Abstract
The combined analysis of precipitation and water scarcity was done with the use of the Standardized Precipitation Index (SPI) and the Standardized Runoff Index (SRI), developed as a monthly, two-variable SPI-SRI indicator to identify different classes of hydrometeorological conditions. Stochastic analysis of a long-term time series (1966–2005) of monthly SPI-SRI indicator values was performed using a first-order Markov chain model. This provided characteristics of regional features of drought formation, evolution and persistence, as well as tools for statistical long-term drought hazard prediction. The study was carried out on two subbasins of the Odra River (Poland) of different orography and land use: the mountainous Nysa Kłodzka basin and the lowland, agricultural Prosna basin. Classification obtained with the SPI-SRI indicator was compared with the output from the NIZOWKA model that provided identification of hydrological drought events including drought duration and deficit volume. Severe and long-duration droughts corresponded to SPI-SRI Class 3 (dry meteorological and dry hydrological), while severe but short-term droughts (lasting less than 30 days) corresponded to SPI-SRI Class 4 (wet meteorological and dry hydrological). The results confirm that, in Poland, meteorologically dry conditions often shift to hydrologically dry conditions within the same month, droughts rarely last longer than 2 months and two separate drought events can be observed within the same year.
Résumé
L’analyse combinée des précipitations et du déficit en eau a été réalisée en utilisant l’indice normalisé des précipitations (INP) et l’indice normalisé du ruissellement (INR), calculés sur une base mensuelle, qui ont été combinés pour fournir une informations selon deux variables et identifier les différentes catégories de conditions hydrométéorologiques. L’analyse stochastique des longues séries chronologiques (1966–2005) des valeurs mensuelles des indicateurs INP et INR a été réalisée en utilisant un modèle de chaîne de Markov d’ordre un. Cela nous a fourni les caractéristiques régionales de la formation, de l’évolution et de la persistance de la sécheresse, ainsi que des outils pour la prévision statistique à long terme du risque de sécheresse. L’étude a été réalisée sur deux sous-bassins de la rivière Odra (Pologne) d’orographie et d’utilisation des sols différentes: le bassin montagneux de Nysa Kłodzka et le bassin agricole de plaine de Prosna. La classification obtenue avec l’indicateur INP-INR a été comparée avec la sortie du modèle Nizowka qui a identifié les sécheresse hydrologiques y compris leur durée et leur volume de déficit. Les sécheresses graves et de longue durée correspondent à la classe 3 de l’indicateur INP-INR (météorologie sèche et hydrologie sèche), tandis que les sécheresses graves, mais courtes (d’une durée inférieure à 30 jours) correspondent à la classe 4 de l’indicateur INP-INR (météorologie humide et hydrologie sèche). Les résultats confirment que, sur le territoire de la Pologne, les conditions météorologiques sèches se transforment souvent en sécheresse hydrologique au cours du même mois, les sécheresses durant rarement plus de deux mois et deux épisodes de sécheresse distincts peuvant être observés au sein d’une même année.
INTRODUCTION
Effective water management requires methods and tools supporting decision making which cope with water scarcity and drought hazard (Hayes et al. Citation2005). Scarcity of water resources results from a range of phenomena, starting from precipitation deficit propagating over time and affecting the successive components of the hydrological cycle (Wilhite Citation2000). Lack of precipitation over a large area that lasts for a longer period of time can lead to the first phase of drought—meteorological drought. Further shortage of precipitation leads to water deficit, which propagates through the subsurface part of the hydrological cycle causing soil dryness and finally streamflow reduction evolving into a hydrological drought. This phase causes decrease in groundwater heads and groundwater discharges, as well streamflow decline (Debski Citation1970, Changnon Citation1987). Therefore, drought can be defined as a sustained and regionally extensive occurrence of below-average natural water availability and is characterized as a deviation from normal conditions (Tallaksen and van Lanen Citation2004).
At the beginning of the 21st century on the territory of Poland droughts were observed almost every year. The most severe droughts were noted in 2003 and 2006 (Lorenc et al. Citation2006, Farat et al. Citation2007). Their severity and duration, as well as the projected climate changes that are likely to increase the frequency and intensity of droughts, highlighted an increasing need to develop tools for drought assessment and prediction so as to support effective decision making and mitigate the impacts of droughts (Kundzewicz and Matczak Citation2010).
To support appropriate monitoring and prediction tools, it is essential to characterize droughts through drought indices (Sivakumar and Wilhite Citation2002, Rossi Citation2003). Drought indices enable drought early warning and are a useful piece of information for policy-makers in water management. Drought indices are important and functional elements for drought monitoring and assessment since they simplify complex interrelationships between many climate and climate-related parameters. Indices make it easier to communicate information about climate anomalies to a diverse user audience and leave the scientists to assess climate anomalies quantitatively in terms of their intensity, duration, spatial extent and frequency, thus allowing the analysis of historical drought events and their recurrence probability (Iglesias et al. Citation2007).
There is a wide range of drought indices applied to identify and monitor drought (Heim Citation2002, Wells et al. Citation2004, Jain et al. Citation2010, Vasiliades et al. Citation2011, Liu et al. Citation2012). Drought indices can be estimated for different spatial and temporal scales, from regional to global, and in relation to various sectors such as agriculture, water management or economic (Wilhite Citation1991). Some drought indices are based on one feature only, i.e. precipitation for meteorological drought assessment, soil moisture for agricultural drought, and streamflow or groundwater level for hydrological drought (Nalbantis and Tsakiris Citation2009, Sharma and Panu Citation2012). Some are highly elaborate and use information on temperature, evapotranspiration, soil moisture, snow cover, surface water and groundwater supply (Steinemann Citation2003). However, there is a continuous effort aiming to derive efficient drought indices in the hope of better monitoring of drought conditions which can be useful for early warning, as well as for deriving better drought parameters (Mishra and Singh Citation2010). Niemeyer (Citation2008) concluded that, instead of developing new single drought indicators, the existing ones should be combined into more comprehensive and integrative monitoring tools. The decision to apply a particular indicator usually depends on the aim of the application, the ability to reproduce the spatial and temporal features of the drought occurrence, as well as on the availability of the data required for its calculation (Tokarczyk and Szalińska Citation2010, Citation2013). Hannaford et al. (Citation2010) found that the Regional Standardized Precipitation Index for meteorological droughts and the Regional Deficiency Index for hydrological droughts were good single indicators to use for drought monitoring and forecasting in Europe. Effective monitoring, early warning for droughts and planning in water sectors promote standardized indices which can be easily transferred and applied in different regions based on an available collection of hydrometeorological data.
