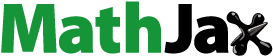
ABSTRACT
Storm runoff from the Marikina River Basin frequently causes flood events in the Philippine capital region Metro Manila. This paper presents and evaluates a system to predict short-term runoff from the upper part of that basin (380 km2). It was designed as a possible component of an operational warning system yet to be installed. For the purpose of forecast verification, hindcasts of streamflow were generated for a period of 15 months with a time-continuous, conceptual hydrological model. The latter was fed with real-time observations of rainfall. Both ground observations and weather radar data were tested as rainfall forcings. The radar-based precipitation estimates clearly outperformed the raingauge-based estimates in the hydrological verification. Nevertheless, the quality of the deterministic short-term runoff forecasts was found to be limited. For the radar-based predictions, the reduction of variance for lead times of 1, 2 and 3 hours was 0.61, 0.62 and 0.54, respectively, with reference to a “no-forecast” scenario, i.e. persistence. The probability of detection for major increases in streamflow was typically less than 0.5. Given the significance of flood events in the Marikina Basin, more effort needs to be put into the reduction of forecast errors and the quantification of remaining uncertainties.
Editor Z.W. Kundzewicz; Associate editor G. Di Baldassarre
1 Introduction
Runoff forecasting in small basins is a challenge for a number of factors. Compared to large basins, runoff predictions in small catchments are generally more sensitive to external forcing, i.e. rainfall. This is due to short drainage paths and limited storage capacities, resulting in short response times. At the same time, hydrological predictions are more vulnerable to spatially inaccurate estimates/forecasts of rainfall. While such errors may balance out at larger scales, this is not the case in small basins (Arnaud et al. Citation2011, Vieux and Imgarten Citation2012). Finally, the probability of extreme areal precipitation generally increases as the considered area becomes smaller (see, e.g. Rodriguez-Iturbe and Mejía Citation1974, Frederick et al. Citation1977).
Runoff prediction involves two major aspects: (1) estimation/forecasting of precipitation; and (2) modelling of the basin’s hydrological response. The hydrological models employed may be either empirical or process-based in nature. While the first class of models (see overview in Solomatine and Ostfeld Citation2008) is easy to calibrate, simple to use, and computationally efficient, empirical models are criticized for their black-box character. Outputs may become unrealistic and even unphysical in extrapolation mode, i.e. when the model is confronted with input signals not contained in its training dataset. This is a particular problem for the prediction of rare extremes, e.g. floods (see Schmitz and Cullmann Citation2008).
In this paper, the focus is on the second class of models built on the mathematical representation of hydrological processes. These models combine the principle of mass conservation with more or less simplified versions of the energy balance equation. Process-based models are commonly used for the purpose of hydrological forecasting (Kitanidis and Bras Citation1980, De Roo et al. Citation2000, Plate Citation2009). Of special interest are spatially distributed models that discretize river basins into smaller sub-units, e.g. sub-basins. This is a prerequisite for taking advantage of high-resolution precipitation fields as produced, for example, by radar-based quantitative precipitation estimation (QPE). Nonetheless, discretization is necessary to represent spatial heterogeneities in terms of states and parameters and, thereby, it is a key to the proper simulation of the dynamic nonlinearities (Robinson et al. Citation1995) occurring in rainfall–runoff transformation.
The kind of precipitation data required for runoff forecasting depends on both the intended maximum lead time (LTmax) as well as the basin’s response time (RT). As long as LTmax ≤ RT, real-time QPE data (i.e. recent observations) are sufficient. If LTmax > RT, the hydrological model must also be fed with a quantitative precipitation forecast (QPF). In cases where LTmax RT, the QPF is typically provided by numerical weather prediction models (e.g. Kneis et al. Citation2012). If LTmax exceeds RT by only minutes to a few hours (at most) the required short-term QPF, also referred to as a nowcast, often originates from extrapolation methods (Toth et al. Citation2000, Pierce et al. Citation2004, Ehret et al. Citation2008). Hybrid approaches to short-term QPF that combine dynamic and statistic rainfall forecasts (e.g. Golding Citation1998) are also in use (Werner and Cranston Citation2009).
This paper demonstrates the performance of a short-term hydrological forecast system for a small basin exploiting the limited information that is typically available in a newly industrialized country. The case study was motivated by the remarkable frequency and magnitude of storm events hitting the Philippines in recent years. The basin of interest (380 km2, RT ≈ 3 h) is located just upstream of the eastern part of Metro Manila. It has been selected for its high-risk status, i.e. the exceptional loss potential in combination with the frequent occurrence of extreme runoff events.
Hourly deterministic forecasts were generated for two rainy seasons using a process-based hydrological model in conjunction with pragmatic but robust approaches to streamflow data assimilation. The model was forced with QPE data only, i.e. no precipitation forecast was used. Therefore, the analysis is limited to LTmax ≈ RT. Since weather radar stations were recently installed in the Philippines, the possible benefit of radar-based precipitation estimates over a conventional, raingauge-based QPE was of particular interest. The specific value of this study lies in the fact that the alternative QPEs are not just evaluated with respect to the quality of hydrological simulations but with respect to forecasts where deficits in QPE are potentially alleviated through data assimilation.
