Abstract
Quantifying the impacts of climate change on the hydrology and ecosystem is important in the study of the Loess Plateau, China, which is well known for its high erosion rates and ecosystem sensitivity to global change. A distributed ecohydrological model was developed and applied in the Jinghe River basin of the Loess Plateau. This model couples the vegetation model, BIOME BioGeochemicalCycles (BIOME-BGC) and the distributed hydrological model, Water and Energy transfer Process in Large river basins (WEP-L). The WEP-L model provided hydro-meteorological data to BIOME-BGC, and the vegetation parameters of WEP-L were updated at a daily time step by BIOME-BGC. The model validation results show good agreement with field observation data and literature values of leaf area index (LAI), net primary productivity (NPP) and river discharge. Average climate projections of 23 global climate models (GCMs), based on three emissions scenarios, were used in simulations to assess future ecohydrological responses in the Jinghe River basin. The results show that global warming impacts would decrease annual discharge and flood season discharge, increase annual NPP and decrease annual net ecosystem productivity (NEP). Increasing evapotranspiration (ET) due to air temperature increase, as well as increases in precipitation and LAI, are the main reasons for the decreasing discharge. The increase in annual NPP is caused by a greater increase in gross primary productivity (GPP) than in plant respiration, whilst the decrease in NEP is caused by a larger increase in heterotrophic respiration than in NPP. Both the air temperature increase and the precipitation increase may affect the changes in NPP and NEP. These results present a serious challenge for water and land management in the basin, where mitigation/adaption measures for climate change are desired.
Editor Z.W. Kundzewicz; Associate editor D. Yang
Citation Peng, H., Jia, Y.W., Qiu, Y.Q., and Niu, C.W., 2013. Assessing climate change impacts on the ecohydrology of the Jinghe River basin in the Loess Plateau, China. Hydrological Sciences Journal, 58 (3), 651–670.
Résumé
Il est important de quantifier les impacts du changement climatique sur l'hydrologie et les écosystèmes du plateau de Loess en Chine, qui est bien connu pour ses taux d’érosion élevés et de la sensibilité de ses écosystèmes aux changements globaux. Un modèle distribué écohydrologique a été développé et appliqué dans le bassin de la rivière Jinghe du plateau de Loess. Ce modèle couple le modèle de végétation « Biome BioGeochemicalCycles » (BIOME-BGC), et le modèle hydrologique distribué « Processus de transfert d'eau et d’énergie dans les bassins de grands fleuves » (WEP-L). Le modèle WEP-L fournit les données hydro-météorologiques à BIOME-BGC, et les paramètres de végétation du WEP-L ont été mis à jour au pas de temps journalier par BIOME-BGC. Les résultats de la validation du modèle sont en bon accord avec les données d'observation sur le terrain et les valeurs de la littérature de l'indice de surface foliaire (ISF), de la productivité primaire nette (PPN) et du débit des rivières. Les projections climatiques moyennes de 23 modèles climatiques globaux (MCG), basées sur trois scénarios d’émissions, ont été utilisées dans les simulations pour évaluer les futures réponses écohydrologiques dans le bassin de la rivière Jinghe. Les résultats montrent que les effets du réchauffement climatique réduiraient les débits annuels et saisonniers, augmenteraient la PPN annuelle et réduiraient la productivité nette de l’écosystème (PNE). L'accroissement de l’évapotranspiration (ET) causée par l'augmentation de température de l'air, ainsi que l'augmentation des précipitations et de l'ISF, sont les principales raisons de la diminution du débit. L'augmentation de la PPN annuelle est causée par une augmentation plus importante de la productivité primaire brute (PPB) que de celle de la respiration des plantes, tandis que la diminution de la PNE est causée par une augmentation plus importante de la respiration hétérotrophe que de celle de la PPN. Tant l'augmentation de la température de l'air que l'augmentation des précipitations peuvent influencer les changements de PPN et de PNE. Ces résultats présentent un sérieux défi pour la gestion des eaux et des terres dans le bassin, où des mesures d'atténuation et d’ adaptation aux changements climatiques sont souhaitées.
INTRODUCTION
Ecohydrology is an interdisciplinary subject that focuses on interactions between ecosystems and hydrological processes (Baird and Wilby Citation1999, Zalewski Citation2002). It is likely that future climate change, such as changes of temperature, precipitation and the frequency of extreme weather will influence ecohydrological processes. The water distribution in different ecosystems will be more variable. Plant water use and the ecosystem carbon cycle will be affected by climate change. Quantifying the impacts of climate change on hydrology and ecosystems still represents a challenge in global change studies.
Ecohydrological models can serve as useful tools for forecasting the impacts of climate change. Some of the distributed hydrological models (Engel et al. Citation1993, Jia and Tamai Citation1998, Andersen et al. Citation2001) involve ecohydrological processes. For example, MIKE-SHE has a module to simulate soil and crop parameters, and, in SWAT, all plants are simplified to simulate them as one kind of entity. These ecohydrological simulations are too simple to reflect real-world processes. However, ecosystem models, such as CENTURY (Parton et al. Citation1993), BIOME-BGC (Running and Coughlan Citation1988, Running and Hunt Citation1993, Wang et al. Citation2005), LPJ (Sitch et al. Citation2003) and TEM-LPJ (Pan et al. Citation2002), have been developed to simulate the energy and material exchange of terrestrial ecosystems. Coupling ecosystem models with distributed hydrological models is one of the research directions for future ecohydrological studies (Walko et al. Citation2000, Sitch 2003, Morales et al. Citation2005, Govind Citation2009). Some ecohydrological models have been developed to represent feedbacks between hydrological and vegetation processes, such as RHESSys (Band et al. Citation1993, Citation1996, Tague and Band Citation2004, Tague et al. Citation2004). However, most of these studies have been conducted in either small basins or at a global scale. Study of large basins (over 10 000 km2) is needed to support regional climate change research.
In climate change impact studies, projections by global climate models (GCM) and regional climate models (RCM) have been widely used. Many studies have used projections by GCM and RCM as input to hydrological or ecosystem models to forecast climate change impacts on hydrology and ecosystems (White et al. Citation1999, Cramer et al. Citation2001, Prudhomme et al. Citation2003, Vicuna and Dracup Citation2007). However, in these studies, the results show various impacts of climate change due to the different features of each region and the uncertainties of the GCM and RCM projections. Therefore, the climate change impacts need to be assessed for a specific region, and the uncertainties of the GCM or RCM projections need to be reduced in the studies.
The Loess Plateau, known for its highly erodible soil and fragile ecosystem, covers an area of over 640 000 km2 in the upper and middle reaches of the Yellow River in China. Centuries of deforestation and intensive agriculture have resulted in the deterioration of ecosystems and desertification. Some investigations have analysed the ecohydrological situation of the Loess Plateau, including the status of the vegetation and ecohydrology, statistical relationships between vegetation and precipitation or runoff (Suo et al. 2005, Chen et al. Citation2008, Qi et al. Citation2009), and regional net primary productivity (NPP) analysis (Xu et al. Citation2005, Mo et al. Citation2007, Song et al. Citation2009). However, prediction of future climate change impacts on ecohydrology in large basins (>10 000 km2), which is needed for ecosystem and water resources protection in this region, is limited.
