at once, especially when revealing local characteristics at small temporal scales. Therefore, a robust and workable analytical procedure of CWT should be established to allow the characteristics of hydrological time series to be readily revealed and understood at multi-temporal scales. We studied the above two issues mainly to improve the effectiveness of CWT in multi-temporal scale analysis of hydrological time series.
By using the improved CWT method, we analysed the periodic characteristics of several time series of hydrological variables with different sampling rates to explore the reason for variation in the first main period of annual hydrological series and, further, to obtain a deeper understanding of the hydrological processes. According to the theory of stochastic hydrology (Yevjevich Citation1972, Kwon et al. Citation2006), the period is one of the most important characteristics of hydrological processes; thus, identification of dominant periods is a frequent problem encountered in hydrological series analysis (Kottegoda et al. Citation2007), and many new and improved methods can be used to solve it, such as maximum entropy spectral analysis (MESA) (Padmanabhan and Rao Citation1988, Kayhan Citation1999), the main series spectral analysis (MSSA) (Sang et al. Citation2009b), as well as the CWT method. A commonly-encountered phenomenon in the process of identification of periods is that the annual hydrological series, first main period (FMP), whose power spectral density is the maximum among all the periods identified, varies with series length; however, no reasonable explanation for this phenomenon has yet been found. By identifying the dominant periods of several typical hydrological time series using the improved CWT method and other methods, the variation of the FMP with length of annual hydrological series is investigated herein. The results indicate that hydrological time series show both global and local characteristics; comparatively, the local characteristics are more complicated and difficult to describe because of their frequent manifestations of irregular variations. Moreover, the variation of FMP has no relationship with the methods used, but just reflects the complicated local characteristics of the hydrological series.
In Section 2 we briefly describe the continuous wavelet transform and in Section 3, the approach for choosing the WBF is proposed by discussing wavelet properties and the basic composition of hydrological series. The analytical procedure of CWT is put forward in Section 4 and in Section 5, the periodic characteristics of six annual hydrological series are revealed using three methods, and the results are compared to investigate the phenomenon of variation in FMP. Finally, this study is summarized and conclusions are drawn.
2 CONTINUOUS WAVELET TRANSFORM
The basic aim of wavelet transform is both to determine the frequency (or temporal scale) contents of a signal and how they vary with time (Heil and Walnut Citation1989). The wavelet basis function (WBF) is the “base” of wavelet transform; thus, choice of appropriate WBF is the foremost task in the wavelet process, and particular attention should be paid to it. A WBF is usually irregular, asymmetric and oscillating (Mallat Citation1989, Daubechies Citation1990). Defining ψ(t) as the WBF, it is dilated (or contracted) and translated in the wavelet transform process according to:
Defining L
2(R) as the measurable square-integral space, the continuous wavelet transform (CWT) of signal f(t)
L
2(R), given the proper wavelet, is obtained by:
a. | the wavelet coefficients curve of Wf (a,b)–a reflects the characteristics at a certain time position b, but at multi-temporal scales, and the curve of Wf (a,b)–b reflects the characteristics at a certain scale a, but at different time positions; | ||||
b. | positive and negative values of wavelet coefficients reflect different characteristics: positive values correspond to wet seasons, negative values correspond to dry seasons, and zero points correspond to changes from wet to dry, or from dry to wet seasons; and | ||||
c. | absolute values of wavelet coefficients show how remarkable the characteristics are: the bigger the absolute values, the more remarkable the characteristics are, and vice versa. |
3 CHOICE OF WAVELET BASIS FUNCTION
3.1 Approach proposed to choose WBF
The essence of wavelet transform is to reveal the similarity between the signal analysed and the wavelet used (Gaucherel Citation2002), so components and characteristics of the signal analysed can be accurately identified, provided that the wavelet used is very similar to it. However, it is difficult in practice to judge whether a certain wavelet is similar to the signal analysed or not, in spite of clearly knowing each wavelet's properties, so we cannot easily choose an appropriate wavelet either. Many papers have discussed this issue; for instance, Torrence and Compo (Citation1998) suggested that four factors, “orthogonal or non-orthogonal”, “complex or real”, “width” and “shape” of wavelets, be considered to choose WBFs; Schaefli et al. (Citation2007) improved this suggestion by proposing three points: they prefer to use wavelets with progressive and linear phases, the wavelet should exhibit good localization in both time and frequency domains, and the trade-off between time and scale resolutions has to be adapted to the analytical process. To the authors' knowledge, these suggestions in general are qualitative, empirical and rough, whereas more objective and more easily worked approaches for choosing a WBF should be offered for wavelet analysis of hydrological series.
