ABSTRACT
The scientific literature has focused on uncertainty as randomness, while limited credit has been given to what we call here the “seventh facet of uncertainty”, i.e. lack of knowledge. This paper identifies three types of lack of understanding: (i) known unknowns, which are things we know we don’t know; (ii) unknown unknowns, which are things we don’t know we don’t know; and (iii) wrong assumptions, things we think we know, but we actually don’t know. Here we discuss each of these with reference to the study of the dynamics of human–water systems, which is one of the main topics of Panta Rhei, the current scientific decade of the International Association of Hydrological Sciences (IAHS), focusing on changes in hydrology and society. In the paper, we argue that interdisciplinary studies of socio-hydrological dynamics leading to a better understanding of human–water interactions can help in coping with wrong assumptions and known unknowns. Also, being aware of the existence of unknown unknowns, and their potential capability to generate surprises or black swans, suggests the need to complement top-down approaches, based on quantitative predictions of water-related hazards, with bottom-up approaches, based on societal vulnerabilities and possibilities of failure.
Editor D. Koutsoyiannis; Associate editor S. Weijs
Introduction
The number seven has been very popular and widely used for a long time: the Seven Deadly Sins, the Seven Wonders of the World, the Seven Hills of Rome, the Seven Dwarves and the much more recent Seven Little People of Murakami’s 1Q84. Mandelbrot (Citation1997) introduced the seven states of randomness, and the number seven also has some popularity among hydrological scientists dealing with uncertainty: Pappenberger and Beven (Citation2006) discussed seven reasons not to use uncertainty analysis, while Juston et al. (Citation2013) provided seven reasons to be positive about uncertainty.
Uncertainty is usually associated with the throwing of dice, which, strangely enough, have only six (and not seven) facets! A die was used, for instance, as the official logo of the 2013 Leonardo Conference in Kos, Greece, titled “Facets of Uncertainty”. We think that this is consciously (or subconsciously) due to the fact that uncertainty is often directly (or indirectly) related to the concepts of randomness and probability.
Sources of uncertainty can be classified in a variety of ways (Knight Citation1921, Ferson and Ginzburg Citation1996, Apel et al. Citation2004, Beven Citation2012). These classifications enable a better exploration of the different sources of (unreducible or reducible) uncertainty and contribute to explicitly recognizing the limitations of our analytical frameworks. It has been shown, for instance, that scientists and experts have a tendency to overconfidence, as we tend to grossly underestimate uncertainty (Cooke Citation1991, Shlyakhter et al. Citation1994) across a variety of studies, experts and questions (Lin and Bier Citation2008). Differentiating the sources of uncertainty also supports the selection of appropriate methods (e.g. probabilistic or fuzzy) to deal with uncertainty and support the decision-making process (Klir Citation2006).
In the 1920s, Frank Knight proposed a differentiation between the uncertainties that can be treated as probabilities and what he called the “true uncertainties” that cannot be quantified in probabilistic terms (Knight Citation1921). More recently, Ferson and Ginzburg (Citation1996) demonstrated the importance of distinguishing between variability and ignorance, as their proper assessment requires different methods. In this paper, we refer to a similar classification, which differentiates between aleatory and epistemic uncertainties (Apel et al. Citation2004, Beven and Smith Citation2015).
Aleatory comes from the Latin alea, which means a die or game of dice. Aleatory uncertainty is related to the random variability of processes (Koutsoyiannis Citation2010, Montanari and Koutsoyiannis Citation2012). Probabilistic methods are valuable tools for dealing with the uncertainty related to chance and randomness, which, at the current time, cannot be reduced by improving our knowledge of the systems through scientific efforts. For instance, flood risk is often estimated by referring to the expected annual flood damage (Arnell Citation1989). However, the actual damage (direct and indirect, tangible and intangible; see e.g. Giupponi et al. Citation2014, Haraguchi and Lall Citation2015) of future flood events will significantly depend on unpredictable factors, such as the exact time of the day, and day of the week, when the big, extreme flood event eventually occurs (Di Baldassarre et al. Citation2009a). For instance, the same flood event would result in significantly different damage if it occurs on Sunday night during summer or during rush hours on a Friday afternoon. While the exact time of occurrence of future flood events cannot be deterministically predicted, this intrinsic uncertainty can be assumed to be predominantly aleatory and can easily be treated in probabilistic terms.
Epistemic comes from the Greek ἐπιστήμη, which means knowledge. Sources of epistemic uncertainty are related to the lack of knowledge (Beven and Young Citation2013). In some instances, we may understand some essential processes and be able to elaborate a number of stylized facts (Kaldor Citation1957), but we do not have adequate or sufficient knowledge of all the details needed to properly capture these essential processes into our analytical frameworks.
As epistemic uncertainty is not about the game of dice, here we call it the “seventh facet of uncertainty” and discuss its role in the observation and modelling of human–water systems, which is one of the main topics of Panta Rhei, the new IAHS Scientific Decade, dealing with changes in hydrology and society (Montanari et al. Citation2013). In particular, as social dynamics and their interplay with hydrological changes are largely unknown, and surprises might play a major role, we posit that the study of human–water systems will require going beyond current approaches, whereby epistemic uncertainty is neglected or treated as if it can be represented as aleatory random variability, and with heavy reliance on quantitative predictions. Epistemic uncertainty may not be so simple: it may have complex non-stationary properties that are not easily represented by a simple probability model, and it can be rather arbitrary in its occurrences, resulting in surprises (e.g. Beven Citation2015).
Our discussion is structured by differentiating three types of lack of understanding, with reference to the study of human–water systems: (i) known unknowns, which are things we know we don’t know; (ii) unknown unknowns, which are things we don’t know we don’t know; and (iii) wrong assumptions, things we think we know, but we actually don’t know.