The major aim of this case study was to evaluate the quality of the hydrological forecasts for the purpose of flood warning and to provide a roadmap for further improvement of the forecast system. Ideally, the study would serve as a precursor to the establishment and verification of runoff forecasting systems for other high-risk basins across the Philippines in the context of the Nationwide Operational Assessment of Hazards (http://noah.dost.gov.ph). It aims especially at fostering the use of data from the country’s recently installed network of weather radar stations. Last but not least, the study demonstrates the productive use of open-source software for radar data processing (Heistermann et al. Citation2015) and hydrological modelling (Kneis Citation2015), enhancing transparency and reproducibility of the methodological approach.
The terms simulation, prediction, forecast and hindcast appear several times in the subsequent text. These words are used according to the following convention: (1) Simulation refers to the situation where the hydrological model is fed with observed meteorological forcings to estimate streamflow of the past. (2) The terms forecast and prediction are used synonymously. They refer to the case where the model is used to estimate future streamflow. In an operational context, the model must then be fed with predicted meteorological forcings. (3) The term hindcast denotes a retrospective forecast experiment, carried out with the intention of comparing the predicted outcome of an event to the actual (known) outcome. This is the usual approach to forecast verification.
2 Study area
The study area () comprises the Marikina River Basin (MRB) up to the gauging station at Montalban (380 km2). The Marikina River and its tributaries originate in the mountain range northeast of the Philippine capital Manila. While the upper parts of the basin are covered by forests, the lower parts are dominated by agricultural use. Urban areas are located near the Montalban gauging station and further downstream.
Figure 1. Upper Marikina Basin with topography (ASTER elevation model), drainage network and sub-basin boundaries. River and raingauges are marked by triangle and squares, respectively. Dashed curves indicate the distance to the radar station at Subic (). Coordinates are in WGS84
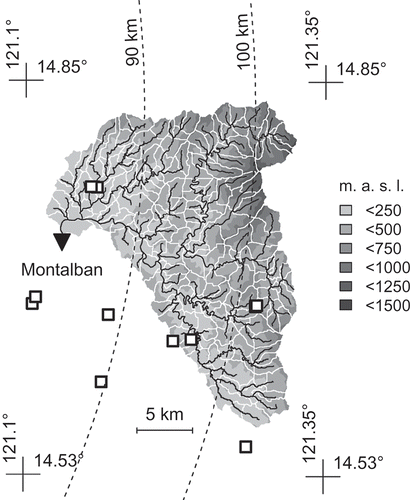
As typical for this part of the Philippines, the climate of MRB is characterized by wet conditions from May to October and a dry season lasting from November to April. During longer periods of drought, streamflow drops to near zero values. Average annual precipitation amounts to 2400 mm. Flood-inducing storm events are often related to typhoons. Strong activity of the southwest monsoon and convective rainfall in the Inter-Tropical Convergence Zone (ITCZ) are relevant as well. Peak discharges of the 10 largest floods for 1959–2000 were reported in Abon et al. (Citation2011), ranging from about 1600 to 2600 m3 s−1 with reference to a catchment area of 540 km2. Severe floods were observed in September 2009 (Abon et al. Citation2011), August 2012 (Heistermann et al. Citation2013a), August 2013 and more recently in September 2014.
Several streamgauges exist in the MRB but most of them do not provide continuous observations of adequate temporal resolution or the water levels are affected by backwater. Hence, only data from the Montalban gauge () were used in this study. A typical lag between onset of rainfall in the upstream catchment and rising stage at this gauge is 3 hours.
3 Data and methods
3.1 Radar-based precipitation estimates
The Subic radar station is located about 90 km west of the study area () at 14.822°N, 120.363°E. The station has been operational since March 2012. Its technical features are summarized in .
Table 1. Features of the weather radar station near Subic
The Subic radar data were processed using routines from the open-source software library wradlib (Heistermann et al. Citation2013b). In the first step, a static clutter map was generated by identifying bins with reflectivity persistently greater than 15 dBZ in spite of clear-air conditions. The original reflectivity in those bins was substituted with data from horizontally neighbouring bins not contaminated with clutter.
Second, reflectivity (dBZ) was corrected for a systematic and persistent underestimation (by a factor of 6 dB). The level and persistence of this underestimation were verified by raingauge observations and were presumably caused by hardware miscalibration. This single-parameter correction needs to be distinguished from actual radar-gauge adjustment (e.g. Goudenhoofdt and Delobbe Citation2009), where parameters typically vary in space and/or time.
In the third step, reflectivity (Z; mm6 m−3) was transformed into rainfall rate (R; mm/h) using Z = 250R 1.2. The same relation is applied by the US national weather service NOAA for tropical cyclone conditions.
Finally, a constant altitude plan position indicator (Pseudo-CAPPI) was generated at 2000 m above sea level based on the sweeps at elevation angles of 1.5° and 2.4° (data from the 0.5° angle had to be discarded due to the study basin’s mountainous terrain and the resulting level of ground clutter contamination). Rainfall intensities at the reference altitude were then interpolated to centroids of sub-basins () as an input for the hydrological model.