In order to predict the ecohydrological impacts of climate change in the Loess Plateau, this paper presents an ecohydrological model that couples a distributed hydrological model, Water and Energy transfer Process in Large river basins (WEP-L), with a terrestrial ecosystem model, the vegetation model BIOME BioGeochemicalCycles (BIOME-BGC), using the Jinghe River basin as a case study. The Jinghe basin (approx. 45 000 km2) is a typical large basin within the Loess Plateau. Global climate model climate projections from the World Climate Research Programme's (WCRP) Coupled Model Intercomparison Project phase 3 (CMIP3) multi-model data set were averaged to deduce the uncertainties, and the averaged values were used as the climate inputs to the ecohydrological model. The prediction results of the model were used to address two questions: (a) How will annual discharge, flood season discharge, annual NPP and net ecosystem productivity (NEP) change under different future emissions scenarios? and (b) What will cause these changes? The prediction results and analysis could provide technological support to the planning of agriculture, soil and water conservation, and other activities in the Loess Plateau that are of critical significance and application value to China.
SITE DESCRIPTION
The Jinghe River is 455 km long, the second-longest tributary of the Weihe River, and a second-level tributary of the Yellow River. The Jinghe basin (area 45 421 km2) is located in the middle of the Loess Plateau (106°20′–108°48′E, 34°24′–37°48′N); 4.3% of the area is mountainous, 41.7% comprises loess tableland and broken plateau areas, and 48.8% loess hilly and gully regions. A digital elevation model (DEM) and the rivers in the basin are shown in . This basin is one of the most soil-eroded areas of the Loess Plateau; it has deep (50–80 m) layers of loess. The particle-size composition of the loess is mainly fine sand, silt and clay, with silt accounting for up to 50%. The loess has high porosity and is prone to landslides. The soil is typical black loam soil with a loose structure that is readily degraded.
The land-use types of the basin are farmland, forest, grass, water, wasteland, roadways and residential land, of which farmland, forest and grass form the main land covers. The vegetation of the basin is temperate forest–steppe transition type. Due to historical development processes, human activity has destroyed much of the vegetation, and now degraded grassland covers much of the area. The growing season is between April and October.
The basin spans the provinces of Ningxia, Gansu and Shanxi, and includes seven cities. The population of six million people inhabits mainly the middle and downstream portions of the basin. Water scarcity and soil desertification have resulted in low levels of agriculture and animal husbandry. The industrial structure of the basin is resource-oriented, with energy, heavy and chemical industries accounting for more than 90% of the total industrial production.
The basin has a typical continental climate and is located between the semi-humid and semi-arid temperate zones. It is dry in winter and spring, and storms are frequent in summer and autumn. The average air temperature is 8°C. The average air temperature of the coldest months is between –8°C and –10°C, while that of the warmest months is 22–24°C; the frost-free period is 150 days long. Annual precipitation ranges between 350 and 600 mm; the summer precipitation accounts for more than 50% of annual precipitation and is intense, and inter-annual variations in precipitation are great. Temperature and precipitation in the south are higher than in the north.
METHODS AND MODELLING
The BIOME-BGC model, developed by the Numeric Terradynamic Simulation Group of the University of Montana, USA, and the WEP-L model developed by Jia et al. (Citation2004) were selected to construct the ecohydrological model. The BIOME-BGC model was selected due to its detailed simulation of the water, carbon and nitrogen cycles within ecosystems, while the WEP-L model was selected for its good performance in hydrological simulation of large basins.
BIOME-BGC model
The BIOME–BGC is a biogeochemical model that can simulate energy, water, carbon and nitrogen fluxes and storage in the plants and soil of terrestrial ecosystems. Processes simulated in the model include photosynthesis, respiration, evapotranspiration and nitrogen mineralization (Running and Hunt Citation1993).
The BIOME-BGC model simulates daily and annual processes. Daily processes related to water flux include interception, evaporation, transpiration, infiltration and outflow (interception is ignored in snow processes). Daily processes related to the carbon cycle include photosynthesis, leaf, stem and root respiration, and respiration of soil and leaf litter (Running and Hunt Citation1993).
The model simulates annual processes including storage and allocation of free carbon, and allocation and loss of free nitrogen. The allocation of carbon content and metabolism takes place among the leaves, stems and roots. Free nitrogen is allocated between the soil and litter. Loss of nitrogen occurs due to litter decomposition and leaching.
There are 34 parameters in the model, categorized into several groups: turnover and mortality; allocation of photosynthetically accumulated carbon; carbon to nitrogen ratios; percentages of lignin; cellulose and labile material in fine roots; leaves and dead wood; tree morphological parameters; ecophysiological parameters; water interception; canopy radiation absorption; and rate of carbon assimilation (White et al. Citation2000). In BIOME-BGC, plants are categorized into seven types: evergreen broadleaf forest; evergreen needle leaf forest; deciduous broadleaf forest; deciduous needle leaf forest; shrub; C3 grass and C4 grass (Running and Hunt Citation1993). BIOME-BGC can calculate and output information on plant growth and the carbon cycle at daily, monthly and annual time scales; the output includes leaf area index (LAI); evapotranspiration (ET); net primary productivity (NPP); net ecosystem productivity (NEP); gross primary productivity (GPP); maintenance respiration (R maint); growth respiration (R growth); and heterotrophic respiration (R heterotr).
WEP-L model
The distributed hydrological model WEP-L was developed from the WEP model, which has been successfully applied to several watersheds in Japan, Korea and China with different climatic and geographic conditions (Jia and Tamai Citation1998, Jia et al. Citation2001, Citation2002, Citation2004, Citation2005, Citation2006, Kim et al. Citation2005, Qiu et al. Citation2006). Compared to WEP, WEP-L is more efficient when applied to larger basins. The simulated hydrological processes include snowmelt, evapotranspiration, infiltration, surface runoff, overland flow, subsurface runoff, groundwater flow, river flow routing and water use. The simulated energy transfer processes include short-wave radiation, long-wave radiation, latent heat flux, sensible heat flux and soil heat flux. The modelling approaches adopted for the hydrological and energy processes are referred to in Jia et al. (Citation2001, Citation2006).
The WEP-L model couples the water cycle with energy transfer processes. Varied time intervals were adopted for each element of the hydrological cycle to ensure both rational description of dynamic mechanisms and efficient calculation. A contour band within each sub-basin was used as the calculation cell. The mosaic method is applied to take into consideration the unevenness of land use in the calculation cell, classifying land into bare land-vegetation, irrigated farmland, non-irrigated farmland, waters and impermeable areas to allow calculation of the water–heat flux through the land surface. The bare land-vegetation area is further subdivided into bare land, grassland and woodland. The impermeable area is further divided into urban land and urban buildings.
From top to bottom, the layers in the vertical structure of WEP-L ((a)) include an interception layer representing interception by vegetation and/or buildings, a storage layer representing storage in ground surface depressions, a topsoil layer, a transition layer, a shallow groundwater layer and a deep groundwater layer. The main state variables include interception by vegetation, storage in ground depressions, soil moisture content, temperature of the ground surface, storage in a transition layer, groundwater table and stream level. The soil layer is divided into three layers in order to reflect changes in soil moisture content with depth, and to describe evaporation from the soil and water absorption by root systems of grass, crops and trees. The snow storage and melting processes are simulated using the temperature-index approach (Maidment Citation1992, Jia et al. Citation2009) on a daily basis.
Fig. 2 Schematic illustration of the WEP-L model structure: (a) vertical structure within a contour band, and (b) horizontal structure within a sub-basin.
