Abstract
Flood frequency estimation is crucial in both engineering practice and hydrological research. Regional analysis of flood peak discharges is used for more accurate estimates of flood quantiles in ungauged or poorly gauged catchments. This is based on the identification of homogeneous zones, where the probability distribution of annual maximum peak flows is invariant, except for a scale factor represented by an index flood. The numerous applications of this method have highlighted obtaining accurate estimates of index flood as a critical step, especially in ungauged or poorly gauged sections, where direct estimation by sample mean of annual flood series (AFS) is not possible, or inaccurate. Therein indirect methods have to be used. Most indirect methods are based upon empirical relationships that link index flood to hydrological, climatological and morphological catchment characteristics, developed by means of multi-regression analysis, or simplified lumped representation of rainfall–runoff processes. The limits of these approaches are increasingly evident as the size and spatial variability of the catchment increases. In these cases, the use of a spatially-distributed, physically-based hydrological model, and time continuous simulation of discharge can improve estimation of the index flood. This work presents an application of the FEST-WB model for the reconstruction of 29 years of hourly streamflows for an Alpine snow-fed catchment in northern Italy, to be used for index flood estimation. To extend the length of the simulated discharge time series, meteorological forcings given by daily precipitation and temperature at ground automatic weather stations are disaggregated hourly, and then fed to FEST-WB. The accuracy of the method in estimating index flood depending upon length of the simulated series is discussed, and suggestions for use of the methodology provided.
Editor D. Koutsoyiannis
Résumé
L’estimation de la fréquence des crues est cruciale tant dans la pratique de l’ingénierie que pour la recherche en hydrologie. L’analyse régionale des débits de pointe est utilisée pour des estimations plus précises des quantiles de crues dans les bassins non jaugés ou mal jaugés. Cette analyse est basée sur l’identification de zones homogènes, où la distribution de probabilité des débits annuels de pointe maximale est invariante, à l’exception d’un facteur d’échelle représenté par un indice de crue. Les nombreuses applications de cette méthode ont permis de montrer que l’obtention d’estimations précises de l’indice de crue était une étape cruciale, en particulier dans les sections non jaugées ou mal jaugées, où l’estimation directe par moyenne de l’échantillon de la série annuelle de crue (AFS) est impossible, ou inexacte. Des méthodes indirectes doivent donc être utilisées. La plupart des méthodes indirectes sont fondées sur des relations empiriques qui lient l’indice de crue aux caractéristiques hydrologiques, climatologiques et morphologiques des bassins versants, qui sont obtenues grâce à une analyse multi-régression, ou à une représentation globale simplifiée des processus pluie-débit. Les limites de ces approches sont de plus en plus évidentes à mesure que la taille et la variabilité spatiale des bassins versants augmentent. Dans ces cas, l’utilisation d’un modèle hydrologique semi-distribué à base physique, et la simulation continue des débits, peuvent améliorer l’estimation de l’indice de crue. Ce travail présente une application du modèle FEST-WB pour la reconstruction de 29 ans de débits horaires pour un bassin versant nival alpin dans le Nord de l’Italie, qui a été utilisée pour l’estimation de l‘indice de crue. Pour prolonger la durée de la série de débits simulés, les forçages météorologiques fournis par les précipitations quotidiennes et de la température aux stations météorologiques automatiques au sol ont été désagrégées au pas de temps horaire, puis fournies au modèle FEST-WB. Nous avons discuté la précision de la méthode pour estimer l’indice de crue en fonction de la longueur de la série simulée, et nous proposons des éléments d’ordre méthodologique.
1 INTRODUCTION
Several approaches are possible for estimating design floods (Ashfaq and Webster Citation2000, Yue et al. Citation2002, Rulli and Rosso Citation2002, Bocchiola et al. Citation2003, Nasri et al. Citation2004, Bocchiola and Rosso Citation2009). When long records of measured streamflow data are available, direct statistical analysis may be feasible. However, the streamflow data series are often too short to perform robust statistical inference. Benson (Citation1962) indicated that reliable quantile estimates can be obtained only for return periods <2n, where n denotes the number of years of observations.
Regionalization procedures overcome these difficulties increasing the sample size by trading space for time (De Michele and Rosso Citation2002). In ungauged or poorly gauged catchments, regional analysis of flood peak discharges is used to obtain more accurate estimates of flood quantiles. This method considers that the statistical distribution of the floods within a homogenous region is similar, except for a scale factor represented by an index flood, usually the mean annual flood (NERC Citation1975).
