Abstract
The rating curve model (RCM) proposed by Moramarco and co-authors is modified here for flood forecasting purposes without using rainfall information. The RCM is a simple approach for discharge assessment at a river site of interest based on relating the local recorded stage and the remote discharge monitored at an upstream gauged river site located some distance away. The proposed RCM for real-time application (RCM-RT), involves only two parameters and can be used for river reaches where significant lateral flows occur. The forecast lead time depends on the mean wave travel time of the reach. The model is found to be accurate for a long reach of the Po River (northern Italy) and for two branches of the Tiber River (central Italy) characterized by different intermediate drainage areas and wave travel times. Moreover, the assessment of the forecast uncertainty coming from the model parameters is investigated by performing a Monte Carlo simulation. Finally, the model capability to accurately forecast the exceedence of fixed hydrometric thresholds is analysed.
Editor D. Koutsoyiannis; Associate editor C. Perrin
Résumé
Le modèle de courbe de tarage (MCT) proposé par Moramarco et co-auteurs a été modifié afin de prévoir des crues sans avoir recours à des informations sur les précipitations. Le MCT est une approche simple pour l’évaluation du débit en un site de la rivière étudiée combinant le niveau mesuré localement et le débit mesuré sur une station de jaugeage située à quelque distance à l’amont. Le modèle de prévision proposé pour l’application en temps réel (MCT-TR) ne comporte que deux paramètres, et peut être utilisé pour des biefs de rivière comportant d’importants écoulements latéraux. L’horizon de prévision dépend de la durée moyenne de propagation de l’onde de crue dans le bief. Le modèle s’est révélé suffisamment précis pour un long bief du Po (Nord de l’Italie) et pour deux branches du Tibre (Italie centrale) dont les zones de drainage intermédiaires et les temps de propagation étaient différents. L’évaluation de l’incertitude des prévisions provenant des paramètres du modèle a en outre été étudiée en effectuant une simulation de Monte Carlo. La capacité du modèle à prévoir avec précision le dépassement de seuils hydrométriques fixés a enfin été analysée.
1 INTRODUCTION
The steady increase in damage due to flooding during the last decades has highlighted the need to develop effective measures focused on reducing the adverse effects of flood events. To this end, structural measures can be used, such as river banks, flood walls, dams and flood storage areas. However, these types of measures cannot eliminate the hydraulic risk and, hence, non-structural measures, typically consisting of a Flood Monitoring and Warning System (FMWS) to be actuated in real time, have also to be implemented. One of the fundamental components of a FMWS is a forecast model able to provide future estimates of the main hydrological quantities by using as input data the measurements of the online hydro-meteorological network. Traditionally, flood forecasting has been approached using rainfall–runoff or flood routing models. The former allow the forecast at the basin outlet starting from rainfall data and the forecast lead time is generally identified by the time of concentration of the basin, and the latter provide forecasts at the downstream end of a selected river reach with a forecast lead time limited by the flood wave travel time; nevertheless, they are more appealing for the limited data required and parameters involved.
For river reaches with negligible intermediate drainage area, flood routing models without incorporating lateral flow can be used when the flood dynamics are verified through selection criteria based on the river hydraulic and geometric characteristics, as well as the inflow shape (Ponce et al. Citation1978, Daluz Vieira Citation1983, Lamberti and Pilati Citation1996, Moussa and Bocquillon Citation1996, Moramarco et al. Citation2008, Perumal et al. Citation2011). It is worth noting that the available hydraulic packages, such as the Hydraulic Engineering Centers River Analysis System (HEC-RAS) of the US Army Corps of Engineers (USACE Citation2002) and MIKE11 (Danish Hydraulic Institute; DHI Citation2003), solve the full Saint Venant equations representing the most complete approach to describe flow regimes in natural channels. However, the use of these models requires detailed topographical surveys of river cross-sections and roughness at close intervals. When these data are not available, lumped hydrologic flood routing models may be usefully employed since they require sparse spatial data.
For long river channels, a model which is capable of quantifying the lateral flow has to be considered. In this context, Price (Citation2009) developed a simple conceptual flow routing model using a nonlinear advection–diffusion equation whose parameters are derived for an equivalent averaged cross-section of the modelled reach. The ungauged lateral flows are generated by a unit hydrograph rainfall–runoff model, with a design unit hydrograph known a priori whose peak value is adjusted to conserve the volume at the outlet. The model involves a large number of parameters and uses a rather empirical representation of lateral flow. Recently, Lerat et al. (Citation2012) thoroughly investigated the connections between hydraulic and hydrological models, identifying clear guidelines for services that routinely develop and also use such models within flood forecasting systems.
In order to avoid the use of rainfall data, an artificial neural network approach can be adopted, such as that proposed by Tayfur et al. (Citation2007), for the discharge forecast along the Tiber River. It is well-known, however, that the dataset selected for the training has a fundamental role to enable satisfactory results to be achieved.
Franchini and Lamberti (Citation1994) presented a simple Muskingum type model to forecast stages at the downstream end of a selected river reach by assuming the lateral flow proportional to the upstream discharge (O’Donnell Citation1985). To reduce the large number of parameters, Moramarco et al. (Citation2006) incorporated a parameter quantifying the lateral flow in real time. Their model, named STAFOM (STAge FOrecasting Model), has been recently enhanced by coupling it to the rating curve model (RCM) proposed by Moramarco et al. (Citation2005) which was developed for a simple estimation of rating curves at ungauged river sites (Barbetta et al. Citation2011a). The RCM involves only two parameters and was derived starting from entropy theory which allowed us to surmise a direct proportionality between the velocity at the downstream end and that observed at the upstream site, but at a time before and equal to the wave travel time of the event (Moramarco and Singh Citation2001).
