Abstract
The process-based Soil and Water Assessment Tool (SWAT) model and the data-driven radial basis neural network (RBNN) model were evaluated for simulating sediment load for the Nagwa watershed in Jharkhand, India, where soil erosion is a severe problem. The SWAT model calibration and uncertainty analysis were performed with the Sequential Uncertainty Fitting algorithm version 2 and the bootstrap technique was applied on the RBNN model to analyse uncertainty in model output. The percentage of data bracketed by the 95% prediction uncertainty (95PPU) and the r factor were the two measures used to assess the goodness of calibration. Comparison of the results of the two models shows that the value of r factor (r = 0.41) in the RBNN model is less than that of SWAT model (r = 0.79), which means there is a wider prediction interval for the SWAT model results. More values of observed sediment yield were bracketed by the 95PPU in the RBNN model. Thus, the RBNN model estimates the sediment yield values more accurately and with less uncertainty.
Editor D. Koutsoyiannis; Associate editor H. Aksoy
Citation Singh, A., Imtiyaz, M., Isaac, R.K., and Denis, D.M., 2014. Assessing the performance and uncertainty analysis of the SWAT and RBNN models for simulation of sediment yield in the Nagwa watershed, India. Hydrological Sciences Journal, 59 (2), 351–364.
Résumé
Le modèle SWAT (Outil d’évaluation du sol et de l’eau) est basé sur les processus et le modèle RBNN (radial basis neural network) est un piloté par les données. Ils ont été évalués pour la simulation de la charge en sédiments du bassin versant de Nagwa (Jharkhand, Inde), où l’érosion des sols est un problème grave. Le calage du modèle SWAT et l’analyse d’incertitude ont été réalisés avec l’algorithme SUFI-2, et la méthode du bootstrap a été appliquée pour analyser l’incertitude de la production du modèle RBNN. Nous avons utilisé le pourcentage de données encadrées par la fourchette de prévision à 95% (95PPU) et le facteur r pour évaluer la qualité de l’étalonnage. La comparaison des résultats des deux modèles indique que la valeur du facteur r du modèle RBNN (r = 0,41) est inférieure à celle du modèle SWAT (r = 0,79), ce qui signifie que l’intervalle de prévision du modèle SWAT est plus étendu. Davantage de valeurs de la charge en sédiments observées sont contenues dans le 95PPU du modèle RBNN. Le modèle RBNN estime donc la production de sédiments de façon plus précise et avec moins d’incertitude.
1 INTRODUCTION
The sediment and nutrients from both agricultural and urban areas often result in increased concentrations of pollutants in downstream water bodies. Excessive erosion of soil from an upstream watershed can impair a stream’s water quality and cause excess biological growth. Damodar Valley Corporation (DVC), Hazaribagh, India, has taken several initiatives to restore and improve the hydrology, reduce sediment loads and nutrient concentrations, and improve the habitat along the Upper Sewani River and its watershed, where erosion is a severe problem. The Nagwa watershed is one of the watersheds which are being monitored by DVC for stream flows and sediment loads only for rainy seasons, i.e. June to October. Proper understanding of the hydrology of the watershed is important for guiding and evaluating the impacts of proposed or ongoing soil and water conservation measures. The use of computer models for modelling the hydrological processes of a watershed can be of great help to watershed management authorities in developing a suitable management programme. In conjunction with monitoring programmes, modelling can also identify critical source areas of non-point source (NPS) pollution for remediation programmes. For the simulation of hydrological processes, several available empirical, physically-based, or conceptual models may be used (Aksoy and Kavvas Citation2005).
In this study, the physically-based Soil and Water Assessment Tool (SWAT) model (Arnold et al. Citation1998) was chosen because of its availability and user-friendliness in handling input data; it was applied to the Nagwa watershed for the prediction of monthly sediment yield. Srinivasan et al. (Citation1998) concluded that SWAT sediment accumulation predictions were satisfactory for Mill Creek watershed located in north central Texas. Arnold and Allen (Citation1999) used the SWAT model to simulate average annual sediment loads for five major Texas river basins and concluded that the SWAT predicted sediment yields compared reasonably well with estimated sediment yields obtained from rating curves. Santhi et al. (Citation2001) found that SWAT-simulated sediment loads matched measured sediment loads well for two Bosque River sub-watersheds. Throughout the world, the SWAT model has been calibrated and validated successfully for different time steps (Jacobs and Srinivasan Citation2005, Setegn et al. Citation2010), suspended sediment production in rivers (Mustafa et al. Citation2012, Santhil Kumar et al. Citation2012), and modelling the discharge–sediment relationship (Ozgur et al. Citation2012). There are a few research outputs of SWAT model applications in India (Tripathi et al. Citation2003, Kaur et al. Citation2004, Mishra et al. Citation2007).