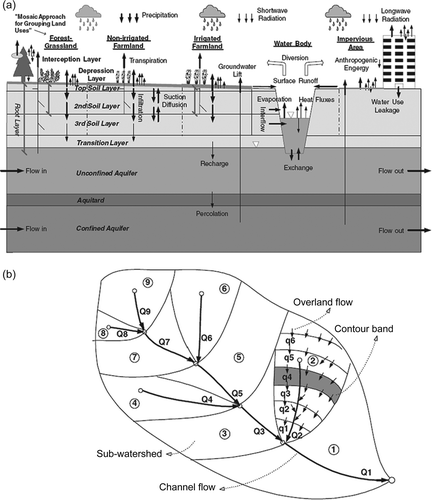
The horizontal structure of WEP-L is shown in (b). Flow confluence processes on slopes are calculated based on elevation, gradient and Manning roughness (average of various types of land uses) in every elevation band by using a one-dimensional (1D) kinematic wave approach. Flows in river channels are calculated by a 1D dynamic wave approach when downstream boundary conditions are available, or by a kinematic wave approach when downstream boundary conditions are not available. Groundwater flows are calculated by separate numerical analyses for the mountain and plain areas, and involve the exchange of groundwater with surface water, soil moisture and streamflow.
Model coupling
The BIOME-BGC model is a point simulation model. For its application in large basins, the model needed to be modified to simulate multiple units at one time. Thus, the main program of the model was rewritten to realize multi-unit simulation.
For the coupling, BIOME-BGC shared the calculation units for sub-basins with the WEP-L model. To represent the variation in land use and plant types, a mosaic approach was used to categorize land into seven types: bare land, grass, forest, irrigation farmland, non-irrigation farmland, waters and impervious land. The simulation was carried out and the average output of each unit was calculated by area. In the coupling process, the BIOME-BGC model provided ecological parameters, such as LAI, while the WEP-L model provided point information. The BIOME-BGC model simulated grasses and forests in every unit, and then transformed the outputs to WEP-L to reflect the influence of the ecosystem on the hydrological processes.
The simulation procedures of the coupled model are as follows:
a. | collection of hydrology, meteorology, geography, economic and societal data; | ||||
b. | database development based on GIS and input data preparation; | ||||
c. | sub-basin delineation and coding; | ||||
d. | physical parameter determination; | ||||
e. | BIOME-BGC model simulation and plant parameter output; | ||||
f. | transferring plant parameters and their input into WEP-L; and | ||||
g. | WEP-L model simulation and verification. |
The flowchart of the model is shown in .
Table 1 List of the collected basic data on which the model input is based
Data collection and parameter preparation
Field observation data The field observation points are located in the Nanxiaohegou catchment, a sub-basin of the Jinghe basin (location and DEM shown in ). The meteorological and hydrological data of the catchment have been collected for more than 50 years by the Xifeng Soil and Water Conservation Station, in Qingyang City, Gansu Province. The catchment is located at the periphery of the Dongzhi tableland and has the typical geomorphology of the gully region of the Loess Plateau. Annual precipitation in the basin is 523.4 mm, the annual average temperature is 8.7°C and the annual evaporation is 1474.6 mm. The basin is within the warm temperate forest-steppe vegetation zone. Common plants are Robinia pseudoacacia, Prunus armeniaca, Platycladus orientalis, Pinus tabulaeformis Carr., Populus, Salix babylonica, Sophora viciifolia Hance, Hippophae rhamnoides, Malus pumila Mill. and Medicago sativa. Due to its topography, geology, vegetation, soil, watershed morphology, hydrological characteristics, soil erosion and other natural conditions, the Nanxiaohegou catchment represents the Jinghe River basin well.
Plots of representative plants (Robinia pseudoacacia, Pinus tabulaeformis Carr. and grasses) were selected in the basin. Photosynthesis and LAI were recorded for Robinia pseudoacacia. Leaf area indices were noted for Pinus tabulaeformis Carr, and the product of grass LAI was measured. The portable photosynthesis system LI-6400 was used to record photosynthesis during cloudless days in July 2009. Observations were carried out every two hours from 08:00 to 18:00 h. A LI-2000 canopy analyser was used to record LAI observations in the evening, when there was no direct sunlight. The observations were carried out every 10 days from July to September 2009. Grass cover within 1 m2 plots was harvested from a sunny slope, and then dried and weighed in order to measure grass production.
Data from existing databases and literature The data collected from existing databases and the literature include: (a) hydro-meteorology; (b) land-cover information including land use, vegetation, soil and water conservation and crop patterns; (c) topography, soil and hydrogeology; (d) river networks, river sections and hydraulic structures (dams/reservoirs); (e) water-use and socio-economic data (Jia et al. Citation2006); and (f) CO2, as detailed in .
The hydro-meteorological data come from two sources: the National Climate Bureau of China (NCBC) and the Yellow River Water Conservancy Commission (YRWCC). The NCBC provided the meteorological data of key national meteorological stations with rain/snow, air temperature, sunshine hours, vapour pressure/relative humidity and wind speed on a daily basis. The YRWCC provided daily rainfall, hourly rainfall and river discharge data. Both data sets have been subject to the strict quality control procedures of the originating organizations and thus are highly accurate. The river discharge data provided by the YRWCC include observed and naturalized monthly data from 1956 to 2000 (Jia et al. Citation2006).
Land-use data for five periods (1960s, 1970s, 1980s, 1990s and 2000) were obtained using Landsat TM data and statistical data in the yearly reports from administrative districts, and a groundtruth investigation demonstrates that these data have an accuracy of 94% (Jia et al. Citation2006). Monthly vegetation information (area fraction of vegetation) from 1982 to 2000 was obtained using the NOAA-AVHRR data. Irrigation districts data and crop patterns were from Introduction to irrigation districts in the Yellow River Basin and Map collections of the Yellow River Basin, both compiled by the YRWCC (Jia et al. Citation2006).
Topographic data were obtained from the global DEM (GTOPO30) data developed by the US Geological Survey's EROS Data Center, and downloaded from: http://edcdaac.usgs.gov/gtopo30/gtopo30.asp The data in and around the Jinghe basin were transformed to 1 km × 1 km using the Albers coordinates projection. The soil data, including the map of soil types and corresponding characteristic parameters, are from the National Second Soil Survey Data and Soil Types of China. The soil map scale is 1:100 000. The thickness and composition of every soil type were obtained from the statistical profiles data in the Soil Types of China. Aquifer hydrogeological data, including hydrogeological unit subdivisions, permeability and storage coefficients, were taken from the Distribution map of hydrogeology in China and the Second countrywide comprehensive water resources planning in China (Jia et al. Citation2006).
Surveyed river networks were obtained from the National Geography Database at a scale of 1:250 000, and were combined with the above DEM to carry out the basin subdivisions and codification. Surveyed shape data were collected for flow routings. In addition, large numbers of silt arresters were considered for the soil and water conservation, and data for reservoirs were collected. The collected data include location data (coordinates) based on the Map collections of the Yellow River Basin compiled by the YRWCC; reservoir initial operation dates, water level–volume–area curves, characteristic volumes and water levels, sedimentation and storage variation series, and operation rules; and water supply purposes and geographic areas (Jia et al. Citation2006).
Water-use and socio-economic data for the Jinghe basin were obtained from the Second countrywide comprehensive water resources planning in China. The statistical units of the data are the national administrative prefecture or municipality. The water-use data include water supply to, and water consumption by five sectors (industrial; farmland irrigation; forest, grassland and fishery water use; urban daily life; and rural daily life) with different sources (surface water; shallow groundwater; and deep groundwater) and monthly water-diversion processes in some representative districts.