By taking both the wavelet properties and the basic composition of the hydrological time series into account, we propose a new approach for choosing the WBF. There are three parts to the main idea:
a. | The choice of WBF should first consider the properties of the wavelet. Four points should be considered according to existing studies: besides the three given by Schaefli et al. (Citation2007), the chosen wavelet should also meet the regularity condition in Equationequation (3) | ||||
b. | The basic composition of the hydrological time series should also be considered when choosing the WBF. According to the theory of stochastic hydrology (Yevjevich Citation1972, Kwon et al. Citation2006), a hydrological time series is composed of deterministic and random components, referred to as the “main series” and “noise”, respectively, in this paper. The former are generated by certain deterministic physical mechanisms, and are the true signals (mainly including periods and trend) in hydrological series, whereas noise is generated by many random factors and contaminates the true signals (Yevjevich Citation1987, Sang et al. Citation2009a). Due to the difference between them, it is deduced that the chosen WBF should be very similar to the main series, but not to noise, provided that it is appropriate; in other words, the appropriate WBF should at least meet the need of accurately separating the main series from the original hydrological time series. | ||||
c. | According to the essence of wavelet transform, if a certain WBF is appropriate and is used for de-noising, the characteristic numbers, including mean ( Table 1 Criteria for evaluation of the de-noising results of hydrological time series |
Wavelet de-noising is a key step in the proposed approach for wavelet choice. The wavelet threshold de-noising (WTD) method is a theoretically effective and widely-used method. It is used to estimate proper wavelet coefficient thresholds, and then adjust the detailed wavelet coefficients of the analysed series under multiple resolutions, as follows:
For details of the mathematical fundaments of WTD readers are referred to Donoho (Citation1995), Bruni and Vitulano (Citation2006), Chanerley and Alexander (Citation2007) and Sang et al. (Citation2010); and current studies and applications of WTD in time series analysis have been reviewed in Sang et al. (Citation2009a). The effectiveness of WTD in practice is influenced by four key factors (Jansen and Bultheel Citation2001), namely, choice of wavelet, choice of decomposition level, estimation of thresholds and choice of thresholding rules. Sang et al. (Citation2009a) reviewed those wavelet de-noising methods in use at present and summarized their main defects; moreover, they proposed an entropy-based WTD method, whose basic idea is to estimate proper thresholds and to determine an accurate de-noising result according to the variations of the complexities of the de-noised series and random nature of the separated noise, characterized by wavelet energy entropy and the principle of maximum entropy, respectively. This method is used herein to de-noise series and to further guide the wavelet choice.
The specific processes of choosing WBF by the proposed approach are as follows:
1. | First choose wavelets according to the above three points, and gather the results as the basic set for wavelet choice. | ||||
2. | De-noise the studied hydrological series by WTD using a certain wavelet in the basic set. | ||||
3. | Separate the main series and noise in the original series, and compute their characteristic numbers ( | ||||
4. | Repeat Step (2) for each wavelet in the basic set. | ||||
5. | Compare the results of the characteristic numbers obtained using different WBFs. The wavelet which enables the de-noising result to best match the criteria () is regarded as the most appropriate WBF, and the corresponding de-noised series is also the most reliable true signal identified in the original series. |
When analysing the hydrological characteristics of a given basin or region, we can first select several typical hydrological time series according to the basin's features: location, shape, topography, vegetation and soil type. Each is analysed according to steps (1)–(5) above, and the wavelet that is the most suitable for all these series is regarded as the best WBF, and the corresponding de-noising results are also the most reasonable.