Depending on the available information, the index flood can be estimated according to different methods (e.g. Rulli and Rosso Citation2002, Bocchiola et al. Citation2003). In ungauged or poorly gauged sections, direct estimation by sample mean of annual flood series is not possible or inaccurate. Prediction in ungauged or poorly gauged basins is a tremendously important issue in modern hydrology, and a number of activities has been fostered within the scientific community in the last decade. In particular, the International Association of Hydrological Sciences (IAHS) launched the Prediction in Ungauged Basins (PUB) initiative, covering the decade 2003–2012, and aimed to foster major advances in our capacity to make predictions in areas with poor coverage of hydrological data (Sivapalan Citation2003, Seibert and Beven Citation2009). Mountainous snow-fed catchments represent typical areas for the application of PUB concepts, where hydrological modelling may be necessary for water budget estimation and flood prediction (Chalise et al. Citation2003, Konz et al. Citation2007, Simaityte et al. Citation2008, Bocchiola et al. Citation2010, Citation2011). Pillars of the PUB initiative and methodology are the concepts of catchment classification (Burn Citation1997, Castellarin et al. Citation2001, Citation2008, Parajka et al. Citation2005, Merz and Blöschl Citation2009) and model portability (Bárdossy Citation2007, Buytaert et al. Citation2008, Castiglioni et al. Citation2010), basic tools to extrapolate results within measured areas to ungauged sites. However, the use of such tools requires accurate knowledge of the physiographic, climatic and hydrologic attributes of some measured catchments within a certain region, and their proper treatment, in order to complement the analysis of unmeasured areas. Most indirect methods are based upon empirical relationships that link the index flood to hydrological, climatological and morphological catchment characteristics, developed by means of multiregression analysis, or simplified lumped representation of the process of transformation of intense rainfall into runoff. The limits of these approaches are increasingly evident as the size and heterogeneity of the catchment increase. In these cases, accurate continuous hydrological simulation enables the reconstruction of river flow time series at a given river site (Boughton and Droop Citation2003, Groppelli et al. Citation2011b). From this, the series of maximum annual flood peaks is extracted, and the index flood can be estimated as the mean of sample data.
Distributed hydrological models have become very common in research activities for their capability to describe the spatial variability of processes, input, boundary conditions and watershed characteristics (Rosso Citation1994, Beven Citation2001, Rosso and Rulli Citation2002). They are potentially able to simulate the river flow at any location of the watershed drainage network. Furthermore state of the art techniques for calibration of parameters related to snowmelt and snow accumulation require remotely sensed images of snow coverage and point site information of snow depth/density (Corbari et al. Citation2009, Boscarello et al. Citation2012). Comparison between snow-covered area, such as from satellite data, is facilitated by the use of a spatially-distributed model, as it better matches the pixel structure of remotely sensed images. Although indeed the output of distributed models can be used to compare with spatially distributed variables obtained through remote sensing, it is not done in this study, but could be carried out in the future. Hydrological models applied to Alpine basins typically simulate hydrological dynamics at the sub-daily (e.g. hourly) scale, depending on the characteristics of the system, and thus requiring the use of consistently measured meteorological variables. When data with coarser (e.g. daily/monthly) resolution than necessary are available, downscaling may be used, to make the meteorological input consistent with the model resolution (e.g. Bocchiola et al. Citation2011).
Index flood assessment is an important issue within the framework of regional flood estimation, and there are many applications in the literature about continuous simulation for flood estimation. A review of continuous simulation applications for design flood estimation is found in Boughton and Droop (Citation2003); they report operational systems for Australia, Europe, South Africa, the UK, and the USA. Cameron et al. (Citation2000) presented a continuous simulation methodology, applied to four UK catchments, based on the rainfall–runoff model TOPMODEL coupled to a stochastic rainfall generator within the uncertainty framework of GLUE. Moretti and Montanari (Citation2008) applied the AFFDEF distributed hydrological model to river basins over the Appennine mountains, in Italy, in conjunction with a 100-year multisite hourly rainfall record synthetically generated by using the multivariate Neyman-Scott rectangular pulses model. Viviroli et al. (Citation2009) applied the PREVAH model to flood estimation of gauged and ungauged mesoscale catchments in Switzerland. None of these papers refers specifically to the index flood in our understanding.
The main objective of this study is to investigate the capability of the continuous distributed hydrological model FEST-WB (Montaldo et al. Citation2007, Rabuffetti et al. Citation2008) to reconstruct river flow time series of the Toce River basin, an Alpine basin located in northern Italy, for the purpose of index flood estimation. The model was driven by hourly precipitation and temperature measurements. In order to increase the number of simulated years and the density of meteorological station networks, daily precipitation and temperature measurements were downscaled to the hourly time step. We show that the distributed hydrological model may be used to build simulated annual flow series by way of long-term hydrological simulation and to fill gaps in measured discharge series.
2 MATERIAL AND METHODS
2.1 The Toce River catchment
The Toce basin is a typical mountain basin, with steep hillslopes surrounding a narrow valley located mainly in the north Piedmont region of Italy, and partially in Switzerland (10% of the total area), with a total drainage area of about 1800 km2 (). Its elevation ranges from 193 m a.s.l. at the outlet, to approximately 4600 m a.s.l. at the Monte Rosa crest; the average elevation is 1641 m a.s.l. The precipitation regime according to the Köppen-Geiger climate classification (e.g. Peel et al. Citation2007) belongs to the temperate/cool continental class, featuring seasonal continuous snow cover above 1000 m a.s.l. or so, a maximum of precipitation during the end of the summer and autumn, and a minimum during the winter. Runoff is mainly influenced therein by snowmelt in spring and by rainfall in early autumn.
The land cover is: forest (70%), bare rocks (9%), agricultural (7%), natural grassland (6%), urban (4%), water bodies (3%), and glaciers and perpetual snow (1%). The Toce basin lithology displays five main classes: augean gneiss (49%), micaceous schists (27%), calcareous schists (11%), grindstones (7%), and granites (6%). The steep hillslopes, forming the most significant area of the basin, are mostly covered by trees, upon thin soil layers resting on bedrock. Soil thickness increases in the downstream narrow alluvial area.
A digital elevation model (DEM) is available at 200 m × 200 m resolution, as derived by 1:10 000-scale topographic maps (Regione Piemonte Citation1997). The digital land-use map was derived by coupling CORINE land cover (CEC Citation2000) for the Italian part and the Swiss land-use map (Arealstatistik) for the part of the basin nested in Switzerland, both derived from remote sensing observations (Montaldo et al. Citation2004).