In the present study, two widely-used indices, namely the Standardized Precipitation Index (SPI) and the Standardized Runoff Index (SRI), were applied for drought assessment. The SPI (McKee et al. Citation1993) offers a quick, handy, simple approach with minimal data requirements to assess meteorological drought (Komuscu Citation1999). The SRI (Shukla and Wood Citation2008) has been developed for efficient analysis of hydrological drought (Keskin and Sorman Citation2010). The applied indices enable prompt classification of actual observations of precipitation and runoff as wet, normal or dry conditions for a particular time of a year. Moreover, formulation of the indices (McKee et al. Citation1993, Citation1995) allows comparison of the obtained results to be made for different locations and climates, while the limited number of required data—precipitation and runoff—makes them easy to be retrieved from standard water datasets.
The two indices, SPI and SRI, usually used to assess drought independently, were combined into one hydrometeorological index, the joint SPI-SRI indicator, which represents the relationship between precipitation and water resources deficit and investigates drought hazard in terms of the conditions contributing to drought formation, evolution and persistence. Dry meteorological conditions occurring while runoff conditions are normal or wet can result in meteorological drought, simultaneous meteorological and hydrologically dry conditions can trigger prolonged drought, whereas hydrologically dry conditions when precipitation has returned to at least normal level can lead to a short-term drought. The indicator was developed on a monthly basis which is a compromise between the short time intervals required to capture the temporal dynamics of moisture conditions and propagation of water in the hydrological cycle and the long observation period recommended to provide meaningful evaluation of drought phenomena.
The aim of this work was to derive an efficient indicator for operational drought hazard assessment based on regional-scale meteorological and hydrological standard information in order to support drought monitoring, early warnings and water management. The two-variable SPI-SRI joint indicator was used to investigate the coincidence and transition scheme of moisture conditions contributing to different stages of drought in order to obtain information on the frequency of each drought stage, the expected residence time in each drought stage, and the time required to reach the non-drought state, or to predict hazard of drought formation.
The study was done for selected gauging and precipitation stations located in two basins of different orography and land use: the mountainous Nysa Kłodzka basin and the lowland, agricultural Prosna basin. Monitoring stations are operated by Institute of Meteorology and Water Management, National Research Institute, Poland.
MATERIALS AND METHODS
Study area
The upper Nysa Kłodzka River is a left-side tributary of the Odra River with a basin area of 1744 km2, significant population density and industrial centres. The land-use pattern shows a clear predominance of arable lands and forests. The Nysa Kłodzka is a mountainous basin with significant precipitation gradient. The annual rainfall varies between 560 and 1100 mm (). The wettest month is July, with precipitation totals of 100–140 mm, while the driest is February. The diverse orography of the area, causing vertical variability of climate conditions, results in complex hydrological conditions in the Nysa Kłodzka basin. The surface runoff is relatively high and its volume mainly depends on the slope of land draining waters to streams and rivers, making the water level in the upper Nysa Kłodzka River respond rapidly to runoff from the mountainous areas. Analogously, a lack of precipitation results in a relatively fast decrease in streamflow.
Fig. 1 Spatial distribution of annual precipitations totals in: (a) the Nysa Kłodzka basin and (b) the Prosna basin.
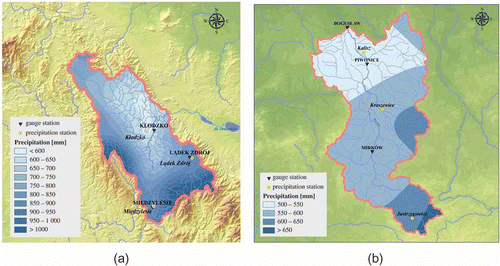
The Prosna River is the largest left-side tributary of the middle Warta River, also a tributary of the Odra River. The total area of the Prosna River basin is 4916.63 km2. The basin is of rather rectangular shape, prominently extended from south to north, and has a typical agricultural character (70% arable lands). Within the predominantly plain area of the Prosna basin, the spatial differentiation of mean multi-year annual precipitation totals is quite significant, ranging from 520 to 640 mm (). The highest mean monthly totals are in July, and the lowest in February. Features of both analysed basins are summarized in .
Table 1 Physical-geographical characteristics of the investigated basins.
In the upper Nysa Kłodzka basin, data from three water gauging (Miedzylesie, Ladek Zdroj and Klodzko) and three precipitation stations (Międzylesie, Kłodzko and Lądek Zdrój) were analysed. The precipitation and water gauging stations were coupled on the basis of their locations and their mutual representativeness. The latter was expressed by correlation coefficient values. Międzylesie precipitation station was coupled with Międzylesie water gauging station, Lądek Zdrój with Lądek Zdrój and Kłodzko with Kłodzko. The same was done with the station located in the Prosna basin. Due to the smaller spatial variability of rainfall and runoff there was a bigger distance between the coupled stations: Jastrzygowice precipitation station was coupled with Mirków water gauging station, Kraszewice with Piwonice and Kalisz with Bogusław. The locations of the stations allowed regional hydrometerological diversity to be captured within each studied basin.