Human–water systems
Societies strongly rely on access to water resources, which are essential to support livelihoods and provide favourable conditions for socio-economic development. While benefiting from water services, humans also alter the hydrological regime (Koutsoyiannis et al. Citation2009, Di Baldassarre et al. Citation2010a, Vörösmarty et al. Citation2010, Wagener et al. Citation2010, Lane et al. Citation2011). Savenije et al. (Citation2014) identified four main types of human impacts on hydrology: (i) direct diversion of water flows (water supplies to cities, industries and agriculture); (ii) stream network transformation (construction of dams and reservoirs); (iii) changing river basin characteristics (deforestation, urbanization, drainage of wetlands and agricultural practices); and (iv) alteration of the regional or global climate (greenhouse gas emissions and land cover changes).
Hence, as societies change the hydrological regime, hydrological changes simultaneously shape societies. shows the coupled dynamics of hydrology and society within a river basin driven by changes in climate, economy, technology and culture at a large scale (hereafter called “regional”). For instance, by following the feedback loops of from the bottom-right, the diagram shows how economic growth can lead to changes in societies, which in turn develop new policies and implement new structures, such as irrigation canals, dams and reservoirs (depending on the available technology) to better exploit water resources and/or cope with hydrological extremes. This process often leads (together with regional climatic changes and variability) to hydrological changes that are then experienced by the same societies, which in turn respond and adapt to these changing conditions.
Figure 1. The socio-hydrological cycle. Societies change the hydrological regime via human activities (urbanization, deforestation) and interventions (e.g. dams, levees) while the experience of hydrological changes shapes societies. Human and water systems are deeply intertwined and respond to regional changes in climate, economy, technology and culture.
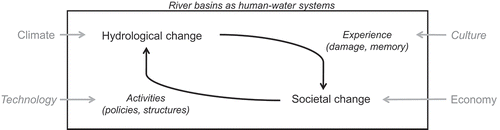
Socio-hydrological frameworks, such as the one depicted in , are being applied more and more for the interpretation of human–water interactions and feedbacks in many different fields: irrigation, agriculture, droughts and floods (Ostrom Citation2009, Sivapalan et al. Citation2012, Citation2014, Srinivasan et al. Citation2012, Montanari et al. Citation2013, Di Baldassarre et al. Citation2013a, Citation2013b, Citation2015, van Emmerik et al. 2014, Gober and Wheater Citation2015, Liu et al. Citation2015, Troy et al. Citation2015).
Recognizing and assessing uncertainty is crucial to provide useful information to decision makers (Pappenberger and Beven Citation2006, Faulkner et al. Citation2007, Montanari Citation2007, Blazkova and Beven Citation2009, Koutsoyiannis Citation2010, Brandimarte and Di Baldassarre Citation2012, Beven Citation2012, Citation2014, Krueger et al. Citation2012, Juston et al. Citation2013). To this end, a number of probabilistic methods have been developed (e.g. Beven Citation2009, Di Baldassarre et al. Citation2010b, Neal et al. Citation2013). As they are often based on an (unavoidably) incomplete collection of potential scenarios, the issue arises of what exactly is meant by probability.
Here we argue that the seventh facet of uncertainty plays a major role in the study of dynamic human–water systems. Fully coupled human–water systems are complex and nonlinear (e.g. Liu et al. Citation2007), and the dynamic interplay between hydrology and society is still poorly understood. Thus, the uncertainty affecting the interconnected dynamics of hydrology and society () is much more complex than the probabilities that e.g. gamblers estimate when playing dice or enjoying casinos. To clarify our point, shows three examples of time series produced by ludic, water and human–water systems.
Figure 2. Examples of time series of ludic (a), water (b) and human–water (c) systems. (a) Synthetic time series of flood levels, randomly generated using the mean and variance of the annual maximum water levels recorded on the Piave River at Ponte delle Alpi, Italy. (b) The actual time series of annual maximum water levels. (c) The entire time series of annual maximum water levels, which includes the surprisingly high (and essentially unpredictable) water level of 9 October 1963. From (a) to (c), the seventh facet of uncertainty plays an increasingly major role.
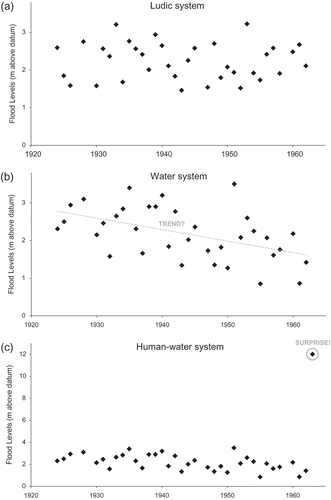
The first diagram () shows a synthetic time series of flood levels, which were randomly generated under the assumption of independent and identically distributed variables and using the mean and variance values of the annual maximum water levels recorded on the Piave River at Ponte delle Alpi (Italy). This is an example of a time series generated by a “ludic” system. Ludic comes from the Latin ludus, which means “play” or “game”. Taleb (Citation2007) introduced the term “ludic fallacy” to refer to the misuse of the narrow world of games, casinos and dice (whereby probabilities are known) to simulate the uncertainty of real-world processes.
shows the actual annual maximum water levels recorded at Ponte delle Alpi. This is an example of a time series generated by a water system. Contrary to the randomly generated time series, shows that higher flood levels were observed in the first period. This negative “trend” could be caused by long duration structure, memory and persistence that are often observed in long time series of hydrological data (Koutsoyiannis and Montanari Citation2007, Blöschl and Montanari 2010, Koutsoyiannis Citation2013). Probabilistic methods can still be used to handle this type of uncertainty, but they may require the identification of a model of the underlying stochastic structure (Montanari and Koutsoyiannis Citation2012).
Lastly, shows the entire time series of annual maximum water levels recorded at Ponte delle Alpi. This is an example of a time series generated by a human–water system. The diagram shows the same data as in , but it also includes the surprisingly high (and essentially unpredictable) flood level of 9 October 1963, which destroyed the hydrometric station.