In this context, Tayfur and Moramarco (Citation2008) investigated the use of a genetic algorithm (GA) to assess the optimal RCM parameter values for three Tiber River reaches. Thanks to the RCM capabilities, the modified forecast model, named STAFOM-RCM, provides accurate stage forecasts for several flood events that occurred along different river reaches of the Upper–Middle Tiber River basin, central Italy (Barbetta et al. Citation2011a). Moreover, the uncertainty of STAFOM-RCM was addressed by estimating the confidence intervals (CIs) related to model errors between observed and forecast stages (Barbetta et al. Citation2010, Citation2011b), even after a Box-Cox transformation (Box and Cox Citation1964) such as proposed by Misirli et al. (Citation2003). Recently, Barbetta et al. (Citation2012a) proposed a different approach consisting of a statistically based procedure allowing the confidence band to be attained by analysing the statistical properties of model output in terms of lateral flows.
Pursuing the concept of a parsimonious model structure for flood forecasting, this work proposes a modified formulation of the original RCM (Moramarco et al. Citation2005, Barbetta et al. Citation2012b) for real-time application, referred to henceforth as RCM-RT. This new model aims to forecast discharge at a river site of interest where the stage is monitored and the flow is known at an upstream section, even at significant distances along the channel. Two parameters, that is, the RCM parameters, are involved and are defined a priori through different discharge hydrographs recorded at both ends of the river reach. For RCM-RT application, the forecast lead time is assumed equal to the observed average wave travel time, while accurate topographical surveys of both the upstream and downstream reach sections are assumed to be available.
The quality of the forecast model is also evaluated using standard forecast verification metrics to assess whether it could be a useful supporting tool for real-time management (Jolliffe and Stephenson Citation2003, Wilks Citation2006). In addition, the “deterministic” forecast provided by the model cannot be considered perfect due to many types of errors affecting the forecast process (Todini Citation2004). Therefore, the assessment of the forecast uncertainty has to be addressed (Pappenberger et al. Citation2005). To this end, this work presents a preliminary investigation in order to assess the forecast uncertainty by performing a Monte Carlo simulation to quantify the CI that represents the uncertainty coming from the model parameters.
Finally, since flood warnings are usually based on a set of critical thresholds, the model performance is evaluated with the contingency table approach to show how well the RCM-RT forecasts the thresholds exceedence (Jolliffe and Stephenson Citation2003, Wilks Citation2006).
The paper is organized as follows: in Section 2, the theoretical background of the original RCM and the mathematical basis of the modified formulation for real-time application (RCM-RT) are described, along with the main steps of the adopted procedure for CI estimation. In Section 3, the statistics used to quantify the goodness of the forecasts and of the identified CIs are described, while Section 4 is dedicated to the description of the selected case studies, that is, a long reach of the Po River (northern Italy) and two reaches of the Tiber River (central Italy). The results obtained are then analysed in terms of both forecast and CIs, and Section 6 considers the obtained results, and the scientific and operational challenges are given.
2 THEORETICAL BACKGROUND
2.1 RCM
The RCM is a simple approach for discharge assessment at local sites where only the stage is monitored, while the streamflow is known at another river section located some distance away and is referred to as remote discharge.
Therefore, the RCM allows the rating curve to be estimated at hydrometric sections where flow velocity measurements cannot be carried out, or are only available for low stages (Barbetta et al. Citation2012b). The RCM was originally developed for downstream discharge estimation when the flow hydrograph is recorded at an upstream site (Moramarco and Singh Citation2001), also taking into account significant lateral contributions (Moramarco et al. Citation2005), and is formulated as follows:
2.1.1 Parameter estimation
To estimate the RCM parameters, α and β, equation (1) is expressed for the baseflow and the peak flow hydraulic conditions (Moramarco et al. Citation2005):
2.1.1.1 Downstream baseflow
The downstream baseflow, Qd(tb + TL), can be easily estimated if the gauged section, although not equipped for velocity measurements during high-flow events, allows velocity measurements to be carried out for low-flow events. Otherwise, assuming that during the baseflow condition the mean velocity does not change from upstream to downstream, Qd(tb + TL) can be estimated as the product of the upstream mean velocity, estimated by Qu(tb), and the downstream flow area Ad(tb + TL). This assumption might produce significant errors in the baseflow estimate if lateral flow is significant; however, it has a negligible effect on the downstream discharge hydrograph reproduction mainly for the peak region (Moramarco et al. Citation2005).
2.1.1.2 Downstream peak discharge
The downstream peak discharge, Qd(tp + TL), can be derived through the following equation (Moramarco et al. Citation2005):
It can be inferred from equation (1) that, by comparing the term [Ad(t + TL)/Au(t)]Qu(t) against the downstream observed discharge, Qd, for different observed floods, each one with its own wave travel time, a linear relationship of the river reach can be assessed. The parameters α and β represent the slope and intercept, respectively (Moramarco et al. Citation2005, Barbetta et al. Citation2011a). Thus, α and β are inherent characteristics of the investigated channel reach and provide significant information on wave routing. In particular, each flood occurring along the branch will follow the identified linear relationship if the actual value of the wave travel time, TL, is considered.
Recently, the original RCM was proven to take account of unsteady flow and, in addition, it was extended for upstream discharge estimation by assuming the flow known at a downstream site and stages measured at both ends (Barbetta et al. Citation2012b). In this new configuration, the model can be applied to river reaches with negligible lateral flow, or to river reaches with significant lateral contribution but where a kinematic flow regime holds. We refer the reader to Barbetta et al. (Citation2012b) for more details.
2.2 RCM for real-time application (RCM-RT)
In order to apply the RCM for real-time discharge forecasting purposes, equation (1) has to be suitably modified. To identify the formulation of the RCM for the real-time forecasting (RCM-RT), the unknown term Ad(t + TL) in equation (1) has to be assessed at each time of forecast t = tf. This can be achieved by considering equation (4) for lateral flow representation that allows the estimation of Ad(tf + TL) as:
Using equation (6) in equation (1) and considering TL as the forecast lead time, henceforth referred to as ∆t* (h), the formulation for the RCM-RT can be derived:
The RCM linear relationship obtained for TL equal to the mean observed wave travel time is very similar to that estimated when considering for each flood its own wave travel time (Barbetta et al. Citation2011a).
