Abstract
River water temperature regimes are expected to change along with climate over the next decades. This work focuses on three important salmon rivers of eastern Canada, two of which warm up most summers to temperatures higher than the Atlantic salmon lethal limit (>28°C). Water temperature was monitored at 53 sites on the three basins during 2–18 summers, with about half of these sites either known or potential thermal refugia for salmon. Site-specific statistical models predicting water temperature, based on 10 different climate scenarios, were developed in order to assess how many of these sites will remain cool enough to serve as refugia in the future (2046–2065). The results indicate that, while 19 of the 23 identified refugia will persist, important increases in the occurrence and duration of temperature events in excess of 24°C and 28°C, respectively, in the mainstems of the rivers, will lead to higher demands for thermal refugia in the salmonid populations.
Editor Z.W. Kundzewicz; Associate editor T. Okruszko
Résumé
Les régimes thermiques des eaux fluviales devraient changer avec le climat au cours des prochaines décennies. Ce travail s’intéresse à trois rivières à saumon de l’Est canadien dont deux présentent des températures plus élevées que la limite létale du saumon atlantique (28°C) à plusieurs reprises la plupart des étés. La température de l’eau a été contrôlée sur 53 sites des trois bassins durant 2 à 18 étés. Environ la moitié de ces sites est connue comme refuges thermiques potentiels pour le saumon. Des modèles statistiques spécifiques de site prévoyant la température de l’eau ont été développés afin d’évaluer combien de ces sites resteront assez frais pour servir de refuge dans l’avenir (2046–2065), sur la base de dix scénarios climatiques différents. Les résultats indiquent que, bien que 19 des 23 refuges identifiés persisteront, l’augmentation importante de l’occurrence et de la durée de températures comprises entre 24 et 28°C dans les chenaux principaux conduiront à une demande plus importante de refuges thermiques pour les populations de salmonidés.
1 INTRODUCTION
Water temperature is highly correlated with atmospheric variables and with air temperature in particular. In northern regions, stream water temperature regimes share a particular seasonal pattern, with temperatures close to 0°C during the ice-covered season and a sinusoidal shaped pattern similar to the air temperature pattern during the free water season, with a maximum during summer (Caissie Citation2006). Eastern Canadian salmonids such as Atlantic salmon (Salmo salar) and brook trout (Salvelinus fontinalis) have adapted to such a thermal regime. Salmonids have specific survival temperature distribution ranges and their reproduction, feeding and growth require even more specific thermal conditions (Jonsson and Jonsson Citation2009, Elliott and Elliott Citation2010). For salmonids in particular, temperature is a critical habitat variable to maintain healthy populations.
High temperature has the double effect of lowering the dissolved oxygen concentration in water, while increasing the metabolic rate and thus the oxygen demand of fish. When oxygen consumption becomes less than oxygen demand, the fish must rely on anaerobic energy pathways.
Most recent studies tend to converge on a number of survival and feeding temperature thresholds for different salmonid species. summarizes the upper critical temperatures for survival and feeding for Atlantic salmon and brook trout (Jonsson and Jonsson Citation2009, Elliot and Elliot Citation2010, Breau et al. Citation2011). These two species are found in many eastern Canadian rivers, including the three rivers of the present study, and are popular species for recreational fishing. Temperature thresholds are defined as: (a) incipient lethal temperature, a temperature that can be tolerated by approx. 50% of fish during an extended period (usually 7 days); (b) upper feeding range, the range of temperatures at which fish may cease to feed; and (c) ultimate lethal temperature, a temperature that cannot be tolerated even for short periods (minutes). It should be noted that the indicated optimal temperatures for growth must be modulated by food availability and other habitat variables (Bacon et al. Citation2005).
Table 1 Upper critical temperatures ranges (°C) for survival and feeding of Atlantic salmon (Salmo salar) and brook trout (Salvelinus fontinalis). Adapted from Elliott and Elliott (Citation2010), Jonsson and Jonsson (Citation2009) and Breau et al. (Citation2011).
Thermally stressed individuals have been observed to move into cooler regions, or thermal refugia, to avoid potentially lethal thermal conditions (Cunjak et al. Citation2005, Breau et al. Citation2007). In a laboratory experiment, Breau et al. (Citation2011) found that the basal metabolic rate of 2+ Atlantic salmon reaches its maximum at 24°C, the same temperature at which maximum oxygen consumption, cessation of feeding and behavioural changes are observed.
This work focuses on three important salmon rivers of eastern Canada () where salmon populations currently are subjected to a gradient of thermal stresses in summer. One system, the Sainte Marguerite River (Quebec), is located further north, where salmon are more rarely affected by thermal stress, while the other two, the Ouelle River (in Quebec) and Little Southwest Miramichi River (in New Brunswick), warm up to temperatures higher than the Atlantic salmon lethal limit (>28°C) on many days during practically every summer. In recent years, high temperature events have led to episodic fishery closures at the Miramichi River by the Canadian Ministry of Fisheries and Oceans. The Little Southwest Miramichi River (LSWM), a tributary of the Miramichi, experiences 28 days per season above 23°C and temperatures as high as 30°C have been recorded on this river. Mortalities were reported in the Ouelle River in 2002 and 2010 (Ministère des ressources naturelles et de la Faune, pers. comm.), two years that experienced particularly severe summer low flows and temperature peaks as high as 32°C. While experiencing such extreme temperature events every year, the Ouelle and LSWM sustain important salmon populations and an important recreational fishery, which demonstrate that, in addition to local adaptation, these populations depend on the availability of discrete thermal refugia along the river courses.
River water temperature regimes are expected to change along with climate in the next few decades. Stenotherm instream species are going to be the more strongly and more rapidly affected by such changes. For instance, studies assessing the instream habitat of a variety of North American salmonid species conclude that the average summer water temperature will be higher, and that temperature thresholds defining thermally stressful conditions for salmonids (approx. 20–22°C, see ) will be exceeded more often and for longer periods in 2050, leading to habitat losses (Eaton and Scheller Citation1996, Chu et al. Citation2005, Monk and Curry Citation2009, Isaak et al. Citation2010, Mantua et al. Citation2010).