Though SWAT has proved its effectiveness in simulating hydrological processes, it needs several spatial data and detailed description of various physical processes that control the hydrological behaviour of a watershed. In contrast, artificial neural network (ANN) models are data driven and do not need spatial data. This is also one of the drawbacks, but ANN models are good to evaluate and compare the simulation of sediment yield at a single outlet of a given watershed. There are several types of model available for ANN application. Radial basis neural networks (RBNNs) are general purpose networks which can be used for a variety of problems including system modelling, prediction and classification. The major advantage of the RBNN model over other types of neural network is its two-stage training procedure. The first phase of training the network is a clustering phase, in which locations of hidden node centres are computed. After the clustering phase, the radii of the Gaussian functions at the cluster centres are set using a nearest-neighbour procedure. The radius of a given Gaussian function is set to the average distance to the two nearest-cluster centres. Park and Sandberg (Citation1993) proved that a RBNN model with one hidden layer is capable of universal approximation. However, the application of RBNNs to hydrological problems is still rare, although recently it is getting more attention due to its advantages over feed-forward networks. Fernando and Jayawardena (Citation1998) reported that the RBNN is found to perform better than feed-forward network trained with a backpropagation algorithm. Many studies have been carried out using RBNNs for modelling and forecasting of hydrological processes and for sediment transport prediction (e.g. Firat and Gungor Citation2005, Agarwal et al. Citation2006).
Comparison of the SWAT and RBNN models has been the objective of a few research works (Morid et al. Citation2002, Demirel et al. Citation2008, Talebizadeh et al. Citation2010). This study aims to compare the performances of two different types of modelling approach, namely SWAT as a physically-based model and RBNN as a black-box model, in simulating monthly sediment yield. As the models are nowadays being used to support decisions about alternative management strategies, it is important for these models to undergo a careful calibration and uncertainty analysis. Sources of model structural uncertainty include processes not accounted for in the model, such as unknown activities in the watershed, and model inaccuracy due to over-simplification of the processes considered in the model. Uncertainties in the outputs of both RBNN and SWAT models will also be analysed herein. In the current study, SWAT was evaluated by performing calibration and uncertainty analysis using the Sequential Uncertainty Fitting algorithm version 2 (SUFI-2), which is a semi-automated inverse modelling procedure for combined calibration-uncertainty analysis. The bootstrap technique was applied to perform uncertainty analysis of the RBNN model.
2 MATERIALS AND METHODS
2.1 Study area and preparation of spatial data
Keeping in view the objectives and the availability of hydrological and meteorological data, a small watershed named Nagwa, located in the Upper Damodar Valley in the DVC, Hazaribagh, India, was selected. The watershed is approximately 92.46 km2, of which about 30–40% is under shrubs and forest, and the remaining area under cultivation. The average elevation of the watershed is 540 m a.m.s.l. and it is bounded by latitudes 23°59′08″–24°05′41″ N and longitudes 85°16′35″–85°23′45″ E. A location map of the study area is presented in .
Satellite data obtained from Thematic Mapper sensor for path no. 141 and row no. 043 (spectral band: 0.45–2.35 µm) for 14 October 2006 was used to prepare a land-use and land-cover (LULC) map of the Nagwa watershed. All the layers were stacked and the area of interest was taken out. The supervised method of classification was used to classify the land uses. The maximum likelihood was used as a parametric rule in performing the classification. The land-use classes used include agriculture, dense forest, water, fallow land and urban settlement. The kappa coefficient, κ, which ranges from 0 to 1, was used for measuring overall accuracy (Congalton et al. Citation1983). The overall classification accuracy of 96.23% and κ of 0.94 were achieved. ArcGIS 9.3.1 was used to analyse the satellite digital data, digitization of contours, construction of a digital elevation model (DEM), automatic extraction of watershed parameters and interpretation of results. The extracted watershed information was used to generate the input parameters of ArcSWAT 2009.93.4. Development and construction of the RBNN model was carried out with NeuralWare Pro Plus II™.