An unrepeatable chain of events and cascade of contingencies generated this incredibly high water level. A few years before, upstream of the hydrometric station of Ponte delle Alpi, in the valley of one of the Piave’s tributaries, an artificial lake was created by the newly built Vajont Dam. The filling of the lake during one of the reservoir tests triggered a huge and fast landslide into the lake, which in turn displaced 50 million cubic metres of water. Two giant waves from the lake overtopped the dam and washed away the town of Longarone located downstream (Ward and Day Citation2011, Delle Rose Citation2012, Bianchizza and Frigerio Citation2013, Di Baldassarre et al. Citation2014). Subsequently, this immense volume of water generated a giant flood event that propagated along the Piave River and led to the surprisingly high water level of 9 October 1963, which destroyed the hydrometric station of Ponte delle Alpi (Viparelli and Merla Citation1968).
While this incredibly high flood level would not be used in traditional hydrological studies that focus on the processes of the water system (), research in dynamic human–water systems should also consider the possibility of generating surprises, such as the one depicted in . As human behaviour is difficult (if not impossible) to predict, here we posit that the seventh facet of uncertainty significantly affects the dynamics of human–water systems. Thus, there is a need to go beyond heavy reliance on quantitative predictions and probabilistic methods for uncertainty assessment.
Known unknowns
Major flooding occurred in Brisbane (Australia) in 2011. More than 10 000 properties were affected and 25 people died (Bohensky and Leitch Citation2014). The local population perceived this flood disaster as a surprise, despite the occurrence of major flooding in 1974. People were surprised because, after the 1974 event, a number of flood protection measures were implemented, including the Wivenhoe Dam. The presence of these flood protection structures “led to the popular belief that Brisbane was flood proofed” (Bohensky and Leitch Citation2014). Similarly, a few years earlier, in 2005, people in New Orleans (USA) were surprised by the catastrophic flooding caused by levee failure during the Katrina event (Kates et al. Citation2006).
New Orleans and Brisbane are only two recent examples of the so-called “levee paradox” (White Citation1945). The consequence of building (or strengthening) flood protection measures is that the non-experience of frequent flooding reduces societal memory and, as a result, more socio-economic development tends to take place in flood-prone areas. Hence, the reduced probability of flooding might generate increasing potential consequences.
This effect can also be explained by looking at the feedback loops in , starting from the implementation of human activities (levee heightening) that produces hydrological change (reduced frequency of flooding), which is experienced by societies, which then develop more in flood-prone areas.
The levee paradox is an example of a known unknown. The paradox was identified by White (Citation1945) and has been discussed by several authors (Kates et al. Citation2006, Montz and Tobin Citation2008, Di Baldassarre et al. Citation2009b, Citation2013a, Citation2013b, Castellarin et al. Citation2011, Viglione et al. Citation2014). Most flood scientists know about it. Yet methods to capture it in the assessment of future flood risk (which can be defined as a combination of flooding probability and potential adverse consequences) are completely lacking. For instance, when flood defence structures are planned and designed, current methods can indeed estimate the corresponding reduction of flooding probability. However, they do not assess how such a reduction might trigger an increase in the adverse consequences of flooding ().
Figure 3. Example of simulating flood risk changes. Current approaches can provide satisfactory results in simulating the reduction of risk immediately after the implementation of structural measures, e.g. decreased probability of flooding due to levee heightening. However, they are unable to capture future changes as they neglect the societal feedback to hydrological change. The diagram shows an example where the actual risk is much higher than the simulated one and well beyond uncertainty bounds, which were computed treating uncertainty as if aleatory. This example reflects the dynamics of risk observed in many real-world cases (e.g. Kates et al. Citation2006, Bohensky and Leitch Citation2014, Di Baldassarre et al. Citation2015), whereby the reduced probability of flooding has triggered unexpected increases of population and economic activities in flood-prone areas (levee paradox; White Citation1945).
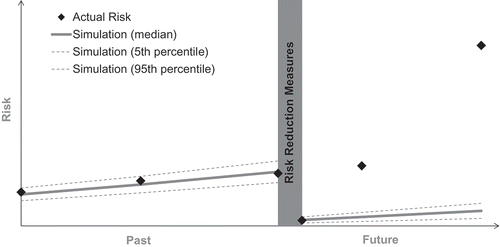
Figure 4. Human population history in the Maya Lowlands. The diagram shows demographic growth periods and collapses, plausibly due to hydrological extremes, i.e. persistent drought conditions (Gill et al. Citation2007).
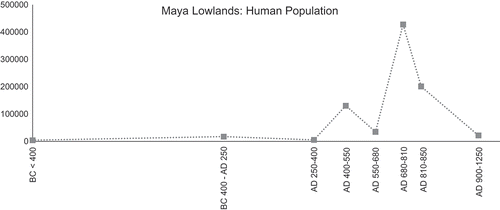
This is not a trivial aspect in assessing a realistic flood risk. There is evidence that flood risk might even increase in the long term as a result of flood protection measures (see e.g. real-world examples provided by Di Baldassarre et al. Citation2015).
Another example of a known unknown is the “adaptation effect”, which relates to the observation that the occurrence of more frequent flooding is often associated with decreasing vulnerability. This can be attributed to spontaneous learning processes and societal responses due to the experience of hydrological change, e.g. the feedback loops of . For instance, there is empirical evidence that flood damage is often lower when a flood event occurs shortly after a similar one. Wind et al. (Citation1999) showed that the losses caused by the 1995 flood on the Meuse River were much lower than those caused by a previous event, of similar magnitude, that occurred in 1993. Moreover, Mechler and Bouwer (Citation2014) recently showed similar dynamics with reference to Bangladesh, and demonstrated the limitations of current analytical frameworks in projecting future disaster risk. Similarly to the levee paradox, this adaptation effect, or learning process, is not captured by the current methods of flood risk assessment.