In order to understand and predict the evolution of river thermal regimes, water temperature models of two main types are generally used (Caissie Citation2006): deterministic models, which explicitly describe the physics of the heat exchange processes, and statistical/empirical models, which establish direct relationships between atmospheric and field conditions and the river temperature (Benyahya et al. Citation2007). Deterministic models have the advantage of making explicit the cause–effect relationships between the water temperature and its different drivers, and to explicitly separate the different contributions to the heat balance (Hebert et al. Citation2011, Leach and Moore Citation2011). Such models, however, usually require a large amount of geospatial and land-use information at the basin and reach scales, as well as quite localised meteorological information (Caissie et al. Citation2007, Hannah et al. Citation2008, Benyahya et al. Citation2010). As they take explicit account of the different processes influencing water temperature, deterministic models can be designed or adapted to evaluate the impact of the construction of hydraulic structures, such as a dam, or water withdrawals, such as an irrigation system, but their implementation in large-scale studies involving many different sites may become data expensive. However, statistical models can be calibrated from available historical water temperature and weather data acquired at a particular site, without explicit knowledge of the local physiography or land use. A statistical model can be used as a predictive tool for management and planning purposes at the specific site for which it was calibrated. Because they do not explicitly model processes, such empirical models assume that all conditions that are not weather-related, such as land use, remain constant. Such empirical models include linear and nonlinear regressions (Mohseni and Stefan Citation1999, Erickson and Stefan Citation2000), stochastic models (Caissie et al. Citation2001, Benyahya et al. Citation2008) and nonparametric models (Chenard and Caissie Citation2008, St-Hilaire et al. Citation2011).
The general objective of this paper is to estimate the severity of future riverine thermal conditions in mainstems and known cooler refugia for Atlantic salmon and brook trout near the southern limit of their current range, in Quebec and New Brunswick provinces, Canada. The specific objectives are to establish site-specific water temperature models based on summer weather conditions at many sites on three eastern Canadian salmon rivers, and to evaluate the evolution of the thermal conditions at these sites by 2046–2065 with regards to salmon and trout stress thresholds.
Water temperature has been monitored at 53 sites distributed on the three basins during 2–18 summers (June–September), with about half of these sites being known or potential thermal refugia (usually cold tributaries). Site-specific stochastic models predicting water temperature are developed using air temperature and precipitation data, in order to assess how many of these sites provided potential thermal refugia between 1970 and 1999 according to historical weather conditions, and how many will remain in the future (2046–2065), according to 10 different climate scenarios.
2 STUDY SITES AND WATER TEMPERATURE DATA
Details about each river location, drainage area, discharge, average summer air temperature and annual summer precipitation are listed in . The catchments of the three study drainage basins are shown in . All three study rivers have healthy populations of Atlantic salmon, with total juvenile (parr) densities locally reaching 30 individuals per 100 m2 based on electrofishing surveys.
Fig. 1 Locations of the meteorological stations and water temperature monitoring sites in each of the three drainage basins: (a) St Marguerite, (b) Ouelle, and (c) LSWM. M and R with site numbers respectively designate mainstem (M) and paired cooler refugia (R) tributary waters.
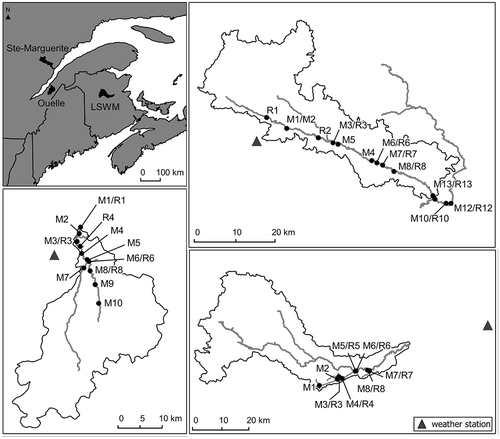
Table 2 Characteristics of the river basins.
The St Marguerite River is located on the Québec North Shore region, which, as its name describes, is located on the north shore of the St Lawrence River in the eastern part of the province. It flows into the Saguenay River, near its confluence with the St Lawrence River. Extensive research has been conducted on this river since 1995, as part of the Centre interuniversitaire de recherche sur le saumon atlantique (CIRSA, www.bio.ulaval.ca/cirsa/) research programme. Water temperature was monitored from June to September at 13–24 sites in 2005, 2007, 2009 and 2010 ().
Table 3 Water temperature monitoring sites and corresponding length of water temperature records. M# codes designate sites in the mainstem of the river and R# codes identify potential refugia. Refugium-mainstem pairs are identified by the same number.
The Ouelle River flows into the St Lawrence River about 100 km downstream of Québec City. Based on available thermal records, the Ouelle is probably one of the warmest salmon rivers in Québec. The Ouelle system is flashy with low baseflow contributions: summer flows are highly variable and low flows can be severe. Flows reached a historical minima in 2010. Fifteen water temperature monitoring sites were installed in the Ouelle and its tributaries between 2008 and 2010.
The Little Southwest Miramichi River (LSWM) is a tributary of the Miramichi River that flows north to east through New Brunswick into the northern portion of Northumberland Strait. The water temperature of the LSWM and its main tributary, Catamaran Brook, has been monitored since 1992 (Caissie et al. Citation2001, Citation2007). Twelve additional water monitoring sites were installed in 2009 and 2010.
Water temperature was measured using Onset Pendant temperature loggers, with a 0.10°C resolution and a ±0.47°C accuracy at 25°C. Each logger was inserted in a perforated white PVC tube that was attached to a concrete block and deposited on the river bed. Temperature was measured every 15 min from May–June to August–September (approx. 100 days each year).
Water temperature was measured at a total of 53 sites across these three watersheds. Most water temperature monitoring sites were chosen in pairs, with one temperature logger installed in a known or potential thermal refugium (usually a cold tributary), and one in the mainstem of the river, a few metres upstream of the refugium. Sites locations and the corresponding number of days with available water temperature data can be found in and .