The radar-based rainfall estimates were compared with observations at raingauges by Abon et al. (Citation2015). In spite of inherent problems (see, e.g. Kitchen and Blackall Citation1992) such a comparison can detect major deficits in the radar data and it is a useful complement to hydrological validation (Heistermann and Kneis Citation2011). According to , the radar-based data moderately underestimate observed rainfall in the Marikina Basin. In addition to the negative bias, considerable random errors are present. While the radar-based estimates correspond well with gauge data at the daily scale (Nash-Sutcliffe index, E > 0.8), the correlation at the hourly level is rather weak (≤ 0.4). An increased mismatch at shorter time intervals has been found in other studies (Seo and Krajewski Citation2010, Sebastianelli et al. Citation2013), and this may be explained partly by the different sampling volumes of raingauge and radar.
Table 2. Error in radar-based precipitation estimates with reference to raingauge observations in the Marikina Basin. E denotes the coefficient of efficiency (Nash-Sutcliffe index)
3.2 Hydrological model
3.2.1 Model engine
The hydrological model engine used is called HYPSO-RR. It is an object-oriented software built with the ECHSE framework (Kneis Citation2015). HYPSO-RR is designed for the continuous simulation of streamflow in mesoscale river basins. It belongs to the class of conceptual model engines that combine both physically-based and empirical equations. Since HYPSO-RR has been developed mainly for forecasting purposes, the mathematical representation of hydrological processes was kept simple for the benefit of computational efficiency. This is essential because data assimilation (namely state updating) and uncertainty estimation (e.g. using precipitation ensembles) require a large number of repeated model runs.
A short summary of the classes is contained in Kneis (Citation2015, Table 4). A comprehensive documentation of the mathematical formulations of hydrological processes can be found in Kneis (Citation2012a) Subsequently, only the two most important classes are outlined.
3.2.2 Sub-basin class
Objects of this class simulate the water balance at sub-basin level. At present, only three basic types of land use are distinguished: vegetated soil, impervious surfaces and water. Runoff generation on vegetated areas was computed from the water balance of a homogeneous soil column. Direct runoff (surface runoff, sub-surface stormflow) was assumed to occur on saturated zones only. The extent of those zones was estimated using the method of Zhao et al. (Citation1980) and the rate of direct runoff was computed analytically following Todini (Citation1996, signs corrected). The slower components (interflow, groundwater recharge) were modelled as functions of the soil water content as in LARSIM (Ludwig and Bremicker Citation2006). The equations to simulate the energy and water balance of a possible snow cover were mostly adopted from Tarboton and Luce (Citation1996).
Potential evapotranspiration (ET) was estimated from short-wave (gross) radiation and temperature data with Makkink’s approach De Bruin (Citation1987). Actual ET was obtained by multiplying the potential rate with a crop factor (see, e.g. Feddes Citation1987) and a linear term accounting for moisture limitation. The leaf area index was used as a proxy for the crop factor and a linear correlation was assumed between the two quantities. This simple ET model is primarily attractive for the minimum set of predictor variables required.
Storage and concentration of runoff at the sub-basin level were modelled as in LARSIM (Ludwig and Bremicker Citation2006). Thus, the generated runoff was routed through a single linear reservoir to emulate the distribution of travel times. This was done separately for the individual runoff components. The retention parameters of the linear reservoirs were derived from a concentration time index (CTI) which accounts for the sub-basin’s size, shape and topography. The CTI was then scaled with dimensionless factors identified from recession analysis and subsequent calibration.
The set of state variables considered in the sub-basin class comprise (1) the soil water content, (2) the storage volumes of the linear reservoirs controlling runoff concentration, as well as (3) snow-related quantities not relevant in this study’s context. The required set of meteorological forcings includes precipitation, air temperature, short-wave radiation, air pressure, wind speed, relative humidity and cloudiness. For regions without snow cover, only the first four variables are relevant. To allow for seasonal variation, the leaf-area index was treated as an external forcing too.
3.2.3 Reach class
In HYPSO-RR, a river reach is modelled as a single linear reservoir (LR) whose retention constant is variable. The LR approach was adopted for its attractive analytical solution. The particular solution presented in Equationequation (1(1)
(1) ) allows for a linear variation of the inflow rate q
in during a discrete time step of length ∆t.
with
In a LR, the outflow rate (q
ex) is linear function of storage (v), and the retention constant (k) is given by k = v/q
ex. For real channel sections this relation is generally nonlinear. However, in order to take advantage of the analytical solution (Equationequation (1(1)
(1) )), a locally linear behaviour (k ≈ ∆v/∆q) is assumed. The curve v(q) can be inferred from information on the cross-section’s geometry, bed slope and roughness using Manning’s equation; thus, empirical functions k = f(v) and k = f(q) are available. They allow for the selection of an appropriate k for use with Equationequation (1
(1)
(1) ), reflecting the reach’s current state v(t
0) as well as its inflow q
in at times t
0 and t
0 + ∆t.
3.3 Model set-up and calibration
Drainage network and catchment boundaries were derived from the ASTER global digital elevation model (DEM), a product of METI and NASA, using software described in Kneis (Citation2012b). The catchment area () was divided into sub-basins with an average size of 2 km2. Information on land use was gathered from the National Mapping and Resource Information Authority (NAMRIA). Data on the spatial distribution of soil types and average texture information were provided by the Philippines Bureau of Soils and Water Management. The hydraulic properties of river reaches were derived from survey data of 42 cross-sections distributed over the basin. First, power models r(h) and a(h) were fitted for the individual survey cross-sections with h, r and a denoting flow depth, hydraulic radius and wet area, respectively. Second, r(h) and a(h) were calculated for every modelled reach as a weighted average of the respective curves at two survey cross-sections. The particular survey cross-sections were selected according to distance to the reach (to be minimized) and their upstream catchment areas (upstream area of the reach to be within the range of the two cross-sections’ upstream areas). The r(h) and a(h) functions obtained provide the basis for inferring the curve v(q) (see Section 3.2.3) by Manning’s equation.