These effects are not mere paradoxes or exceptions. They actually shape the dynamics of many human–water systems around the world and make many state-of-the-art methods, neglecting them, unrealistic. For example, current methods consistently indicate that decreasing frequency and magnitude of floods would lead to a reduction of risk. However, the levee paradox shows that this would not necessarily be the case. Also, traditional methods consistently indicate that an increasing frequency and magnitude of floods would lead to higher flood risk. However, the adaptation effect proves that this would not necessarily be the case.
Thus, while there has been an enormous development of rigorous, formal, probabilistic methods to estimate uncertainty in flood risk assessment, we still lack fundamental understanding of how flood risk actually evolves in time. It is bizarre that we provide estimates of future flood risk with sophisticated uncertainty bounds, while neglecting crucial dynamics that might determine if flood risk will actually increase or decrease!
Moreover, many puzzles encountered in dealing with water sustainability challenges are caused by our inadequate explanatory power (e.g. water trade paradox, efficiency paradox, peak-water effect; see discussion in Sivapalan et al. Citation2014) of feedbacks between social and hydrological processes (). Sometimes, experts argue that these paradoxes are caused by irrational human behaviour, or mismanagement. But our point is that the study of human–water systems should first aim to understand and simulate how the human–water systems actually work, including spontaneous social dynamics, informalities, values and norms.
The development of new knowledge and the reduction of epistemic uncertainty typically require research efforts that can be classified in five main steps (McDonald Citation1989):
Data collection and analysis;
Examination of these data to determine “salient facts”, which still need a formal explanation;
Theory development via formulation of models capturing the salient facts;
Model calibration, validation and uncertainty analysis;
Application of models to support the decision-making process.
As discussed by McDonald (Citation1989), some scientists are deeply “engaged in work that refines or makes use of the generally accepted model” (steps 4 and 5), while others are “in the process of questioning the generally accepted model” (steps 1–3).
To better understand the behaviour of human–water systems and therefore reduce epistemic uncertainty, we think that research efforts should focus on steps 1–3, as the interplay between hydrological and social processes is still largely unknown. In particular, to develop socio-hydrological theory, there is a need of iterations between historical analyses and formal explanations (e.g. conceptual modelling) of the salient facts, such as the aforementioned levee paradox and adaptation effect.
Historical analyses of human–water interactions over long time scales, i.e. centuries, can be very challenging. For instance, shows the growth and collapse of the Maya civilization in terms of human population. Many studies have related this to hydrological conditions and shown how technological revolutions triggered population and economic growth in the Maya lowlands, whereas the persistence of drought conditions eventually led to the societal collapse (Haug et al. Citation2003, Gill et al. Citation2007). However, it has been difficult to rigorously test these causal links because the temporal resolution of human population data reconstructed from archaeological records is very coarse (), while the proxies used for dating historical droughts are affected by significant uncertainties (Aimers and Hodell Citation2011; see also Yancheva et al. Citation2007, related discussion).
Empirical studies of human–water interactions at time scales relevant for water management and disaster risk reduction, i.e. years to decades, can be much more feasible. Urbanized deltas and floodplains, for instance, are examples of ideal laboratories for the (inter- or trans-disciplinary) study of the interplay between social and hydrological processes as the interactions between human and water systems are apparent and have relevant impacts (Di Baldassarre et al. Citation2013a). In these flood-prone areas, human settlements, flood control measures, and memory of flooding have gradually co-evolved at similar temporal (years to decades) and spatial (floodplain) scales, while they have been also significantly affected by the sudden and localized occurrence of flooding events.
Long time series of demographic, economic and hydrological data along with information about human adjustments to floods are already available for many case studies, such as New Orleans, the Tiber in Rome, and the Dutch delta (Werner and McNamara Citation2007, Aldrete Citation2007, De Moel et al. Citation2011). Also, social science literature on water-related disasters (e.g. Ewen Citation2014) can give useful information about human–water interactions.
Yet, to better understand the dynamics emerging from the (poorly explored) interactions and feedbacks between human and water systems, there is a need to start collecting more empirical evidence. For instance, while the levee paradox had already been identified by White (Citation1945) and been widely discussed in the literature, systematic datasets about its magnitude in real-world case studies are still missing. Conceptual models, i.e. hypotheses of how socio-hydrological systems work (e.g. Di Baldassarre et al. Citation2013b, Viglione et al. Citation2014), can provide insights about the type of data that we need to collect to observe these feedback mechanisms between physical and social processes.
Hence, empirical studies and hypothesis formulation and testing via conceptual models (steps 1–3) can significantly contribute to a better understanding of the dynamics of human–water systems, and therefore reduce this type of epistemic uncertainty.
Unknown unknowns
The study of human–water systems requires an explicit treatment of the interplay between social physical processes. In this context, besides the aforementioned paradoxes that urge more understanding, there are many other things we don’t even know we don’t know (as stated by Donald Rumsfeld in February 2002). Some of these unknown unknowns may occasionally result in the so-called “black swans”: unexpected events with an extremely high impact on the system, which are essentially impossible to forecast. They are one form of epistemic uncertainty, which is unlikely to be captured in any error distribution derived from an analysis of past data. Yet, after their occurrence we will usually attempt to rationalize and explain them (Taleb Citation2013). These unexpected events are typically created by unique, unrepeatable combinations of contexts and cascades of contingencies, such as the surprisingly high water level at Ponte delle Alpi (). However, after they occur, humans tend to overreact and overestimate their probability, potentially leading to some unintended consequences.
While, as discussed above, more interdisciplinary research exploring the dynamics of human–water systems can help reduce the epistemic uncertainty related to known unknowns, there is nothing we can do about unknown unknowns as we don’t even know what we don’t know. However, being aware of their potential occurrence is crucial in supporting the process of water management and disaster risk reduction. In this sense, black swans remind us about the importance of reducing the negative impacts of unexpected events, rather than focusing only on the precise (but most likely inaccurate) estimation of their close-to-zero probability (Makridakis and Taleb Citation2009a, Citation2009b). Decreasing the potential adverse consequences of water-related disasters by enhancing the resilience (and reducing the vulnerability) of human societies can be more robust than relying heavily on predictions of the close-to-zero (but essentially unknown) probability of water-related disasters caused by unrepeatable combinations of contexts and cascades of contingencies. For instance, improving evacuation and contingency plans does not necessarily require an accurate and precise estimation of probabilities, but it can significantly increase the resilience of human–water systems, i.e. the ability to recover after an event.