Air temperature and precipitation time series were obtained from Environment Canada weather stations at Ste-Rose-du-Nord (48°24′N; 70°32′W), La Pocatière (47°21′21″N; 70°01′55″W) and Miramichi RCS (47°00′34″N; 65°27′54″W) for the St Marguerite, Ouelle and LSWM basins, respectively (). shows the 2010 summer air temperature, precipitation and water temperature for a mainstem-refugium pair located on the Ouelle.
3 METHODS
The water temperature models that were developed are site-specific: one empirical statistical model was calibrated for each water temperature monitoring site, given the available water temperature data collected at that site. Based on antecedent air temperature and precipitation information, each model predicts daily minimum, maximum and mean water temperature at the respective thermograph site. Subsequently, simulated air temperature and precipitation data obtained from 10 climate change scenarios are put into each calibrated model to obtain daily water temperature for the 1970–1999 reference period, and for the future 2046–2065 period. Statistics of the reference and future temperature regimes for each site are then compared in order to evaluate the evolution of the temperature regimes of the three rivers, as well as the persistence of the potential thermal refugia for salmon and trout in these systems.
3.1 Water temperature models
The models used for water temperature are an improved version of the models presented by Jeong et al. (Citation2013). The architecture, calibration and validation of the models are summarized here, and the reader is referred to Jeong et al. (Citation2013) for further details.
The water temperature models are multilayer perceptrons (MLPs) relating cumulative precipitation of the d1 previous days and average air temperature residuals of the current and d2 previous days (the predictors), to the daily minimum, mean and maximum water temperature residuals of that day (the predictands).
Residuals are obtained by subtracting the seasonal component (interannual mean for each day) to the daily value of the air/water temperature:
where TR(t) is the air/water temperature residual at day t, T(t) is the air/water temperature at day t, and TS(t) is the air/water temperature seasonal component value at day t. The air temperature seasonal component at each site is modelled by a sinusoidal function of the form (Cluis Citation1972):
where a, b and t0 are coefficients estimated by a nonlinear least square approach. An example of the air temperature seasonal component and residuals for the LSWM air temperature is shown in .
Fig. 3 Top: Inter-annual mean daily air temperature recorded at Miramichi RCS meteorological station between 1970 and 1999 (light grey line) and the modelled seasonal component (dark line). Bottom: air temperature residuals for 1995.
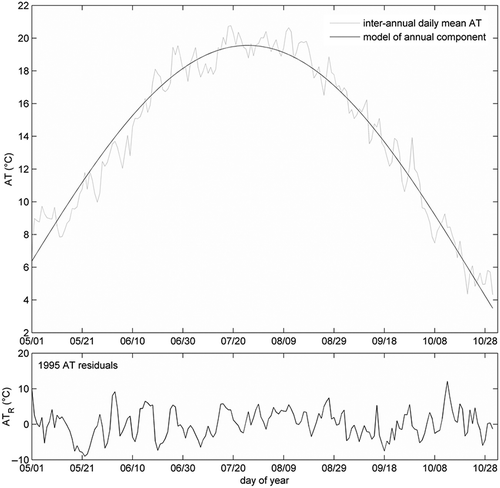
The numbers of previous d1 and d2 days for the computation of the predictors were chosen based on the best modelling performances obtained with different values of d1 and d2 (Jeong et al. Citation2013). These were found to be d1 = 10 and d2 = 1. The two predictors are thus defined as P10(t), the cumulative precipitations from the 10 previous days (days t – 10 to t), and ATR2(t), the average of the air temperature residuals of the current and the previous day (days t and t – 1).
Multilayer perceptrons (MLPs) are well-known feed-forward artificial neural networks that have been successfully used in water temperature modelling (Chenard and Caissie Citation2008, Daigle et al. Citation2010). The MLPs used in the present study have three layers: the input layer is two-dimensional as two predictors are used; the hidden layer is also two-dimensional; and the network has three outputs, corresponding to the three predictands: the minimum, the mean and the maximum daily water temperature residuals. Each of the three outputs is calculated with the following equation:
where is the predicted (min, mean or max) water temperature residual at day t; w1j, wj1, wj2, wj0 and w10 are adjustable parameters (also known as weights); and g1 and g2 are tangent sigmoid activation functions. The MLPs are trained using a Bayesian regularization backpropagation optimization algorithm. Such optimization consists of the minimization of an objective function combining the sum of squared errors and the sum of the squares of all adjustable parameter values in the neural network, in order to produce more stable outputs (MacKay Citation1992, Demuth and Beale Citation2000).
The performance of the water temperature models is evaluated given two performance measures, the root mean square error (RMSE) and the mean bias error (MBE):
In general, the predictions of statistical models calibrated based on error minimization will have a variance smaller than that of the observed data. Here, this means that , and thus that highest absolute residuals, and consequently water temperature extremes, will be underestimated. To overcome this limitation, a variance inflation procedure was adopted. Variance inflation consists of artificially increasing the variance of a time series, in order to better reproduce its natural variability. This is done by multiplying the predicted time series by a variance inflation factor:
where is the variance inflated water temperature residual at day t. Variance inflation helps to reproduce the variance of the observed data, but increases prediction RMSE slightly (<0.1°C; see Jeong et al. Citation2013).
The MLPs are calibrated using 60% of the available data, and the remaining 40% are used for validation. Calibration and validation sets are sampled randomly. This split-sample validation is repeated 25 times in order to test the stability of the prediction performance.
Since time series available at some sites are short, the outputs of the calibrated MLPs are combined using an ensemble approach. Ensemble approaches are used to produce more robust estimates by taking advantage of all available data, and are especially useful when the sample size is small. Here, 25 series of water temperature residuals are estimated by the 25 models calibrated in the split-sample procedure. The final estimated water temperature time series is produced by adding the water temperature seasonal component to the ensemble mean of the 25 estimated water temperature residual series.