When a reliable rating curve is available at the downstream site, the forecast stage, hd for, can be easily derived through RCM-RT:
It is worth noting that RCM-RT cannot take account of unsteady effects because the forecast stage is derived through the available rating curve that is typically a one-to-one relationship.
The model results are presented here and discussed in terms of both forecast discharge and stage. Moreover, by considering that the warning of flood threats is typically based on fixed hydrometric thresholds, the capability of RCM-RT to correctly forecast the hydrometric threshold exceedence in a timely way is also investigated.
2.3 Forecast uncertainty analysis and estimation of CIs
The RCM-RT model provides future estimates of both discharge and stage with a forecast horizon ∆t* assumed equal to the mean wave travel time observed for the selected river reach. When considering a single estimate of each parameter α and β, that is, the characteristic values αmeanT and βmeanT, the RCM-RT model produces a perfectly sharp (deterministic) forecast.
However, even though a deterministic forecast can reach a certain degree of accuracy in flow prediction, it is affected by many sources of error and uncertainty in the forecast process. Therefore, the forecast uncertainty has to be assessed and uncertainty estimates should be provided during the forecast. In order to define the forecast uncertainty in terms of CI, a Monte Carlo-based approach is used here to investigate the impact of the model parameters on the model outputs. It is well known that the Monte Carlo method can be used with almost any deterministic model and that it relies on the development of a probability distribution function for each parameter or boundary condition for the model that is uncertain (Scharffenberg and Kavvas Citation2011). Therefore, analysis of the statistical properties of parameters α and β is performed by considering a set of recorded flood events for each of which the parameters value is first computed through equation (2).
The procedure for estimating the CIs is as follows:
Identification of the α and β parameter value samples In order to identify a sample of both parameters, RCM, equation (1), is applied in simulation mode for each flood event of a selected dataset and equation (2) is considered to estimate (α, β) values.
Estimation of the probability distribution of α and β For each parameter (α and β), the values are ranked to identify the ascending order; the mean,
and
, and standard deviation, σα and σβ, as well as the probability density function, pdf, are defined. The pdf assessment is based on the well-known Chi-square (χ2) test.
Estimation of the forecast CI A large number of forecast model “realizations” is carried out by sampling N values for each parameter, that is, α and β, from the distribution functions identified at step (ii). It is worth noting that the two parameters are assumed independent for identifying N pairs of values (α, β) used to apply the forecast model and derive N forecasts. At each time t, the 5th forecast percentile and the 95th forecast percentile define the lower and upper limits of the 90%CI.
3 EVALUATION MEASURES
In order to investigate the RCM-RT model performance and the quality of the estimated CIs, the following performance criteria are considered.
3.1 RCM-RT performance
The accuracy with which the model reproduces the observed discharge hydrographs can be quantified using the Nash-Sutcliffe efficiency coefficient (NS; Nash and Sutcliffe Citation1970), recommended by the ASCE Task Committee (Citation1993). The model efficiency for discharge hydrograph reproduction, NS, is given by:
The accuracy in predicting the peak discharge is estimated by computing the percentage error in the peak hydrograph, eQp, yielding:
Moreover, the coefficient of persistence, PCris (Kitanidis and Bras Citation1980), which takes values of ≤1 (corresponding to perfect forecasting performance), is considered. This verification metric compares the forecast with a persistent forecast for the rising limb of the hydrograph to evaluate whether the forecast is any better than persistence potential flood events. PCris, is computed as:
It is worth noting that the quality of the discharge forecast depends only on the RCM-RT performance, whereas the stage forecast depends also on the rating curve quality, although the assumption is that the quality is mostly influenced by that of the RCM-RT model.
3.2 CI quality
For a useful CI, with 5th and 95th percentiles as the lower and the upper bounds, respectively, 90% of the observations (associated with the forecast values) should fall within it. Therefore, the quality of the identified CI is tested by verifying the percentage of included observed discharge and stage values, referred to henceforth as QCI and hCI, respectively.
3.3 Quality of critical threshold exceedence forecast
The contingency table metric (Wilks Citation2006) is used to investigate how correctly the model forecasts the exceedence or non-exceedence of fixed hydrometric thresholds, on which the warning of flood threats is typically based. The contingency table is a useful way to see what types of errors are being made by the model (Jolliffe and Stephenson Citation2003, Wilks Citation2006). A perfect forecast model would produce only Hits (event forecast to occur, and did occur) and Correct negatives (event forecast not to occur, and did not occur), and no Misses (event forecast not to occur, but did occur) or False Alarms (event forecast to occur, but did not occur). Based on the contingency table, the following metrics can be computed:
Moreover, the average width of the CI, AW, as proposed by Zhang et al. (Citation2009), that can be considered as a description of the forecast sharpness, is computed as:
4 STUDY AREA AND DATASET
A long reach of the Po River, in northern Italy, and two river reaches located along the Tiber River, in central Italy, were selected for the case study. The main properties of the investigated case studies are summarized in .
Table 1 Main geomorphological properties of the selected river reaches. DAu and DAd: drainage area subtended by the upstream and downstream section, respectively; DAint: intermediate drainage area; L: channel length; S0: mean channel slope.
The reach on the Po River is bounded by the gauged sections of Piacenza and Pontelagoscuro (see ), for which accurate rating curves and topographic surveys are available and regularly updated, and is referred to herein as the Pia-PLag reach. As can be seen from , this is a long river branch characterized by a non-negligible intermediate drainage area that represents almost 40% of the basin subtended by the downstream end.