2.2 Model description
2.2.1 SWAT model
The SWAT model is a physically-based, semi-distributed parameter and watershed-scale model that works on a continuous daily time step. It simulates hydrological processes, sediment yield, nutrient loss and pesticide losses into surface water and groundwater, and the effects of agricultural management practices on water in large watersheds (Arnold et al. Citation1998). The SWAT model incorporates the effects of weather, surface runoff, evapotranspiration, crop growth, irrigation, groundwater flow, nutrient loading, pesticide loading and water routing, as well as the long-term effects of varying agricultural management practices (Neitsch et al. Citation2005). In this study, however, the focus is on only the hydrological component of SWAT. The model requires input of the DEM, land-use and soil maps, as well as weather data such as daily precipitation and temperature. The watershed is divided into small sub-basins that are further subdivided into hydrological response units (HRUs) based on unique land cover, soil and topographic conditions. The hydrology component of the model determines a soil water balance at each time step based on daily data of precipitation, runoff, evapotranspiration, percolation and baseflow. The SWAT model can be used to simulate a single watershed, or a system of multiple hydrologically connected watersheds; it calculates the surface erosion within each HRU using the Modified Universal Soil Loss Equation (MUSLE) (Williams Citation1975):
SWAT model application
The Soil Conservation Service Curve-Number (CN) method was used to estimate surface runoff (USDA-SCS Citation1972). The CN is a function of the permeability of soil, land use and antecedent soil moisture conditions. The watershed parameterization and the model input were derived using the ArcSWAT 2009.93.4, which provides a graphical support to the disaggregation scheme and allows the construction of the model input from digital maps. The input parameters of the model were extracted from the DEM analysis, satellite imagery, soil maps and field observations. The Nagwa watershed was subdivided into 19 sub-basins. The parameterization of the stream reaches and the sub-basin geomorphology are carried out automatically by the interface. For creation of HRUs, the SWAT model needs LULC, soil and slope layers and their threshold inputs. For HRU definition, four classes of soil type (loamy, clay, sandy loam and loamy sandy) and five classes of land-use category were used. The LULC map layer was reclassified into SWAT land cover/plant types and the soil map was also connected to the SWAT soil database with the corresponding soil properties. HRU analysis in ArcSWAT includes division of HRUs by slope class in addition to land use and soils. In this study, multiple slopes with three classes of slope discretization (0–1%, 1–4% and 4–9999%) were evaluated. The SWAT model was run for several simulations with different values of input parameters to get an adequately calibrated model. All the values of the input parameters were chosen within the defined limits of the parameter values and on scientific understanding of the hydrological processes of the watershed. Single weather station data for the entire watershed have been used, because of the availability of only one gauging station in the entire Nagwa watershed.
The Latin hypercube one-factor-at-a-time (LH-OAT) method of sensitivity analysis, as proposed by Morris (Citation1991), was used in this study; this was implemented in SWAT. The ArcSWAT 2009.93.4 interface for SWAT 2009 consists of a number of tools that can be used to evaluate the sensitivity of a parameter, aid in model calibration and assess parameter uncertainty (Veith and Ghebremichael Citation2009). This global sensitivity analysis approach has the advantage of being quite fast compared to similar procedures and, as a result, one does not obtain an absolute measure of the sensitivity but rather a ranked order of the parameters. The parameters affecting sediment yield were tested for sensitivity analysis; these included: a linear parameter for calculating the maximum amount of sediment that could be re-entrained during channel sediment routing; a channel cover factor; a channel erodibility factor; a USLE support practice factor; an exponent parameter for calculating sediment re-entrained in channel sediment routing; and a USLE factor for land cover/plant type. The monthly calibration and validation of the SWAT model for sediment yield were performed after conducting sensitivity analysis. The SUFI-2 algorithm was employed to fine tune the manually calibrated SWAT model. The calibration process was started initially with ranked parameters obtained during the sensitivity analysis, and only six parameters were found to be sensitive to sediment yield in the fourth and last iterations. In each iteration, a total of 1000 simulations were performed. Seventeen years of meteorological and measured sediment yield data were used for calibration and validation. The periods 1991–2004 (14 years) and 2005–2007 (3 years) were used for calibration and validation, respectively, including 2 years of warm-up period (1991 and 1992). The warm-up period minimizes the effect of the estimated initial state variables such as soil water content and surface residue (Zhang et al. Citation2007).
Uncertainty analysis using SUFI-2
As hydrological models are increasingly being used for deciding on the appropriate soil and water conservation measures and management programmes to take up, it is logical that they need to be calibrated and validated, with due care given to sensitivity and uncertainty analysis (Abbaspour et al. Citation2010). There are three major sources of uncertainty in the outputs of a hydrological model: structural uncertainty, input uncertainty and parameter uncertainty. The structural uncertainity stems from adopting a set of assumptions to simplify the modelling of the desired process. The uncertainty in input and model parameters may be induced, respectively, by the error in various meteorological inputs, such as rainfall and temperature, and errors related to the non-uniqueness of sets of model parameters (Abbaspour Citation2008). Some examples of this type of uncertainty are: the effects of reservoirs and wetlands on hydrology; interactions between surface water and groundwater; landslides, and major construction of roads, dams and bridges, which could produce considerable amounts of sediment during short timespans; excessive application of fertilizers and pesticides; and unrecorded irrigation activities and water diversions. Input uncertainty is associated with spatially interpolated measurements of model input or initial conditions (Yang et al. Citation2008). The uncertainties are quantified by a measure known as the P factor, which is the percentage of observed data bracketed by the 95% prediction uncertainty (95PPU). The 95PPU is calculated at the 2.5% and 97.5% levels of the cumulative distribution of an output variable obtained through Latin hypercube sampling. This is calculated by the 2.5th and 97.5th percentiles of the cumulative distribution of every simulated point. The goodness of fit is assessed by the uncertainty measures calculated from the percentage of measured data bracketed by the 95PPU band, and the average distance d between the upper and the lower 95PPU can be determined from:
where σx is the standard deviation of the measured variable x. A value of less than 1 is a desirable measure for the r factor. The goodness of fit and the degree to which the calibrated model accounts for uncertainties are assessed by the above two measures. Theoretically, the value of the P factor ranges between 0% and 100%, while that of the r factor ranges between 0 and infinity. A P factor of 1 and r factor of zero indicates that a simulation exactly corresponds to the measured data. SUFI-2 seeks to bracket most of the measured data (large P factor, maximum 100%) with the smallest possible value of r factor (minimum 0). Parameter uncertainty increases with increased uncertainty in the output. SUFI-2 starts by assuming a large parameter uncertainty within a physically justifiable range, so that the measured data initially falls within the 95PPU, then decreases this uncertainty in steps while monitoring the P factor and r factor. In each step, previous parameter ranges are updated by calculating the sensitivity matrix, and the equivalent of a Hessian matrix, followed by the calculation of a covariance matrix, 95% confidence intervals of the parameters, and correlation matrix. Parameters are then updated in such a way that the new ranges are always smaller than the previous ranges, and are centred on the best simulation (Abbaspour et al. Citation2007). If observed values are of good quality, then 80–100% of the observed data should be bracketed by the 95PPU, while poor quality data may contain many outliers and it may be sufficient to bracket only 50% of the observed data in the 95PPU. Further, the average distance between the upper and lower levels of 95PPU should be smaller than the standard deviation of the observed data. A balance was made between the two measures to ensure bracketing most of the observed data within the 95PPU, while seeking the smallest possible uncertainty band.