The hydrological model was calibrated using hourly streamflow data from the Montalban gauge (). Model runs were initialized at least 10 years before start of the actual calibration period and the outputs for this warm-up period were discarded. The initial state for the warm-up period was generated in a long-term simulation repeatedly using meteorological data for the years 2004–2011 as input.
The calibration of nine parameters was carried out by a supervised stochastic approach (Kneis et al. Citation2012, Citation2014) characterized by the following steps:
-
Sampling ranges are specified for all parameters. Plausible ranges can be deduced from basic soil properties or hydrograph analysis (Kneis Citation2012a) or previous model applications.
-
Random parameter sets are drawn from within these ranges by latin hypercube sampling (Carnell Citation2012) and the model is run for all sets (typically 200–1000 runs).
-
For all runs, the objective function is computed and plotted against the corresponding parameter values (one plot for each parameter).
-
The plots are visually inspected and the sampling ranges for sensitive parameters are manually adjusted, i.e. narrowed or shifted.
-
Steps 2–4 are repeated until further modification of the sampling ranges no longer results in an improvement of the objective function after reasonable rounding (e.g. considering three significant digits).
In this study, the Nash-Sutcliffe index (Nash and Sutcliffe Citation1970) was used as the primary objective function. Being a rescaled sum of squared residuals, the Nash-Sutcliffe index naturally puts the focus on peak flows (Krause et al. Citation2005). Information on the bias was used to confine the sampling range (Step 4 above) in cases where the primary objective function was insensitive to changes in the respective parameter. Parameters that control groundwater recharge and drainage were fitted by analysing the model error during periods of low flow.
The model was calibrated separately for (1) precipitation information from raingauges; and (2) radar-based precipitation estimates (see Section 3.4.2). This was considered necessary since errors in forcings may be compensated partly by parametrization (see, e.g. Gourley and Vieux Citation2005, Heistermann and Kneis Citation2011). In the case of gauge-based rainfall, the calibration dataset covered the years 2004–2011; hence, there was a proper distinction between the period of parameter estimation and the period of forecast verification (2012–2013). A Nash-Sutcliffe index of 0.67 was obtained for the entire period 2004–2011. The annual values of Nash-Sutcliffe index ranged from 0.42 to 0.85 and the bias varied between about 25 and +25%. The model performed worst in 2008 and 2010 due to underestimation of peaks.
In contrast to raingauge data, radar-based precipitation estimates were available for the years 2012 and 2013 only, making a distinction between calibration and verification periods impossible. Consequently, some artificial skill was to be expected in the radar-based forecasts, resulting in a too optimistic assessment of forecast performance. In order to examine the hypothesised effect, a cross-check was carried out by feeding the model with radar rainfall while using parameters optimized for the gauge-based QPE. Contrary to expectation, the substitution of model parameters did not result in a relevant drop in the Nash-Sutcliffe index. Hence, the acceptable fit of the radar-driven simulations (Nash-Sutcliffe index of 0.79, bias −0.3%) is unlikely to result from parameter (over)fitting and, therefore, artificial skill in the radar-based forecasts appears to be less of a concern.
Simulated flow rates based on either raingauge or radar data are plotted in together with observations.
3.4 Generation of forecasts
3.4.1 Time frame and basic concepts
The study follows the approach of retrospective forecast verification known as hindcasting. That is, runoff forecasts are generated for a series of events in the past and predictions are then compared to the known outcome, i.e. observed flow data.
The verification experiment covered the two rainy seasons 10 June 2012–15 October 2012 and 6 June 2013–10 September 2013 according to the availability of radar data. During these periods, a new forecast was computed every hour unless streamflow data were missing/invalid at the respective time. The total number of verifiable forecasts amounts to 3810.
The quality of hydrological forecasts strongly depends on adequate initialization of the model’s state variables at the time of forecasting. Those initial values cannot be measured because the state variables often do not strictly correspond to observable quantities (e.g. filling of conceptual reservoirs). Even if they do (e.g. in the case of soil moisture), it is difficult to obtain real-time data with sufficient spatial resolution (see Bronstert et al. Citation2012). Hence, proper initial states must be generated with the model itself by means of a “warm-up” run (leftmost arrow in ). The warm-up run is typically a long-term simulation based on observed meteorological forcings. The warm-up period must be chosen long enough to eliminate the effect of a purely guessed initial state.
Figure 3. Outline of a single forecast experiment. Arrows indicate individual runs of the hydrological model. T0 and LTmax denote the forecast initialization time and the maximum lead time, respectively
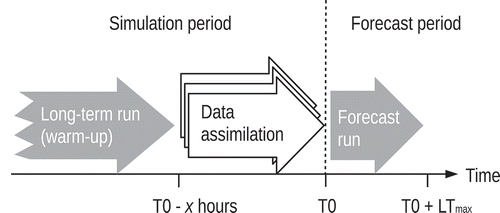
The set of arrows in the centre of indicates repeated simulations carried out in the context of sequential data assimilation (DA, see Section 3.4.3 for details). The DA aims to further refine values of state variables obtained from the warm-up run. Specifically, DA attempts to optimally estimate the values of state variables at time T0 taking into account recent observations.