The two reaches selected on the Tiber River are bounded by the gauged sections of Pierantonio, Ponte Nuovo and Monte Molino (see ), where reliable rating curves and topographic surveys are also available. As can be inferred from , the Pierantonio-Monte Molino (Pie-MM) reach is quite a long river branch characterized by a significant intermediate drainage area that represents about 70% of the basin subtended by the Monte Molino site. For the Ponte Nuovo-Monte Molino (PN-MM) reach, the effect of lateral flow on the flood wave formation might be less significant considering that the intermediate area is only about 20% of the total basin area.
To investigate the quality of the RCM-RT model and the forecast CIs, 35 and 32 recorded flood events were selected for the Po River and Tiber River, respectively. Details of the investigated events, in terms of peak discharges and wave travel time for the investigated river reaches on the Po and Tiber, are summarized in and , respectively. It has to be underlined that some of the flood events are characterized by high stage and extensive flooding.
Table 2 Po River—Piacenza-Pontelagoscuro reach: main characteristics of the investigated floods (Qp: peak discharge; TL: observed wave travel time). The percentage of observed stages within the confidence interval (hCI) is also shown, along with the AW of the 90%CI for all the selected events.
Table 3 Tiber River reaches: main characteristics of the investigated floods (Qp: peak discharge; TL: observed wave travel time). The percentage of observed stages within the confidence interval (hCI) is also shown, along with the AW of the 90%CI for all the selected events.
5 RESULTS AND DISCUSSION
5.1 RCM-RT accuracy
5.1.1 Po River
In order to test the performance of the forecast model, the recorded flood events listed in were divided into a calibration dataset and a validation dataset. In particular, six flood events that occurred in the period May–December 2002 were considered for parameter calibration, that is, to assess the characteristic values αmeanT and βmeanT, and the mean wave travel time identifying the forecast lead time (TL = ∆t*). The calibration dataset was selected by giving priority to the magnitude of floods that occurred in 2002.
For the Pia-PLag reach, a mean wave travel time of 32 h is observed for the calibration dataset. The identified characteristic values of the model parameters, to be used for real-time forecast application, are given in and and . It is worth noting that the loops in are due to the own wave travel time of each flood, which is different from that used as forecast lead time, that is, 32 h. Indeed, if the actual wave travel time was considered, the scattering would decrease and the dots of all events would tend to lie on a straight line in accordance with equation (1) (see ).
Table 4 RCM-RT parameters for all the investigated reaches, wherein αmeanT and βmeanT are the RCM characteristic parameters and ∆t* is the forecast lead time. See for abbreviations.
Fig. 3 Linear relationship between the downstream discharge, Qd, and the quantity (Ad(t + TL)/Au(t))Qu(t) for the Piacenza-Pontelagoscuro reach (six calibration events): (a) mean (TL = 32 h) and (b) observed wave travel time; the Pierantonio-Monte Molino reach (14 calibration events): (c) mean (TL = 9 h) and (d) observed wave travel time; and the Ponte Nuovo-Monte Molino reach (14 calibration events): (e) mean (TL = 4 h); and (f) observed wave travel time.
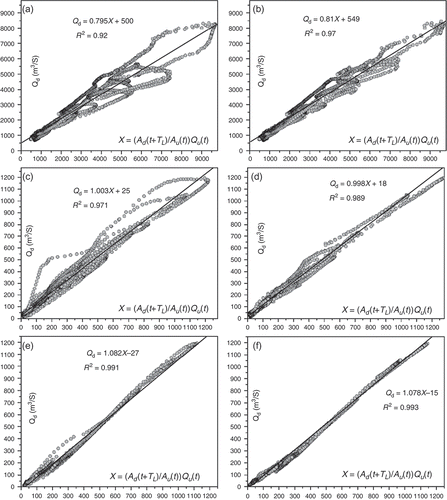
The results provided by the RCM-RT model, in terms of both discharge and stage for the six calibration flood events and for the validation dataset (29 flood events recorded in the period 2003–2011), are summarized in . The interval and the mean, in absolute values, of the selected accuracy measures reported in show that the model applied to the Pia-PLag reach is able to accurately forecast the peak region. In particular, mean absolute errors on peak discharge of 14% and 12% were found for the calibration and validation events, respectively, while the respective values of mean error in peak stage were 0.55 and 0.46 m. Inspecting , one can infer good performance of the RCM-RT model in terms of Nash-Sutcliffe efficiency (NS), both for calibration (means of 0.75 and 0.82 for discharge and stage hydrograph, respectively), and validation (means of 0.74 and 0.76, respectively). The coefficient of persistence for the rising limb in calibration has mean values of 0.61 and 0.74 for discharge and stage hydrograph, respectively, while it slightly decreases in validation with mean values of 0.62 and 0.66, respectively.
Table 5 Percentage lateral flow for all the investigated case studies. The variation range and mean, m (both in absolute value) of the evaluation measures of the RCM-RT performance are also shown (eQp: error in peak discharge; ehp: error in peak stage; PCris: coefficient of persistence for rising limb; NS: Nash-Sutcliffe efficiency coefficient).
The accuracy of the forecast is also verified in terms of peak timing quality. In particular, the results show that, for the validation dataset, the model provides anticipated peak values for most of the flood events, with a lead time of 10 h, on average. Such a lead time on peak flows is critical to support flood risk management activities in real time.
5.1.2 Tiber River
Following Barbetta et al. (Citation2012a), a calibration dataset of 14 flood waves was selected for both the river reaches, while 18 events were considered for validation purposes. All events are detailed in .
From analysis of the calibration dataset, forecast lead times of 9 and 4 h were identified for the Pie-MM and PN-MM reaches, respectively. Moreover, the characteristic values of the RCM parameters, αmeanT and βmeanT, were derived (see and –). It is worth noting that, as expected, the value obtained for α is almost the same for both the river reaches, while a slight variation is observed for β. These variations are most likely due to different geometric characteristics (length, mean bed slope and mean section width) of the investigated branches of the Tiber River. Previous studies on the Tiber River corroborate these results. In particular, Tayfur and Moramarco (Citation2008), applying the GA to estimation of RCM parameters for the Tiber River, found mean values of α and β very close to those assessed in this work.
shows that, for the Pie-MM reach, the model provides accurate forecasts of the peak flood region. In particular, mean errors in peak discharge of 9% and 11% and in peak stage of 0.31 and 0.38 m were observed for the calibration and validation datasets, respectively.