2.2.2 RBNN model
A RBNN, developed by Powell (Citation1987) and Broomhead and Lowe (Citation1988), has an input layer, a hidden layer and an output layer. The neurons in the hidden layer contain Gaussian transfer functions whose outputs are inversely proportional to the distance from the centre of the neuron. RBNNs have a variable number of neurons that is usually much less than the number of training points, and they can be used for a variety of problems including system modelling, prediction and classification. In general, a RBNN is any network which makes use of radially symmetric and radially bounded transfer functions in its hidden layer. The Euclidean distance is computed from the point being evaluated to the centre of each neuron, and a radial basis function is applied to the distance to compute the weight for each neuron. The radial basis function is so named because the radius distance is the argument to the function. The Gaussian activation function is mostly used as activation function for the training data set:
where x is the input sets of training, cj is the centre value and σ is the variance. During the training of the data set, the variance and centre determine the properties of each function. The response of each hidden unit is scaled by its connecting weights to the output units and then summed to produce the overall network output. The response of network is calculated by:
where ψj(x) is the response of the jth hidden neuron, wjk is the weight coefficient between the hidden unit j and the kth output unit, and w0 is the bias. In the RBNN model, the radial basis function can be used for constructing the input vectors. The length of input data set, location of neurons and determination of other training parameters are important during the training of the RBNN. The location of the first centre is chosen from the training data set, and the standard deviation σ (i.e. width) of the jth neuron can be expressed as:
where dmax is the maximum distance between the training data set, is the response of the network and yobs is the observed value. The training process continues until the error reaches an acceptable value. If there is more than one predictor variable, then the radial basis function has as many dimensions as there are variables. The best predicted value for the new point is found by summing the output values of the radial basis functions multiplied by weights computed for each neuron. The radial basis function for a neuron has a centre and a radius, or a spread. The radius may be different for each neuron in each dimension.
At the beginning of training, the weights were initialized with a set of random values and systematically changed by the learning algorithm in such a way that the difference between the RBNN output and the actual output was small for a given input. The root mean square error (RMSE), correlation coefficient (R) and coefficient of determination (R2) over the training samples were the objective functions to be minimized. In the case of the SWAT model, results were obtained from the period 1993–2004 because 1991 and 1992 were used as warm-up periods, and the simulation of warm-up periods was not included in the analysis. For fair comparison, the daily rainfall and stream flow for the monsoon season (June–October) for the 1993–2004 period were taken as input to train the model. The RBNN model was validated for the period 2005–2007. For the present study, a three-layer feed-forward neural network with the gradient descent algorithm was used for learning. Four sets of input data were used for determining the type and number of inputs to the RBNN model (). Eighty percent of input data were selected for training and the remaining 20% as the validation data set. Since the number of neurons in the hidden layer plays an important role in the model performance, 2–30 neurons were tested. Beyond six neurons, no considerable difference was noticed; therefore, six neurons were accepted. The best neural network structure was selected based on the performance criteria (RMSE, R2 and R). The input data were arranged sequentially in date order and the daily output was later aggregated into monthly values for comparison with the SWAT model.
Table 1 Best neural network architecture and performance criteria for each scenario for the RBNN model.