2.2 Hydrological and meteorological data
Meteorological and hydrological data were collected by a telemetric monitoring system of the Regione Piemonte flood warning system (). Hourly precipitation and air temperature time series are available at 25 stations. The number of stations increased during 1988–2010, as reported in . Daily time series are available for the period 1982–1990 at four precipitation and three air temperature stations ().
Fig. 2 Available hourly precipitation and air temperature measuring stations for the period 1998–2010.
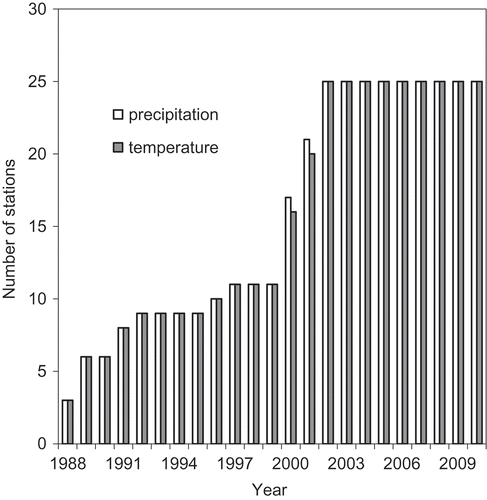
Table 1 Meteorological stations, and available hourly precipitation and temperature data.
Hourly river discharge data at Candoglia (basin area: 1534 km2, ) are available for the period 1 January 2000 to 31 December 2010. Maximum annual flow series (AFS) data are available for the period 1933–1998 for 53 available years.
2.3 Downscaling of precipitation and temperature in the time domain
Over the period 1982–1990, daily values of precipitation (four stations, daily cumulate) and temperature (three stations, max, min daily values) were available (), incompatible with the hourly simulation required herein. We thus developed a procedure for downscaling (in time) the daily data. We did not pursue spatial downscaling of precipitation (normally much more variable in space than temperature, more regularly controlled by altitude), because (a) the precipitation stations are quite evenly spread within the catchments from north to south (), and (b) a preliminary study indicated that interpolation of precipitation fields using the Thiessen method was accurate enough for the purpose of mimicking flood formation in the area. Concerning hourly precipitation, we adopted a downscaling method based upon the theory of Stochastic Time Random Cascade (STRC; Bocchiola and Rosso Citation2006, Bocchiola et al. Citation2011), including intermittence (i.e. dry spells), calibrated herein using currently available hourly data within the Toce catchments from raingauges near those with daily data. This is done based on the hypothesis that hourly structure of precipitation remains unchanged in time for gauges nearby, while daily average intensity may vary. For an explanation of the downscaling approach, the reader is referred to previous papers (Bocchiola and Rosso Citation2006, Bocchiola et al. Citation2011, Groppelli et al. Citation2011a). Concerning temperature, we had available min and max daily temperatures, Tmin and Tmax. To obtain hourly temperatures, we adopted a sinusoidal function, with phase shift Sh = 3 h, and period P°C = 24 h, with a minimum at 06:00 (dawn) and maximum at 15:00 (Waichler and Wigmosta Citation2003). By visual analysis, we found that this pattern is representative enough of hourly temperature as observed within stations, with hourly data available for in the period 1990–1999.
2.4 The distributed hydrological model FEST-WB
For rainfall–runoff transformation we employed the flash-Flood Event-based Spatially distributed rainfall–runoff Transformation, including Water Balance model (FEST-WB; Rabuffetti et al. Citation2008, Pianosi and Ravazzani Citation2010), developed on the MOSAICO library (Ravazzani Citation2013). The FEST-WB model computes the main processes of the hydrological cycle: evapotranspiration, infiltration, surface runoff, flow routing, subsurface flow and snow dynamics. The computation domain is discretized with a mesh of regular square cells (200 m × 200 m herein) in each of which water fluxes are calculated at the hourly time step. Previous studies have shown that the 200 m × 200 m resolution is a good compromise between accuracy and computational cost for the Toce case study (Montaldo et al. Citation2007).
Most of the parameter maps were produced in fulfilling the European Union research project ‘Runoff and Atmospheric Processes for flood HAzard forEcasting and control’ (RAPHAEL), the objective of which was to improve flood forecasting in complex mountain watersheds (Bacchi and Ranzi Citation2003, Montaldo et al. Citation2007). Further details of the parameters used by the model can be found in Montaldo et al. (Citation2007).
Five principal components can be identified in FEST-WB (): (1) the flow paths and channel network definition, (2) the spatial interpolation of meteorological forcings, (3) the simulation of glaciers and snow pack dynamics, (4) the runoff computation, and (5) the overland flow and base flow routings.
In the first component, the flow path network is automatically derived from the DEM, using a least-cost path algorithm (Ehlschlaeger Citation1989). It delivers flow from each pixel to its eight neighbours, without the need for pits removal in the elevation grid. The domain is divided into hillslope and channel cells using the area–slope algorithm (Giannoni et al. Citation2005).
In the second component, ground station data are interpolated onto a regular grid using the Thiessen (Citation1911) polygon method. Spatial distribution of point-wise air temperature takes into account the reduction of temperature with altitude, with a constant lapse rate of −0.0065°C m-1. Thermal inversion phenomena are neglected. Precipitation interpolation does not take into account elevation differences, as the number of available gauges is enough to give a good description of precipitation field.