Applied drought indices
The Standardized Precipitation Index (SPI) is an indicator developed by McKee et al. (Citation1993) that evaluates the lack or surplus of precipitation during a given period of time. It is used to identify drought periods and the severity of droughts at multiple time scales: typically from 1 to 48 months. The calculation of SPI is based on a long-term record of precipitation totals observed for the same period of time that are fitted to a probability distribution function. Cumulative distribution is then transferred into a standard normal variable with zero mean and variance equal to one. The SPI results are given in units of standard deviation. The magnitude of the departure from zero is a probabilistic measure of the severity of a wet or dry event. The defined categories of SPI for drought severity are: 0 to – 0.99: mild drought; –1 to –1.49: moderate drought; –1.50 to –1.99: severe drought; and >2.00 extreme drought (McKee et al. Citation1993). The normalization allows for comparison between different climate regimes. Nowadays, the SPI is one of the most popular indexes for drought monitoring (Wilhite Citation2005).
The Standardized Runoff Index (SRI) is based on the methodology developed for the SPI with the application for assessment of hydrological drought (Shukla and Wood Citation2008). The computation of the SRI involves fitting a probability density function to an empirical frequency distribution of mean runoff values for given time intervals. Typically, the gamma probability density function is used. This cumulative probability is then transformed to the standardized normal distribution with mean zero and variance one, which results in the value of the SRI. The drought severity categorization follows the same gradation as the SPI. Despite being a relatively new index, the SRI is gaining popularity (Mo Citation2008, Shukla et al. Citation2011). On monthly to seasonal time scales, the SRI is a useful complement to the SPI for depicting hydrological aspects of droughts (Mishra and Singh Citation2010).
The scale for drought severity assessment with the use of SPI and SRI reflects the probability of occurrence of given range of values. The original scale developed for the SPI was modified in further applications. For example the US National Climatic Data Center (of the NOAA; National Oceanic and Atmospheric Administration) introduced near normal conditions to range from – 0.5 to 0.5. These values were also found to correspond to normal meteorological moisture conditions in the area of Poland (Labedzki and Bak Citation2002) and were used in this study. Both SPI and SRI values follow a normal distribution with the same mean and variance. Therefore, the same range for normal conditions was applied to SRI values.
Combined SPI-SRI indicator
Two separate indices were interpreted as a complementary information on meteorological and hydrological moisture conditions. The new two-variable indicator for drought hazard assessment was developed as a pair of SPI and SRI values obtained for the same month for paired rainfall and stream gauge stations. The developed SPI-SRI indicator values were divided into classes describing different hydrometeorological conditions. The range of values representing normal conditions for both SPI and SRI indicators independently corresponds to the given probability range. Normal conditions for the SPI-SRI indicator are the superposition of probabilities of normal SPI and normal SRI values. Due to the positive correlation between SPI and SRI values, the range of equal probabilities of SPI-SRI indicator forms an ellipse.
The classification criteria for hydrometeorological conditions were constructed using a two-step approach proposed by Eyton (Citation1984) to determine the class membership of a given pair of correlated values. The first step indicates whether or not a SPI-SRI pair of values falls within the equal probability ellipse. This was done by calculating the Mahalanobis distance (Citation1936) between a given point and the origin (0,0). If this distance is smaller than or equal to 0.5, the point is classified as belonging to normal conditions (Class 0). If the calculated value exceeds 0.5, and the SPI-SRI point falls outside the ellipse, the second classification step takes place. The point is assigned to Class 1 if the SPI and SRI values are positive, Class 2 if the SPI is negative and SRI is positive, Class 3 if both SPI and SRI are negative, and Class 4 if SPI is positive and SRI negative.
This approach allows five classes of combined SPI-SRI indicator to be established that correspond to ():
Class 0: normal meteorological and hydrological conditions;
Class 1: wet meteorological and wet hydrological conditions;
Class 2: dry meteorological and wet hydrological conditions;
Class 3: dry meteorological and dry hydrological conditions; and
Class 4: wet meteorological and dry hydrological conditions.
Fig. 2 Classification of the SPI versus SRI scatter plots for the selected paired meteorological and hydrological stations in: (a) the Nysa Kłodzka basin and (b) the Prosna basin.
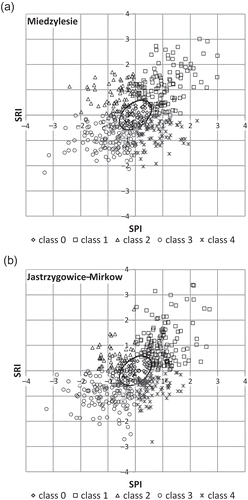
To establish the hazard of drought formation, the evolution and persistence these SPI-SRI classes can be interpreted in the following way. Class 2 represents conditions in the first stage of drought—meteorological drought. Further shortage of precipitation leads to the reduction of water resources and hydrological drought formation (Class 3). Restoring precipitation deficit may not be enough to instantly restore the water resource; therefore, Class 4 corresponds to the hydrological drought, while meteorological conditions are back to normal.
Preliminaries on Markov chains
Markov chain models have been commonly used for drought analysis. Gabriel and Neumann (Citation1962) were among the first to apply Markov models for dry spell analysis. Lohani and Loganathan (Citation1997) forecast drought conditions for future months, based on the current drought class described by the Palmer drought index. Fernández and Salas (Citation1999) presented a method for estimating the return period of droughts when underlying hydrological data series (annual streamflow) are autocorrelated. They assumed that the binary process consisting of dry years (D: Xt < x0) and wet years (W: Xt ≥ x0) follows a simple (first-order) Markov chain with two states (dry and wet). Bayazit and Önöz (Citation2005) analysed the probability distribution and return periods of joint droughts at a number of sites assuming that streamflows are cross-correlated first-order Markov processes. The stochastic properties of the SPI time series were explored for predicting drought class transitions using Markov chain modelling (Paulo et al. Citation2005, Paulo and Pereira Citation2007). Akyuz et al. (Citation2012) applied first- and second-order Markov chain models to dry and wet periods of annual streamflow series to reproduce the stochastic structure of hydrological droughts. Sharma and Panu (Citation2012) applied Markov chain models as a simple tool for predicting the T-year drought lengths based on annual, monthly and weekly SHI (Standardized Hydrological Index) sequences. They reported that the Markov chain-2 model was found satisfactory on monthly and weekly time scales, as the river flows under consideration were strongly autocorrelated.