Data preparation to run WEP-L included: (a) stream network generation, basin subdivision and coding, determination of computation units and flow routing sequence; (b) treatment of river section and reservoir data; (c) treatment of land cover information including land use, soil, hydrogeology, vegetation, reservoirs/dams, lakes, rivers, irrigation systems and soil conservation measures; (d) spatial and temporal interpolation of meteorological data including rainfall; (e) spatial and temporal interpolation of social and economic data, such as population, GDP, irrigation area and grain production, and water-use data; and (f) feature tables of sub-watersheds, stream links and computation units (contour bands). Remote sensing data (Landsat TM and AVHRR) and GIS (ArcGIS) were utilized to accomplish the data preparation (Jia et al. Citation2006).
Table 2 Main parameters of the WEP-L model
Model parameter estimation
The BIOME-BGC model is based on plant physiological processes, and the physiological parameters are important in the simulation. Due to the difficulty in acquiring actual parameters, most of those used in this study were taken from White et al. (Citation2000). Given the vegetation of the Jinghe basin, the parameters for deciduous broadleaf forest were used in forest land cover and the parameters of C3 grass were used for grass land cover. Some highly sensitive parameters (White et al. Citation2000) were calibrated according to field observations (see following section). Other parameters were set to the default parameters suggested by NTSG; all the default parameters can be found at: http://www.ntsg.umt.edu/project/biome-bgc
The WEP-L model parameters can be grouped into three categories: (a) parameters of the land surface and river channel system; (b) vegetation parameters; and (c) soil and aquifer parameters. Because WEP-L is physically-based, most of the parameters have physical meaning. All of the parameters were estimated based on land-cover information, observation data and remote sensing data, and some were selected for model calibration (see following section). The parameters of the WEP-L model are given in . Estimation of the main parameters is briefly explained below.
The land surface and river channel parameters include the maximum depression storage depth of the land surface, permeability of riverbed material, impervious ratios of residential and industrial areas, and Manning roughness for overland and river channel flows. The land surface maximum depression storage depth was set based on the literature (Black Citation1991, David et al. Citation2001, He et al. Citation2002) and calibration. Manning roughness of overland flow was set on the basis of land-use type, and the Manning roughness of a contour band was taken as the harmonic average of the values of different land uses. Manning roughness values of some representative river channel sections were obtained by the inverse simulation of observed floods, and those used for the remaining sections are referred to in Wang and Li (Citation2002).
The required vegetation parameters include vegetation fraction, maximum interception depth, LAI, aerodynamic resistance, canopy resistance and root distribution parameters. Vegetation fraction was estimated from NOAA-AVHRR remote sensing data with a temporal resolution of 10 days and a spatial resolution of 8 km × 8 km. In this study, the data were first interpolated to 1 km × 1 km data, and then the NDVI and vegetation fraction were deduced from the CHI and CH@ data of NOAA-AVHRR. The LAI is calculated by the BIOME-BGC model. The remaining parameters are taken from Dickinson et al. (Citation1991) and Jia et al. (Citation2001).
The soil and aquifer parameters include soil-layer thickness, soil porosity, soil saturated hydraulic conductivity, soil suction at infiltration wetting front, a soil moisture–hydraulic conductivity relationship curve, thickness and hydraulic conductivities of unconfined and confined aquifers, specific yield of unconfined aquifers, and storage coefficient of confined aquifers. Based on the soil texture information (percentages of sand, loam and clay), 11 soil types were identified initially; these were grouped into four categories: sand, loam, clay loam and clay. The soil experimental results in the Loess Plateau (Yang and Shao Citation2000) in the basin were referred to for soil parameter estimation. The aquifer thickness, hydraulic conductivity and storage coefficient parameters were based on the hydrogeology data of the Second countrywide comprehensive water resources planning in China. Aquifer hydraulic conductivity was calibrated in the model simulations.
Snowmelt parameters, including the degree-day factor and the threshold temperature for melt were taken from Maidment (Citation1992) and adjusted after model calibration. The degree-day factor was set as 1, 2, 3, 5 and 2 mm °C−1 d−1 for forest, grassland, bare soil, urban area and farmland, respectively. The threshold temperature for snow melt is 0°C for all land uses.
Model calibration and verification
Calibration of BIOME-BGC White et al. (Citation2000) analysed the sensitivity of the BIOME-BGC model parameters and identified those that are very sensitive. Some of these were calibrated in this study using field observation data from Nanxiaohegou basin, a sub-basin of the Jinghe basin. Forest physical parameters were calibrated based on photosynthesis and LAI observations (). The physical parameters of grasses were calibrated according to grass production observations ().
Table 3 Physical parameters of forests and grasses
The comparison of the simulation and observation results for LAI is shown in . The simulation results mostly match the observation values, but not in and after September when the simulated values are too high.
Table 4 Comparison of the simulation and observation results for LAI
shows the simulated and observation values of daily NPP. The simulation results are higher than the observation values, because the observations missed the photosynthesis that occurred before 08:00 h, when photosynthesis is intense in summer. However, the January–August 2009 cumulated simulated NPP is 87.2 g C m−2, compared with 78.0 g C m−2 according to observations—an error of less than 20%, which is acceptable.
Table 5 Comparison of simulation results and observation values of daily NPP
Verification of BIOME-BGC Two approaches were used in the verification of BIOME-BGC. The first compared the annual average NPP of every plant type in Jinghe basin with literature values. Simulation of all types of plant in 493 units was carried out by the model for 1956–2000, and the results were analysed and weight-averaged by area to obtain the annual average NPP of every plant type in the Jinghe basin. While research in the Jinghe basin is limited, research results from elsewhere on the Loess Plateau could be used in the verification, for example those of Xu et al. (Citation2005), who simulated the ecosystem of the Zhifanggou basin in the Loess Plateau. In addition, Yu et al. (Citation2005) simulated all types of plants in northeast Asia with the CAVA model, and Feng et al. (Citation1999) researched the forest products of China, studies which are useful in the verification. Comparing the results of this simulation and literature values (), it is clear that the present results are similar to those of Xu et al. (Citation2005), but lower than the others. The differences may reflect the fact that the studies were conducted in different research areas.
Table 6 Comparison of annual NPP (g C m−2 year−1) results with values in the literature
The second verification approach was to compare average NPP and LAI of the Jinghe River basin with Moderate Resolution Imaging Radiometer (MODIS) NPP and LAI products. Annual average NPP for the year 2000, and average LAI for 20 August 2000 were compared with Terra/MODIS Net Primary Production Yearly L4 Global 1-km products and Leaf Area Index – Fraction of Photosynthetically Active Radiation 8-Day L4 Global 1-km products and Leaf Area Index – Fraction of Photosynthetically Active Radiation 8-Day L4 Global 1-km products. The mean values of the MODIS products were calculated in ArcGIS. The NPP and LAI results of BIOME-BGC showed good agreement with the MODIS products (see ), demonstrating that the model captures the ecosystem processes of the region.
Table 7 Comparison of NPP and LAI results with MODIS products
Table 8 The calibrated parameters
Table 9 Verification results of the monthly discharge
Calibration of WEP-L Monthly discharge data from three gauging stations (Zhangjiashan, Jingchuan, Yangjiaping) were selected to calibrate and verify WEP-L. Zhangjiashan station, located near the mouth of the Jinghe River basin, covers the entire basin. Yangjiaping station is near the mouth of the Puhe River basin, a sub-basin of the Jinghe River basin, covering 7819 km2. Jingchuan station is near the mouth of the Jingchuan River basin, and is a 5418 km2 sub-basin of the Puhe River basin.
The period 1991–1996 was selected for calibration. A trial-and-error calibration procedure was performed. The calibration parameters include depression storage depth of the land surface, soil saturated hydraulic conductivity, permeability of riverbed material, Manning roughness of the river and canopy resistance. The Jinghe basin was divided into three parts, each controlled by one gauging station. The parameters varied between the three parts. The calibration and verification criteria for discharge were minimum annual runoff errors and maximum Nash-Sutcliffe index. Because the main object of the study was to perform a long-term impacts analysis, priority was given to the first criterion. The calibrated parameter values are shown in , and the calibration results in and .