Thus, potential surprises and the impact of epistemic uncertainties suggest the need to go beyond heavy reliance on quantitative predictions and traditional top-down approaches, based on probabilistic assessments of water-related hazards and associated uncertainty. In this context, Blöschl et al. (Citation2013) comprehensively discussed the potentialities of complementing traditional top-down approaches with bottom-up ones. Bottom-up approaches do not start from probabilistic predictions, but rather from the societal and economic vulnerability of communities and individuals, and they explore the possibilities (rather than the probabilities) of failure by explicitly considering the expertise of local stakeholders and risk managers (see e.g. Lane et al. Citation2011). For instance, Wilby and Dessai (Citation2010) showed how the vulnerability of human–water systems to droughts and water scarcity can be reduced by enhancing the connectivity of water supply infrastructures and making abstraction licences time-limited. While this combination of measures could not be considered “optimal”, it is more robust than alternative low-regret options to the potential occurrence of unexpected events with potentially devastating consequences. Thus, it is by acting from a vulnerability’s viewpoint that water managers and scientists can reduce the negative impacts of unknown unknowns and potential surprises.
Wrong assumptions
Besides unknowns, there are also things we think we know, but we actually don’t know. This is what we call here “wrong assumptions” (also sometimes called “ontological uncertainties”). Any scientific work, including hydrological or socio-hydrological studies, is necessarily based on a number of assumptions. Aware of the limitations of our hypotheses, we typically follow a parsimonious approach and focus on the dominant processes that drive the dynamics. However, while some assumptions may work for a number of case studies, they can be significantly wrong in other cases, including assumptions about the nature of the uncertainties involved.
To make this point, we introduce two fictitious characters who are inspired by the ludic fallacy (Taleb Citation2007): Dr Maria Smith, graduated summa cum laude at MIT, the youngest professor at Princeton University. She is a “logical positivist”, who believes in the power of deductive reasoning based on fundamental assumptions. “Smart” Angie, perhaps graduated somehow/somewhere, is a great entrepreneur who became very rich in a few years. She is, on the other hand, a “sceptical empiricist”, who puts more faith in putting the available evidence to robust testing.
A test is made. A coin is flipped 99 times, and each time it comes up heads. The two observers are asked what the odds are that the 100th flip would also come up heads.
Without any hesitation, Dr Smith says: “Odds are not affected by previous outcomes, so the odds must be 50%!”
Smart Angie thinks about it and eventually says: “Well, if it came up heads 99 times in a row there must be something wrong with this coin! So, odds must be much more than 50%!”
The point made by Smart Angie is that the coin must be loaded. In classical terms, odds of the coin coming up heads 99 times in a row are so low that the assumption that the coin had a 50% chance of coming up heads is most likely wrong.
Similarly, the occurrence of many 1-in-100 year flood events within a few years may suggest issues with the typical assumption of treating annual maximum flows as time series of independent and identically distributed random variables. Hydrological extremes can be affected by long-term persistence and memory related to climate variability. Blöschl and Montanari (Citation2010) as well as Hall et al. (Citation2013) showed examples of occurrence of flood-rich and flood-poor periods (see also e.g. ).
This is an example of the many hypotheses that have become standard and have not been sufficiently challenged (similarly to the way Dr Smith keeps assuming the coin to be fair). While the assumption of treating hydrological extremes as independent and identically distributed can be deemed acceptable (from a practical viewpoint) in a few instances, this assumption should be tested and not merely be taken for granted. For another hydrological example see the discussion of preferential flows in relation to the transport of phosphorus and pesticides in Beven and Germann (Citation2013).
When it comes to the nonlinear dynamics of human–water systems, the issue of making fundamentally wrong assumptions becomes even larger because we lack fundamental understanding about how different social groups respond to hydrological changes, as attitudes to risk can strongly vary across societies depending on political and socio-economic conditions as well as cultural values (Kahneman and Tversky Citation1979, Thompson et al. Citation1990, Viglione et al. Citation2014). And given wrong assumptions, of course, we should expect surprises in the future (as seen also in other disciplines, such as economics).
It should be noted that some wrong assumptions could be related to known unknowns. For instance, while historical changes show that the dynamics of future flood risk can be significantly affected by socio-hydrological feedback loops, these feedbacks are, as discussed above, often neglected in state-of-art assessments of future flood risk (). Moreover, unknown unknowns can also cause some other wrong assumptions as we (might) become aware of their fallacy only after the occurrence of surprises or black swans. So, the three categories used in this paper can potentially overlap.
Conclusions
Despite centuries of water management, we still lack fundamental knowledge of the essential dynamics driving the long-term behaviour of human–water systems. Over the past decades, the focus has been on assessing in probabilistic terms the uncertainty of the assumed behaviour (how it should work) of the system, rather than exploring ranges of the actual behaviour of the system (how it actually works). This has been related to the focus on uncertainty as randomness along with limited credit to epistemic uncertainty, which here we called the seventh facet of uncertainty.
The increasing impact of human activities on hydrological dynamics, in a time that some call the Anthropocene, has led to a growing interest in the study of water–society interactions (). As the dynamics of human–water systems are still largely unknown, and social dynamics are highly unpredictable, we argued that there is a need to go beyond current approaches (focusing on uncertainty as randomness and probabilistic methods) and give more credit to the seventh facet of uncertainty.
To increase our knowledge of the behaviour of fully coupled human–water systems and reduce epistemic uncertainty, we proposed an iteration of new observations, empirical studies and conceptual modelling (for formulating and testing hypotheses) of coupled dynamics of hydrology and societies. Hence, scientific understanding is believed to be a way to deal with wrong assumptions and known unknowns in the study of the interplay between social and hydrological processes, which is one of the main focuses of Panta Rhei, the IAHS’s current scientific decade.