The rightmost arrow in finally represents the actual forecast run initialized at T0. Since the model is simulating into the future, it must be fed with predicted meteorological forcings in that case (see Section 3.4.2).
3.4.2 Meteorological forcings
In simulation mode, i.e. for all times prior to T0, the hydrological model was forced with meteorological observation data. In the case of rainfall, two alternative variants of QPE were tested. In the first variant, denoted GG, hourly observations from the raingauges shown in were interpolated to the sub-basin centroids by inverse distance weighting (IDW). Terrain was not used as background information since data did not show a close correlation between rainfall and elevation. In the second variant (hereafter denoted RR), the radar-based precipitation estimates according to the specifications in Section 3.1 were used. Observations of other meteorological variables (Section 3.2.2) were also regionalized by IDW.
The forcings used to drive the hydrological model in forecast mode, i.e. beyond T0, were chosen on a pragmatic basis. Regarding rainfall, replicates of the latest known values (i.e. those corresponding to time T0) were used as estimates for future time intervals. This implies stationarity of the precipitation field as well as persistence with respect to rainfall intensities. The assumption was applied to both variants of QPE, i.e. GG and RR. For all other meteorological variables monthly average values were used as dummy forecasts.
The pragmatic choice with respect to meteorological forecasts is justified by the study’s emphasis on short-term predictions. Consequently, the discussion in Section 5 focuses on lead times being not much greater than the basin’s response time (recall Section 1). Experiments targeted at extending the forecast range by means of rainfall nowcasting are currently in preparation.
3.4.3 Data assimilation
Data assimilation (DA) aims to estimate a system’s true state by combining recent observation data with model-generated information. An introduction to DA in the context of hydrological forecasting can be found in Refsgaard (Citation1997). Recent developments and challenges are reviewed in Liu et al. (Citation2012). Many techniques of DA (e.g. state updating through filtering or optimization methods) are targeted at optimal initialization of the model at the forecast time (T0). In contrast, error prediction (EP) refers to the correction of the forecasts (i.e. model outputs) based on the statistics of recent simulation errors. From a theoretical point of view, EP is clearly inferior to updating techniques since predicted flow rates are related to the catchment’s initial state in a nonlinear way. Nevertheless, EP is attractive for practical applications as it is simple to implement and cheap in terms of computational cost.
In this study, we generally relied on EP to merge model predictions with recent observational data. The correction scheme (Equationequation (2)(2)
(2) ) was adopted from an operational model commonly used in Germany (Ludwig and Bremicker Citation2006, pp. 78–79). In the following equation,
is the corrected forecast at lead time with index k and pk
is the corresponding “raw” forecast; symbols or
and sr
denote the observed and simulated flow rates just before forecast initialization:
The distinction between the two cases of Equationequation (2(2)
(2) ) is important. The multiplicative approach (first case) guards against negative values in the case of predicted flow recession. The additive correction (second case) avoids excessive amplification of predicted floods in situations where or
/sr
is large while the absolute error is small, e.g. during low flow. In its present form, Equationequation (2
(2)
(2) ) is applicable to short-term predictions only. An extension of the equations may be necessary in the case of medium- and long-term forecasts, so as to allow for a gradual attenuation of the correction terms over the forecast period.
In addition to EP, combined input and state updating of the hydrological model was applied. In this study, rainfall (observed input) as well as storage in the linear reservoirs controlling sub-basin runoff (state variables; see Section 3.2.2) were updated. The approach belongs to the class of non-sequential DA methods; hence, the goal was to minimize the discrepancy between observed (o) and simulated flow rates (s) in a time period prior to T0. This time period is subsequently called the assimilation period (AP; see ). Its length was set to 24 hours. To measure the discrepancy between o and s, a weighted sum of squared errors (wSSE, Equationequation (3(3)
(3) )) was adopted as the objective function. The weights (w) were chosen to gradually increase over the AP from w
1 = 0 to wn
= 1, in order to give greater weights to observations close to T0 (n: number of hourly observations; equal to 24). The length of the AP and the weights were fixed based on empirical tests.
In the given context, rainfall input during the AP and the initial catchment storage at T0 − 24 h represent the parameters to be optimized when minimizing Equationequation (3(3)
(3) ). Varying these quantities directly is rather inconvenient, not least because rainfall is a time series rather than a scalar. Therefore, the optimization was performed with respect to dimensionless multipliers applied to the original values of rainfall and initial catchment storage. Optimization was generally started with an initial guess of 1 for all multipliers; hence, it was assumed that updating of input and states is not actually necessary. Minimization of the objective function with respect to the multipliers was achieved by stepwise line search. The original algorithm (Kuzmin et al. Citation2008) was adapted to speed up convergence in cases where the objective function is insensitive with respect to a parameter (i.e. a multiplier). To prevent very strong corrections, optimization was constrained. The multipliers’ allowed ranges were set to 0.5–1.5 for rainfall and 0.75–1.25 for initial catchment storage. This is equivalent to maximum corrections by ±50% and ±25%, respectively.
Note that error prediction can easily be combined with model updating. In that case, symbol sr
in Equationequation (2(2)
(2) ) represent the optimized hydrograph after updating of states and inputs.