3.2 Verification analysis
Considering that true signals in observed hydrological series are unknown, we used synthetic series to investigate the effectiveness of the proposed approach. Three generated synthetic series (S1, S2 and S3) have the same length of 500, and their true SNR (signal-to-noise ratio) values are −1.958, −7.603 and −2.819, respectively. Series S1 has two periods of 100 and 250; and for series S2, the first part (1–200) has a period of 50, and the second part (201–500) a period of 150. Both the amplitude and period of series S3 are damped. depicts the de-noising results of three synthetic series using five wavelets. The characteristic numbers of the original synthetic series, de-noised series and separated noise are computed and presented in .
Fig. 1 Three synthetic series used (upper) and their de-noising results using different wavelets (lower).
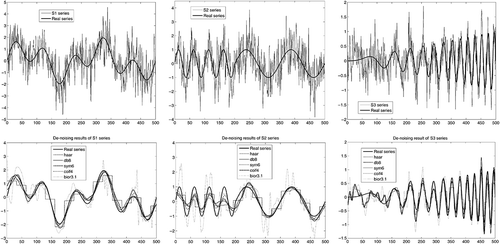
Table 2 Synthetic series used in this paper and their de-noising results using different wavelets
The results clearly indicate that the de-noising results of certain series vary with the wavelets used, and they also differ from the true series. For the S1 series example, using the Bior3.1 wavelet, the and r
1 values of the separated noise have the biggest values of 0.026 and 0.119, respectively, and using the Haar wavelet the de-noised series has very different shape from the true S1 series, so the two wavelets are unsuitable. The de-noising results of S1 series by Db8, Sym6 and Coif4 have no obvious difference. Comparatively, the result with the Sym6 wavelet is the best, because by using the Sym6 wavelet, the
value (−0.003) of the de-noised series is close to the true value, the σ value of de-noised series is the same as the true value (1.001), and the r
1 value (0.998) of the de-noised series is much bigger than that of series S1; the r
1 of separated noise (0.025) is the smallest. also shows that the de-noising results of series S1 by the Haar and Bior3.1 wavelets are the worst, but that by the Sym6 wavelet is the closest to the true series, and the SNR value (−1.967) computed by using Sym6 is close to the true SNR of −1.958. All these results prove the suitability of the chosen Sym6 wavelet for analysis of series S1. The same analyses were done to series S2 and S3, and the results indicate the same conclusions as for the S1 series. However, the earlier parts of S2 and S3 series cannot be accurately identified using any wavelets, and this is due to the edge effects of wavelet analysis.
To sum up the results in , the most appropriate wavelets for analyses of three synthetic series are Sym6, Coif4 and Db8, because by using them the true signals can be accurately identified; other wavelets cannot meet this need and thus cannot reveal the true characteristics of the series either. Moreover, the results of the three synthetic series not only demonstrate the effectiveness of the proposed approach, but also indicate that the wavelet choice is mainly determined by the true signals in the studied series. In the authors' opinion, this conclusion about wavelet choice accords with the essence of wavelet transform, so it is reasonable from the physical point of view.
4 DETERMINATION OF ANALYTIC PROCEDURE OF CWT
In addition to wavelet choice, determination of the analytical procedure of CWT is a key issue in the CWT process. Such a procedure, whose basic idea is “from global to local, and from qualitative to quantitative”, is demonstrated in and is explained as follows:
Fig. 2 Analytical process of hydrological time series by the CWT procedure proposed. CWT: continuous wavelet transform; TSR: temporal scale range; CTS: central temporal scale.