Uncertainty analysis using the bootstrap technique
The sources of uncertainty related to the neural network model are noisy and subject to incomplete training data, model limitations regarding local minima, sub-optimal optimization procedures and inappropriate model parameterization (Talebizadeh et al. Citation2010). In this study, uncertainties originating from random sampling of the training data set and different network initialization were considered. The bootstrap technique was employed for uncertainty analysis of the RBNN models. The bootstrap technique uses intensive resampling with replacement, in order to reduce uncertainty. It is a simple approach, since it does not require the complex computations of derivatives and Hessian-matrix inversion involved in linear methods, or the Monte Carlo solutions of the integrals involved in the Bayesian approach (Dybowski and Roberts Citation2000). The bootstrap technique can also be used to produce statements about probabilities, to generate inferences about true parameters, or to determine confidence intervals. Bootstrap sampling requires that B bootstrap samples be drawn at random with replacement from the original training set of np input–output patterns, D {x,y}. The generic bth sample is constituted by the same number np of input–output patterns drawn among those in D, although, due to the sampling with replacement, some of the patterns in D will appear more than once in Db, whereas some will not appear at all. Each bootstrap set Db was used as a data set for training a different neural network to give a regression function
where
is the obtained network weight values. For a new input
, the bootstrap estimate
is given by the average of the
regression functions:
and the bootstrap estimate of the variance
is given by:
2.3 Model evaluation techniques
The model performance was evaluated using three well-known statistical criteria, the coefficient of determination (R2), the Nash-Sutcliffe efficiency (NSE) and percent bias (PBIAS). The R2 ranges from 0 to 1 and explains the proportion of variance in observed data, with higher values indicating less error variance; the NSE is a normalized statistic and estimates the relative magnitude of the residual variance as compared to the observed (Nash and Sutcliffe Citation1970), and demonstrates how well the plot of observed versus simulated data fits the 1:1 line; and PBIAS has the ability to indicate poor model performance and measures the average tendency of the simulated data to be larger or smaller than observed data. Low values of PBIAS indicate accurate model simulation, positive values indicate model underestimation bias, and negative values indicate model overestimation bias (Gupta et al. Citation1999). The values of R2, NSE and PBIAS are determined by equations (10)–(12), respectively:
where Ysim and Yobs are the simulated values and observed values, respectively; is the mean of n observed values; and
is the mean of n simulated values.
3 RESULTS AND DISCUSSION
3.1 SWAT model
The total monthly sediment yield at the reach outlet that was carrying the outlet of the whole watershed was simulated. The performance of the model for simulating hydrological variables was evaluated with the help of statistical tests as described in Section 2. Depending on data availability, the potential evapotranspiration can be estimated using different methods available in the SWAT model, and the Hargreaves-Samani method was applied in this study. The Muskingum method of routing was selected to route water through the channel network.
The major parameters affecting sediment yield were modified to increase agreement between the simulated and observed monthly sediment yield. During calibration of the SWAT model, the final range of parameters represented the characteristics of the watershed. The curve number was adjusted within the range ±10% from the curve number value for moisture condition II. These curve numbers were also adjusted for slopes greater than 4%. For simulation of the baseflow in the watershed, the baseflow recession constant was adjusted to 0.05. This is directly proportional to groundwater flow response to changes in recharge. Groundwater delay time was adjusted to 42 days. This represents the lag between the times that water exits the soil profile and enters the shallow aquifer. The groundwater revap coefficient represents the rate of transfer of water from the shallow aquifer to the root zone; this was adjusted to 0.02. The soil evaporation compensation factor was adjusted to 0.70. The calibrated values disclose the response of land cover, land management practices, soil properties and topographic condition of the watershed.
The calibration process significantly reduced the difference between the measured and simulated sediment yield. The extent of sediment yield from a watershed is related to the complex interaction between topography, climate, soil, vegetation and land use. The sediment parameters considered during the calibration process were the USLE C factor for water erosion applicable to land cover, the USLE equation support practice factor, a linear parameter for calculating the maximum amount of sediment that can be re-entrained during channel sediment routing, the channel cover factor, the channel erodibility factor and an exponent parameter for calculating sediment re-entrained in channel sediment routing. These parameters were adjusted to the level where they could represent the characteristics of the existing land use and topographic condition of the watershed. The final fitted values are listed in .
Table 2 Sensitivity parameters for sediment yield prediction and calibrated values. USLE_P: USLE equation support practice factor; Ch_Erod: channel erodibility factor; Spcon: linear parameter for calculating the maximum amount of sediment that can be re-entrained during channel sediment routing; Spexp: exponent parameter for calculating sediment re-entrained in channel sediment routing; Ch_Cov: channel cover factor; USLE_C: USLE C factor for land cover/plant type.