In the third component, snow pack modelling is performed. The snow module includes snowmelt and snow accumulation dynamics. The partitioning of total precipitation, P [L T-1], in liquid, Pl [L T-1], and solid, Ps [L T-1] phases is a function of air temperature, Ta (°C) (Tarboton et al. Citation1994):
where αP (-) is computed by:
where Tlow (°C) and Tup (°C) are air temperatures below/above which all precipitation falls as snow/rain, respectively, to be found by means of calibration (Corbari et al. Citation2009, Boscarello et al. Citation2012). Snowmelt is modelled according the degree-day concept (Martinec and Rango Citation1986). The melt rate, Msnow [L T-1] is proportional to the difference between air temperature, Ta (°C), and a predefined threshold temperature, Tb,snow (°C), typically equal to 0°C:
where Csnow [L °C-1 T-1] is an empirical coefficient depending upon meteorological conditions and geographical location. The terrain covered by snow is assumed to be frozen and, hence, melted water cannot infiltrate into the soil. Conversely, the liquid fraction of snow water equivalent, Rs, the sum of melted water and liquid precipitation, is supposed to flow cell by cell through the snow pack with a linear reservoir routing scheme (Ponce Citation1989), with a celerity of 1.7 × 10-3 m s-1 (Salandin et al. Citation2004). When Rs reaches a cell not covered by snow, it is added to liquid precipitation in that cell.
In the fourth component, runoff is computed for each cell according to a modified Soil Conservation Service curve number (SCS-CN) method extended for continuous simulation (Ravazzani et al. Citation2007), where the potential maximum retention S is updated at the onset of storms as a linear function of the degree of saturation, ε:
where S1 (mm) is the maximum value of S (mm) for dry soil (AMC 1).
The dynamic of the volumetric soil moisture, θ [-], for cells not covered by snow, is cast by the water balance equation:
where Su is net subsurface contribution routed from upstream cell [L T-1], R is the surface runoff flux [L T-1], D is the drainage flux [L T-1], ET is actual evapotranspiration rate [L T-1] and Sd is the soil depth [L]. On a hillslope cell, liquid precipitation, Pl, includes run-on, that is the fraction of runoff routed from the upstream cell. The volumetric soil moisture in cells covered by snow is assumed constant with time.
The actual evapotranspiration rate is given by:
where Ebs is the actual rate of bare soil evaporation [L T-1], T is the actual rate of transpiration [L T-1], and fbs [-] and fv [-] are the fraction of the bare soil and vegetated area, respectively (fbs + fv = 1).
The actual rates of bare soil evaporation and transpiration are computed as a fraction of potential evapotranspiration, PET [L T-1]:
where
and θfc [-] and θwp [-] are the field capacity and wilting point, respectively.
Potential evapotranspiration is given by:
where Kc is a crop coefficient (Allen et al. Citation1998) and PET0 (mm d-1) is the reference potential evapotranspiration, computed with a temperature-based equation specifically developed for the Alpine environment (Ravazzani et al. Citation2012):
where z is elevation (m a.s.l.), Ra is extraterrestrial radiation evaporative power (mm d-1), Tmax is daily maximum air temperature (°C), Tmin is daily minimum air temperature (°C), HC is an empirical coefficient (HC = 0.0023), HE is an empirical exponent (HE = 0.5), and HT is needed to convert units of Fahrenheit to Celsius (HT = 32/1.8 = 17.8) (Hargreaves Citation1994).
In the fifth component of FEST-WB, the surface flow routing for snow-free cells is computed using the Muskingum-Cunge method in its nonlinear form, with a time-variable celerity (Montaldo et al. Citation2007). Subsurface flow routing is computed using a linear reservoir routing scheme (Ponce Citation1989), with a celerity calculated as a function of the soil saturated conductivity.
For further details on the development of the FEST-WB model, the reader is referred to Ravazzani et al. (Citation2009, Citation2011).
2.5 Performance of the hydrological model
The FEST-WB model was not calibrated by tuning against data, given that the first values assigned to parameters, based upon either measured values, the use of reference literature, or an educated guess, provided satisfactory results in terms of time series discharge simulation. Calibration of snow module parameters was performed in a previous study described in Boscarello et al. (Citation2012). Performance of the model was assessed by comparison of daily simulated and observed discharge at Candoglia for the period 2000–2010.
Goodness-of-fit indices used herein are the root mean square error, RMSE, and Nash-Sutcliffe efficienty (Nash and Sutcliffe Citation1970), η, defined, respectively, as:
where n is the total number of time steps, Qisim is the ith simulated discharge, Qiobs is the ith observed discharge, and is the mean of the observed discharges. The values of RMSE and η were 29.53 m3 s-1 and 0.88, respectively.
In , a comparison between observed and FEST-WB simulated discharge at the hourly scale is shown, for the two flood events that occurred in 2004 and 2008.