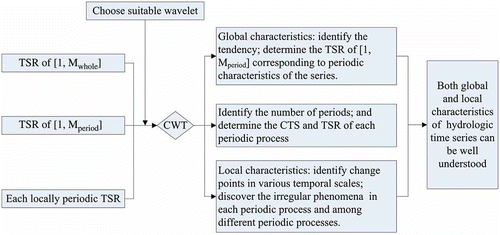
a. | Choose an appropriate WBF by the approach proposed above, and choose the temporal scale Mwhole that is about two times the length of the studied hydrological series. Then apply CWT to the series at the temporal scale range (TSR) of [1, Mwhole] and run significance testing to qualitatively reveal the global characteristics of the series: detect the tendency, and determine the TSR of [1, Mperiod] corresponding to periodic characteristics of the studied series. | ||||
b. | Apply CWT to the series again at the TSR of [1, Mperiod], and compute the WVE by Equationequation (5) | ||||
c. | Use the TSR of each period and apply CWT to the series to quantitatively reveal its local characteristics: detect the transient processes (i.e. change points) according to the variations of wavelet coefficients, identify and describe the irregular phenomena in each periodic process, and describe the variation with time of significant degree for each period. |
Summarization of the above results can reveal, and enable one to understand, both the global and local characteristics of the studied hydrological time series.
5 ANALYSIS OF VARIATION IN FMP OF THE HYDROLOGICAL SERIES
5.1 Data
Eight observed time series of runoff and precipitation data, measured at the sampling rates of day, month and year, are used as examples to discuss the phenomenon of the variation in FMP of hydrological time series. To ensure the reliability of the results and conclusions, we selected data from various basins with obviously different climatic conditions, as described in and shown on the map of China (). Specifically, the P1 and P5 series data were measured in the temperate continental monsoon climatic zone; the P2 and R1 series data in the temperate East-Asian monsoon climatic zone; the P3 and P4 series data in the subtropical climatic zone; the R2 series data in the inland climatic zone; and the R3 series data in the subtropical East-Asian monsoon climatic zone.
Table 3 The eight observed hydrological series used in this study. Precipitation series data (P1–P5) are from the China Meteorological Data Sharing Service System. Runoff series data sources: R1—Hydrology Bureau, Yellow River Conservancy Committee, Ministry of Water Resources; R2—Zuo and Gao (Citation2004); R3—National Natural Science Foundation of China
The data in indicate that the characteristics and composition of these selected hydrological series are clearly different. The variability of series P5 is the most obvious, because it has a large coefficient of variation, Cv of 3.77; the coefficient of skewness, Cs of series R3 is the largest with a value of 2.27, but the Cs of series P3 is the smallest (−0.10). The lag-1 autocorrelation coefficient, r 1 of series R1 has the highest value of 0.69, whereas the r 1 of series P4 is the smallest (0.004). Human impacts on these hydrological series are also different; for instance, human activities have great impacts on series R1 (Chen et al. Citation1996), but little impact on series R2 (Zuo and Gao Citation2004) and R3 (Sang and Wang Citation2008). Therefore, it is considered that the conclusions and findings obtained from this selection of different hydrological series are reliable and convincing.
Table 4 De-noising results of the eight hydrological series using the chosen wavelet basis functions (WBF)
5.2 Chosen wavelets
The wavelets for analyses of the eight series were chosen by the proposed approach. Calculation of characteristic numbers in and the de-noising results in indicate that results for all these series using the chosen wavelets accord well with the criteria in . The noises separated from the eight original series have r 1 values of −0.19, −0.18, −0.18, −0.21, 0.21, 0.14, −0.24 and −0.21 respectively, which indicate that they show pure random characters. Therefore, it is thought that the chosen WBFs are appropriate. As a result, the wavelets chosen for analyses of the eight series measured in various basins and at different sampling rates are the Db2, Coif3, Dmey, Db4, Db3, Db2, Bior2.4 and Sym3. In addition, their degrees of noise contamination also differ: series P2 has a minimum SNR value of −0.35, but series P5 has a maximum SNR value of 49.70. Therefore, the original and de-noised series P2 shows obvious difference, while the original and de-noised P5 series is similar. The SNR values of series P1, P3 and P4 are similar to each other with a value of about 5. The SNR values of runoff series R1, R2 and R3 are 18.43, 0.84 and 28.64, respectively.