We also discussed that, while unknown unknowns cannot be understood as we don’t even know what we don’t know, being aware of their existence, and their potential capability to generate surprises or black swans, suggests the need to complement top-down approaches, based on quantitative predictions of water-related hazards, with bottom-up approaches, based on societal vulnerabilities and possibilities of failures.
Acknowledgements
We would like to acknowledge the guest editor Dr Steven Wejis and two anonymous reviewers for providing constructive comments on our paper. The present work was developed within the framework of the Panta Rhei Research Initiative of the International Association of Hydrological Sciences (IAHS).
Disclosure statement
No potential conflict of interest was reported by the authors.
References
- Aimers, J. and Hodell, D., 2011. Societal collapse: drought and the Maya. Nature, 479 (7371), 44–45. doi:10.1038/479044a
- Aldrete, G.S., 2007. Floods of the Tiber in ancient Rome. Baltimore: John Hopkins University, 368 p.
- Apel, H., et al., 2004. Flood risk assessment and associated uncertainty. Nature Hazards Earth Systems Sciences, 4, 295–308. doi:10.5194/nhess-4-295-2004
- Arnell, N., 1989. Expected annual damages and uncertainties in flood frequency estimation. Journal of Water Resources Planning and Management, 115 (1), 94–107. doi:10.1061/(ASCE)0733-9496(1989)115:1(94)
- Beven, K.J., 2009. Environmental modelling – an uncertain future? London: Routledge, 294 p.
- Beven, K.J., 2012. Causal models as multiple working hypotheses about environmental processes. Comptes Rendus Geoscience, Académie de Sciences, Paris, 344, 77–88. doi:10.1016/j.crte.2012.01.005
- Beven, K.J., 2014. What we see now: event-persistence in predicting the responses of hydro-eco-geomorphological systems? Ecological Modelling. doi:10.1016/j.ecolmodel.2014.07.019
- Beven, K.J., 2015. EGU Leonardo lecture: facets of hydrology - epistemic error, non-stationarity, likelihood, hypothesis testing, and communication. Hydrological Sciences Journal. doi:10.1080/02626667.2015.1031761
- Beven, K.J. and Germann, P.F., 2013. Macropores and water flow in soils revisited. Water Resources Researcher, 49 (6), 3071–3092. doi:10.1002/wrcr.20156
- Beven, K.J. and Smith, P., 2015. Concepts of information content and likelihood in parameter calibration for hydrological simulation models. Journal of Hydrologic Engineering, 20, A4014010. doi:10.1061/(ASCE)HE.1943-5584.0000991
- Beven, K.J. and Young, P., 2013. A guide to good practice in modeling semantics for authors and referees. Water Resources Research, 49 (8), 5092–5098. doi:10.1002/wrcr.20393
- Bianchizza, C. and Frigerio, S., 2013. Domination of or adaptation to nature? A lesson we can still learn from the Vajont. Ital Journal of Engineering Geological Environment, 6, 523–531.
- Blazkova, S. and Beven, K., 2009. A limits of acceptability approach to model evaluation and uncertainty estimation in flood frequency estimation by continuous simulation: Skalka catchment, Czech Republic. Water Resources Researcher, 45, W00B16. doi:10.1029/2007WR006726
- Blöschl, G. and Montanari, A., 2010. Climate change impacts—throwing the dice? Hydrological Processes, 24 (3), 374–381.doi:10.1002/hyp.7574
- Blöschl, G., Viglione, A., and Montanari, A., 2013. Emerging approaches to hydrological risk management in a changing world. In: F. Hossain, ed. Climate Vulnerability. Waltham, MA: Elsevier Inc., Academic Press, 3–10.
- Bohensky, E.L. and Leitch, A.M., 2014. Framing the flood: a media analysis of themes of resilience in the 2011 Brisbane flood. Regional Environment Change, 14, 475–488. doi:10.1007/s10113-013-0438-2
- Brandimarte, L. and Di Baldassarre, G., 2012. Uncertainty in design flood profiles derived by hydraulic modelling. Hydrology Research, 43 (6), 753–761. doi:10.2166/nh.2011.086
- Castellarin, A., Di Baldassarre, G., and Brath, A., 2011. Floodplain management strategies for flood attenuation in the river Po. River Research and Applications, 27 (8), 1037–1047. doi:10.1002/rra.v27.8
- Cooke, R.M., 1991. Experts in uncertainty: opinion and subjective probability in science. New York: Oxford University Press, 366 p.
- de Moel, H., Aerts, J.C.J.H., and Koomen, E., 2011. Development of flood exposure in the Netherlands during the 20th and 21st century. Global Environmental Change, 21 (2), 620–627. doi:10.1016/j.gloenvcha.2010.12.005
- Delle Rose, M., 2012. Decision-making errors and sociopolitical disputes over the Vajont Dam disaster. Disaster Advancement, 5, 144–152.