Similarly, the error in peak flood is very low for the shorter river reach (PN-MM) in terms of both stage and discharge, with a mean value of ehp and eQp less than 0.2 m and 6%, respectively, for both the calibration and validation datasets. Moreover, in terms of error in the time to peak, the model forecasts the peak region for both reaches, on average, 4 h in advance.
Finally, for the two investigated reaches, the values of PCris and NS for the calibration flood events are found to be good, while they are slightly less satisfactory for the validation dataset for both the stage and the discharge forecast hydrographs.
As shown in , for the calibration dataset, NS is, on average, 0.80 and 0.91 for Pie-MM and PN-MM, respectively, while, for the validation dataset, NS is slightly lower and equal to 0.70 and 0.88 for Pie-MM and PN-MM, respectively. Similarly, the PCris value in calibration is 0.85 for Pie-MM and 0.78 for PN-MM. In validation, the PCris value is lower and equal to 0.67 and 0.63 for Pie-MM and PN-MM, respectively. This mainly depends on the fact that some validation events are characterized by a downstream rising limb starting before, or at the same time as, the upstream one, showing a temporal and spatial distribution of the lateral contribution that is not coherent with the hypothesis of uniform lateral flow in the RCM-RT model. This condition is ascribed to the presence of a tributary just upstream of the downstream end, that is, the Monte Molino section that, for some events, causes a downstream rising limb to be anticipated with respect to that observed at the upstream end. However, it must be stated that this occurs for floods of insignificant magnitude, considering that high floods at the downstream end are triggered by the contribution of the whole intermediate basin. Indeed, for the Monte Molino section, flood events with a significant flood volume (>100 hm3) and peak discharge greater than 700 m3 s-1 (see ) have average values of PCris and NS for the validation dataset of 0.76 and 0.78, respectively.
5.2 Quality of the CI
In order to assess the forecast uncertainty, the CI, to be provided along with the future estimates of RCM-RT, is identified by following the procedure described in Section 2.3 and based on Monte Carlo simulation. For this purpose, all events were considered for the investigated river reaches and the CI quality is discussed for both the calibration and the validation flood events.
5.2.1 Po River
The samples of α and β parameter values were derived by considering all the selected 35 flood events listed in . Thus, a statistically significant sample size was obtained, allowing estimation of the probability distribution for the two model parameters. In particular, a log-normal and a Gaussian distribution were identified for α and β, respectively, with mean ( and
) and standard deviation (σα and σβ) reported in .
Table 6 Statistical analysis of the RCM parameters α and β. SD: standard deviation.
Considering the above identified probability density functions, 1000 (α, β) pairs (realizations) were randomly selected and used to run RCM-RT considering a forecast lead time of 32 h. Therefore, at each time t, 1000 different forecast discharge and stage values are provided and used for the 90% confidence interval (90%CI) estimate by assessing the 5th and 95th percentiles.
The quality of the CI was tested by computing the values of QCI and hCI. It is worth noting that hCI is mostly the same as QCI considering that stages are forecast using equation (9). As can be seen from , on average, CI is verified for both the calibration and validation events, with mean hCI of 95% and 93%, respectively. Considering each investigated flood event separately, the results obtained show that 74% of all events are characterized by hCI values greater than 90%. Similar results are found when the CI of the forecast discharges is considered, that is, in terms of QCI.
As an example, shows the comparison between the observed and the forecast stage and discharge hydrographs, along with the estimated CI for the events of December 2002 and 26 September 2006. The first event is the highest recorded flood during the available data period and 100% of the observed hydrograph for this event is within 90%CI (see and ). The percentage of included observed points is only 92% for the second event, for which the model provides an overestimated forecast peak flood wave. However, as shown in and , the CI includes the observed peak region almost completely.
Fig. 4 Piacenza-Pontelagoscuro reach (Po River, ∆t* = 32 h): comparison between observed and forecast hydrograph provided by the RCM-RT model for: (a) and (b) the calibration flood event of December 2002; (c) and (d) the validation flood event of 26 September 2006. The assessed CI is also shown along with the alarm threshold.
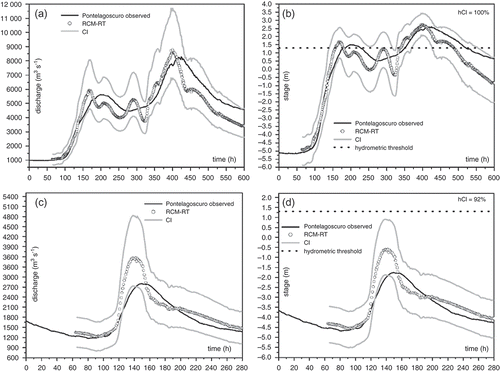
5.2.2 Tiber River
As for the Po River case study, the samples of α and β parameter values were obtained by considering all the selected 32 flood events listed in for both the investigated reaches. Statistical analysis of the parameter samples allows a log-normal distribution to be identified for α and a Gaussian distribution for β. The mean, standard deviation and χ2 values are listed in . Based on the identified probability density functions, 1000 (α, β) pairs were randomly selected and considered for generating 1000 different forecasts at each time t, with a forecast lead time of 9 and 4 h for Pie-MM and PN-MM, respectively. The quality of the identified CI was tested through the value of QCI and hCI, that is, the percentage of observed discharges and stages within the CI. summarizes the results for the forecast stages alone, considering that QCI is mostly the same.
Therefore, the estimated CI is, on average, verified for the calibration dataset, with mean values of hCI of 95% and 94% for the longer and the shorter reaches, respectively. Considering the validation flood events, the assessed CI is also verified, with mean values of 91% and 94% for Pie-MM and PN-MM, respectively.