5.3 Characteristics of series
Following the proposed analytical procedure of CWT, the characteristics of eight hydrological series at multi-temporal scales are revealed. The results in clearly show their differences, and trends are depicted in using wavelet coefficient curves at the biggest corresponding scales. The P1 series shows a falling first and then rising tendency, and two periodic variations at scales of 1–5 years and 5–10 years with central scales of 3 and 7 years, respectively. The P2 series shows a rising-falling-rising tendency, and three periodic variations at scales of 1–4 years, 4–7 years and 7–11 years with central scales of 3, 5 and 9 years, respectively. The P3 series shows a rising tendency, and three periodic variations at scales of 1–4 years, 5–10 years and 11–25 years with central scales of 2, 6 and 18 years, respectively. The P4 series shows a falling first and then rising tendency, and two periodic variations at scales of 1–5 years and 8–15 years with central scales of 2 and 10 years, respectively. The P5 series shows a rising first and then falling tendency, and two periodic variations at scales of 3–10 days and 15–20 days with central scales of 7 and 18 days, respectively. The R1 series shows a rising first and then falling tendency, and four periodic variations at scales of 1–4 years, 6–8 years, 9–12 years and 16–22 years with central scales of 3, 7, 11 and 18 years respectively. The trend of the R1 series is so strong that its periodic characteristics are not clearly presented in the global wavelet spectrum. The R2 series shows a falling first and then rising tendency, and two periodic variations at scales of 1–5 years and 7–10 years with central scales of 3 and 7 years, respectively. The R3 series shows a falling first and then rising tendency, and two periodic variations at scales of 6–15 months and 20–25 months with central scales of 12 and 24 months, respectively.
The local characteristics of the eight series are described in , using the wavelet coefficient curves at different central scales. Comparatively, the local characteristics of series R1 are the most complicated, because it has many change points and irregular variations at multi-temporal scales that are caused by the great impacts of human activities (soil-water conservation projects, utilization of water resources, operations of water reservoirs, etc.) on the Yellow River watershed (Chen et al. Citation1996); whereas series R2 only shows two simple periods of 3 years and 8 years. Common features are focused on here and details are not described. The improved understanding gained by comprehensive analyses of these results shows that the characteristics of hydrological time series are far more complicated than was previously known and described. To be specific, hydrological time series usually show both global and local characteristics; local characteristics are more complicated than global ones. For example, many change points exist at multi-temporal scales, such as one change point of the P2 series in 1987 at the scale of 7–11 years, and one change point of the R1 series in 1970 at the scale of 9–12 years. The significant degrees of many periods vary irregularly with length of the series, as shown in the variations of wavelet coefficient curves; and there are combined and cross-over phenomena among different periodic processes. However, the characteristics of hydrological series, especially complicated local characteristics, can be accurately revealed step by step following the proposed analytical procedure of CWT.
5.4 Discussion of results of the identification of periods
Based on the above CWT results of hydrological series, we investigated the variation in FMP of annual hydrological series according to the following process: (a) we first randomly set the initial length and length interval of the annual series analysed, then extended the series length gradually; (b) we identified the FMP of each extended series using different methods, and compared the results; (c) if the period results identified vary with the methods used, it means that the method is at least one of the reasons for the FMP variation; and (d) if the period results identified by different methods are just the same, it indicates that these results are accurate and reliable, i.e. not the method, but the hydrological series itself is the essential reason, and the periodic characteristics of the hydrological time series should be further studied and understood.
Three methods, CWT, MSSA and WVE, were used here to identify the periods of hydrological series. The results by CWT are described in ; MSSA is a spectral analysis method combining MESA with wavelet de-noising (Sang et al. Citation2009b); and WVE can describe the energy distribution of series and thus be used to identify dominant periods (Labat Citation2005).