Fig. 5 Calibration (1991–1995, left) and verification (1996–2000, right) results for monthly discharge of: (a), (b) Zhangjiashan; (c), (d) Yangjiaping; and (e), (f) Jingchuan.
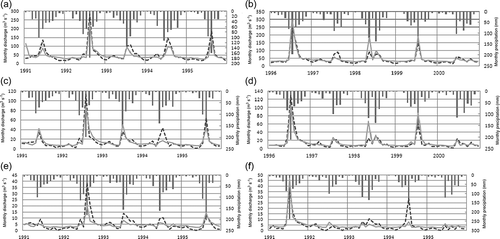
Verification of WEP-L After calibration, the parameters were kept unchanged to verify the model. The verification period was 1996–2000 and the results are given in and . The Nash-Sutcliffe index for Jingchuan station is relatively small due to the difficulty of simulating the reservoir regulation. As the focus of this study is long-term ecohydrological impacts, the relative error of average discharges is more important in the verification. Generally speaking, the verification results are quite encouraging, and the simulated discharge hydrographs are a good match with the observed ones.
Climate change scenario settings
The future climate change projections used were from WCRP CMIP3 multi-model data set (National Climate Centre of China Citation2008). The multi-model data set includes the simulation and projection results of 23 models (seven American, three Japanese, two British, two French, two Canadian, two Chinese, and one each German, German/Korean, Australian, Norwegian and Russian). The data were collected, analysed and provided by National Climate Centre of China. The results of these models were downscaled by a dynamical downscaling method and interpolated into the climate stations of the East Asia region. The Abdus Salam International Centre for Theoretical Physics Regional Climate Model (ICTP RegCM3) was nested within the GCMs for dynamical downscaling for the 2021–2050 period in East Asia. The results from the different models were arithmetically averaged to reduce the uncertainty of the GCM projections. These projection results have been validated for East Asia. The projection scenarios are those in the Special Report on Emissions Scenarios (SRES, Nakicenovic and Swart 2000), as follows:
- | Scenario A1B: A future world of very rapid economic growth, low population growth and rapid introduction of new and more efficient technology. Major underlying themes are economic and cultural convergence and capacity building, with a substantial reduction in regional differences in per capita income. In this world, people pursue personal wealth rather than environmental quality. | ||||
- | Scenario A2: A very heterogeneous world. The underlying theme is that of strengthening regional cultural identities, with an emphasis on family values and local traditions, high population growth, and less concern for rapid economic development. | ||||
- | Scenario B1: A convergent world with the same global population as in the A1 storyline, but with rapid changes in economic structures towards a service and information economy, with reductions in material intensity, and the introduction of clean and resource-efficient technologies. |
The air temperature and precipitation for 1961–1990 in the Jinghe basin are the baseline of the climate change scenarios. Based on multi-model average data, precipitation change rates and air temperature changes for 2021–2050 relative to 1961–1990 were calculated. The statistical downscale skill and spatial interpolation methods were used to distribute these data to the 493 sub-basins within the Jinghe River basin. Other basic data (e.g. meteorological data, soil data and plant physical parameters) remain as those for 1961–1990.
shows that the temperature changes of the three scenarios are similar; the rise in air temperature declines from the northwest to the southeast. However, the spatial distribution of precipitation change differs between scenarios. Precipitation increases over most of the basin in all three scenarios. Precipitation increase is greatest in the north of the basin under scenarios A1B and A2, but also increases significantly in the southern part under Scenario A2. Scenario B1 has the greatest increase in basin average precipitation. The area-weighted basin average air temperature and precipitation change are given in .
Table 10 Change of annual air temperature and precipitation, and CO2 concentrations of the climate change scenarios
Fig. 6 Air temperature and precipitation changes within the Jinghe basin in climate change scenarios: (a) air temperature change in A1B; (b) precipitation change in A1B; (c) air temperature change in A2; (d) precipitation change in A2; (e) air temperature change in B1; (f) precipitation change in B1.
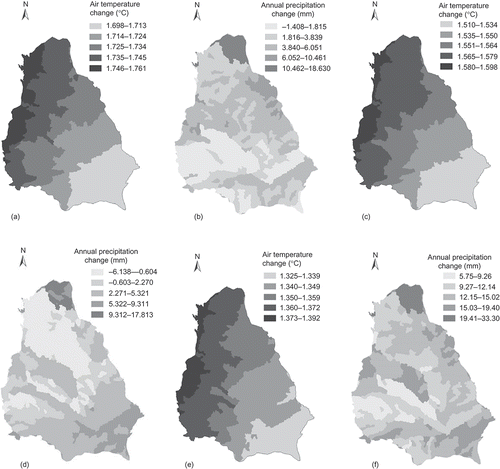
The CO2 concentrations for 2021–2050 in the three scenarios were set according to the Fourth Assessment Report of the Intergovernmental Panel on Climate Change (IPCC Citation2007) and are also shown in . The CO2 concentrations under scenarios A1B and A2 are the same in 2021, and lower than those under Scenario B1 in 2050. By 2050, CO2 concentrations are highest under Scenario A2. The CO2 concentration changes between 2021 and 2052 are set to a linear function to obtain annual values for input to BIOME-BGC.
Table 11 Historical simulation and predicted annual average discharge (108 m3) of the climate change scenarios (change rate in parentheses)
Table 12 Flood season and non-flood season discharge (108 m3) and changes (%) for the climate change scenarios relative to the historical simulation, for the three sub-basins
RESULTS
Hydrological change
Annual discharge Annual discharge is the most important aspect for water resources. The average annual discharges from the three gauge stations for the climate change scenarios A1B, A2 and B1 were calculated from the model outputs. Relative to the historical simulated annual average discharge for each gauge, all scenarios have negative impacts on annual discharge (). Annual discharge of the three gauge stations decreases by 10.75–15.05% under Scenario A1B, by 11.67–13.05% under Scenario A2, but only by 4.98–7.66% under Scenario B1.
Seasonal discharge July–October is the flood season in Jinghe basin and discharge in these three months counts for about 40% of the annual total. Discharge in the non-flood season varies little in a year. To analyse the impacts of climate change on seasonal discharge, the flood and non-flood season discharges were compared. It was found that flood season discharge decreased by a larger amount than non-flood season discharge at most gauge stations ().
Annual evapotranspiration change compares the historical simulated annual average evapotranspiration with that for each scenario. All scenarios result in increased annual evapotranspiration; the greatest increase, 3.58%, is with Scenario B1.
Water balance Changes in the basin water balance components (precipitation, evapotranspiration, discharge and water storage change in soil and bedrock) are summarized in , and show a decrease in annual discharge (Zhangjiashan gauge station) of 2.01 × 108, 2.19 × 108 and 0.93 × 108 m3 under scenarios A1B, A2 and B1, respectively.
Table 13 Annual ET of historical simulation and climate change scenarios, and the change in the components of the water balance
Ecological change
NPP change Net primary productivity (NPP), the net carbon gain by vegetation, is the balance between carbon gained by gross primary productivity (GPP) and carbon released by plant mitochondrial respiration (R plant):
The basin-average annual NPP increases by 8–9% in all three climate scenarios (), and shows little variation in the spatial distribution of the NPP increase between the scenarios. Compared to the historic simulation, the annual NPP of the western and northern parts of the basin show a relatively larger increase in all three climate change scenarios.