Thus, the results obtained show that 72% of the events are characterized by hCI values >90% for both reaches. Similar outcomes were found on analysing the CI for discharge.
By way of example, comparisons between the observed and forecast discharge and stage hydrographs, along with the estimated CI, are shown in and for the Pie-MM and PN-MM reaches, respectively. The event shown in and is the calibration event recorded in February 2009, while the very significant flood observed in January 2010 is the validation event shown in and . As can be seen, for the calibration event, the peak region is accurately forecasted in terms of both magnitude and timing, and, correctly, 100% of the observed hydrograph within 90%CI does not reach the warning threshold. For the validation flood, one of the most severe in the available dataset, the forecast peak hydrograph depicts the observed event quite well, with an error of 2%. However, the model forecasts the peak some hours in advance, providing double peaks in the predicted hydrograph that can be ascribed to the double peak observed in the upstream flood hydrograph recorded at Pierantonio station. Nevertheless, the percentage of included observed points is 91% and only a short period of the flood peak region is outside the interval, as well as a part of the rising limb that, however, is very close to the upper limit of CI.
Fig. 5 Pierantonio-Monte Molino reach (Tiber River, ∆t* = 9 h): comparison between observed and forecast hydrograph provided by the RCM-RT model for: (a) and (b) the calibration flood event of February 2009; (c) and (d) the validation flood event of January 2010. The assessed CI is also shown along with the warning and alarm hydrometric thresholds.
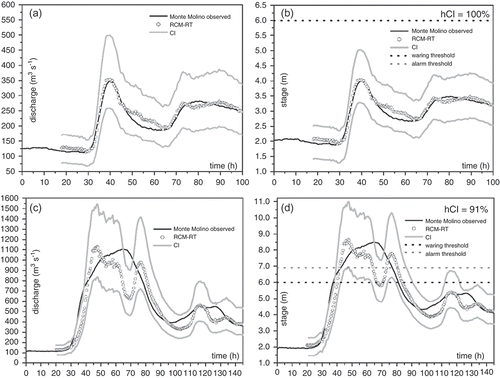
Fig. 6 Ponte Nuovo-Monte Molino reach (Tiber River, ∆t* = 4 h): comparison between observed and forecast hydrograph provided by the RCM-RT model for: (a) and (b) the calibration flood event of 30 December 2005; (c) and (d) the validation flood event of 2 December 2005. The assessed CI is also shown along with the warning and alarm hydrometric thresholds.
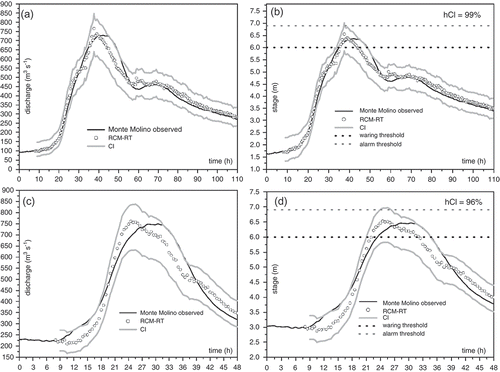
Referring to the PN-MM reach, shows the events of 30 December 2005 (calibration) and 2 December 2005 (validation). For the calibration event, 99% of the observed hydrograph is within 90%CI ( and ), while the percentage of included observed points decreases to 96% for the validation event, for which the model forecasts the peak discharge in advance ( and ).
5.3 Quality of critical threshold exceedence forecast
Analysis of the model capability to correctly forecast the exceedence or non-exceedence of available hydrometric thresholds was also carried out, and the results are shown in the contingency table (). Moreover, the AW of the CI was computed and is shown in for the forecast stage CI.
Table 7 Contingency table showing the capability of the RCM-RT model to predict exceedence/non-exceedence of hydrometric thresholds. halarm: alarm threshold; hwar: warning threshold.
5.3.1 Po River
For the Pontelagoscuro site, the alarm threshold, halarm, adopted by the Hydro-meteorological Service is 1.3 m above the hydrometric zero. This is placed 11.70 m above the lowest point of the river bottom (13 m of water depth).
As can be seen in , for the 35 investigated floods, halarm was exceeded during seven events in total, which was correctly predicted by RCM-RT (Hits = 7), although the model also provided three false alarms. While halarm was exceeded by RCM-RT for these three events, the CI includes halarm, showing that the lower limit of CI is below the alarm threshold anyway.
The accuracy metric (0.91) indicates that 91% of all forecasts were correct. Considering the BIAS score of 1.43, the RCM-RT model slightly over forecast the extreme events.
Moreover, it is worth noting that the average width of the CI for forecasting stage is nearly 2 m and always less than 2.5 m (see ). Considering that the water depth at the halarm level is 13 m, it can be inferred that the forecast sharpness is meaningful and informative for supporting decision-makers.
Therefore, the results are satisfactory, considering that, for 32 of the 35 investigated events, the model provided accurate forecasts of threshold exceedence, that is, 7 Hits and 25 Correct non-events, as shown in .
Referring to the severe flood events of December 2002 shown in , the exceedence of the threshold, although slightly anticipated, was correctly forecasted by the model. In contrast, even with a larger and earlier peak in the forecast, the alarm threshold was not reached during the flood reported in , as correctly forecasted by the RCM-RT model. In addition, it is worth noting that the warning level is above the upper limit of the CI.
5.3.2 Tiber River
For the Tiber River case studies, the analysis was carried out considering the warning threshold (hwar) and alarm threshold (halarm) values above the hydrometric zero, which is 1.1 m above the river bottom; available values for the Monte Molino gauged section were 6.0 and 6.9 m, respectively.