The Markov chain (Cinlar Citation1974) is a stochastic process {Xt}, characterized by a set of states (S) and the transition probability (pij) between states. The transition probability pij is the probability that the Markov chain is at the next time point in state j, provided that it is at the present time point in state i. First-order time dependence implies that, at each time t, the transition probability for the Xt+1 depends only on the most recent state Xt. The transition probability is defined as:
where i,j ∈ S.
The transition probabilities are estimated from the sample by counting the number of transitions nij that conditions in a time period t pass to state j from state i in a time period t – 1:
A steady-state probability is the unconditional probability of being in state j (πj); it is the fraction of time, the process in the long-run is in state j. The steady-state probabilities are obtained while solving the system of equations:
The probability of an uninterrupted stay, during m months, in a particular class i is computed as the product of the probabilities of the successive transitions between X0 and Xm:
From the probabilities of uninterrupted stay in a state i during m months (m = 1,2,…, k), the expected residence time E(Ti|X0), is computed as:
where hij(n) is the probability that the first passage time from state i to state j is equal to n. The first passage time probabilities can be found recursively as follows: hij(1)= pij, and for :
Then the expected first passage time μ from state i to state j is calculated from:
Conditional probabilities were used for dry condition predictions according to the prediction scheme proposed by Paulo et al. (Citation2005). It is based on the selection of the most probable state pij(n), which occurs n months later (Xt+n = j, n = 1,2,3). For n = 2, the evaluation of all of the possible paths from the present state i at time point t (Xt = i) to any future state j is determined:
The test statistics to investigate the statistical significance of the sample autocorrelation is computed as follows (Wilks Citation2006):
With the aim of determination of the order of Markov chain, it is prudent to assess the order of memory contained in the series of the analysed variable. This was done with the commonly used Akaike Information Criterion (AIC) in order to choose among the alternative orders of Markov chain. The AIC criterion is based on the log-likelihood function obtained from transition counts and estimated transition probabilities of the fitted Markov chains of different orders (Wilks Citation2006). The exemplary log-likelihoods for s-state Markov chains of order r = 2 are computed:
Long-term time series of developed SPI-SRI classes represent a stochastic process which takes one from the five possible stages in each month. So the defined discrete state and discrete time variables were investigated for having Markov chain properties. To do so, the analysed series of SPI-SRI classes were tested for being generated by a first-order Markov chain against alternative hypothesis of their serial independence. Once the series satisfied the adopted criteria, it was necessarily to assess the order of Markov chain. Further analysis of the statistical characteristics of the recognized Markov chain model provided vital information for drought hazard assessment and prediction. With this aim, the following properties of time series of SPI-SRI classes were investigated in the study:
probabilities of transition from one class to another;
return period of each class;
expected residence time in each class;
expected first passage time from one class to another; and
conditional class prediction for the next three months.
Procedure for calculating SPI and SRI
Series of monthly SPI and monthly SRI values were calculated for the period between 1966 and 2006 for the two study basins. The SRI was computed from monthly median runoff (mm) time series. Using the monthly median instead of monthly mean runoff gave better characteristics of those months with low flows, which contained a very few peaks from individual events of intensive rainfall. The SPI and SRI were calculated according to the methodology developed by Edwards and McKee (Citation1997). A two-parameter gamma distribution was presumed to approximate both monthly precipitation and monthly runoff data. This distribution is skewed to the right with a lower bound of zero, much like precipitation and runoff frequency distributions (Thom Citation1966, Aksoy Citation2000, Nalbantis and Tsakiris Citation2009), and was found suitable for the larger part of Europe (Lloyd Huges and Saunders Citation2002). The SPI and SRI values were found by using an approximation provided by Abramowitz and Stegun (Citation1965) that converts cumulative probability into the standard normal random variable. presents an example of time series of SPI and SRI values for Klodzko meteorological station and Klodzko hydrological station for the period between 1993 and 2006.
Procedure to identify droughts using SPI-SRI indicators and NIZOWKA model
The classification of hydrometeorological conditions obtained according to SPI-SRI indicator was verified with the use of information derived from the NIZOWKA model (Jakubowski Citation2003, Tallaksen Citation2003). The model analyses the stochastic process of streamflow drought and allows the following parameters of drought periods to be characterized: deficit volume and duration of drought events; drought frequency; and the largest drought deficit volume and duration. A sequence of drought events is obtained from the streamflow hydrograph by considering periods when the discharge is below or equal to the Q70 threshold level. Minor droughts that are separated by a short excess period are pooled and the volume of the pooled event is calculated as the sum of the two events. The total duration is the duration from the start of the first event to the end of the second. In the present study, the NIZOWKA model was used to identify hydrological drought events for the period 1966–2006 in the Nysa Kłodzka basin and to provide the parameters of drought duration and deficit volume.
The selected parameters derived from the NIZOWKA model were used to divide the identified hydrological drought events into four categories: (1) droughts of small deficit volume and lasting less than 30 days; (2) droughts of small deficit volume and lasting more than 30 days; (3) droughts of large deficit volume and lasting less than 30 days; and (4) droughts of large deficit volume and lasting more than 30 days. This discrimination between small and large deficit volume (D) was done with reference to the median drought deficit volume (Dm) during the analysed period. The results derived from the NIZOWKA model were upscaled to a monthly time step by assigning the category of the particular drought event to all months during which this event occurred. Consequently, the SPI-SRI class and the category obtained using the NIZOWKA model were attributed to each month. The coincidence of a given SPI-SRI class and the adopted drought categorization was investigated by developing the frequency distribution of a number of months belonging to each SPI-SRI class (0, 1, 2, 3, 4) from the population of months categorized according to NIZOWKA model outputs. This verification procedure was done for the Nysa Kłodzka basin ().
Table 2 Frequency distribution (%) of months belonging to each SPI-SRI class from the population of months categorized according to NIZOWKA model outputs.