3.2 Climate scenarios
To produce simulated water temperature time series for the reference (1970–1999) and projected future (2046–2065) periods, the calibrated water temperature models are input with air temperature and precipitation data from five different climate simulations that were provided by the Ouranos Consortium (Huard and Chaumont Citation2011). Five simulations were selected amongst more than 40, obtained from the World Climate Research Programme Coupled Model Inter-comparison Project phase 3 (Meehl et al. Citation2007) and the North American Regional Climate Change Assessment Program (www.narccap.ucar.edu/). The five simulations were selected so that most of the range of projected future changes in summer temperature and precipitation on the three study river basins was covered. The selected simulations involve two regional simulations from the Canadian Regional Climate Model (CRCM), two global models (CSIRO and MIROC) simulations using three SRES (Special Report on Emissions Scenarios) scenarios ().
Table 4 Climate models and SRES scenarios employed in the present study.
Climate model outputs generally present systematic differences with local historical meteorological conditions over a reference period. These biases may be due to model error, including misrepresentation of long-term natural variability and/or to the difference in scale between the model spatial resolution and the scale of the processes that affect the system under study. Climate model outputs are often bias-corrected (downscaled) when used for regional hydrological impact studies, as these involve factors and processes over drainage basin areas that operate at smaller scales than the spatial resolution of climate models (Wu Citation2010, Chun et al. Citation2013, Perazzoli et al. Citation2013). For the present study, the simulated air temperature and precipitation time series, provided by the Ouranos Consortium, were bias-corrected given daily scaling (DS) and daily translation (DT) approaches. These are two statistical methods that belong to the model output statistics family (Chiew et al. Citation2010, Themeßl et al. Citation2010). They are based on the computation of a mapping (the former on the mean monthly values, and the latter on different percentiles) between the historical and simulated climate variable distributions (on the same reference period), and the application of this mapping to the climate model raw outputs. Details about the present application can be found in Huard and Chaumont (Citation2011). Applying these two debiasing techniques to the outputs of the five climate model simulations results in 10 different climate scenarios for each of the reference and future periods. These 10 debiased simulated air temperature and precipitation time series will thereafter be referred to as climate scenarios.
3.3 Temporal evolution of the water temperature regimes
A water temperature model calibrated at one site with inputs from 10 future climate scenarios will provide 10 future water temperature scenarios for this site. The evolution of the water temperature regimes at every study site is made by comparing these future water temperature scenarios to the water temperature time series simulated using observed meteorological conditions in the 1970–1999 reference period. Comparisons are made for a number of water temperature metrics as listed in . These temperature metrics were chosen to:
evaluate the evolution of thermal regimes in eastern Canadian rivers between 1970–1999 and 2046–2065;
determine which sites identified as potential thermal refugia during the reference period are likely to remain sufficiently cold in the future period;
evaluate the recurrence and duration of stressful events at mainstem sites in the reference and future periods (metrics 7Dmax, %max24-28, Dmax24-28); and
evaluate the evolution of the cooler conditions, during which the fish may recover from more thermally stressful daytime hours (metric %min20).
The maximum, 7 day mean daily temperature (7Dmax) gives a measure of the maximum multi-day temperature periods encountered at a site, without over-emphasising events of short time duration (e.g. instantaneous values). The frequency, duration and timing of temperature events >24°C, and >28°C are quantified by the percentage of summer days exceeding these thresholds, the mean number of consecutive days meeting this criterion and the average date of first occurrence. The choice of 24°C and 28°C temperature thresholds was motivated by the Atlantic salmon and brook trout critical temperature ranges found in the literature (). The percentage of summer days with a minimum daily temperature exceeding 20°C was also computed in order to represent the cooler period during which the fish may recover from the warmer daytime hours.
Table 5 Temperature metrics used to compare reference (1970–1999) and future (2046–2065) simulated water temperature regimes. Each statistic is computed over the whole series.
4 RESULTS
4.1 Water temperature models
An example of water temperature simulation, for site M7 on the Ouelle, is shown on . Prediction performances (after variance inflation) of the ensemble water temperature models on the daily minimum, mean and maximum temperatures among sites are illustrated by histograms in . The validation performance values are the median of the 25 RMSE values achieved by 25 MLPs when tested on validation sets (see Section 3.1). The simulation performances are the RMSEs achieved by the ensemble of 25 MLPs. Most MBE absolute values are <0.1°C and all are <0.2°C (not shown).
Fig. 4 Observed and simulated summer daily minimum, mean and maximum water temperatures at site M7 on the Ouelle, for 2001 (top row), 2002, 2003, 2008, 2009 and 2010 (bottom row). RMSE values for predicted daily minimum, mean and maximum temperatures at this site are 1.4, 1.3 and 1.9°C, respectively.
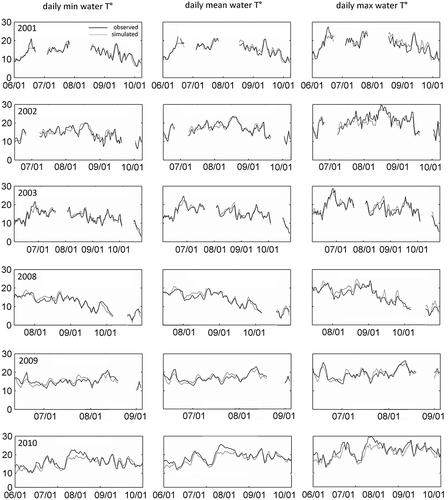
Fig. 5 Distributions of the RMSE validation and simulation values for predicted daily minimum, mean and maximum temperatures at all sites.