It can be inferred from that hwar was exceeded for 13 of the 32 investigated floods, while halarm was reached for seven flood events. When the RCM-RT model was applied to the Pie-MM reach, hwar exceedence was correctly forecasted for 10 flood events, while three events were missed. In contrast, for the PN-MM reach, all 13 hwar exceedences were correctly forecasted. The halarm exceedence was accurately predicted for six and seven flood events for Pie-MM and PN-MM, respectively. The RCM-RT model provided one false alarm and one missing alarm for the longer river reach, and two false alarms and no misses for PN-MM. However, it is worth noting that, when the three false alarms and missing alarm occur, the alarm threshold is always within the assessed CI.
Moreover, the AW of the stage confidence interval was, on average, lower than 1.7 m and 1.0 m for Pie-MM and PN-MM, respectively (see ). Considering that the water depth at the alarm threshold at Monte Molino site is 8 m, it can be inferred that the forecast sharpness is meaningful and informative for supporting the actions of decision-makers in real time.
For both the investigated river reaches, the rate of correctly predicted events is found to be very satisfactory. In particular, for the warning threshold, the model provided accurate forecasts (Hits + Correct non-events) for 28 and 32 flood events, for Pie-MM (accuracy = 0.88) and PN-MM (accuracy = 1), respectively. For the alarm threshold, the model provided a correct forecast for 30 floods for both the selected Tiber River branches (accuracy = 0.94). This also explains the good performance in terms of BIAS (see ) of 1 (perfect score) for Pie-MM and PN-MM when considering halarm and hwar, respectively.
6 SUMMARY AND CONCLUSIONS
The ability of the RCM-RT model to relate local stage and remote discharge along natural channels can be conveniently adopted for forecast purposes.
For real-time application, the model requires: stage and discharge values at an upstream site; stages at the site of interest; characteristic values of two parameters defined a priori through different discharge hydrographs recorded at both ends of the river reach; the mean wave travel time of the reach; and topographical surveys of the upstream and downstream sections of the reach.
The accuracy of the model was tested for a long reach on the Po River, northern Italy, and two reaches of the Tiber River, central Italy, characterized by different intermediate drainage areas and mean wave travel times.
The obtained results on the flood peak and hydrograph show that the RCM-RT model provides accurate flood forecasts with low errors on peak prediction and satisfactory agreement between forecast and observed hydrographs.
To better support risk-based decision-making, a Monte Carlo simulation led to estimation of the 90% CI associated with the perfectly sharp (deterministic) forecast provided by the RCM-RT model to quantify the uncertainty coming from the model parameters. The results show that the 90%CI is, on average, verified for all the investigated river reaches, with a percentage of included observed points between 91% and 95%. This finding represents a good starting point to apply and verify RCM-RT in other river reaches with different geometric and hydraulic characteristics. However, comparison of the different methodologies for assessing the CI of the deterministic forecasts provided by the model is definitely required. It is worth noting that quantification of the forecast uncertainty, by CIs, could be of considerable interest for supporting real-time decisions on flood-risk management, especially in terms of the information provided on the probability of warning-threshold exceedence.
The model simplicity, along with the fact that it does not need rainfall data as input, make it very appealing for implementation as an element of the forecast modelling system that is a fundamental component of FMWS to support Civil Protection activities.
The model is accurate when the forecast lead time is assumed equal to the mean wave travel time of the river reach and, hence, allows forecast horizons that are typically shorter than those provided in the application of rainfall–runoff models.
Finally, there is a need to improve as much as possible the understanding among modellers, forecasters and end users (Pappenberger et al. Citation2013) of a risk-based decision process with forecast that includes uncertainty. Moreover, the communication of the forecast uncertainty has to be enhanced, including additional forecast uncertainty descriptions that could better meet the needs of end users. These challenges are of particular interest, because a probabilistic forecast system may provide support by producing information to decision-makers, but it does not provide a decision.
Acknowledgements
The authors wish to thank Umbria Region, Department of Environment, Planning and Infrastructure, for providing Tiber River basin data. They are also thankful to the Interregional Agency for the Po River (AIPo) for providing the Po River data. Finally, the two Reviewers and the Associate Editor are gratefully acknowledged for their very useful comments.
REFERENCES
- ASCE Task Committee on Definition of Criteria for Evaluation of Watershed Models of the Watershed Management Committee, Irrigation and Drainage Division, 1993. Criteria for evaluation of watershed models. Journal of Irrigation and Drainage Engineering, 119 (3), 429–442. doi:10.1061/(ASCE)0733-9437(1993)119:3(429).
- Barbetta, S., et al., 2010. Uncertainty assessment for real-time stage forecasting. In: R.N. Palmer, ed. World environmental and water resources congress 2010—challenges of change. Providence, RI: American Society of Civil Engineers, 2313–2323.
- Barbetta, S., et al., 2011a. Case study: improving real-time stage forecasting Muskingum model by incorporating the rating curve model. Journal of Hydrologic Engineering, 16 (6), 540–557. doi:10.1061/(ASCE)HE.1943-5584.0000345.
- Barbetta, S., et al., 2011b. Addressing the uncertainty assessment for real-time stage forecasting. In: R.E. Beighley II and M.W. Killgore, eds. World environmental and water resources congress 2011—bearing knowledge for sustainability. Palm Springs, CA: American Society of Civil Engineers, 4791–4800.
- Barbetta, S., et al., 2012a. Confidence interval of real-time forecast stages provided by the STAFOM-RCM model: the case study of the Tiber River (Italy). Hydrological Processes. doi:10.1002/hyp.9613.
- Barbetta, S., et al., 2012b. Enhancement and comprehensive evaluation of the rating curve model for different river sites. Journal of Hydrology, 464–465, 376–387. doi:10.1016/j.jhydrol.2012.07.027.
- Box, G.E.P. and Cox, D., 1964. An analysis of transformations. Journal of the Royal Statistical Society, 26, 211–252.
- Daluz Vieira, J.H., 1983. Conditions governing the use of approximations for the Saint-Vénant equations for shallow surface water flow. Journal of Hydrology, 60 (1–4), 43–58. doi:10.1016/0022-1694(83)90013-6.
- DHI, 2003. User’s manual and technical references for MIKE 11 (version 2003b). Denmark: Danish Hydrologic Institute.