RESULTS AND DISCUSSION
Compatibility of drought classes using the NIZOWKA model and SPI-SRI indicators
The results indicate that, for the months categorized as non-drought months according to the NIZOWKA model, the great majority (about 70–90%) corresponded to SPI-SRI classes 0, 1 or 2. Short drought periods (T < 30 days) with low deficit volume corresponded to SPI-SRI classes 0, 1 or 2 in 50 to 70% of cases. Months with droughts of large deficit volume but short time duration were corresponded to SPI-SRI Class 4 in 60% of cases for Kłodzko and Lądek Zdrój and in 33% of cases for Międzylesie. In the latter locations, there were many cases of droughts starting in the middle of the month, which strongly influenced the results. Severe and long-lasting droughts were mainly connected with the months classified as SPI-SRI Class 3. This was recognized for close to 50% of the cases for Międzylesie and more than 60% of the cases for Kłodzko and Lądek Zdrój.
Identification of the order of the Markov chain
Prior to applying Markov model to describe drought properties it is recommended to quantify the nature of the developed drought characteristic. Various authors investigating return periods of droughts have found different levels of persistence in precipitation as well as in streamflow data. The identified order of Markov chain depended on the applied thresholds to distinguish between wet and dry conditions, the analysed time interval and the type of the indicator (Chin Citation1977, Roldan and Woolhiser Citation1982, Şen Citation1990, Fernández and Salas Citation1999, Cancelliere and Salas Citation2004, Citation2010, Bayazit and Önöz Citation2005, Schoof and Pryor Citation2008, Sharma and Panu Citation2008, Citation2010, Citation2012, Akyuz et al. Citation2012).
Time series of SPI-SRI indicator for the 1966–2006 period were analysed as a discrete-state, discrete-time variable with five possible states S = {0,1,2,3,4}. At each time step the process can either stay in the same state or move to another state. All transitions between the states are possible. Both SPI and SRI values are produced by standardization and normalization of the precipitation and runoff data respectively. This operation assures that past and future values of the analysed variable have the same statistical properties, regardless of the time of observation. Different time intervals of SPI and SRI indicator values can be regarded as having the same mean, variance and covariance. Furthermore, the correlation between variables is determined only by their separation in time and not by their absolute position in time. This was the basis for assuming stationarity of the data series of SPI-SRI indicator values. The values of lag-1 autocorrelation were found to vary between 0.2 and 0. 35, while values of lag-2 autocorrelation were below 0.2. Cancelliere and Salas (Citation2010) concluded that, when a time series exhibits a significant autocorrelation, applying a first-order Markov chain model may not be adequate. The estimated low level of persistence for time series of SPI-SRI class legitimized their investigation as a first-order Markov chain (MC1).
The time series of the SPI-SRI class was validated to be generated by homogenous MC1 against the null hypothesis of serial data independence. The developed contingency tables of actual and expected counts under null hypothesis contained 5 × 5 elements representing transitions between all possible states. The distribution of test statistics follows a χ2 distribution with 42 degrees of freedom. For all analysed locations, the test statistic values were greater than χ2 (α = 0.01, ν = 16), which indicated that the null hypothesis can be rejected.
In order to verify whether only values from recent months condition the probability of transition from state i to state j at time t, the AIC criterion was calculated for: (a) a random model (Markov chain of order 0), (b) a Markov chain-1 model (first-order Markov chain, MC1) and (c) a Markov chain-2 model (second-order Markov chain, MC2). According to the adopted criterion (smallest AIC values), the MC1 was selected ().
Table 3 Values of AIC criterion for three analysed models of the time series of SPI-SRI class.
The identified persistence of hydrometeorological conditions reflects the combined properties of meteorological moisture conditions and hydrological moisture conditions on a monthly basis at a local scale.
Conditional probabilities
Transition probability matrices Ps, s = {0,1,2,3,4} contain values of pij representing empirical probabilities to move to state j in the next month under condition of being in state i in the actual month. summarizes the ranges of the obtained probability values for the Nysa Kłodzka and Prosna basins. A similar pattern of the dominant transition probability was recognized for both basins. The highest probability (more than 30%) was recorded for moving from normal, wet or meteorologically dry conditions (classes 0, 1 and 2) to wet conditions. The observed hydrological and meteorologically dry conditions (Class 3) is continued with high probability, reaching 48% in the Prosna basin. Hydrologically dry conditions (Class 4) are likely to be prolonged with 20% probability in the Nysa Kłodzka basin and 30% probability in the Prosna basin. The probability of overlapping drought events (moving from Class 4 to Class 3) ranges from 30% in the Nysa Kłodzka basin to 40% in the Prosna basin.
Table 4 Empirical transition probabilities of moving from state i to j in next month for the Nysa Kłodzka and Prosna basins.
Steady-state probabilities
The values of steady-state probabilities inform about the frequency of a given class. They are calculated as a long-term probability of given classes regardless of the preceding state. The obtained values were presented as a return period of each class expressed in months (). Class 3 was found to be the most frequent one, for both the Nysa Kłodzka and Prosna basins. It was established that both hydrological and meteorologically dry conditions are observed every three months. It appeared that, in the Prosna basin exclusively, meteorologically dry conditions occur less frequently than in the Nysa Kłodzka basin. Still, for both basins, this class is the rarest one.
Table 5 Return period (months) of each class developed for the locations in Nysa Kłodzka and Prosna river basins.
Expected residence time
The expected residence time represents the anticipated duration of persistence of each class. The longest duration time (more than 1.5 months) was established for Class 3 and Class 1 (). The time of residence in hydrological drought phase (Class 4) is expected to last around 1.2 months in the Nysa Kłodzka basin and around 1.3 months in the Prosna basin.
Table 6 Expected residence time of a given SPI-SRI class developed for the locations in the Nysa Kłodzka and Prosna river basins.