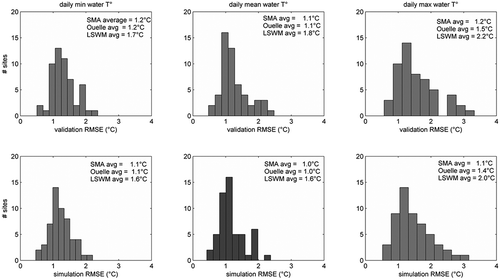
There are site to site variations in the simulation performances of the water temperature models (). Mean (standard deviation) RMSE values for the daily mean temperature in the St Marguerite, Ouelle and LSWM basins are 1.0(0.3)°C, 1.0(0.3)°C and 1.6(0.4)°C, respectively, with mean validation RMSEs 0.05–0.14°C higher. In general, RMSE values achieved for mainstem sites are higher than for refugia sites on the Ouelle and LSWM, but no appreciable mainstem-refugia difference was noted for the St Marguerite models. For example, daily mean temperature validation RMSEs are 0.25°C and 0.35°C higher, on average, for the mainstem sites than for refugia sites on the Ouelle and LSWM, respectively. Validation and simulation performances are close in general, indicating a good generalisation performance for the MLPs. MBE absolute values are all <0.1°C, with one exception.
4.2 Climate scenarios and the evolution of water temperature regimes
The summer water temperature metrics listed in were computed from the water temperature time series simulated given historical (observed during 1970–1999), reference (1970–1999) and future (2046–2065) air temperature and precipitation data. The resulting daily mean temperatures are shown for every site in . Reference and future values in are the median and standard deviations of the 10 daily mean values obtained using the 10 climate scenarios. Sites listed on the same line are refugium-mainstem (R-M) pairs.
Table 6 Daily mean temperatures (°C) for June–September computed from the water temperature time series simulated given historical (1970–1999), reference (1970–1999) and future (2046–2065) air temperature and precipitation data. Median and standard deviations (SD) of the 10 values obtained using the 10 climate scenarios are shown for every site. Sites on the same line are refugium-mainstem (R-M) pairs.
The mean summer temperature contrasts between R-M pairs are shown in . This metric is computed as the average of the daily mean temperature differences between the two paired sites. Due to a lack of data, no model could be calibrated right next to the St Marguerite R1 site, and the latter was thus paired with site M2 (see and ).
Table 7 Mean summer temperature contrast across refugium-mainstem pairs (R-M pair contrast, °C); computed from the water temperature time series simulated given historical, reference and future air temperature and precipitation data.
All other temperature metric values for the historical, reference and future periods were computed, and results for 7Dmax, %max24, %min20, Dmax24 and Tmax24 can be found in the Appendix (Tables A1–A5). Historical and reference values are very similar for all temperature metrics. The largest differences are found in the mean timing of the first events >24°C and >28°C, with most differences ranging from 0–9 d and most reference period standard deviation values between 1–8 d (). Almost all these differences are overestimations on the part of the reference scenarios, meaning that these tend to place the first high temperature events slightly later than what was observed.
A general picture of the thermal regime evolution in each of the three rivers is shown in . The box plots combine values for metrics computed for all mainstem sites and all 10 climate scenarios in a given basin, for reference and future periods. Metrics shown are 7Dmax, %max24, %max28, Dmax24, Tmax24 and %min20. shows the same information for the refugia sites.
Fig. 6 7Dmax, %max24, %max28, Dmax24, Tmax24 and %min20 values computed for all mainstem sites and all 10 climate scenarios in the three basins, for reference and future periods. On each box plot, the horizontal line is the median value, the edges of the box are the 25th and 75th percentiles, the vertical dashed line extend to the most extreme values not considered outliers, and the crosses are outliers.
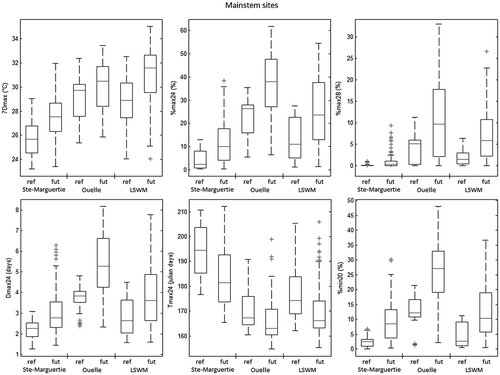
5 DISCUSSION
As expected, modelling performances on the extreme daily values are poorer than on the daily mean values, with mean RMSEs increasing by 0.1–0.4°C (). While daily mean values combine the whole range of daily temperatures, extreme values are responses to a combination of short-duration conditions. The temporal resolution of the predictors is too coarse to capture such conditions. In addition, as stated before, statistical models calibrated based on error minimization will, in general, underestimate the variance of the observed data. The variance inflation method used helped to improve the replication of the variance of the data, but at the expense of prediction precision (Jeong et al. Citation2013).
Validation and simulation RMSEs on the LSWM sites are approx. 0.6°C higher, on average, than those of the two other basins. This cannot be attributed to the amount of available data, or to a higher average summer temperature, which are similar, on average, for the three basins. This lower performance of the LSWM model could however be due to the fact that the meteorological data used to calibrate the water temperature models on the LSWM sites were acquired at a more remote station than that of the other basins (). Previous water temperature modelling studies have shown that the use of regional scale meteorological data for reach-scale modelling can lead to lower modelling performances, especially for more sheltered streams (Marcé and Armengol Citation2008, Benyahya et al. Citation2010, Hebert et al. Citation2011).
As expected, historical and reference values are very similar for all temperature metrics (, , A1–A5), showing that the debiased climate models were successful in representing the historical climatic environment of the rivers. The larger standard deviations obtained for the future period metrics reflects the fact that the climate models/SRES scenarios were deliberately selected based on their contrasting projected changes in summer air temperatures and precipitation.
The large overlaps between reference and future period values in and are mostly due to the fact that these combine all site values and thus the whole water temperature range in a given stream. Nevertheless, the comparisons of all the metric median values indicate a warming of the summer water temperatures between the reference and future periods, in all of the three watersheds. The 7-day maximum median values, for instance, show 1.9, 0.7 and 2.7°C increases for the St Marguerite, Ouelle and LSWM mainstems, and 1.5, 0.6 and 1.9°C increases in the refugia of the same respective rivers. The highest relative changes occur in the median values of %max24 and %min20 in the river mainstems, with percentages of days exceeding 24°C daily maximum and 20°C daily minimum thresholds doubling and even quadrupling from reference to future periods. The %max24, %max28 and %min20 values remain low in most refugia sites, but the median duration of events >24°C (Dmax24) increase by 0.3–0.8 d, and the first >24°C event (Tmax24) occurs 3–7 d earlier. All reference and future period mean values were tested for significantly different means (one-way ANOVA test) and the null hypothesis of no difference was rejected with p < 10-4 for all mainstem sites, and with p < 0.01 for all but two refugia sites (%28max for the St Marguerite sites and Tmax24 for the Ouelle sites).