Table 14 Annual average NPP and NEP (g C m−2 year−1) of historical simulation and climate change scenarios and percentage change relative to the historical simulation
Fig. 7 Annual NPP distribution in the Jinghe basin: historical simulation and under different climate change scenarios.
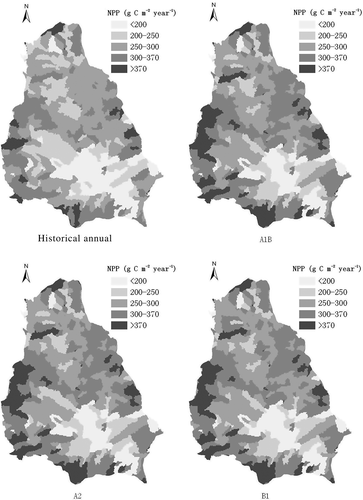
NEP change Net ecosystem productivity (NEP) is the net accumulation of carbon by an ecosystem, i.e. the balance between carbon entering and leaving the ecosystem, and can be expressed as NPP minus heterotrophic respiration from microbes and animals (R heterotr):
The NEP is ecologically important, because it represents the increment in carbon stored by an ecosystem. When integrated globally, NEP determines the impact of the terrestrial biosphere on the quantity of CO2 in the atmosphere, which strongly affects climate and the amount of carbon transferred to oceans (Chapin et al. Citation2002).
Annual NEP decreases by 9.44–15.03% under all three climate change scenarios (). shows that the spatial distribution of annual NEP in the Jinghe basin is similar for all three scenarios. The decrease in annual NEP relative to the historic simulation is greater in the western and northern parts of the basin.
Fig. 8 Annual NEP distribution in the Jinghe basin: historical simulation and under different climate change scenarios.
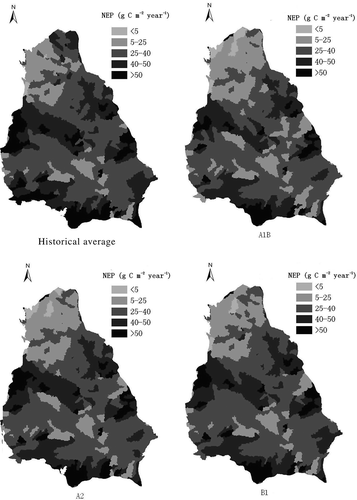
LAI change Daily average LAI and annual maximum LAI were calculated from BIOME-BGC outputs, and the values for forest and grass increase in all three climate change scenarios (). For forest, daily average LAI increases by about 0.1 m2 m−2 and annual maximum LAI by about 0.2 m2 m−2. For grass, the increases are 0.02 and 0.04 m2 m−2, respectively.
Other carbon cycle process changes The annual average GPP, R plant and R heterotr of the Jinghe River basin were calculated from the outputs of BIOME-BGC, and all increased relative to the historical simulation, as detailed in .
DISCUSSION
Some previous studies which use GCM projections as the input to hydrological models have also found that mean river discharge would decrease (Nijssen et al. Citation2001, Christensen et al. Citation2004, Kundzewicz et al. Citation2008, Chiew et al. Citation2009, Vaze et al. Citation2011) in tropical and mid-latitude basins. A few studies have shown that mean discharge would increase in high-latitude regions (Nijssen et al. Citation2001, Manabe et al. Citation2004, Graham et al. Citation2007). The uncertainty of the GCM projection is a major reason for the uncertainty in climate change impacts on discharge (Prudhomme et al. Citation2003, Thomson et al. Citation2005, Wilby et al. Citation2006).
This study found a decrease in discharge, in agreement with other studies of the mid-latitudes. The main reason for discharge decrease was the ET increase, as clear from the water balance (). The increase in the sum of ET plus water storage change in soil and bedrock (mainly due to ET increase) is larger than the increase in precipitation in the climate change scenarios, hence, discharge decreases.
The observed ET increase is likely due to the combined effects of increase in temperature, precipitation and LAI. In Jinghe basin, air temperature increased in all three climate change scenarios. Similarly, in all scenarios, daily averaged LAI and annual maximum LAI increased, which could lead to greater transpiration. Together, increasing air temperature and LAI can cause increase of potential ET. The Jinghe basin is in a region suffering from water scarcity and so the actual ET is limited by water supply. The increase in precipitation in all the three climate change scenarios increases the water supply to the basin, which, in turn, would increase actual ET. The influence of precipitation can be clearly seen under Scenario B1: it has the smallest increase in air temperature and LAI, but because the increase in precipitation is the largest, the increase in ET is the greatest.
In the ecosystem carbon cycle, NPP and NEP are as expressed in Equationequations (1)(1) and (2). Future climate change may impact on every process of the ecosystem carbon cycle. The GPP will increase because of growing season extension, LAI increase, CO2 concentration elevation and temperature increase. Plant respiration may increase due to temperature increase and CO2 concentration elevation, and, similarly, heterotrophic respiration will increase.
Table 15 LAI (m2 m−2) of historical simulation and climate change scenarios
Previous studies have agreed on NPP increase under climate change impacts (Parton et al. Citation1995, Peng and Apps Citation1999, Ravindranath et al. Citation2005). Some studies show that the increase of NPP is caused by the combined influence of climate change and CO2 concentration elevation. However, there are many different predictions for NEP (Karjalainen et al. Citation2002, Lenihan et al. Citation2003, Levy et al. Citation2004, Woodward and Lomas Citation2004).
This study found that, in all areas of Jinghe basin, NPP will increase and NEP will decrease under future climate change scenarios. In order to fully understand the impacts of climate change on the ecosystem carbon cycle, other components of the ecosystem were checked. The results showed that GPP and R plant increase in all three climate change scenarios, but the GPP shows a larger increase compared to R plant, hence the NPP increase. The R heterotr increases more than the increase in NPP; hence, NEP decreases in all three climate change scenarios.
Table 16 GPP, R plant and R heterotr of historical simulation and climate change scenarios (g C m−2 year−1)
The average annual NPP and NEP of the western and northern parts of the basin show relatively greater changes in the three climate change scenarios, which correspond to the spatial changes of air temperature and precipitation; air temperature increases more in the west of the basin and precipitation increases more in the north. This demonstrates that increases in air temperature and precipitation have similar effects on NPP and NEP. Although vegetation will obtain more carbon under the impacts of climate change, ecosystems will lose carbon in this basin.
Most of the uncertainties in climate change impacts on hydrology (Prudhomme et al. Citation2003, Thomson et al. Citation2005, Wilby et al. Citation2006) and ecosystems (Bachelet et al. Citation2001, Berthelot et al. Citation2005, Olesen et al. Citation2007) come from the GCM projections and the regional differences. This study reduced the uncertainties of the GCM projections by averaging 23 GCM projections. However, the uncertainties are still a challenge to the accuracy of ecohydrological impact prediction.
There are some important improvements to make, which may aid future studies in this field. First, the plant types can be updated dynamically, facilitating research of the long-term evolution of ecohydrological systems. Second, the coupling could be investigated more closely to evaluate the impacts between the hydrological cycle and ecosystem processes. Third, more detailed physical parameters could be used by incorporating more observational experiments. Finally, soil erosion processes need to be considered in the ecohydrological simulation of the Loess Plateau.
CONCLUSIONS
By coupling the WEP-L model (a distributed hydrological model) with the BIOME-BGC model (a terrestrial ecosystem model), the ecohydrology of the Jinghe River basin was simulated and potential climate change impacts were predicted. The model was verified using field experiments, literature and observational data. Ecohydological impacts under different future emissions scenarios were predicted by using GCM climate projections from the CMIP3 multi-model data set. To reduce the uncertainties of the GCM projections, the average of 23 GCM projections were used as the climate inputs to the ecohydrological model.