First passage time
The obtained values of the expected first passage time were interpreted in terms of drought hazard formation, evolution and persistence. Drought hazard formation was evaluated as the expected number of months required to move from wet conditions to meteorologically dry (1→2) or from normal conditions to meteorologically and hydrologically dry (0→3). Hazard of drought evolution was assessed as the expected number of months required to move from the state of meteorologically dry conditions to hydrologically dry conditions (2→3) or as the number of months required to evolve from hydrometeorologically dry conditions to hydrologically dry (3→4). Hazard of drought persistence was evaluated as the expected number of months to pass from hydrologically dry to normal or wet conditions (3 or 4 → 0 or 1). All of the analysed first passages of times are presented in the form of radar plots summarizing the analysed hazards (). In order to keep the consistency of hazard presentation, hazard of drought persistence was inversed.
Fig. 4 Interpretation of the developed first passage of time in terms of hazard of drought formation, evolution and persistence.
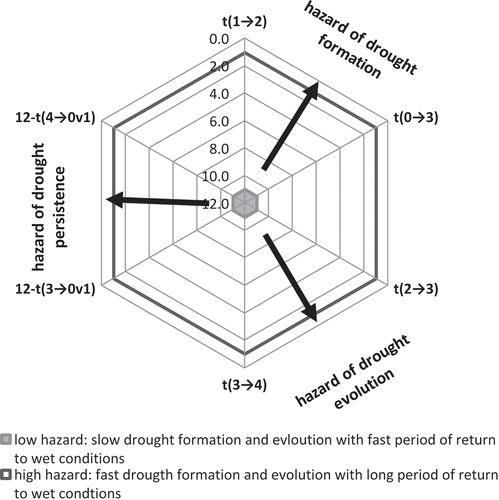
The area of the resulting plots provides information on the magnitude of hazard where the direction of the area towards which it is shifted indicates the dominant source of hazard. The obtained results indicate that there is a greater hazard of meteorological drought formation in the Nysa Kłodzka basin than in the Prosna basin. Also in the Nysa Kłodzka basin there is a slightly greater hazard of remaining in hydrological drought phase once the meteorological drought is finished. However, in the case of the Prosna River basin, it takes longer to move back from hydrologically dry conditions to normal or wet ones ().
Long-term drought hazard predictions
Prediction of a long-term drought hazard was performed for 1-month, 2-month and 3-month time horizons. The resulting plots represent the probability of appearance of hydrologically dry conditions (classes 3 or 4) when the initial hydrological conditions were normal or wet (classes 0, 1 and 2). The ultimate probability of the hydrologically dry condition was found to exceed 40% and is reached in 3 months regardless of the initial state for all of the locations in both analysed basins (). The highest probabilities of hydrologically dry conditions after 1 and 2 months were found for normal initial conditions, the smallest for wet conditions.
Table 7 Probability (%) of arriving at hydrologically dry conditions (class 3 or 4) for the time horizons of 1, 2 and 3 months while the actual month was classified as Class 0, 1 or 2.
The developed methodology focused on runoff, the primary concern for water managers. The works that assess hydrological drought from observed stream gauges are useful for hydrological drought detection, but they are difficult to apply for drought prediction since they do not include information on drought triggers. The combined analysis of SPI-SRI indices allows the propagation of water deficit from meteorological phase to hydrological phase to be accounted for, with the temporal resolution adjusted to regional drought characteristics and climate conditions.
Due to the temperate climate of Poland, the hazard of drought formation may be subject to seasonal variations. Therefore, the research will continue in order to investigate seasonal variability of the analysed characteristics of drought hazard. This will be achieved by analysing five classes of combined SPI-SRI variable as a discrete-state, discrete-time, non-homogenous Markov chain, with the probability of transition between different states depending on the season of a year.
The simultaneous evaluation of meteorological and hydrological conditions and their temporal variability allows different phases of drought and the direction of drought evolution to be determined. The latter is expressed as the probability of transition from one class to another and can be applied for stochastic prediction of drought hazard. The verification of the SPI-SRI classification with the analysis performed using the NIZOWKA model outputs shows great potential in terms of detecting droughts and predicting their development in an operational manner.
CONCLUSIONS
The combined analysis of precipitation and water surplus or deficit was performed using the two complementary indices: SPI and SRI. These two separate indicators of meteorological and hydrological drought, respectively, were combined in order to provide two-variable information on hydrometeorological conditions on a monthly basis. The classes of combined indicator reflected the propagation of water deficit within the hydrological cycle. Drought hazard was investigated in terms of conditions contributing to the drought formation process by looking at the atmospheric and hydrological phases. Stochastic analysis of the developed SPI-SRI indicator allows the dynamics of the transition between drought phases and the recurrence and duration of dry conditions to be assessed. A time series of 40 years of monthly hydrometeorological data represented by SPI-SRI class was analysed as a discrete-state, discrete-time, homogenous Markov chain. A first-order Markov chain was identified as the best fit using the Akaike Information Criterion. The evaluated stochastic properties were summarized in the form of radar plots that allow integrated visualization of the results of drought hazard assessment. The estimated conditional probabilities of transition from one class to another formed the basis of long-term drought hazard prediction.
For the analysed basins, the biggest differences were found in the value of expected time to move from wet conditions to meteorological dry ones and in the value of probability of occurrence of hydrological drought. The Nysa Kłodzka basin was characterized by greater dynamics of changes between the developed classes, while the Prosna basin proved to have greater inertia of the hydrological cycle. For both basins, there is a high coincidence of dry meteorological and hydrological conditions, resulting in severe droughts. The coincidence of dry meteorological and hydrological conditions was observed almost every four months and lasted more than 1.5 months, on average. The duration of hydrologically dry conditions was found to be typically between 1.2 and 1.4 months. The situation when the effect of meteorologically dry conditions is attenuated by hydrological wet conditions within the same month is relatively rare, but still more frequently observed in the Nysa Kłodzka basin (once every 7 months) than in the Prosna basin (once every 9 months). Meteorologically dry conditions lasted typically less than 1.4 months. Good correspondence was found between months classified as hydrometeorologically dry (SPI-SRI Class 3) and drought periods of long duration and large deficit volume indicated by the NIZOWKA model output. Intensive but short-term droughts corresponded to the SPI-SRI Class 4.