The evaluation of the number of potential thermal refugia available in the reference and future periods requires a definition of a thermal refugium, which does not exist in terms of seasonal water temperature requirements and is species dependent. Such a requirement could be that the temperature never reaches stressful values. A less restrictive, but equally relevant perspective, could be that the temperature stays lower than in the river mainstem, providing a permanent lower temperature option to the fish. To comply with these two perspectives, we chose to identify a site as a thermal refugium on the basis of two criteria: (1) a mean summer water temperature lower than 16°C, which is the lower/upper optimal water temperature for Atlantic salmon/brook trout parr growth (Jonsson and Jonsson Citation2009); and (2) a mean mainstem-refugium water temperature difference (as measured by the contrast metric) >1°C.
The second criterion is met by all listed refugia in the reference period (). Results show that the mean temperature contrast between the mainstem and potential refugia is rather stable between reference and future periods, even showing small increases for some sites.
According to the first criterion, all 23 listed R-sites in can be considered as thermal refugia during the reference period, and 19 remain in the future period (). Submitting the corresponding mainstem sites to the same criterion shows that half of the M-sites have mean daily temperature <16°C in the reference period, and only two (in the upstream St Marguerite) remain in the future period. This, and the general increases in the occurrence and duration of high temperature events, is an indication that the need for thermal refugia can be expected to grow in the future.
These results are consistent with studies attempting to quantify river temperature trends and predict future river water temperature. In a study conducted on streams in northwest United States, Arismendi et al. (Citation2013) measured significant warming trends of ~0.10–0.29°C per decade between 1979 and 2009. Isaak et al. (Citation2010) measured an increase in mean and maximum water temperatures of 0.3°C per decade in a network located in a mountainous region of Idaho. They estimate that this increase resulted in a 8–16% bull trout habitat loss per decade. Using relationships between stream isotherm shift rates, spatial temperature gradient, slope and sinuosity, Isaak and Rieman (Citation2013) estimate that isotherms shifted from 1.5 to 43 km during the 20th century in rivers of slope 0.1–3%, and will shift another 5–143 km in the next 50 years, assuming an additional 2°C warming prediction. Monk and Curry (Citation2009), using logistic stream temperature models for northeastern North American rivers, found that the consecutive number of weeks with mean weekly maximum temperature exceeding 20°C is projected to increase from 7.4 weeks (1980–2002 reference period) to 8.7–10.0 weeks, on average, in 2046–2055. On a more global scale, Van Vliet et al. (Citation2013) coupled physically-based hydrological and thermal models to predict river temperature at 347 sites distributed in different hydroclimatic zones on five continents, and found that the mean river temperatures are projected to increase by 0.5–2.4°C by 2071–2100, as compared to the 1971–2000 reference period.
While the main advantage of deterministic models is to explicitly represent causal relationships between reach-scale water temperature and its different drivers, the reliability of such models is generally limited by the poor availability of large amounts of diverse input data with adequate resolution. For example, the parameters representing a highly variable component of the model (e.g. direct heating by short wave solar radiation), will yield realistic physical conditions only if local weather and riparian zone and topographic shading conditions are known at the valley segment scale (Benyahya et al. Citation2010, Hebert et al. Citation2011). The data demands, regarding reach-scale groundwater–surface-water thermal exchanges, are similarly significant and daunting. For studies that aim to model water temperature at a large number of sites, the application of a complex deterministic model can thus be very data and time demanding. Depending on the objectives of the study, statistical models that define direct relationships between water temperature and a small number of easily available variables (e.g. air temperature), offer an interesting alternative. In the present study, site-specific empirical relationships were only defined between water temperature, air temperature and precipitation. Future changes in processes that are not directly expressed by air temperature and precipitation, such as changes in the flow regimes and riparian vegetation, are thus not accounted for by the models. For example, expected decreases in winter snow pack could lead to more severe summer low flows and thus more frequent high temperature events. These models may also not hold in extrapolation conditions, and may be unable to correctly model the impact of changes in climate variability. The models are also site-specific; inclusion of additional physiographic, geospatial and/or groundwater information could yield basin-scale or regional models, taking explicit account of the specific characteristics of the individual river reaches.
6 CONCLUSION
The main improvement from the models presented by Jeong et al. (Citation2013) is the fact that the models used in the present study can produce minimum and maximum daily water temperature, in addition to the mean daily temperature. The maximum daily temperature is useful to point out thermally stressful events. The minimum daily temperature was modelled because it represents the cooler period during which the fish may recover from the more thermally stressful hours. The %min20 metric thus computes days for which this recovering period is not available.
The aim of the models presented here is to contrast the thermal regime responses to climatic variability for many sites in three different river basins. Statistical empirical models were calibrated on a site-specific basis, providing site-specific adapted transfer functions between meteorological conditions and daily mean, minimum and maximum water temperatures. 25 MLPs were calibrated and validated for each site, and water temperature time series were simulated using this ensemble.
The abundance and persistence of thermal refugia is of capital importance for the survival of salmonid populations in many Canadian rivers. The calibrated models were input with future climate scenarios in order to evaluate the evolution of the thermal regimes, and to assess how many potential thermal refugia will remain in the future (2046–2065).
Results suggest that 19 of the 23 identified potential refugia will remain potentially useful in the future. Results also indicate that according to 10 water temperature metrics describing mean daily values, weekly maximum values and occurrence, duration and timing of high temperature events, the thermal regimes of all three rivers will be significantly affected by climatic changes. Even if most tributaries studied here are expected to stay at daily mean temperatures <16° on average, between June and September, the higher mean temperature and important increases in occurrence and duration of >24°C and >28°C temperature events in the mainstems of rivers will lead to higher demands for thermal refugia in the salmonid populations.