- Di Baldassarre, G., et al., 2009a. Probability weighted hazard maps for comparing different flood risk management strategies: a case study. Natural Hazards, 50 (3), 479–496. doi:10.1007/s11069-009-9355-6
- Di Baldassarre, G., Castellarin, A., and Brath, A., 2009b. Analysis of the effects of levee heightening on flood propagation: example of the River Po, Italy. Hydrological Sciences Journal, 54 (6), 1007–1017. doi:10.1623/hysj.54.6.1007
- Di Baldassarre, G., et al., 2010a. Flood fatalities in Africa: from diagnosis to mitigation. Geophysical Research Letters, 37 (L22402). doi:10.1029/2010GL045467
- Di Baldassarre, G., et al., 2010b. Floodplain mapping: a critical discussion on deterministic and probabilistic approaches. Hydrological Sciences Journal, 55 (3), 364–376. doi:10.1080/02626661003683389
- Di Baldassarre, G., et al., 2013a. Towards understanding the dynamic behaviour of floodplains as human-water systems. Hydrology and Earth System Sciences, 17, 3235–3244. doi:10.5194/hess-17-3235-2013
- Di Baldassarre, G., et al., 2013b. Socio-hydrology: conceptualising human-flood interactions. Hydrology and Earth System Sciences, 17, 3295–3303. doi:10.5194/hess-17-3295-2013
- Di Baldassarre, G., et al., 2014. Floods and societies: the spatial distribution of water-related disaster risk and its dynamics. WIREs Water, 1, 133–139. doi:10.1002/wat2.1015
- Di Baldassarre, G., et al., 2015. Debates—perspectives on socio-hydrology: capturing feedbacks between physical and social processes. Water Resources Researcher, 51. doi:10.1002/2014WR016416
- Ewen, S., 2014. Sheffield’s great flood of 1864: engineering failure and the municipalisation of water. Environment and History, 20 (2), 177–207. doi:10.3197/096734014X13941952680954
- Faulkner, H., et al., 2007. Developing a translational discourse to communicate uncertainty in flood risk between science and the practitioner. AMBIO: A Journal of the Human Environment, 36 (8), 692–704. doi:10.1579/0044-7447(2007)36[692:DATDTC]2.0.CO;2
- Ferson, S. and Ginzburg, L.R., 1996. Different methods are needed to propagate ignorance and variability. Reliability Engineering & System Safety, 54, 133–144. doi:10.1016/S0951-8320(96)00071-3
- Gill, R.B., et al., 2007. Drought and the Maya collapse. Ancient Mesoamerica, 18 (02), 283–302. doi:10.1017/S0956536107000193
- Giupponi, C., et al., 2014. Integrated risk assessment of water related disasters. In: P. Paronand and G. Di Baldassarre, eds. Hydro-meteorological hazards, risk and disasters. Amsterdam, The Netherlands: Elsevier, 164–197.
- Gober, P. and Wheater, H.S., 2015. Debates—perspectives on socio-hydrology: modeling flood risk as a public policy problem. Water Resources Researcher, 51. doi:10.1002/2015WR016945
- Hall, J., Arheimer, B., and Borga, M., et al., 2013. Understanding flood regime changes in Europe: a state of the art assessment. Hydrology and Earth System Sciences Discussions, 10, 15525–15624. doi:10.5194/hessd-10-15525-2013
- Haraguchi, M. and Lall, U., 2015. Flood risks and impacts: a case study of Thailand’s floods in 2011 and research questions for supply chain decision making. International Journal of Disaster Risk Reduction. doi:10.1016/j.ijdrr.2014.09.005
- Haug, G.H., et al., 2003. Climate and the collapse of Maya civilization. Science, 299 (5613), 1731–1735. doi:10.1126/science.1080444
- Juston, J.M., et al., 2013. Smiling in the rain: seven reasons to be positive about uncertainty in hydrological modelling. Hydrological Processes, 27, 1117–1122. doi:10.1002/hyp.v27.7
- Kahneman, D. and Tversky, A., 1979. Prospect theory: an analysis of decision under risk. Econometrica, 47, 263–291. doi:10.2307/1914185
- Kaldor, N., 1957. A model of economic growth. The Economic Journal, 67 (268), 591–624. doi:10.2307/2227704
- Kates, R.W., et al., 2006. Reconstruction of New Orleans after Hurricane Katrina: a research perspective. Proceedings of the National Academy of Sciences, 103, 14653–14660. doi:10.1073/pnas.0605726103
- Klir, G., 2006. Uncertainty and Information. Chichester: Wiley.
- Knight, F.H., 1921. Risk, uncertainty and profit. New York: Houghton-Mifflin Co. (reprinted University of Chicago Press, 1971).
- Koutsoyiannis, D., 2010. HESS opinions: “A random walk on water”. Hydrology and Earth System Sciences, 14 (3), 585–601. doi:10.5194/hess-14-585-2010
- Koutsoyiannis, D., 2013. Hydrology and change. Hydrological Sciences Journal, 58 (6), 1177–1197. doi:10.1080/02626667.2013.804626
- Koutsoyiannis, D., et al., 2009. HESS opinions: “climate, hydrology, energy, water: recognizing uncertainty and seeking sustainability”. Hydrology and Earth System Sciences, 13, 247–257. doi:10.5194/hess-13-247-2009
- Koutsoyiannis, D. and Montanari, A., 2007. Statistical analysis of hydroclimatic time series: uncertainty and insights. Water Resources Research, 43 (5), W05429. doi:10.1029/2006WR005592
- Krueger, T., et al., 2012. The role of expert opinion in environmental modelling. Environmental Modelling & Software, 36, 4–18. doi:10.1016/j.envsoft.2012.01.011
- Lane, S.N., et al., 2011. Doing flood risk science differently: an experiment in radical scientific method. Transactions Institute British Geographic, 36, 15–26. doi:10.1111/j.1475-5661.2010.00410.x
- Lin, S.W. and Bier, V.M., 2008. A study of expert overconfidence. Reliability Engineering & System Safety, 5 (93), 711–721. doi:10.1016/j.ress.2007.03.014
- Liu, J., et al., 2007. Complexity of coupled human and natural systems. Science, 317 (5844), 1513–1516. doi:10.1126/science.1144004
- Liu, D., et al., 2015. A conceptual socio-hydrological model of the co-evolution of humans and water: case study of the Tarim River basin, western China. Hydrological Earth Systems Sciences, 19, 1035–1054. doi:10.5194/hess-19-1035-2015
- Makridakis, S. and Taleb, N., 2009a. Living in a world of low levels of predictability. International Journal of Forecasting, 25, 840–844. doi:10.1016/j.ijforecast.2009.05.008
- Makridakis, S. and Taleb, N., 2009b. Decision making and planning under low levels of predictability. International Journal of Forecasting, 25 (4), 716–733. doi:10.1016/j.ijforecast.2009.05.013
- Mandelbrot, B., 1997. Fractals and scaling in finance. London: Springer, 548 p.