2.6 Index flood estimation
In ungauged or poorly gauged catchments, regional analysis of flood peak discharges can be used for flood quantile design. This is based on the identification of homogeneous zones, where the probability distribution of annual maximum peak flows is invariant, except for a scale factor represented by an index flood, μQ (Dalrymple Citation1960, Bocchiola et al. Citation2003). The index flood method is based on the estimation of the regional growth curve of a dimensionless quantile, xT. Accordingly, the T-year flood flow qT is estimated as (Brath et al. Citation1997, De Michele and Rosso Citation2002):
Direct assessment of the index flood could be performed from the annual flood series (AFS). If, at a given river site, an n-year maximum annual flood peak series of measurements is available, the index flood can be estimated as the mean of sample data q1, …, qn:
2.7 Accuracy of index flood
One is interested in knowing how many years of model simulation (i.e. how many values of greatest annual peaks ny simulated using the FEST-WB model) are necessary to estimate the index flood with a given degree of accuracy. This could be assessed by investigating the mean square error of estimation of index flood as a function of ny, namely σny. To investigate this, we developed the following approach. For an increasing number of samples (i.e. years) ny, we calculated the sample value of index flood, μny, and the standard error of estimate, σny, similarly to equations (14) and (15) as:
for ny < n. To avoid dependence upon sample effects as given by sequential use of the observed discharge, and to make the sample more robust, we evaluated µny and σny using 100 sample as obtained by random choice of ny values of qj within the n size initial samples. We then evaluated an average (of the 100 extractions) value of σny. We used (a) observed data, (b) partial simulation data (1982–2010|24), and (c) total simulation data (1982–2010). This is reasonable because (i) one may have a not continuous series of observed data, i.e. when conspicuous lack of data is found, and (ii) one may not be able to simulate flow data using FEST-WB (or any other hydrological model) for a continuous series of years, i.e. when meteo data are not available for some years, or short periods, say during the flood season, so hampering peak flood estimation.
3 RESULTS AND DISCUSSION
3.1 Extreme values
Continuous simulation performed by means of a distributed hydrological model allows the reconstruction of hourly river discharge at any given section. From these data, time series of maximum annual discharge can be computed. In maximum annual discharges computed by hydrological model at Candoglia and Toce outlets are shown. Moreover, river discharges at Candoglia are compared against maximum annual observed discharges therein. Note that 5 years of observations are missing (1985–1988 and 1999). Significant lack of data was found in 1985 and 1987, when two major floods occurred. The 1987 flood () was a catastrophic event that occurred in August, caused by heavy precipitation associated with high temperatures (freezing level in the range 3000–3200 m a.s.l.). A large amount of damage was reported to buildings and roads, including the collapse of some bridges caused by both flooding, debris flow and soil slips (Giampani et al. Citation2008). In both 1985 and 1987, the high flood discharges induced failure of river gauging equipment, so that the observed series are missing.
3.2 Benchmarking of index flood estimates
In , the index flood, standard error of estimate and standard deviation are reported as retrieved from samples of observed and simulated maximum annual discharges at Candoglia for the period 1982–2010. Moreover, we report the index flood as calculated for 1982–2010, but excluding the years when the corresponding observations are missing. Also, the index flood is calculated using all the available maximum annual discharges observed during 1933–2010.
The index flood computed on the basis of 24 observations in the period 1982–2010 is 945 m3 s-1, with a standard error of 132 m3 s-1 (standard deviation of the AFS series, SD is 648 m3 s-1). Very similar results are obtained when considering the sample of simulated discharge including only data when corresponding observations are available. This confirms the accuracy of the hydrological model in the reconstruction of the AFS series at Candoglia. When all 29 simulated years are included in the analysis, the index flood increases to 1068 m3 s-1, with a standard error of estimation of 152 m3 s-1 (SD: 816 m3 s-1). This difference is mainly due to the two floods that occurred in 1985 and 1987, which exceeded 2000 and 3000 m3 s-1, respectively. The index flood computed using the complete observed dataset (62 years) for the period 1933–2010 is 1090 m3 s-1, with a standard error of estimate of 82 m3 s-1, and SD of 644 m3 s-1.
3.3 Accuracy of index flood estimation vs number of available years
In the results of the procedure described in Section 2.7 are shown. We report the three cases of peak flood discharge values: (a) observed, (b) partially simulated (with FEST-WB), and (c) totally simulated (with FEST-WB). For reference, the dimensionless variable is given by σny* = σny/µn (%), i.e. the percentage standard estimation error of index flood. Except for the case of ny = 3, which is very low, σny* seems substantially equivalent for the three cases. This seemingly indicates that an increasing number of available years of simulation provides increased accuracy. Expected accuracy (made dimensionless with respect to index flood) for simulation data behaves similarly to that for observed data, this meaning that the use of peak floods coming from an accurate hydrological model to increase flood information may trade for lack of observed data, i.e. provide a similar gain in index flood estimation accuracy. Notice that, when using flood simulation for years with no available flood data, a slightly different result is observed here. This indicates a clear dependence upon the adopted samples (i.e. on the flood statistics, especially standard deviation). Here, in particular, slightly higher values of σny* are seen when considering the total simulation series (1982–2010). This is given by the increased standard deviation of flood peaks (). However, the observed behaviour is qualitatively similar, meaning that an increase of simulation length (i.e. more years) leads to a similarly small decreased standard error, i.e. to greater accuracy. The chart in may therefore be used preliminarily to provide an indication of the expected degree of accuracy obtained when using hydrological simulation for index flood assessment.
Fig. 7 Expected dimensionless accuracy of estimation of index flood σny* depending upon number of available years for estimation ny. Solid triangles indicate index flood as calculated using model simulations during 1982–2010, excluding the five years when the corresponding observations were missing. Empty triangles indicate index flood as calculated using model simulations for 1982–2010 using data from all years. Black circles indicate index flood calculated from the sample of observed discharges for 1982–2010.