The models presented are empirical relationships between air temperature, precipitation and water temperature. The limited spatial resolution of the water temperature monitoring network, and the limited length of the water temperature time series, remain major limitations in the development of empirical water temperature models. In spite of the fact that water temperature monitoring has become inexpensive and its importance is gaining recognition, high resolution and/or long-term surveys are still rare in Canada.
Disclosure statement
No potential conflict of interest was reported by the author(s).
Acknowledgements
The authors would like to thank D. Caissie for providing water temperature and local meteorological data for the LSWM, A. Maheu for producing the maps presented in this paper, T. Linnansaari and his team for collecting water temperature data in the LSWM, A. Boivin for his assistance in deploying thermographs on the SMA, as well as the graduate and summer students who contributed to the field work.
Additional information
Funding
REFERENCES
- Arismendi, I., et al., 2013. Descriptors of natural thermal regimes in streams and their responsiveness to change in the Pacific Northwest of North America. Freshwater Biology, 58, 880–894. doi:10.1111/fwb.12094
- Bacon, P.J., et al., 2005. Seasonal growth patterns of wild juvenile fish: partitioning variation among explanatory variables, based on individual growth trajectories of Atlantic salmon (Salmo salar) parr. Journal of Animal Ecology, 74, 1–11. doi:10.1111/j.1365-2656.2004.00875.x
- Benyahya, L., et al., 2007. A review of statistical water temperature models. Canadian Water Resources Journal, 32 (3), 179–192. doi:10.1111/j.1095-8649.2010.02762.x
- Benyahya, L., et al., 2008. Comparison of non-parametric and parametric water temperature models on the Nivelle River, France. Hydrological Sciences Journal, 53 (3), 640–655. doi:10.1623/hysj.53.3.640
- Benyahya, L., et al., 2010. Comparison of microclimate vs. remote meteorological data and results applied to a water temperature model (Miramichi River, Canada). Journal of Hydrology, 380, 247–259. doi:10.1016/j.jhydrol.2009.10.039
- Breau, C., Cunjak, R.A., and Bremset, G., 2007. Age-specific aggregation of wild juvenile Atlantic salmon Salmosalar at cool water sources during high temperature events. Journal of Fish Biology, 71, 1179–1191. doi:10.1111/j.1095-8649.2007.01591.x
- Breau, C., Cunjak, R.A., and Peake, S.J., 2011. Behaviour during elevated water temperatures: can physiology explain movement of juvenile Atlantic salmon to cool water? Journal of Animal Ecology, 80, 844–853. doi:10.1111/j.1365-2656.2011.01828.x
- Caissie, D., 2006. The thermal regime of rivers: a review. Freshwater Biology, 51, 1389–1406. doi:10.1111/j.1365-2427.2006.01597.x
- Caissie, D., El-Jabi, N., and Satish, M.G., 2001. Modelling of maximum daily water temperatures in a small stream using air temperatures. Journal of Hydrology, 251 (1–2), 14–28. doi:10.1016/S0022-1694(01)00427-9
- Caissie, D., Satish, M.G., and El-Jabi, N., 2007. Predicting water temperatures using a deterministic model: application on Miramichi River catchments (New Brunswick, Canada). Journal of Hydrology, 336 (3–4), 303–315. doi:10.1016/j.jhydrol.2007.01.008
- Chenard, J-F. and Caissie, D., 2008. Stream temperature modelling using artificial neural networks: application on Catamaran Brook, New Brunswick, Canada. Hydrological Processes, 22, 3361–3372. doi:10.1002/hyp.6928
- Chiew, F., et al., 2010. Comparison of runoff modelled using rainfall from different downscaling methods for historical and future climates. Journal of Hydrology, 387 (1–2), 10–23. doi:10.1016/j.jhydrol.2010.03.025
- Chu, C., Mandrak, N.E., and Minns, C.K., 2005. Potential impacts of climate change on the distributions of several common and rare freshwater fishes in Canada. Diversity and Distributions, 11, 299–310. doi:10.1111/j.1366-9516.2005.00153.x
- Chun, K.P., Wheater, H.S., and Onof, C., 2013. Comparison of drought projections using two UK weather generators. Hydrological Sciences Journal, 58 (2), 295–309. doi:10.1080/02626667.2012.754544
- Cluis, D., 1972. Relationship between stream water temperature and ambient air temperature – a simple autoregressive model for mean daily stream water temperature fluctuation. Nordic Hydrology, 3 (2), 65–71.
- Cunjak, R.A., et al., 2005. Using stable isotope analysis with telemetry or mark-recapture data to identify fish movement and foraging. Oecologia, 144, 636–646. doi:10.1007/s00442-005-0101-9
- Daigle, A., Ouarda, T.B.M.J., and Bilodeau, L., 2010. Comparison of parametric and non-parametric estimations of the annual date of positive water temperature onset. Journal of Hydrology, 390 (1–2), 75–84. doi:10.1016/j.jhydrol.2010.06.032
- Demuth, H. and Beale, M., 2000. Neural Network Toolbox User’s Guide v. 4. The MathWorks Inc., 465 p.
- Eaton, J.G. and Scheller, R.M., 1996. Effects of climate warming on fish thermal habitat in streams of the United States. Limnology and Oceanography, 41 (5), 1109–1115. doi:10.4319/lo.1996.41.5.1109
- Elliott, J.M. and Elliott, J.A., 2010. Temperature requirements of Atlantic salmon Salmosalar, brown trout Salmotrutta and Arctic charr Salvelinusalpinus: predicting the effects of climate change. Journal of Fish Biology, 22, 1793–1817.
- Erickson, T.R. and Stefan, H.G., 2000. Linear air/water temperature correlations for streams during open water periods. Journal of Hydrologic Engineering, 5, 317–321. doi:10.1061/(ASCE)1084-0699(2000)5:3(317)
- Gordon, H.B., et al., 2002. The CSIROMk3 climate system model. Tech. Rep. 60, CSIRO Atmos. Res., Aspendale, Victoria, Australia.