The projected climate change may have three main results:
1. | The annual discharge will decrease, and the flood season discharge will decrease more than non-flood season discharge. The ET increase caused by air temperature increase, precipitation increase and LAI increase is the main reason for the decrease in discharge. | ||||
2. | The average annual NPP of the basin will increase and annual NEP will decrease. The increase in annual NPP is caused by a larger increase in GPP than in plant respiration; however, the decrease in NEP is caused by a larger increase in heterotrophic respiration than in NPP. | ||||
3. | The spatial distribution of the NPP and NEP changes show that the air temperature and precipitation increases have similar impacts on NPP and NEP. |
These climate change impacts may influence human water use and ecosystem stability. More effort should be addressed to water resources management and ecosystem planning in this region in the future.
Acknowledgements
This study was financially supported by programmes of the National Natural Science Foundation of China (50939006, 50779074 and 51021006) and the National Key Technology R&D Programme (2010BAC69B02-02). The authors also thank the National Climate Centre of China for providing the downscaled GCM results, the Program for Climate Model Diagnosis and Intercomparison (PCMDI) and the World Climate Research Programme Coupled Model Intercomparison Project for collecting and archiving the GCM output. Mr Zhaobao Li is thanked for his assistance in the field observation experiments of this study.
REFERENCES
- Andersen , J. , Refsgaard , J.C. and Jensen , K.H. 2001 . Distributed hydrological modelling of the Senegal River basin—model construction and validation . Journal of Hydrology , 247 : 200 – 214 .
- Bachelet , D. 2001 . Climate change effects on vegetation distribution and carbon budget in the United States . Ecosystems , 4 : 164 – 185 .
- Baird , A.J. and Wilby , R.L. 1999 . Eco-hydology—plant and water in terrestrial and aquatic environment , London : Routledge .
- Band , L.E. 1993 . Forest ecosystem processes at the watershed scale: incorporating hillslope hydrology . Agricultural and Forest Meteorology , 63 : 93 – 126 .
- Band , L. E. 1996 . Ecosystem processes at the watershed scale: sensitivity to potential climate change . Limnology and Oceanography , 41 ( 5 ) : 928 – 938 .
- Berthelot , M. 2005 . How uncertainties in future climate change predictions translate into future terrestrial carbon fluxes . Global Change Biology , 11 : 959 – 970 .
- Black , P.E. 1991 . Watershed hydrology , Englewood Cliffs , NJ : Prentice-Hall .
- Chapin , F.S. III , Matson , P.A. and Mooney , H.A. 2002 . Principles of terrestrial ecosystem ecology , New York : Springer .
- Chen , C. 2008 . Analysis of Jinghe watershed vegetation dynamics and evaluation of its relation to precipitation . Acta Ecologica Sinica , 28 ( 3 ) : 925 – 938 . (in Chinese)
- Chiew , F.H.S. 2009 . Estimating climate change impact on runoff across southeast Australia: method, results, and implications of the modeling method . Water Resources Research , 45 : W10414 doi: 10.1029/2008WR007338
- Christensen , N.S. 2004 . The effects of climate change on the hydrology and water resources of the Colorado River Basin . Climatic Change , 62 : 337 – 363 .
- Cramer , W. 2001 . Global response of terrestrial ecosystem structure and function to CO2 and climate change: results from six dynamic global vegetation models . Global Change Biology , 7 : 357 – 373 .
- David , W.O. 2001 . Chloride persistence in a deiced access road drainage system . Journal of Environmental Quality , 30 : 1756 – 1770 .
- Dickinson , R.E. 1991 . Evapotranspiration models with canopy resistance for use in climate models: a review . Agricultural and Forest Meteorology , 54 : 373 – 388 .
- Engel , B.A. 1993 . Nonpoint source (NPS) pollution modeling using models integrated with Geographic Information Systems (GIS) . Water Science and Technology , 28 ( 3–5 ) : 685 – 690 .
- Feng , Z.W. 1999 . Biomass and productivity of forest ecosystem in China , Beijing : Science Press . in Chinese
- Govind , A. 2009 . A spatially explicit hydro-ecological modeling framework (BEPS-TerrainLab V2.0): model description and test in a boreal ecosystem in Eastern North America . Journal of Hydrology , 367 : 200 – 216 .
- Graham , L. , Andréasson , J. and Carlsson , B. 2007 . Assessing climate change impacts on hydrology from an ensemble of regional climate models, model scales and linking methods—a case study on the Lule River basin . Climatic Change , 81 : 293 – 307 .
- He , X. 2002 . Adapting a drainage model to simulate water table levels in coastal plain soils . Soil Science Society of America Journal , 66 : 1722 – 1731 .
- IPCC (Intergovernmental Panel on Climate Change) . 2007 . Climate change 2007: Synthesis report , Cambridge : Cambridge University Press . Fourth Assessment Report of the IPCC
- Jia , Y. 2001 . Development of WEP model and its application to an urban watershed . Hydrological Processes , 15 ( 11 ) : 2175 – 2194 .
- Jia , Y. 2002 . Coupling simulation of water and energy budgets and analysis of urban development impact . Journal of Hydrologic Engineering ASCE , 7 ( 4 ) : 302 – 311 .
- Jia , Y. 2004 . Distributed hydrologic modeling and river flow forecast for water allocation in a largescaled inland basin of Northwest China . In: Proceedings of 2nd APHW Conference, Singapore, vol. , 2 July 2004 : 285 – 292 . Tokyo: The Asia Pacific Association of Hydrology and Water Resources Publishing
- Jia , Y. 2006 . Development of the WEP-L distributed hydrological model and dynamic assessment of water resources in the Yellow River basin . Journal of Hydrology , 331 : 606 – 629 .
- Jia , Y. 2009 . Distributed modeling of landsurface water and energy budgets in the inland Heihe River basin of China . Hydrology and Earth System Sciences , 13 ( 10 ) : 1849 – 1866 .
- Jia , Y. , Kinouchi , T. and Yoshitani , J. 2005 . Distributed hydrologic modeling in a partially urbanized agricultural watershed using WEP model . Journal of Hydrologic Engineering ASCE , 10 ( 4 ) : 253 – 263 .
- Jia , Y. and Tamai , N. 1998 . Integrated analysis of water and heat balance in Tokyo metropolis with a distributed model . Journal of Japan Society of Hydrology and Water Resources , 11 ( 1 ) : 150 – 163 .
- Karjalainen , T. 2002 . An approach towards an estimate of the impact of forest management and climate change on the European forest sector carbon budget: Germany as a case study . Forest Ecology and Management , 162 : 87 – 103 .
- Kim , H. 17–19 January 2005 2005 . “ Monitoring and analysis of hydrological cycle of the Cheonggyecheon watershed in Seoul, Korea ” . In International Conference on Simulation and Modeling 2005 , Edited by: Kachitvichyanukul , V. , Purintrapiban , U. and Utayopas . 17–19 January 2005 , Thailand: NakornPathom : Paper C4-03 .
- Kundewicz , Z.W. 2008 . The implications of projected climate change for freshwater resources and their management . Hydrological Sciences Journal , 53 ( 1 ) : 3 – 10 .
- Lenihan , J.M. 2003 . Climate change effects on vegetation distribution, carbon, and fire in California . Ecological Applications , 13 : 1667 – 1681 .