- McDonald, J.F., 1989. Econometric studies of urban population density: a survey. Journal of Urban Economics, 26 (3), 361–385. doi:10.1016/0094-1190(89)90009-0
- Mechler, R. and Bouwer, L.M., 2014. Understanding trends and projections of disaster losses and climate change: is vulnerability the missing link? Climatic Change. doi:10/1009/s10584-014-1141-0
- Montanari, A., 2007. What do we mean by ‘uncertainty’? The need for a consistent wording about uncertainty assessment in hydrology. Hydrological Processes, 21 (6), 841–845. doi:10.1002/(ISSN)1099-1085
- Montanari, A., et al., 2013. “Panta Rhei - everything flows”: change in hydrology and society - The IAHS Scientific Decade 2013–2022. Hydrological Sciences Journal, 58 (6), 1256–1275. doi:10.1080/02626667.2013.809088
- Montanari, A. and Koutsoyiannis, D., 2012. A blueprint for process-based modeling of uncertain hydrological systems. Water Resources Research, 48, W09555. doi:10.1029/2011WR011412
- Montz, B.E. and Tobin, G.A., 2008. Livin’ large with levees: lessons learned and lost. Natural Hazards Reviews, 9, 150–157. doi:10.1061/(ASCE)1527-6988(2008)9:3(150)
- Neal, J., et al., 2013. Probabilistic flood risk mapping including spatial dependence. Hydrological Processes, 27 (9), 1349–1363. doi:10.1002/hyp.v27.9
- Ostrom, E., 2009. A general framework for analyzing sustainability of social-ecological systems. Science, 325, 419–422. doi:10.1126/science.1172133
- Pappenberger, F. and Beven, K.J., 2006. Ignorance is bliss: or seven reasons not to use uncertainty analysis. Water Resources Research, 42 (5), W05302. doi:10.1029/2005WR004820
- Savenije, H.H.G., Hoekstra, A.Y., and van der Zaag, P., 2014. Evolving water science in the Anthropocene. Hydrology and Earth System Sciences, 18, 319–332. doi:10.5194/hess-18-319-2014
- Shlyakhter, A.I., et al., 1994. Quantifying the credibility of energy projections from trends in past data—the United States energy sector. Energy Policy, 22 (2), 119–130. doi:10.1016/0301-4215(94)90129-5
- Sivapalan, M., et al., 2014. Socio-hydrology: use-inspired water sustainability science for the Anthropocene. Earth’s Future, 2. doi:10.1002/2013EF000164
- Sivapalan, M., Savanjie, H.H.G., and Blöschl, G., 2012. Socio-hydrology: a new science of people and water. Hydrological Processes, 26, 1270–1276. doi:10.1002/hyp.8426
- Srinivasan, V., Gorelick, L., and Thompson, R., 2012. The nature and causes of the global water crisis: syndromes from a meta-analysis of coupled human-water studies. Water Resources Research, 48, W10516. doi:10.1029/2011WR011087
- Taleb, N.N., 2007. The black swan: the impact of the highly improbable. London: Penguin, 444 p.
- Taleb, N.N., 2013. Antifragile: things that gain from disorder. London: Penguin, 520 P.
- Thompson, M., Ellis, R., and Wildavsky, A., 1990. Cultural theory. Boulder, CO: Westview Press, 296 p.
- Troy, T.J., Pavao-Zuckerman, M., and Evans, T.P., 2015. Debates—perspectives on socio-hydrology: socio-hydrologic modeling: tradeoffs, hypothesis testing, and validation. Water Resources Researcher, 51. doi:10.1002/2015WR017046
- van Emmerik, T.H.M., et al., 2014. Socio–hydrologic modeling to understand and mediate the competition for water between agriculture development and environmental health: Murrumbidgee River basin, Australia. Hydrology and Earth System Sciences, 18, 4239–4259. doi:10.10.5194/hess-18-4239-2014
- Viglione, A., et al., 2014. Insights from socio-hydrology modelling on dealing with flood risk–roles of collective memory, risk-taking attitude and trust. Journal of Hydrology, 518, 71–82.in press. doi:10.1016/j.jhydrol.2014.01.018
- Viparelli, M. and Merla, G., 1968. L’onda di piena seguita alla frana del Vajont. Atti dell’Accademia Pontaniana (in Italian). Italy: Napoli, Vol. 17, p 229.
- Vörösmarty, C.J., et al., 2010. Global threats to human water security and river biodiversity. Nature, 467, 555–561. doi:10.1038/nature09440
- Wagener, T., et al., 2010. The future of hydrology: an evolving science for a changing world. Water Resources Research, 46, W05301. doi:10.1029/2009WR008906
- Ward, S.N. and Day, S., 2011. The 1963 landslide and flood at Vajont reservoir Italy: a tsunami ball simulation. Ital Journal of Geosciences, 130, 16–26.
- Werner, B.T. and McNamara, D.E., 2007. Dynamics of coupled humanlandscape systems. Geomorphology, 91, 393–407. doi:10.1016/j.geomorph.2007.04.020
- White, G.F., 1945. Human adjustments to floods. Department of Geography Research, Paper no. 29, The University of Chicago, Chicago.
- Wilby, R.L. and Dessai, S., 2010. Robust adaptation to climate change. Weather, 65, 180–185. doi:10.1002/wea.v65:7
- Wind, H.G., et al., 1999. Analysis of flood damages from the 1993 and 1995 Meuse Floods. Water Resources Researcher, 35 (11), 3459–3465. doi:10.1029/1999wr900192
- Yancheva, G., Nowaczyk, N.R., and Mingram, J., et al., 2007. Influence of the intertropical convergence zone on the East Asian monsoon. Nature, 445 (7123), 74–77. doi:10.1038/nature05431