shows average monthly rainfall, surface runoff and sediment yield in the study area during the monsoon period. It is evident from that the higher the rainfall, the higher was the surface runoff and the sediment yield. Rainfall and runoff are the main contributing factors for the detachment, transport and deposition of sediment particles. During onset of the monsoon, i.e. the first week of June, most of the water is lost through infiltration and other losses, thereby reducing sediment yield at the outlet. In fact, sediment concentration increased rapidly during June. Sediment yield and runoff reached a peak in September. Higher values of sediment yield are observed during August and September for both the calibration and validation periods. Time series of observed and simulated monthly sediment yield are compared graphically in , which shows that the trend of simulated monthly sediment yield follows well the observed sediment yield during the calibration period. The over-production of total sediment is contributed mainly by the high rains during the month of June, and the model simulated a high rate of sediment based on the rainfall quantity. The overall prediction of the monthly sediment yield during the whole calibration period was in close agreement with its observed values. The scatter plot between the observed and simulated monthly sediment yield, along with the regression line, are presented in for the calibration and validation periods. shows an even distribution of the simulated values about the regression line for higher measured values. A close relationship between observed and simulated sediment yields is indicated by values of R2 of 0.78 and 0.68, and NSE values of 0.76 and 0.66, respectively.
Fig. 2 Correlation between monthly (monsoon period) distribution of rainfall, runoff and sediment yield for calibration and validation periods.
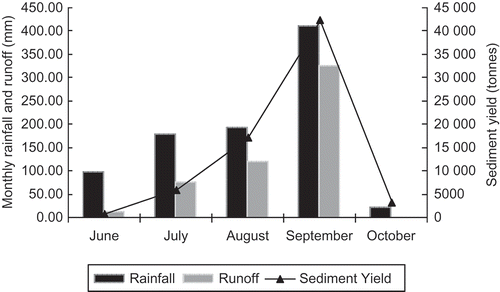
3.2 RBNN model
The major inputs, such as daily rainfall and runoff, were used to develop the neural network model for predicting sediment yield. The number of neurons in the hidden layer was determined by trial and error, and for each scenario the best neural network structure was chosen based on R2, R and RMSE values. Initially, only average daily discharge was used as the input. Later, daily rainfall was added and improved results were obtained. It is evident from that adding the previous day’s average discharge did not have a significant effect on the output. Hence, the RBNN model with daily rainfall and daily average discharge as its input was taken as the optimum neural network model for the present study. The trend of simulated monthly sediment follows quite well the observed sediment yield during the training period (). The model under-predicted in the year receiving high rainfall. The overall prediction of the monthly sediment yield during the whole calibration period was in close agreement with its observed values. A scatter plot between observed and predicted sediment yield data provides a strong basis to assess the accuracy of a model. The closer the scatter points are to the line of best fit, the better the model. presents the scatter plot between the observed and simulated monthly sediment yield together with the regression line for the calibration and validation periods. shows an even distribution of the simulated values about the regression line, especially for lower and medium measured values. The inability of the RBNN model to estimate large values of sediment load can be attributed to different nonlinear relationships governing the process of sediment detachment and final sediment load generated from a basin. The R2 and NSE values obtained were 0.91 and 0.90, respectively, during the training period. The trained model was validated for the period June–October, for the years 2005–2007 for sediment yield loss from a small watershed. The scatter plot for the validation period (2005–2007) shown in indicates the coefficient of determination, R2 = 0.88 and NSE = 0.83, which demonstrate that the model predicted closely the observed values of sediment yield.
3.3 Uncertainty analysis of SWAT and RBNN models
Although the uncertainties of outputs related to RBNN and SWAT models were compared in this study, it is worth mentioning that the sources of uncertainty in the two models and the way that the uncertainties were estimated are completely different. In the RBNN model, estimation of uncertainty was achieved by training the model with different training sets and initialization conditions, whereas in the SWAT model uncertainty was estimated through the SUFI-2 procedure and expressed in terms of parameter uncertainty. Calibration of models at the watershed scale is a challenging task because of the possible uncertainties that may exist in the form of process simplification, processes not accounted for by the model, and processes in the watershed that are unknown to the modeller. In SWAT, rainfall and temperature data for every sub-basin are furnished by the station nearest to the centroid of the sub-basin. Direct accounting of rainfall or temperature distribution error is quite difficult, as information from many stations would be required. A common problem in the prediction of sediment is that of the second-storm effect. After a storm, a small amount of sediment is detached and the remaining surface layer is more difficult to mobilize. Hence, sediment loads of a consequent similar storm or even a larger second or third storm could be smaller. The model produces a good simulation of sediment load for the first storm, while in the second and the third storms it overestimates the load.