3.5 Verification
Skill scores (Equationequation (4(4)
(4) )) rate the quality of a forecast in relation to a reference forecast. They are particularly useful if the performance of alternative forecasts is to be compared.
For a perfect forecast, the skill score takes a value of 1 (upper bound). A value of 0 indicates “no skill” as compared to the reference. The lower limit depends on the choice of the actual score used to rate the individual forecasts. In this application, the mean squared error (MSE) was chosen, hence the first term in the denominator of Equationequation (4(4)
(4) ) was set to zero. The resulting skill score (lower bound −∞) is also known as the reduction of variance (RV, Equationequation (5
(5)
(5) )). Identical values of RV were obtained if MSE was substituted by the Nash-Sutcliffe index and the score of the perfect forecast was set to 1, accordingly.
In this study, persistence was used as the reference forecast. It is the natural first choice for short-term predictions because streamflow data exhibit strong auto-correlation for lag times not much greater than the basin’s response time. In other words, recent observations are already valuable predictors for future conditions.
In order to assess the potential value of the deterministic runoff forecasts in absolute numbers, the probability of detection, POD, and the false alarm ratio, FAR (Jolliffe and Stephenson Citation2003), were also evaluated. In order to apply POD and FAR to forecasts of continuous variables, the latter need to be converted to binary variables by means of a threshold value X (see, e.g. Bürger et al. Citation2009). Then, POD represents the fraction of cases where an observed exceedence of X was actually forecast (optimum value 1). FAR represents the fraction of cases where an exceedence of X was predicted but not observed (optimum value 0).
There is often particular interest in POD and FAR for large X corresponding to major events. If X is chosen too large, however, estimates of POD and FAR may be corrupted by the low number of cases (major events are also rare events). In this study, POD and FAR were analysed for a whole range of thresholds (see ), but results based on fewer than five cases were omitted from the presentation.
Figure 4. Reduction of variance (Equationequation (5)(5)
(5) ) as a function of lead time for different forecast variants. GG and RR denote model-based forecasts driven by raingauge or radar data, respectively. Active input/state updating is indicated by the final character “u” while “p” stands for plain forecasts without updating (error prediction was still enabled). A simplistic forecast obtained by linear extrapolation of the observed hydrograph is labelled “Extra”. The vertical line at +3 h marks the basin’s typical response time (RT)
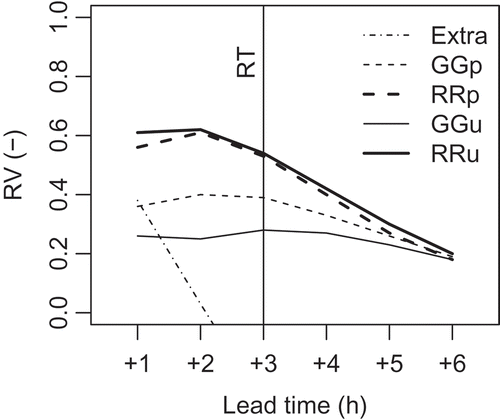
Figure 5. Correspondence between observed (obs) and predicted (prd) flow rates for the “best” forecast according to (variant “RRu”). Results are shown for lead times of 1, 2 and 3 hours (from left to right). E represents the coefficient of efficiency (Nash-Sutcliffe index)
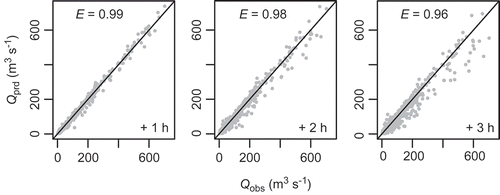
4 Results
All model-based forecasts were found to be skilful for lead times up to 3 h (and beyond) as indicated by a positive reduction of variance (). Forecasts based on radar QPE outperformed forecasts relying on interpolated raingauge data only. Interestingly, the activation of input/state updating did not lead to a consistent improvement in performance. While a slight increase in RV was obtained for the radar-based forecast, updating caused a significant drop in RV for the gauge-based forecasts (see discussion in Section 5). In the longer run, i.e. for lead times greater than the basin’s response time, the difference between the forecasts in terms of RV basically vanishes. It is emphasized that results for lead times beyond +3 h are increasingly affected by the simplistic assumptions regarding future rainfall (recall Section 3.4.2).
For benchmarking purposes, also contains a graph corresponding to a purely data-based forecast (labelled “Extra”). It was generated by linear extrapolation of the observed hydrograph using just the two most recent flow records. Apparently, this simple approach also yields skilful 1 h forecasts but RV is close to zero or negative for all other lead times.
allows for a convenient comparison of different forecast variants. The practical usefulness of a particular variant, however, can only be assessed by means of additional analyses. In the following, the focus is on the best-performing forecast according to RV, i.e. the model-based forecast using radar QPE and input/state updating (labelled “RRu” in ).
illustrates the correspondence between observed and predicted streamflow. For the 1 h forecast, all points lie close to the 1:1 line. Even though the scatter becomes more pronounced at lead times of +2 and +3 h, the results look quite impressive at first glance (E > 0.95). It must be recognized, however, that does not differentiate between the contributions of the known initial conditions (i.e. streamflow at T0) and the model-based prediction. Therefore, it is informative to look at a persistence-adjusted variant of the original scatter plot (). Instead of absolute values (Q), this figure considers the change in streamflow (∆Q) based on the known initial value at T0. Compared to , the scatter plots in appear much more dispersed (but note the different scaling of axes). Dispersion is particularly large in the case of positive values of ∆Q, i.e. when flow rates are increasing.