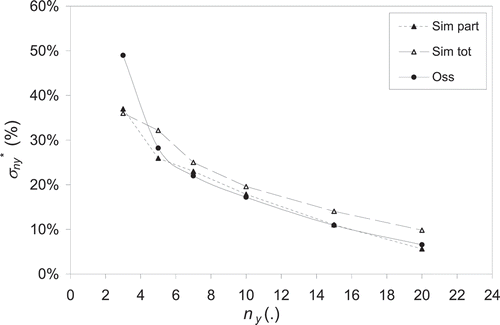
Table 2 Sample size, n, index flood, μQ (m3 s-1), standard error of estimate, σμQ (m3 s-1), and standard deviation, σ (m3 s-1) for samples of observed and simulated maximum annual discharges for the period 1982–2010, simulated maximum annual discharges for 1982–2010 excluding data when corresponding observations are missing, and all available observed maximum annual discharges (1933–2010).
The standard deviation error of index flood may be used, together with regional growth curve parameters, to assess the expected accuracy in flood quantile estimation for given return periods when using regional approaches (e.g. Bocchiola and Rosso Citation2009). Therefore, when the index flood is estimated using a hydrological model, knowledge of the expected accuracy therein is most useful for the flood design exercise.
4 SUMMARY AND CONCLUSIONS
We have presented an approach for index flood assessment of an Alpine catchment of Italy, the Toce River, by simulating discharge time series with a spatially-distributed hydrological hourly model. The model displayed good accuracy in simulating river discharge. Downscaling of precipitation and temperature from daily to hourly temporal resolution allowed us to extend the simulation period to nearly 30 years. We showed that a distributed hydrological model may be used to (a) fill AFS series displaying lack of observed data, and (b) build simulated AFS series by way of long term hydrological simulation. Indeed, risk of failure of the measuring device may be high during major floods in Alpine catchments, possibly leading to lack of fundamental data for index flood estimation. The absence of five data out of 29 years here led to more than 11% underestimation of the index flood and more than 12% underestimation of the standard error of estimate. We further demonstrated the use of an accuracy-driven approach for the choice of hydrological simulation extent that is highly necessary when dealing with flood design under a regional approach. We thus demonstrated that hydrologically based reconstruction of flood discharges is an effective tool for flood frequency estimation in ungauged or poorly gauged river catchments, where partial or total lack of flood discharge observations hampers flood design, and subsequent land-use planning.
Disclosure Statement
No potential conflict of interest was reported by the author(s).
Acknowledgements
We acknowledge ARPA Piemonte for providing temperature, precipitation and discharge data from their database. We thank the two anonymous reviewers for their comments and suggestions which contributed to improve the quality of the manuscript.
ORCID
G. Ravazzani http://orcid.org/0000-0002-6850-0883
Additional information
Funding
REFERENCES
- Allen, R.G., et al., 1998. Crop evapotranspiration—guidelines for computing crop water requirements. FAO Irrigation and Drainage Paper 56. Rome: FAO.
- Ashfaq, A. and Webster, P., 2000. The timing of runoff response in design flood analysis. Hydrological Processes, 14, 1217–1233. doi:10.1002/(SICI)1099-1085(200005)14:7<1217::AID-HYP36>3.0.CO;2-5
- Bacchi, B. and Ranzi, R., 2003. Hydrological and meteorological aspects of floods in the Alps: an overview. Hydrology and Earth System Sciences, 7 (6), 785–798. doi:10.5194/hess-7-785-2003
- Bárdossy, A., 2007. Calibration of hydrological model parameters for ungauged catchments. Hydrology and Earth System Sciences, 11, 703–710. doi:10.5194/hess-11-703-2007
- Benson, M.A., 1962. Evolution of methods for evaluating the occurrence of floods. US Geological Survey Water Supply Paper 1580-A.
- Beven, K.J., 2001. Rainfall–runoff modelling—the primer. New York: John Wiley & Sons Ltd.
- Bocchiola, D., De Michele, C., and Rosso, R., 2003. Review of recent advances in index flood estimation. Hydrology and Earth System Sciences, 7 (3), 283–296. doi:10.5194/hess-7-283-2003
- Bocchiola, D. and Rosso, R., 2006. The use of scale recursive estimation for short term quantitative precipitation forecast. Physics and Chemistry of the Earth, 31 (18), 1228–1239. doi:10.1016/j.pce.2006.03.019
- Bocchiola, D. and Rosso, R., 2009. Use of a derived distribution approach for extreme floods design: a case study in Italy. Advances in Water Resources, 32 (8), 1284–1296. doi:10.1016/j.advwatres.2009.05.005
- Bocchiola, D., et al., 2010. Flow prediction in high altitude ungauged catchments: a case study in the Italian Alps (Pantano Basin, Adamello Group). Advances in Water Resources, 33 (10), 1224–1234. doi:10.1016/j.advwatres.2010.06.009
- Bocchiola, D., et al., 2011. Prediction of future hydrological regimes in poorly gauged high altitude basins: the case study of the upper Indus, Pakistan. Hydrology and Earth System Sciences, 15, 2059–2075. doi:10.5194/hess-15-2059-2011
- Boscarello, L., et al., 2012. Integrating glaciers raster-based modelling in large catchments hydrological balance: the Rhone case study. Hydrological Processes, 28 (3), 496–508. Article first published online: 16 NOV 2012. doi:10.1002/hyp.9588
- Boughton, W.C. and Droop, O., 2003. Continuous simulation for design flood estimation—a review. Environmental Modelling & Software, 18 (4), 309–318. doi:10.1016/S1364-8152(03)00004-5
- Brath, A., De Michele, C., and Rosso, R., 1997. Combining statistical and conceptual approaches for index flood estimation. In: A. Gustard, et al., eds. Regional hydrology: concepts and models for sustainable water resource management. Wallingford, UK: International Association of Hydrological Sciences, IAHS Publ. 246, 287–295.