The 95PPU of SWAT and RBNN models with the monthly observed and best-simulated sediment for the calibration and validation periods are illustrated in and , respectively. The shaded region (95PPU), which is the simulation result, quantifies all uncertainties because it brackets a large amount of the measured data, which contain all uncertainties. The calibration and uncertainty results of the SWAT model are not unique, as calibration of a model depends on several factors, such as the number of parameters, calibration procedure, type of objective function to be minimized and number of measured variables. The 95PPUs are the combined outcome of the uncertainties in the hydrological model, the parameters and the input data. In SUFI-2, these uncertainty sources are not separately estimated but are attributed as total model uncertainty to the parameters. Each hydrological model suffers from conceptual model uncertainties and this is true especially for large watershed models, in which many natural or man-made processes are not represented. The results of SWAT monthly sediment calibration for Nagwa watershed are shown in . The P factor values range from 0.67 to 0.82, while those of the r factor range from 0.79 to 0.88. The calibration and validation statistics show larger uncertainties. It is the smaller sediment values that are mostly not bracketed by the prediction band. This is probably due to the poor accuracy and the dispersed nature of the measured data. Comparison of the results of the two models indicates that the value of r factor in the RBNN model of 0.41 () is less than its counterpart in the SWAT model (r = 0.79), which means there is a wider prediction interval in the results of the SWAT model. The value of P factor related to each model shows that the percentage of observed sediment values bracketed by the 95PPU in the RBNN model of 0.92 is higher than the P factor of 0.82 in the SWAT model. In other words, the RBNN model estimates the sediment load values more accurately and with less uncertainty. The SWAT model overestimated sediment yield by 11% during calibration and by 10% during validation, as shown by the PBIAS values. However, the RBNN model underestimated sediment yield by 2% during calibration and overestimated it by 9% during validation.
4 CONCLUSIONS
An attempt was made to calibrate and validate the SWAT model, a process-based model, and the RBNN model, a data-driven neural network model, along with their corresponding uncertainties to simulate sediment yield for a watershed where erosion and water quality problems exists. The SWAT model calibration and uncertainty analysis were performed with SUFI-2, whereas bootstrap methodology was applied for the uncertainty analysis of the RBNN model. Four scenarios of input data were considered and it was observed that daily rainfall and average daily discharge together improved the performance of the RBNN model in the estimation of sediment load. Generally, both the model predictions were close to the measured values during calibration and validation. The RBNN model performed better than the SWAT model in simulating sediment yield at the single outlet of the study watershed. The value of r factor was 0.41 in the case of the RBNN model as compared to 0.79 for the SWAT model. This indicates a wider prediction interval for the results of the SWAT model. Further, the RBNN model was able to bracket more measured data (P = 0.92) by 95PPU than the SWAT model. The uncertainty analysis results indicate that the RBNN model can estimate the sediment yield values more accurately and with less uncertainty. To study the simulation of sediment yield at a single outlet of a similar watershed, the RBNN model could be employed as an alternative, or for estimation of missing data. There is a need to create large databases in India, for improving RBNN or other ANN models for sediment yield estimation and prediction.
Acknowledgements
The authors would like to thank the Department of Soil Conservation, Damodar Valley Corporation, Hazaribagh, Jharkhand, India, for sharing their data for this research work.
REFERENCES
- Abbaspour, K.C., et al., 2007. Modelling hydrology and water quality in the pre-alpine/alpine Thur watershed using SWAT. Journal of Hydrology, 333 (2–4), 413–430.
- Abbaspour, K.C., 2008. SWAT-CUP2: SWAT calibration and uncertainty programs—a user manual. Duebendorf: Department of Systems Analysis, Integrated Assessment and Modelling (SIAM), Eawag, Swiss Federal Institute of Aquatic Science and Technology.
- Abbaspour, K.C., Vejdani, M., and Srinivasan, R., 2010. SWAT-CUP: a calibration and uncertainty analysis program for SWAT. In: International SWAT conference, 14–16 August. College Station and Goyang-Si Gyeonggi-Do: Texas A & M University and Korea Institute of Construction Technology, 21.
- Agarwal, A., et al., 2006. Simulation of runoff and sediment yield using artificial neural networks. Biosystems Engineering, 94 (4), 597–613.
- Aksoy, H. and Kavvas, M.L., 2005. A review of hillslope and watershed scale erosion and sediment transport models. Catena, 64 (2–3), 247–271.
- Arnold, J.G., et al., 1998. Large area hydrologic modelling and assessment part I: model development. Journal of American Water Resources Association, 34 (1), 73–89.
- Arnold, J.G. and Allen, P.M., 1999. Automated methods for estimating baseflow and groundwater recharge from stream flow records. Journal of American Water Resources Association, 35 (2), 411–424.
- Broomhead, D.S. and Lowe, D., 1988. Multivariable functional interpolation and adaptive networks. Complex System, 2, 321–355.
- Congalton, R., Oderwald, R., and Mead, R., 1983. Assessing Landsat classification accuracy using discrete multivariate analysis statistical techniques. Photogrammetry Engineering and Remote Sensing, 49 (12), 1671–1678.
- Demirel, M.C., Venancio, A., and Kahya, E., 2008. Flow forecast by SWAT model and ANN in Pracana basin, Portugal. Advance Engineering Software, 40 (7), 467–473.
- Dybowski, R. and Roberts, S.J., 2000. Confidence and prediction intervals for feed-forward neural networks. In: R. Dybowski and V. Gant, eds. Clinical applications of artificial neural networks. New York: Cambridge University Press, 298–326.
- Fernando, A.K. and Jayawardena, A.W., 1998. Runoff forecasting using RBF networks with OLS algorithm. Journal of Hydrologic Engineering, 3, 203–209.