REFERENCES
- Abramowitz, M. and Stegun, A., 1965. Handbook of mathematical formulas, graphs, and mathematical tables. New York: Dover Publications, Inc.
- Aksoy, H., 2000. Use of gamma distribution in hydrological analysis. Turkish Journal of Engineering and Environmental Sciences, 24, 419–428.
- Akyuz, D.E., Bayazit, M., and Onoz, B., 2012. Markov chain models for hydrological drought characteristics. Journal of Hydrometeorology, 13, 298–309. doi:10.1175/JHM-D-11-019.1
- Bayazit, M. and Önöz, B., 2005. Probabilities and return periods of multisite droughts. Hydrological Sciences Journal, 50 (4), 605–615. doi:10.1623/hysj.2005.50.4.605
- Cancelliere, A. and Salas, J.D., 2004. Drought length probabilities for periodic-stochastic hydrological data. Water Resources Research, 40, W02503. doi:10.1029/2002WR001750
- Cancelliere, A. and Salas, J.D., 2010. Drought probabilities and return period for annual streamflows series. Journal of Hydrology, 391, 77–89. doi:10.1016/j.jhydrol.2010.07.008
- Changnon, S.A., 1987. Detecting drought conditions in Illinois. Illinois State Survey Circular, ISWS/CIR-169/87, Champaign.
- Chin, E.H., 1977. Modeling daily precipitation occurrence process with Markov chain. Water Resources Research, 13 (6), 949–956. doi:10.1029/WR013i006p00949
- Cinlar, E., 1974. Introduction to stochastic processes. Englewood Cliffs, NJ: Prentice-Hall.
- Debski, K., 1970. Hydrologia. Warszawa: Dział Wydawnictw SGGW.
- Edwards, D.C. and McKee, T.B., 1997. Characteristics of 20th century drought in the United States at multiple timescales. Fort Collins: Colorado State University, Climatology Report No. 97–2.
- Eyton, J.R., 1984. Complementary-color, two-variable maps. Annals of the Association of American Geographers, 74 (3), 477–490. doi:10.1111/j.1467-8306.1984.tb01469.x
- Farat, R., Kasprowicz, T., and Pijawka, I., 2007. Determination of Polish climate variability based on the long homogeneous data. Project DS-K2.7, typescript, IMWM-NRI.
- Fernández, B. and Salas, J.D., 1999. Return period and risk of hydrologic events. II: applications. Journal of Hydrologic Engineering (ASCE), 4 (4), 308–316. doi:10.1061/(ASCE)1084-0699(1999)4:4(308)
- Gabriel, K.R. and Neumann, J., 1962. A Markov chain model for daily rainfall occurrence at Tel Aviv. Quarterly Journal of the Royal Meteorological Society, 88, 90–95. doi:10.1002/qj.49708837511
- Hannaford, J., et al., 2010. Examining the large-scale spatial coherence of European drought using regional indicators of precipitation and streamflow deficit. Hydrological Processes, published online in Wiley InterScience. doi:10.1002/hyp.7725
- Hayes, M.J., et al., 2005. Drought monitoring: new tools for the 21st century. In: D.A. Wilhite, ed. Drought and water crises: science, technology, and management issues. New York: Taylor and Francis.
- Heim, R.R.Jr, 2002. A review of twentieth-century drought indices used in the United States. Bulletin of the American Meteorological Society, 83, 1149–1165.
- Iglesias, G., et al., 2007. Drought management guidelines [online]. Available from: http://sustainabledevelopment.un.org/content/documents/3907MEDROPLAN%20guidelines_english.pdf [Accessed 16 July 2014].
- Jain, S.K., et al., 2010. Application of meteorological and vegetation indices for evaluation of drought impact: a case study for Rajasthan, India. Natural Hazards, 54, 643–656. doi:10.1007/s11069-009-9493-x
- Jakubowski, W., 2003. Guide and computer program NIZOWKA. Mathematic Division. Wroclaw: Agricultural University.
- Keskin, F. and Sorman, A.U., 2010. Assessment of the dry and wet period severity with hydrological index. International Journal of Water Resource and Environmental English, 2 (2), 29–39.
- Komuscu, A.U., 1999. Using the SPI to analyze spatial and temporal patterns of drought in Turkey. Drought Network News, 11 (1), 7–13.
- Kundzewicz, Z. and Matczak, P., 2010. Zagrożenia naturalnymi zdarzeniami ekstremalnymi. Wyd. Biuro Upowszechniania i Promocji Nauki PAN. Nauka, 4 (2010), 77–86.
- Labedzki, L. and Bak, B., 2002. Monitoring suszy za pomocą standaryzowanego opadu. Woda-Środowisko-Obszary Wiejskie, 2 (5), 9–19.
- Liu, L., et al., 2012. Hydro-climatological drought analyses and projections using meteorological and hydrological drought indices: a case study in Blue River basin, Oklahoma. Water Resources Management, 26, 2761–2779.
- Lloyd Hughes, B. and Saunders, M.A., 2002. A drought climatology for Europe. International Journal of Climatology, 22, 1571–1592. doi:10.1002/joc.846
- Lohani, V.K. and Loganathan, G.V., 1997. An early warning system for drought management using the palmer drought index. Journal of the American Water Resources Association, 33 (6), 1375–1386. doi:10.1111/j.1752-1688.1997.tb03560.x
- Lorenc, H., et al., 2006. Susza w Polsce – 2006 (przyczyny, natężenia, zasięg, wnioski na przyszłość), Raport Instytutu Meteorologii i Gospodarki Wodnej, Warszawa.
- Mahalanobis, P., 1936. On the generalized distance in statistics. Proceedings of the National Institute of Sciences of India(Calcutta), 2, 49–55.
- McKee, T.B., Doesken, N.J., and Kleist, J., 1993. The relationship of drought frequency and duration to timescales. Paper presented at: 8th conference on applied climatology (17–22 January 1993, Anaheim, CA). Boston, MA: American Meteorological Society.