- Hasumi H. and Emori S., eds., 2004. K-1 coupled model (MIROC description. K-1 Tech. Rep. 1, Cent for Clim. SySt Res., Univ. of Tokyo, Tokyo, Japan.
- Hannah, D.M., et al., 2008. A comparison of forest and moorland stream microclimate, heat exchanges and thermal dynamics. Hydrological Processes, 22, 919–940. doi:10.1002/hyp.7003
- Hebert, C., et al., 2011. Study of stream temperature dynamics and corresponding heat fluxes within Miramichi River catchments (New Brunswick, Canada). Hydrological Processes. doi:10.1002/hyp.8021
- Huard, D. and Chaumont, D., 2011. Climate Change Scenarios – Thermal refuge for salmonids. Ouranos report SCEN-No. 2011–02.
- Isaak, D.J., et al., 2010. Effects of climate change and wildfire on stream temperatures and salmonid thermal habitat in a mountain river network. Ecological Applications, 20 (5), 1350–1371. doi:10.1890/09-0822.1
- Isaak, D.J. and Rieman, B.E., 2013. Stream isotherm shifts from climate change and implications for distributions of ectothermic organisms. Global Change Biology, 19, 742–751. doi:10.1111/gcb.12073
- Jeong, D.I., Daigle, A., and St-Hilaire, A., 2013. Development of a stochastic water temperature model and projection of future water temperature and extreme events in the Ouelle River basin in Quebec, Canada. River Research and Applications, 29 (7), 805–821. doi:10.1002/rra.2574
- Jonsson, B. and Jonsson, N., 2009. A review of the likely effects of climate change on anadromous Atlantic salmon Salmo salar and brown trout Salmo trutta, with particular reference to water temperature and flow. Journal of Fish Biology, 75, 2381–2447. doi:10.1111/j.1095-8649.2009.02380.x
- Leach, J.A. and Moore, R.D., 2011. Stream temperature dynamics in two hydrogeomorphically distinct reaches. Hydrological Processes, 25, 679–690. doi:10.1002/hyp.7854
- MacKay, D.J.C., 1992. Bayesian interpolation. Neural Computation, 4 (3), 415–447. doi:10.1162/neco.1992.4.3.415
- Mantua, N., Tohver, I., and Hamlet, A., 2010. Climate change impacts on streamflow extremes and summertime stream temperature and their possible consequences for freshwater salmon habitat in Washington State. Climatic Change, 102, 187–223. doi:10.1007/s10584-010-9845-2
- Marcé, R. and Armengol, J., 2008. Modelling river water temperature using deterministic, empirical, and hybrid formulations in a Mediterranean stream. Hydrological Processes, 22, 3418–3430. doi:10.1002/hyp.6955
- Meehl, G.A., et al., 2007. Global Climate Projections. In: S. Solomon, D. Qin, M. Manning, Z. Chen, M. Marquis, K.B. Averyt, M. Tignor, H.L. Miller, eds. Climate Change 2007: the physical science basis. Contribution of Working Group I to the Fourth Assessment Report of the Iintergovernmental Panel on Climate Change. Cambridge: Cambridge University Press.
- Mohseni, O. and Stefan, H.G., 1999. Stream temperature/air temperature relationship: a physical interpretation. Journal of Hydrology, 218, 128–141. doi:10.1016/S0022-1694(99)00034-7
- Monk, W.A. and Curry, R.A., 2009. Models of past, present and future stream temperatures for selected Atlantic salmon rivers in Northeastern North America. American Fisheries Society Symposium, 69, 215–230.
- Perazzoli, M., Pinheiro, A., and Kaufmann, V., 2013. Assessing the impact of climate change scenarios on water resources in southern Brazil. Hydrological Sciences Journal, 58 (1), 77–87. doi:10.1080/02626667.2012.742195
- Roeckner, E., et al., 2006. Sensitivity of simulated climate to horizontal and vertical resolution in the ECHAM5 atmosphere model. Journal of Climate, 19, 3771–3791. doi:10.1175/JCLI3824.1
- Scinocca, J.F. and McFarlane, N.A., 2004. The variability of modeled tropical precipitation. Journal of Atmospheric Science, 61, 1993–2015. doi:10.1175/1520-0469(2004)061<1993:TVOMTP>2.0.CO;2
- St-Hilaire, A., et al., 2011. Daily river water temperature forecast model with a k-nearest neighbour approach. Hydrological Process. doi:10.1002/hyp.8216.
- Themeßl, M.J., Gobiet, A., and Leuprecht, A., 2010. Empirical-statistical downscaling and error correction of daily precipitation from regional climate models. International Journal of Climatology. doi:10.1002/joc. 2168.
- van Vliet, M.T.H., et al., 2013. Global river discharge and water temperature under climate change. Global Environmental Change, 23, 450–464. doi:10.1016/j.gloenvcha.2012.11.002
- Wu, S.-Y., 2010. Potential impact of climate change on flooding in the Upper Great Miami River Watershed, Ohio, USA: a simulation-based approach. Hydrological Sciences Journal, 55 (8), 1251–1263. doi:10.1080/02626667.2010.529814
APPENDIX
Table A1 7-day maximum daily temperature (7Dmax) computed from the water temperature time series simulated given historical, reference and future air temperature and precipitation data. SD: standard deviation.
Table A2 Percentage of days with maxima exceeding 24°C (%max24); computed from the water temperature time series simulated given historical, reference and future air temperature and precipitation data.
Table A3 Percentage of days with minimum temperature exceeding 20°C (%min20); computed from the water temperature time series simulated given historical, reference and future air temperature and precipitation data.
Table A4 Duration (in days) of events exceeding 24°C daily maxima (Dmax24); computed from the water temperature time series simulated given historical, reference and future air temperature and precipitation data.
Table A5 Timing of first event exceeding 24°C daily maxima (Tmax24); computed from the water temperature time series simulated given historical, reference and future air temperature and precipitation data.