- Firat, M. and Gungor, M., 2005. Estimation of suspended sediment amount by radial basis neural networks. In: 2nd Water engineering symposium. Izmir: Izmir Institute of Technology, 682–693.
- Gupta, H.V., Sorooshian, S., and Yapo, P.O., 1999. Status of automatic calibration for hydrologic models: momparison with multilevel expert calibration. Journal of Hydrologic Engineering, 4 (2), 135–143.
- Jacobs, J.H. and Srinivasan, R.. 2005. Application of SWAT in developing countries using readily available data. In: 3rd international SWAT conference, 11–15 July. Zurich and College Station: Swiss Federal Institute for Environmental Science and Technology (EAWAG) and Texas A & M University.
- Kaur, R., et al., 2004. Comparison of a subjective and a physical approach for identification of priority areas for soil and water management in a watershed: a case study of Nagwan watershed in Hazaribagh District of Jharkhand, India. Environmental Modelling Assessment, 9 (2), 115–127.
- Mishra, A., Kar, S., and Singh, V.P., 2007. Prioritizing structural management by quantifying the effect of land use and land cover on watershed runoff and sediment yield. Water Resources Management, 21 (11), 1899–1913.
- Morid, S., Gosain, A.K., and Keshari, A.K., 2002. Comparison of the SWAT model and ANN for daily simulation of runoff in snowbound ungauged catchments. In: Fifth international conference on hydroinformatics. Cardiff: International Water Association Publishing.
- Morris, D., 1991. Factorial sampling plans for preliminary computational experiments. Technometrics, 33 (2), 161–174.
- Mustafa, M.R., et al., 2012. River suspended sediment prediction using various multilayer perceptron neural network training algorithms—a case study in Malaysia. Water Resources Management, 26 (7), 1879–1897.
- Nash, J.E. and Sutcliffe, J.V., 1970. River flow forecasting through conceptual models: part I. A discussion of principles. Journal of Hydrology, 10 (3), 282–290.
- Neitsch, S.L., et al., 2005. Soil and water assessment tool (SWAT), theoretical documentation. Temple, TX: Blackland Research Center, Grassland, Soil and Water Research Laboratory, Agricultural Research Service.
- Ozgur, K., Ozkan, C., and Bahriye, A., 2012. Modelling discharge–sediment relationship using neural networks with artificial bee colony algorithm. Journal of Hydrology, 428–429, 94–103.
- Park, J. and Sandberg, I.W., 1993. Universal approximations using radial basis function networks. Neural Computation, 3 (2), 246–257.
- Powell, M.J.D., 1987. Radial basis functions for multivariable interpolation: a review. In: J.C. Mason, and M.G. Cox, eds. Proceedings of IMA conference on algorithms for approximation. New York: Oxford University Press, 143–167.
- Santhi, C., et al., 2001. Validation of the SWAT model on a large river basin with point and non point sources. Journal of American Water Resources Association, 37 (5), 1169–1188.
- Senthil Kumar, A.R., et al., 2012. Modelling of suspended sediment concentration at Kasol in India using ANN, Fuzzy logic and decision tree algorithms. Journal of Hydrologic Engineering, 17 (3), 394–404.
- Setegn, S.G., et al., 2010. Modelling of sediment yield from Anjeni-Gauged watershed, Ethiopia using SWAT Model. Journal of American Water Resources Association, 46 (3), 514–526.
- Srinivasan, R., et al., 1998. Large area hydrologic modelling and assessment part II: model application. Journal of American Water Resources Association, 34 (1), 91–101.
- Talebizadeh, M., et al., 2010. Uncertainty analysis in sediment load modelling using ANN and SWAT model. Water Resources Management, 24 (9), 1747–1761.
- Tripathi, M.P., Panda, R.K., and Raghuwanshi, N.S., 2003. Identification and prioritization of critical sub-watersheds for soil conservation management using the SWAT model. Biosystems Engineering, 85 (3), 365–379.
- USDA-SCS (United States Department of Agriculture – Soil Conservation Service), 1972. National engineering handbook, Section 4 Hydrology, Chapters 4–10, Washington, DC: United States Department of Agriculture – Soil Conservation Service.
- Veith, T.L. and Ghebremichael, L.T., 2009. How to: applying and interpreting the SWAT Auto-calibration tools. In: Fifth international SWAT conference proceedings, 5–7 August. Boulder and College Station: University of Colorado and Texas A & M University.
- Williams, J.R., 1975. Sediment routing for agricultural watersheds. Water Resources Bulletin, 11 (5), 965–974.
- Yang, J., et al., 2008. Comparing uncertainty analysis techniques for a SWAT application to the Chaohe Basin in China. Journal of Hydrology, 358, 1–23.
- Zhang, X., Srinivasan, R., and Hao, F., 2007. Predicting hydrologic response to climate change in the Luohe river basin using the SWAT model. American Society of Agricultural and Biological Engineers, 50 (3), 901–910.