Probability of detection and false alarm rate were also computed with respect to changes, ∆Q, rather than absolute flow rates, Q. According to , POD hardly exceeds 0.5 and, for higher thresholds and lead times, values go down to a level of about 0.3. Thus, the fraction of cases where an observed rise in streamflow was predicted successfully (in terms of threshold exceedence) amounts to 30–50% only. At the same time, a considerable number of the predictions (20–40%) are classified as false alarms.
5 Discussion
The comparison of different forecast variants with respect to the reduction of variance () indicated clear superiority of the radar-based QPE over the raingauge-based QPE. While this meets our expectations, it is not a trivial finding because other case studies (e.g. Kneis and Heistermann Citation2009, Gourley et al. Citation2011) came up with different results. In this specific case, superiority of the radar-based QPE is probably due to the unfavourable configuration of the current raingauge network (). The northeastern part of the basin is essentially ungauged in terms of rainfall. Moreover, most of the gauges are located at low elevations. Thus, rainfall rates in the mountains of the Sierra Madre, which are probably controlled by orographic effects, cannot be well represented. Given the current configuration of the raingauge network, the radar-based QPE should preferably be used for basin-scale hydrological applications. This finding is particularly relevant because, in spite of the unfavourable configuration, MRB is still one of the most densely gauged basins in the entire Philippines.
In the presented case, data assimilation (DA; Section 3.4.3) essentially contributed to forecast quality. Without any DA, i.e. when recent observations of streamflow were ignored, the correspondence between observed and predicted flow rates would deteriorate dramatically (results not shown). The simple error prediction scheme (EP) was effective irrespective of the source of rainfall data. The additional benefit from input and state updating (Section 3.4.3), however, was comparatively small, as indicated by the graphs “RRu” and “RRp” in . This finding also holds for relaxed constraints on the optimized multipliers. In fact, the overall verification statistics deteriorated if stronger corrections of rainfall data and initial storage were allowed. This was due to massive overestimation of catchment wetness in a small number of cases, possibly triggered by non-representative observations of flow, rainfall, or both. The deterioration of forecasts through updating in the case of the gauge-based QPE (graphs “GGu” and “GGp” in ) is surprising. This gives rise to the assumption that input updating, i.e. the adjustment of precipitation estimates, may be counterproductive if the original estimate shows major deficits, e.g. with respect to spatial patterns. It appears that the drop in RV is explained by a small number of severe overpredictions while, on average, updating had the intended effect.
The closer analysis of forecast performance revealed substantial deficits with respect to the system’s predictive capabilities. These deficits become evident if the initial conditions (known flow rates at forecast time T0) are considered explicitly in the analysis of forecast errors. While the predicted flow rates correspond rather well with the observed ones (E > 0.95, ), the correlation between forecast and observed changes is moderate at best (). The scatter is particularly large in the case of positive ∆Q, i.e. for predictions of rising flow rates. The tendency for underestimation is visible in both and .
The mentioned deficits are also reflected in the statistics of POD and FAR with respect to predicted flow changes (). Given the high number of missed events (POD ≤ 50% for positive ∆Q at lead times greater +1 h), the suitability of the forecast system for flood-warning purposes seems questionable. Besides the low POD, a considerable number of false alarms (FAR > 20%) was identified. The problem of overprediction is, however, restricted to small positive ∆Q (cf. ).
In view of the identified deficits, several options for improvement of the forecast system need to be considered. These options may be assigned to one of three categories: (1) reduction of errors in deterministic forecasts, (2) quantification of predictive uncertainty through probabilistic forecasts, and (3) general extensions of the forecast system, as discussed in the subsequent paragraphs.
5.1 Reduction of errors
One strategy to minimize forecast errors may be targeted at enhanced data assimilation. At present, the greatest potential is seen in the exploitation of additional data sources such as gauge readings from upstream sites. If the forecast system were to be used operationally, quick and reliable data transmission would be important prerequisites. Moreover, a frequent update of rating curves would be necessary since the assimilation of incorrect observation data may result in predictions being worse than those produced with the model alone.
The optimization procedure used in the context of data assimilation (Section 3.4.3) is a rather simplistic one and alternative algorithms should be explored in future studies. Algorithms eligible for operational applications ideally share the following features: (1) the balance between computational speed and accuracy should be tunable via parameters; (2) support for box constraints is important unless a confrontation with corrupted observation data is definitely impossible; (3) non-finite return values of the objective function, e.g. due to a crashed model, need to be handled; and (4) a deterministic algorithm should be selected if reproducibility of results matters as, e.g., in verification experiments. In the context of this study, the employed line-search procedure proved to be robust and computational speed was no cause for concern. For more demanding applications, requiring data assimilation at multiple gauges, for example, the algorithm could be adapted to allow for parallel processing.