- Levy , P.E. , Cannell , M.G.R. and Friend , A.D. 2004 . Modelling the impact of future changes in climate, CO2 concentration and land use on natural ecosystems and the terrestrial carbon sink . Global Environmental Change , 14 : 21 – 30 .
- Maidment , D.R. 1992 . Handbook of hydrology , New York : McGraw-Hill .
- Manabe, S., Milly, P.C.D., and Wetherald, R., 2004. Simulated long-term changes in river discharge and soil moisture due to global warming. Hydrological Sciences Journal, 49 (4), 625–642 http://dx.doi.org/10.1623/hysj.49.4.625.54429 (http://dx.doi.org/10.1623/hysj.49.4.625.54429)
- Mo , X. , Lin , Z. and Liu , S. 2007 . Climate change impacts on the ecohydrological processes in the Wuding River basin . Acta Ecologica Sinica , 27 ( 12 ) : 4999 – 5007 . in Chinese
- Nakicenovic , N. and Swart , R. 2000 . Emissions scenarios. A special report of Working Group III of the Intergovernmental Panel on Climate Change , Cambridge : Cambridge University Press .
- National Climate Centre of China . 2008 . Manual of China climate change prediction dataset [online]. Available from: http://bcc.cma.gov.cn/en
- Nijssen , B. 2001 . Hydrologic sensitivity of global rivers to climate change . Climatic Change , 50 : 143 – 175 .
- Olesen , J. 2007 . Uncertainties in projected impacts of climate change on European agriculture and terrestrial ecosystems based on scenarios from regional climate models . Climatic Change , 81 : 123 – 143 .
- Morales , P. 2005 . Comparing and evaluating process-based ecosystem model predictions of carbon and water fluxes in major European forest biomes . Global Change Biology , 11 : 2211 – 2233 .
- Pan , Y. 2002 . A biogeochemistry-based dynamic vegetation model and its application along a moisture gradient in the continental United States . Journal of Vegetation Science , 13 : 369 – 382 .
- Parton , W.J. 1993 . Observation and modeling of biomass and soil organic matter dynamics for the grassland biome worldwide . Global Biogeochemical Cycles , 7 : 485 – 809 .
- Parton , W.J. 1995 . Impact of climate change on grassland production and soil carbon worldwide . Global Change Biology , 1 : 13 – 22 .
- Peng , C. and Apps , M.J. 1999 . Modelling the response of net primary productivity (NPP) of boreal forest ecosystems to changes in climate and fire disturbance regimes . Ecological Modelling , 122 : 175 – 193 .
- Prudhomme , C. , Jakob , D. and Svensson , C. 2003 . Uncertainty and climate change impact on the flood regime of small UK catchments . Journal of Hydrology , 2 ( 77 ) : 1 – 23 .
- Qi , Q. 2009 . Temporal and spatial changes of vegetation cover and the relationship with precipitation in Jinghe Watershed of China . Chinese Journal of Plant Ecology , 33 ( 2 ) : 246 – 253 . in Chinese
- Qiu , Y. 2006 . Preliminary analysis of hydrological and water resources effects under the impacts of water and soil conservation engineering in the Fenhe River basin . Journal of Nature Resources China Society of Nature Resources , 21 ( 1 ) : 24 – 30 . in Chinese
- Ravindranath , N.H. 2005 . “ Impact of climate change on forests in India ” . In arXiv:q-bio/0511001
- Running , S.W. and Coughlan , J.C. 1988 . A general model of forest ecosystem processes for regional applications. I, Hydrologic balance, canopy gas exchange and primary production processes . Ecological Modelling , 42 : 125 – 154 .
- Running , S.W. and Hunt , R.E. 1993 . Generalization of a forest ecosystem process model for other biomes (BIOME-BGC) and an application for global-scale models , San Diego , CA : Academic Press .
- Sitch , S. , Smith , B. and Prentice , I.C. 2003 . Evaluation of ecosystem dynamics, plant geography and terrestrial carbon cycling in the LPJ dynamic vegetation model . Global Change Biology , 9 : 161 – 185 .
- Song , F. 2009 . Estimation of vegetation net primary productivity on North Shaanxi Loess Plateau . Chinese Journal of Ecology , 28 ( 11 ) : 2311 – 2318 . in Chinese
- Sun , G. 2000 . Climate change impacts on the hydrology and productivity of a pine plantation . Journal of the American Water Resources Association , 36 : 367 – 374 .
- Suo , A. 2005 . Regulation of vegetation landscape pattern on runoff in watershed of Loess Plateau . Journal of Soil and Water Conservation , 19 ( 4 ) : 13 – 34 . in Chinese
- Tague , C. 2004 . Application of the RHESSys model to a California semiarid shrubland watershed . Journal of the American Water Resources Association , 40 ( 3 ) : 575 – 589 .
- Tague , C.L. and Band , L.E. 2004 . RHESSys: Regional Hydro-Ecologic Simulation System—an object-oriented approach to spatially distributed modeling of carbon, water, and nutrient cycling . Earth Interactions , 19 : 1 – 42 .
- Thomson , A. 2005 . Climate change impacts for the conterminous USA , 67 – 88 . Netherlands : Springer .
- Vaze , J. 2011 . Impact of climate change on water availability in the Macquarie-Castlereagh River basin in Australia . Hydrological Processes , 25 : 2597 – 2612 .
- Vicuna , S. and Dracup , J. 2007 . The evolution of climate change impact studies on hydrology and water resources in California . Climatic Change , 82 : 327 – 350 .
- Walko , R.L. 2000 . Coupled atmosphere–biophysics–hydrology models for environmental modeling . Journal of Applied Meteorology , 39 : 931 – 944 .
- Wang , G. and Li , W. 2002 . Reasonability analysis of hydrologic design results , Zhengzhou : Huanghe Water Conservancy Press, ISBN 7806214356 . in Chinese
- Wang , Q. , Watanabe , M. and Zhu , O . 2005 . Simulation of water and carbon fluxes using BIOME-BGC model over crops in China . Agricultural and Forest Meteorology , 131 : 209 – 224 .
- White , A. , Cannell , M.G.R. and Friend , A.D. 1999 . Climate change impacts on ecosystems and the terrestrial carbon sink: a new assessment . Global Environmental Change , 9 ( Supplement 1 ) : S21 – S30 .
- White , M.A. 2000 . Parameterization and sensitivity analysis of the BIOME-BGC terrestrial ecosystem model: net primary production controls . Earth Interactions , 4 : 1 – 85 .
- Wilby , R.L. 2006 . Integrated modelling of climate change impacts on water resources and quality in a lowland catchment: River Kennet . Journal of Hydrology , 330 : 204 – 220 .
- Woodward , F.I. and Lomas , M.R. 2004 . Vegetation dynamics—simulating responses to climatic change . Biological Reviews , 79 : 643 – 670 .
- Xu , H.M. , Jia , H.K. and Huang , Y.M. 2005 . A simulation model of net primary production at watershed scale in hilly area of Loess Plateau, China . Acta Ecologica Sinica , 25 ( 5 ) : 1064 – 1074 . in Chinese
- Yang , W. and Shao , M. 2000 . Study on the soil moisture in the Loess Plateau , Beijing : China Science Press . in Chinese
- Yu , D.Y. 2005 . Spatial and temporal response of NPP of East Asian vegetation to climate changes . Journal of Beijing Forestry University , 27 ( Supplement 2 ) : 96 – 101 . in Chinese
- Zalewski, M., 2002. Ecohydrology—the use of ecological and hydrological processes for sustainable management of water resources. Hydrological Sciences Journal, 47 (5), 823–832 http://dx.doi.org/10.1080/02626660209492986 (http://dx.doi.org/10.1080/02626660209492986)