- Burn, D.H., 1997. Catchment similarity for regional flood frequency analysis using seasonality measures. Journal of Hydrology, 202, 212–230. doi:10.1016/S0022-1694(97)00068-1
- Buytaert, W., et al., 2008. Why can’t we do better than topmodel? Hydrological Processes, 22, 4175–4179. doi:10.1002/hyp.7125
- Cameron, D., et al., 2000. Flood frequency estimation by continuous simulation (with likelihood based uncertainty estimation). Hydrology and Earth System Sciences, 4, 23–34. doi:10.5194/hess-4-23-2000
- Castellarin, A., Burn, D.H., and Brath, A., 2001. Assessing the effectiveness of hydrological similarity measures for flood frequency analysis. Journal of Hydrology, 241, 270–285. doi:10.1016/S0022-1694(00)00383-8
- Castellarin, A., Burn, D.H., and Brath, A., 2008. Homogeneity testing: how homogeneous do heterogeneous cross-correlated regions seem? Journal of Hydrology, 360, 67–76. doi:10.1016/j.jhydrol.2008.07.014
- Castiglioni, S., et al., 2010. Calibration of rainfall–runoff models in ungauged basins: A regional maximum likelihood approach. Advances in Water Resources, 33, 1235–1242. doi:10.1016/j.advwatres.2010.04.009
- CEC, 2000 CORINE land cover. Technical guide, Commission of the European Communities, Luxembourg.
- Chalise, S.R., et al., 2003. Management of water resources and low flow estimation for the Himalayan basins of Nepal. Journal of Hydrology, 282, 25–35. doi:10.1016/S0022-1694(03)00250-6
- Corbari, C., et al., 2009. Elevation based correction of snow coverage retrieved from satellite images to improve model calibration. Hydrology and Earth System Sciences, 13 (5), 639–649. doi:10.5194/hess-13-639-2009
- Dalrymple, T., 1960. Flood frequency analysis. Reston, VA: US Geological Survey Water Supply Paper 1543-A.
- De Michele, C. and Rosso, R., 2002. A multi-level approach to flood frequency regionalization. Hydrology and Earth System Sciences, 6, 185–194. doi:10.5194/hess-6-185-2002
- Ehlschlaeger, C.R., 1989. Using the AT search algorithm to develop hydrologic models from digital elevation data. In: Proceedings of the International Geographic Information System (IGIS) Symposium, Baltimore, 275–281.
- Giampani, C., Girelli, C., and Pelosini, R., 2008. 24–26 August 1987 Flood event in Ossola valley, Quaderno no. 20, Collana Informativa Tecnico Scientifica (in Italian).
- Giannoni, F., Roth, G., and Rudari, R., 2005. A procedure for drainage network identification from geomorphology and its application to the prediction of the hydrologic response. Advances in Water Resources, 28, 567–581. doi:10.1016/j.advwatres.2004.11.013
- Groppelli, B., Bocchiola, D., and Rosso, R., 2011a. Spatial downscaling of precipitation from GCMs for climate change projections using random cascades: a case study in Italy. Water Resources Research, 47, W03519. doi:10.1029/2010WR009437.
- Groppelli, B., et al., 2011b. Evaluation of future hydrological cycle under climate change scenarios in a mesoscale Alpine watershed of Italy. Natural Hazards and Earth System Sciences, 11, 1769–1785. doi:10.5194/nhess-11-1769-2011.
- Hargreaves, G.H., 1994. Defining and using reference evapotranspiration. Journal of Irrigation and Drainage Engineering, 120 (6), 1132–1139. doi:10.1061/(ASCE)0733-9437(1994)120:6(1132)
- Konz, M., et al., 2007. Implementation of a process-based catchment model in a poorly gauged, highly glacierized Himalayan headwater. Hydrology and Earth System Sciences, 11 (4), 1323–1339. doi:10.5194/hess-11-1323-2007
- Martinec, J. and Rango, A., 1986. Parameter values for snowmelt runoff modelling. Journal of Hydrology, 84, 197–219. doi:10.1016/0022-1694(86)90123-X
- Merz, R. and Blöschl, G., 2009. A regional analysis of event runoff coefficients with respect to climate and catchment characteristics in Austria. Water Resources Research, 45, W01405. doi:10.1029/2008WR007163
- Montaldo, N., Mancini, M., and Rosso, R., 2004. Flood hydrograph attenuation induced by a reservoir system: analysis with a distributed rainfall–runoff model. Hydrological Processes, 18 (3), 545–563. doi:10.1002/hyp.1337
- Montaldo, N., Ravazzani, G., and Mancini, M., 2007. On the prediction of the Toce alpine basin floods with distributed hydrologic models. Hydrological Processes, 21, 608–621. doi:10.1002/hyp.6260
- Moretti, G. and Montanari, A., 2008. Inferring the flood frequency distribution for an ungauged basin using a spatially distributed rainfall–runoff model. Hydrology and Earth System Sciences, 12, 1141–1152. doi:10.5194/hess-12-1141-2008
- Nash, J.E. and Sutcliffe, J.V., 1970. River flow forecasting through the conceptual models, Part 1—a discussion of principles. Journal of Hydrology, 10 (3), 282–290.
- Nasri, S., et al., 2004. Use of a geomorphological transfer function to model design floods in small hillside catchments in semiarid Tunisia. Journal of Hydrology, 287, 197–213. doi:10.1016/j.jhydrol.2003.10.001
- Natural Environment Research Council (NERC), 1975. Flood studies report. Wallingford: Natural Environment Research Council.