- Franchini, M. and Lamberti, P., 1994. A flood routing Muskingum type simulation and forecasting model based on level data alone. Water Resources Research, 30 (7), 2183–2196. doi:10.1029/94WR00536.
- Jolliffe, I.T. and Stephenson, D.B., 2003. Forecast verification: a practitioner’s guide in atmospheric science. Chichester: Wiley.
- Kitanidis, P.K. and Bras, R., 1980. Real-time forecasting with a conceptual hydrologic model: 2. applications and results. Water Resources Research, 16 (6), 1034–1044. doi:10.1029/WR016i006p01034.
- Lamberti, P. and Pilati, S., 1996. Flood propagation models for real time forecasting. Journal of Hydrology, 175, 239–265. doi:10.1016/S0022-1694(96)80013-8.
- Lerat, J., et al., 2012. Towards robust methods to couple lumped rainfall-runoff models and hydraulic models: a sensitivity analysis on the Illinois River. Journal of Hydrology, 418–419, 123–135. doi:10.1016/j.jhydrol.2009.09.019.
- Linsley, R.K., Kohler, M.A., and Paulhus, J.L.H., 1982. Hydrology for engineers. 3rd ed. New York: McGraw-Hill Book Company.
- Misirli, F., et al., 2003. Bayesian recursive estimation of 682 parameter and output uncertainty for watershed models. In: Q. Duan, et al., eds. Calibration of 683 watershed models. Water Science and 684 Application Series, 6. Washington, DC: AGU, 113–124.
- Moramarco, T., et al., 2005. Relating local stage and remote discharge with significant lateral inflow. Journal of Hydrologic Engineering, 10 (1), 58–69. doi:10.1061/(ASCE)1084-0699(2005)10:1(58).
- Moramarco, T. and Singh, V.P., 2001. Simple method for relating local stage and remote discharge. Journal of Hydrologic Engineering, 6 (1), 78–81. doi:10.1061/(ASCE)1084-0699(2001)6:1(78).
- Moramarco, T., et al., 2006. A real-time stage muskingum forecasting model for a site without rating curve. Hydrological Sciences Journal, 51 (1), 66–82. doi:10.1623/hysj.51.1.66.
- Moramarco, T., Pandolfo, C., and Singh, V.P., 2008. Accuracy of kinematic wave and diffusion wave approximations for flood routing. I: steady analysis. Journal of Hydrologic Engineering, 13 (11), 1078–1088. doi:10.1061/(ASCE)1084-0699(2008)13:11(1078).
- Moussa, R. and Bocquillon, C., 1996. Criteria for the choice of flood routing methods in natural channels. Journal of Hydrology, 186, 1–30. doi:10.1016/S0022-1694(96)03045-4.
- Nash, J.E. and Sutcliffe, J.V., 1970. River flow forecasting through conceptual models part I–a discussion of principles. Journal of Hydrology, 10 (3), 282–290. doi:10.1016/0022-1694(70)90255-6.
- O’Donnell, T., 1985. A direct three-parameter Muskingum procedure incorporating lateral inflow. Hydrological Sciences Journal, 30 (4), 479–496. doi:10.1080/02626668509491013.
- Pappenberger, F., et al., 2005. Cascading model uncertainty from medium range weather forecasts (10 days) through a rainfall–runoff model to flood inundation predictions within the european flood forecasting system (EFFS). Hydrology and Earth System Sciences, 9, 381–393. doi:10.5194/hess-9-381-2005.
- Pappenberger, F., et al., 2013. Visualizing probabilistic flood forecast information: expert preferences and perceptions of best practice in uncertainty communication. Hydrological Processes, 27, 132–146. doi:10.1002/hyp.9253.
- Perumal, M., et al., 2011. Real-time flood stage forecasting by Variable Parameter Muskingum stage hydrograph routing method. Hydrology Research, 42 (2–3), 150–161. doi:10.2166/nh.2011.063.
- Ponce, V.M., Li, R.M., and Simons, D.B., 1978. Applicability of kinematic and diffusion models. Journal of the Hydraulics Division, 104 (3), 353–360. ASCE.
- Price, R.K., 1973. Flood routing methods for British rivers. ICE Proceedings, 55 (4), 913–930. doi:10.1680/iicep.1973.4147.
- Price, R.K., 2009. An optimized routing model for flood forecasting. Water Resources Research, 45, W02426. doi:10.1029/2008WR007103.
- Raudkivi, A.J., 1979. Hydrology: an advanced introduction to hydrological processes and modeling. New York: Pergamon.
- Scharffenberg, W.A. and Kavvas, M.L., 2011. Uncertainty in flood wave routing in a lateral-inflow-dominated stream. Journal of Hydrologic Engineering, 16 (2), 165–175. doi:10.1061/(ASCE)HE.1943-5584.0000298.
- Tayfur, G. and Moramarco, T., 2008. Predicting hourly-based flow discharge hydrographs from level data using genetic algorithms. Journal of Hydrology, 352, 77–93. doi:10.1016/j.jhydrol.2007.12.029.
- Tayfur, G., Moramarco, T., and Singh, V.P., 2007. Predicting and forecasting flow discharge at sites receiving significant lateral inflow. Hydrological Processes, 21 (14), 1848–1859. doi:10.1002/hyp.6320.
- Todini, E., 2004. Role and treatment of uncertainty in real-time flood forecasting. Hydrological Processes, 18 (14), 2743–2746. doi:10.1002/hyp.5687.
- USACE, 2002. HEC-RAS river analysis system hydraulic reference manual (version 3.1). Davis, CA: USACE-HEC.
- Wilks, D.S., 2006. Statistical methods in atmospheric sciences. 2nd ed. San Diego, CA: Academic Press.
- Zhang, X., et al., 2009. Estimating uncertainty of streamflow simulation using Bayesian neural networks. Water Resources Research, 45, W02403. doi:10.1029/2008WR007030.