Six annual hydrological series (P1, P2, P3, P4, R1, and R2) were analysed by the three methods. presents the results of identification of the periods of the series by MSSA and MVE. shows that many extended precipitation series have a similar period of about 2–3 years, which is a common period of annual precipitation in China; in addition, many extended runoff series have a similar period of about 7 years, which is a common period of runoff in the dry season in northern China. Furthermore, clearly indicates that the FMP results of any annual series of given length by the three methods are the same; for example, the FMP of the 30-year P2 series identified by MSSA is 8.8 years, the result by WVE is 9.0 years, and the CWT results in show that wavelet coefficients fluctuate between −1.5 and 1.5 in TSR of 7–11 years in 1963–1985, which are the most obvious characteristics in 1956–1985. Thus, it is thought that these results of period identification are accurate and reliable, although the physical reasons for some periods cannot be understood clearly. However, the identified FMPs vary with annual series length. We take the P2 series as an example again; the FMP of the extended P2 series with lengths of 20 and 35 years is about 3 years; for lengths of 25 and 30 years it is about 9 years; but for lengths of 40, 45 and 47 years it is about 5 years. The conclusions from the results of other annual series are similar to those of the P2 series.
Table 5 The first main periods of six annual hydrological series with different lengths identified by the main series spectral analysis method (MSSA) and wavelet variance estimator (WVE)
By comprehensive analyses of the results in and , it was found that the variations of FMP accord well with the local characteristics of annual hydrological series. To be specific, the absolute values of wavelet coefficients determine the significant degrees of periodic characteristics of a hydrological series at a given temporal scale, while the variations with time in the absolute values of wavelet coefficients result in variations in the dominant periods of the hydrological series. Therefore, one conclusion can be that the variation in FMP truly reflects the complicated local characteristics of the hydrological time series. In addition, the periodic characteristics of the daily P5 series and the monthly R3 series at multi-temporal scales also vary with series length, which further indicates that not only the FMP of annual hydrological time series, but the variation in periods is a common phenomenon in hydrological processes.
From the above discussion of the results, it should be realized that the traditional and prevailing practices in period identification, which regard the dominant periods of hydrological series as constants and then identify them by certain effective methods, are not sufficient and also may be unreasonable. In future, more research is needed to reveal and describe the variation of periods to further understand the complicated hydrological processes.
6 CONCLUSIONS
In this paper, we first made improvements on CWT by studying the two key issues of wavelet choice and determination of the analytical procedure of CWT. The improved CWT method was then applied to several typical hydrological series to investigate the variation in dominant periods of hydrological series. From the results, the following understandings and conclusions have been drawn:
1. | The wavelet chosen by the proposed approach can meet the need of accurately identifying true signals in observed hydrological series; therefore, by using the chosen wavelet, the characteristics and components of a hydrological series can also be accurately revealed. | ||||
2. | By using the improved CWT method, the characteristics of hydrological time series, especially complicated local characteristics, can be revealed step-by-step and be accurately understood. | ||||
3. | Hydrological processes in nature usually show multi-temporal scale characteristics. These include both global and local characteristics; the local characteristics, being more complicated and difficult to describe than the global ones, should be studied in more detail. | ||||
4. | The variation in dominant periods of hydrological series is a very common phenomenon, and reflects the highly complicated local characteristics of hydrological processes. Therefore, traditional practices in period identification should be better understood and developed. |
More attention should still be paid to the following two issues when coping with hydrological series analysis by wavelet analysis. The first issue is that further studies are needed on wavelet analysis, which has the advantage of analysing the time series with non-stationary characteristics, and is thus very suitable for hydrological series analysis. In this study two key issues on CWT were investigated. However, many other key issues that relate to wavelet decomposition, wavelet de-noising and so on, still need study. To further improve the WA method, further studies should be conducted applying WA to various practical cases. The second issue is the description and simulation of the local characteristics of hydrological series. Local characteristics cause the variation in dominant periods, and may further cause difficulty in hydrological forecasting. Whenever they are greatly affected by climatic change and human activities, local characteristics of hydrological series (such as the R1 series) are more complicated. In this study, we mainly focus on revealing such local characteristics. While this may provide useful guidance in practical scenarios, a more effective approach to describe and simulate local characteristics should be the focus of future research. In the authors' opinion, only when the physical mechanisms of local characteristics have been determined and clearly understood, can they be described and simulated accurately.