- Parajka, J., Merz, R., and Blöschl, G., 2005. A comparison of regionalisation methods for catchment model parameters. Hydrology and Earth System Sciences, 9, 157–171. doi:10.5194/hess-9-157-2005
- Peel, M.C., Finlayson, B.L., and McMahon, T.A., 2007. Updated world map of the Köppen-Geiger climate classification. Hydrology and Earth System Sciences, 11, 1633–1644. doi:10.5194/hess-11-1633-2007
- Pianosi, F. and Ravazzani, G., 2010. Assessing rainfall–runoff models for the management of Lake Verbano. Hydrological Processes, 24 (22), 3195–3205. doi:10.1002/hyp.7745
- Ponce, V.M., 1989. Engineering hydrology, principles and practices. Englewood Cliffs, NJ: Prentice Hall, 260–261.
- Rabuffetti, D., et al., 2008. Verification of operational Quantitative Discharge Forecast (QDF) for a regional warning system—the AMPHORE case studies in the upper Po River. Natural Hazards and Earth System Sciences, 8, 161–173. doi:10.5194/nhess-8-161-2008
- Ravazzani, G., 2013. MOSAICO, a library for raster based hydrological applications. Computers & Geosciences, 51, 1–6. doi:10.1016/j.cageo.2012.08.007
- Ravazzani, G., et al., 2007. Effects of soil moisture parameterization on a real-time flood forecasting system based on rainfall thresholds, In: E. Boegh, et al., eds. Quantification and reduction of predictive uncertainty for sustainable water resources management. Wallingford: International Association of Hydrological Sciences, IAHS Publ. 313, 407–416.
- Ravazzani, G., et al., 2012. Modified Hargreaves-Samani equation for the assessment of reference evapotranspiration in Alpine river basins. Journal of Irrigation and Drainage Engineering, 138 (7), 592–599. doi:10.1061/(ASCE)IR.1943-4774.0000453.
- Ravazzani, G., Mancini, M., and Meroni, C., 2009. Design hydrograph and routing scheme for flood mapping in a dense urban area. Urban Water Journal, 6 (3), 221–231. doi:10.1080/15730620902781434
- Ravazzani, G., Rametta, D., and Mancini, M., 2011. Macroscopic cellular automata for groundwater modelling: a first approach. Environmental Modelling & Software, 26 (5), 634–643. doi:10.1016/j.envsoft.2010.11.011
- Regione Piemonte, 1997. DTM 1/10000. Torino (Italy): Servizio Cartografico (Cartographic Service).
- Rosso, R., 1994. An introduction to spatially distributed modelling of basin response. In: R. Rosso, et al., eds. Advances in distributed hydrology. Littleton, CO: Water Resources Publications, 3–30.
- Rosso, R. and Rulli, M.C., 2002. An integrated simulation method for flash-flood risk assessment. 2. Effects of changes in land use under a historical perspective. Hydrology and Earth System Sciences, 6 (2), 285–294. doi:10.5194/hess-6-285-2002
- Rulli, M.C. and Rosso, R., 2002. An integrated simulation method for flash-flood risk assessment: 1. Frequency predictions in the Bisagno river by combining stochastic and deterministic methods. Hydrology and Earth System Sciences, 6 (2), 267–284. doi:10.5194/hess-6-267-2002
- Salandin, A., et al., 2004. Il lago effimero sul ghiacciaio del Belvedere: monitoraggio e simulazione numerica del fenomeno finalizzata alla previsione e gestione dell’emergenza. Neve E Valanghe, 51, 58–65. (in Italian).
- Seibert, J. and Beven, K.J., 2009. Gauging the ungauged basin: how many discharge measurements are needed? Hydrology and Earth System Sciences, 13 (6), 883–892. doi:10.5194/hess-13-883-2009
- Simaityte, J., et al., 2008. Use of a snowmelt model for weekly flood forecast for a major reservoir in Lithuania. Annals of Glaciology, 49, 33–37. doi:10.3189/172756408787814988
- Sivapalan, M., et al., 2003. IAHS decade on Predictions in Ungauged Basins (PUB), 2003–2012: shaping an exciting future for the hydrological sciences. Hydrological Sciences Journal, 48 (6), 857–880. doi:10.1623/hysj.48.6.857.51421
- Tarboton, D.G., Chowdhury, T.G., and Jackson Thomas, H., 1994. A spatially distributed energy balance snowmelt model. Utah Water Research Laboratory.
- Thiessen, A.H., 1911. Precipitation averages for large areas. Monthly Weather Review, 39 (7), 1082–1084.
- Viviroli, D., et al., 2009. Continuous simulation for flood estimation in ungauged mesoscale catchments of Switzerland—Part II: parameter regionalisation and flood estimation results. Journal of Hydrology, 377, 208–225. doi:10.1016/j.jhydrol.2009.08.022
- Waichler, S.R. and Wigmosta, M.S., 2003. Development of hourly meteorological values from daily data and significance to hydrological modeling at H.J. Andrews experimental forest. Journal of Hydrometeorology, 4 (2), 251–263. doi:10.1175/1525-7541(2003)4<251:DOHMVF>2.0.CO;2
- Yue, S., et al., 2002. Approach for describing statistical properties of flood hydrograph. Journal of Hydrologic Engineering, 7, 147–153. doi:10.1061/(ASCE)1084-0699(2002)7:2(147)