- McKee, T.B., Doesken, N.J., and Kleist, J., 1995. Drought monitoring with multiply scales. Paper presented at: 9th conference on applied climatology (15–20 January 1995, Dallas, TX). Boston, MA: American Meteorological Society.
- Mishra, A.K. and Singh, V., 2010. A review of drought concepts. Journal of Hydrology, 391 (1–2), 202–216. doi:10.1016/j.jhydrol.2010.07.012
- Mo, K.C., 2008. Model-based drought indices over the United States. Journal of Hydrometeorology, 9, 1212–1230. doi:10.1175/2008JHM1002.1
- Nalbantis, I. and Tsakiris, G., 2009. Assessment of hydrological drought revisited. Water Resources Management, 23, 881–897. doi:10.1007/s11269-008-9305-1
- Niemeyer, S., 2008. New drought indices. Options Mediterranéennes 80, Series A,.
- Paulo, A.A., 2005. Drought class transition analysis through Markov and Loglinear models, an approach to early warning. Agricultural Water Management, 77, 59–81. doi:10.1016/j.agwat.2004.09.039
- Paulo, A.A. and Pereira, L.S., 2007. Prediction of SPI drought class transitions using Markov chains. Water Resources Management, doi:10.1007/s11269-006-9129–9
- Roldan, J. and Woolhiser, D.A., 1982. Stochastic daily precipitation models: 1. A comparison of occurrence processes. Water Resources Research, 18, 1451–1459. doi:10.1029/WR018i005p01451
- Rossi, G., 2003. Requisites for a drought watch system. In: G. Rossi, et al., eds. Tools for drought mitigation Mediterranean Regions. Dordrecht: Kluwer. 147–157.
- Schoof, J.T. and Pryor, S.C., 2008. On the proper order of Markov chain model for daily precipitation occurrence in the contiguous United States. Journal of Applied Meteorology and Climatology, 47, 2477–2486. doi:10.1175/2008JAMC1840.1
- Şen, Z., 1990. Critical drought analysis by second-order Markov chain. Journal of Hydrology, 120, 183–202. doi:10.1016/0022-1694(90)90149-R
- Sharma, T.C. and Panu, U.S., 2008. Drought analysis of monthly hydrological sequences: a case study of Canadian rivers. Hydrological Sciences Journal, 53, 503–518. doi:10.1623/hysj.53.3.503
- Sharma, T.C. and Panu, U.S., 2010. Analytical procedures for weekly hydrological droughts: a case of Canadian rivers. Hydrological Sciences Journal, 55, 79–92. doi:10.1080/02626660903526318
- Sharma, T.C. and Panu, U.S., 2012. Prediction of hydrological drought durations based on Markov chains: case of the Canadian prairies. Hydrological Sciences Journal, 57 (4), 705–722. doi:10.1080/02626667.2012.672741
- Shukla, S., Steinemann, A., and Lettenmaier, D., 2011. Drought monitoring for Washington state: indicators and applications. Journal of Hydrometeorology [online], 12, 66–83. http://dx.doi.org/10.1175/2010JHM1307.1
- Shukla, S. and Wood, A.W., 2008. Use of a standardized runoff index for characterizing hydrologic drought. Geophysical Research Letters, 35, doi:10.1029/2007GL032487
- Sivakumar, M.V.K. and Wilhite, D.A., 2002. Drought preparedness and drought management. In: Drought mitigation and prevention of land desertification (Proc. Intern. Conf., Bled, Slovenia), UNESCO and Slov. Nat. Com. ICID, Ljubljana, paper 2.
- Steinemann, A., 2003. Drought indicators and triggers: a stochastic approach to evaluation. Journal of the American Water Resources Association, 39 (5), 1217–1233. doi:10.1111/j.1752-1688.2003.tb03704.x
- Tallaksen, L.M. 2003. Background Information NIZOWKA [online]. Available from: http://www.geo.uio.no/edc/software/NIZOWKA/Background_Information_NIZOWKA.pdf [Accessed 15 September 2011].
- Tallaksen L.M. and van Lanen H.A.J., eds., 2004. Hydrological drought – processes and estimation methods for streamflow and groundwater. Developments in water science, 48. Amsterdam: Elsevier Science B.V.
- Thom, H.C.S., 1966. Some methods of climatological analysis. Geneva: World Meteorological Organization, WMO-TN No. 81.
- Tokarczyk, T. and Szalińska, W., 2010. Operacyjny system oceny zagrożenia suszą. In: B. Wiezik, red. Hydrologia w Inżynierii i Gospodarce Wodnej, t. I. . Wyd. PAN Komitet Inżynierii Środowiska, Monografie 68, 285–294.
- Tokarczyk, T. and Szalińska, W., 2013. The operational drought hazard assessment scheme – performance and preliminary results. Archives of Environmental Protection, 0 (0), 93–109. doi:10.2478/aep-2013-0028. ISSN (Online) 2083–4810, ISSN (Print) 2083–4772. July 2013.
- Vasiliades, L., Loukas, A., and Liberis, N., 2011. A water balance derived drought index for Pinios River Basin, Greece. Water Resources Management, 25, 1087–1101. doi:10.1007/s11269-010-9665-1
- Wells, N., Goddard, S., and Hayes, M.J., 2004. A self-calibrating Palmer drought severity index. Journal of Climate, 17, 2335–2351. doi:10.1175/1520-0442(2004)017<2335:ASPDSI>2.0.CO;2
- Wilhite, D.A., 1991. Drought planning: a process for state government. Journal of the American Water Resources Association, 27 (1), 29–38. doi:10.1111/j.1752-1688.1991.tb03110.x
- Wilhite, D.A., 2000. Droughts as a natural hazard: concept and definitions. In: D.A. Wilhite, ed. Drought, a global assessment. Vol. I. London: Routledge Hazards and Disasters Series, 3–18.
- Wilhite, D.A., 2005. Drought and water crises: science, technology, and management issues. Boca Raton, FL: CRC Press.
- Wilks, D.S., 2006. Statistical methods in the atmospheric sciences. 2nd ed. Amsterdam: Elsevier.