Acknowledgements
The authors gratefully acknowledge the most appropriate comments and suggestions given by Co-editor, Demetris Koutsoyiannis, and the anonymous reviewers. The authors also thank Ms Fei-Fei Liu and Ms Die Zhu for their assistance in preparation of the manuscript. This study was supported by the National Natural Science Foundation of China (nos 41201036, 41071018 and 41030746).
REFERENCES
- Blanco , S. 1998 . Time-frequency analysis of electroencephalogram series. III. Wavelet packets and information cost function . Physical Review, E , 57 : 932 – 940 .
- Blazkova , S. and Beven , K. 1997 . Flood frequency prediction for data limited catchments in the Czech Republic using a stochastic rainfall model and TOPMODEL . Journal of Hydrology , 195 : 256 – 278 .
- Box , G.E.P. , Ienkins , G.M. and Reinsel , G.C. 1994 . Time series analysis: Forecasting and control. 3rd edition , Englewood Cliffs , NJ : Prentice Hall .
- Bruni , V. and Vitulano , D. 2006 . Wavelet-based signal de-noising via simple singularities approximation . Signal Processing , 86 : 859 – 876 .
- Cahill , A.T. 2002 . Determination of changes in streamflow variance by means of a wavelet-based test . Water Resources Research , 38 : W01065
- Chanerley , A.A. and Alexander , N.A. 2007 . Correcting data from an unknown accelerometer using recursive least squares and wavelet de-noising . Computer Structure , 85 : 1679 – 1692 .
- Chen , X.D. 1996 . Yellow river hydrology (in Chinese) , Zhengzhou : Water Conservancy of the Yellow River .
- Chui , C.K. 1992 . An introduction to wavelets , San Diego , CA : Academic Press .
- Coulibaly , P. and Burn , D.H. 2004 . Wavelet analysis of variability in annual Canadian streamflows . Water Resources Research , 40 : W03105
- Daubechies , I. 1990 . The wavelet transform time-frequency localization and signal analysis . IEEE Transactions on Information Theory , 36 : 961 – 1004 .
- Daubechies , I. 1992 . Ten lectures on wavelets . Society for Industrial and Applied Mathematics , : 357
- Donoho , D.H. 1995 . De-noising by soft-thresholding . IEEE Transactions on Inform Theory , 41 : 613 – 617 .
- Fleming , S.W. 2002 . Practical applications of spectral analysis to hydrological time series . Hydrological Processes , 16 : 565 – 574 .
- Gaucherel , C. 2002 . Use of wavelet transform for temporal characterization of remote watersheds . Journal of Hydrology , 269 : 101 – 121 .
- Hanson , R.T. , Newhouse , M.W. and Dettinger , M.D. 2004 . A methodology to assess relations between climatic variability and variations in hydrological time series in the southwestern United States . Journal of Hydrology , 287 : 252 – 269 .
- Heil , C.E. and Walnut , D.F. 1989 . Continuous and discrete wavelet transforms . SIAM Review , 31 : 628 – 666 .
- Jansen , M. and Bultheel , A. 2001 . Asymptotic behavior of the minimum mean squared error threshold for noisy wavelet coefficients of piecewise smooth signals . IEEE Transactions on Signal Processes , 49 : 1113 – 1118 .
- Katz , R. 2002 . Techniques for estimating uncertainty in climate change scenarios and impact studies . Climate Research , 20 : 167 – 185 .
- Kayhan , A.S. 1999 . Evolutionary maximum entropy spectral analysis of chirps in noise . Signal Processing , 78 : 151 – 157 .
- Kottegoda , N.T. , Natale , L. and Raiteri , E. 2007 . Gibbs sampling of climatic trends and periodicities . Journal of Hydrology , 339 : 54 – 64 .
- Kwon , H.H. 2006 . Episodic inter-annual climate oscillations and their influence on seasonal rainfall in the Everglades National Park . Water Resources Research , 42 : W11404
- Labat , D. 2005 . Recent advances in wavelet analyses: Part 1. A review of concepts . Journal of Hydrology , 314 : 275 – 288 .
- Mallat , S.G. 1989 . A theory for multiresolution signal decomposition: the wavelet representation . IEEE Transactions on Pattern Analysis and Machine Intelligence , 11 : 674 – 693 .
- Matalas , N.C. 1967 . Mathematical assessment of synthetic hydrology . Water Resources Research , 3 : 937 – 945 .
- Meyer , Y. 1992 . Wavelet and applications , Berlin : Springer .
- Neupauer , R.M. 2006 . Characterization of permeability anisotropy using wavelet analysis . Water Resources Research , 42 : W07419
- Padmanabhan , G. and Rao , A.R. 1988 . Maximum entropy spectral analysis of hydrological data . Water Resources Research , 24 : 1519 – 1533 .
- Pelgrum , H. 2000 . Length-scale analysis of surface albedo, temperature, and normalized difference vegetation index in desert grassland . Water Resources Research , 36 : 1757 – 1765 .
- Percival , D.B. and Walden , A.T. 2000 . Wavelet methods for time series analysis , Cambridge , , UK : Cambridge University Press .
- Ravines , R.R. 2008 . A joint model for rainfall–runoff: the case of Rio Grande basin . Journal of Hydrology , 353 : 189 – 200 .
- Sang , Y.F. and Wang , D. 2008 . Wavelets selection method in hydrologic series wavelet analysis (in Chinese with English abstract) . Journal of Hydraulic Engineering , 39 : 295 – 300, 306 .
- Sang , Y.F. , Wang , D. and Wu , J.C. 2010 . Entropy-based method of choosing the decomposition level in wavelet threshold de-noising . Entropy , 12 : 1499 – 1513 .
- Sang , Y.F. 2009a . Entropy-based wavelet de-noising method for time series analysis . Entropy , 11 ( 4 ) : 1123 – 1147 .
- Sang , Y.F. 2009b . The relation between periods’ identification and noises in hydrologic series data . Journal of Hydrology , 368 : 165 – 177 .
- Sang , Y.F. , Wang , Z.G. and Liu , C.M. 2012a . Discrete wavelet-based trend identification in hydrologic time series . Hydrological Processes , doi: 10.1002/hyp.9356
- Sang , Y.F. , Wang , Z.G. and Liu , C.M. 2012b . Period identification in hydrologic time series using empirical mode decomposition and maximum entropy spectral analysis . Journal of Hydrology , 424 : 154 – 164 .
- Schaefli , B. , Maraun , D. and Holschneider , M. 2007 . What drives high flow events in the Swiss Alps? Recent developments in wavelet spectral analysis and their application to hydrology . Advance in Water Resources , 30 : 2511 – 2525 .
- Seungho , H. 2005 . Temporal analysis of the frequency and duration of low and high streamflow: years of record needed to characterize streamflow variability . Journal of Hydrology , 310 : 78 – 94 .
- Smith , L.C. , Turcotte , D.L. and Isacks , B.L. 1998 . Stream flow characterization and feature detection using a discrete wavelet transform . Hydrological Processes , 12 : 30 – 56 .
- Torrence , C. and Compo , G.P. 1998 . A practical guide to wavelet analysis . Bulletin of the American Meteorological Society , 79 : 61 – 78 .
- Walker , J.S. 1999 . A primer on wavelets and their scientific applications , New York : Chapman and Hall, CRC .
- Yevjevich , V. 1972 . Stochastic processes in hydrology , Littleton , CO : Water Resources Publications .
- Yevjevich , V. 1987 . Stochastic models in hydrology . Stochastic Hydrology and Hydraulics , 1 : 17 – 36 .
- Zuo , Q.T. and Gao , F. 2004 . “ Periodic overlapping prediction model and its three improved models of hydrological time series (in Chinese with English abstract) ” . In Journal of Zhengzhou University (Engineering Science) Vol. 25 , 67 – 73 .