5.2 Quantification of uncertainty
Although a further reduction of the error in deterministic forecasts may be achievable, predictive uncertainty cannot be fully eliminated. Consequently, quantification of uncertainty needs to be addressed whenever the forecast system is to be used in decision making, e.g. flood warning. Many concepts to produce probabilistic forecasts by means of deterministic hydrological modelling have evolved over the past two decades. Usually, different sources of uncertainty need to be distinguished, such as inputs, model structure and parameters (e.g. Montanari and Brath Citation2004) as well as initial conditions. While input uncertainty is typically accounted for explicitly by means of meteorological ensembles, the contribution of all other sources is often treated in a lumped way, commonly termed “hydrological uncertainty” (Krzysztofowicz Citation1999, Citation2001). The approaches used to quantify hydrological uncertainty largely fall into one of two groups. The first group encompasses simulation techniques such as GLUE (Beven and Binley Citation1992), and more formal Bayesian methods (Engeland and Gottschalk Citation2002, Liu et al. Citation2005, Sadegh and Vrugt Citation2013). The second group consists of statistical methods to predict the error in forecasts produced by the mechanistic hydrological model (Krzysztofowicz Citation1999, Seo et al. Citation2006, Todini Citation2008, Citation2013, Weerts et al. Citation2011, Brown and Seo Citation2013). Statistical post-processing can be applied to both single-valued forecasts or ensembles originating from explicit treatment of input uncertainty. The statistical methods are particularly attractive for operational uses (see, e.g. Demargne et al. Citation2014) as they can treat uncertainty from various sources at low computational cost.
In this case study, a quite limited number of hindcasts was examined, making it impossible to calibrate an advanced statistical error prediction model. In order to quantify the total uncertainty anyway, a simple but straightforward approach was used to generate a probabilistic forecast of the change in flow rates, ∆Q, (cf. ). Similarly to Weerts et al. (Citation2011), an additive model was adopted (Equationequation (6(6)
(6) )). Here, ∆Q
prd represents the deterministic prediction and e is the corresponding error:
Quantile-quantile plots suggest that the transformed variable follows a normal distribution reasonably well if cases where
(not being of practical concern anyway) are dropped (). Hence, confidence limits of ∆Q can be obtained by picking a quantile from that normal distribution at the desired probability, e.g. at p = 0.95, and adding/subtracting the back-transformed quantile to/from the deterministic forecast ∆Q
prd.
Figure 8. Normal quantile-quantile plots of transformed forecast errors for lead times up to +3 hours
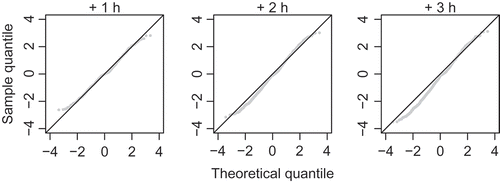
Selected values of e for a number of probabilities are reported in . These are “global” estimates of forecast uncertainty conditioned on the studied events. Attempts to produce more case-specific error estimates by (1) relating e to the value of the deterministic forecast, or (2) exploiting information on the flow rate at T0 or the last known model error were not successful. Correlations between e and these potential predictors were generally weak. differentiates between forecasts of rising and falling flow rates at least.
Table 3. Statistical errors of the deterministic forecasts ∆Q prd for different lead times, probability levels and signs. All values correspond to model set-up “RRu” (best-performing variant according to ). Example: Row 2 indicates that predictions of increasing discharge at a lead time of +1 hour are associated with absolute errors of less than about 15 m3 s−1 with a probability of 90%
5.3 Extensions of the forecast system
Our study focused on lead times of up to 3 hours. Within this time frame, runoff predictions are not sensitive to future precipitation. This was also verified experimentally by re-running the verification experiment with alternative assumptions on future rainfall (e.g. zero values). For practical use, e.g. in flood early warning, an extension of the forecast range beyond the basin’s response time may be desirable. Based on these premises, the generation of radar-based, short-term precipitation forecasts is currently under development. Nevertheless, given the deficits in the short-term forecasts ( and ), the possible quality of runoff predictions for lead times greater than +3 h is limited.
There are many options to further develop the hydrological model engine and to refine parameter estimates. For example, one could explore alternative sub-models for runoff generation, distinguish a greater number of land uses, or increase the overall spatial resolution. From the perspective of an operational user, however, it might be more interesting to see whether an empirical model, e.g. based on an artificial neural network, was a competitive substitute for the conceptual hydrological model. Empirical alternatives to a conceptual model are generally attractive for their unbeaten computational performance and low data requirements. The training of empirical models employed in forecasting, however, requires special techniques (Schmitz and Cullmann Citation2008) to compensate for lack of extrapolation capability.
6 Conclusions
The verification of model-based runoff predictions over two seasons revealed severe deficits in forecast quality. This is true even for short-term predictions entirely controlled by observable rainfall rather than by future precipitation. The performance is particularly limited with regard to the prediction of strong rises in streamflow. The observed deficits are probably rooted in both the insufficient quality of quantitative precipitation estimates (QPE) and the simulation of catchment-scale hydrological processes. Regarding QPE, the case study yields a clear recommendation: given the present configuration of raingauges, runoff forecasting for the study basin should rely on the radar-based QPE.
Storm runoff from the Marikina River Basin has repeatedly caused casualties and high economic losses in recent years. Reliable early warnings are, therefore, of vital interest. Given that, further investment in the forecast system is necessary to lessen the identified deficits. Priorities include the use of additional gauge readings for model updating and further improvements of radar-based QPE, e.g. by enhanced hardware calibration, by applying dynamic raingauge adjustment, and by combining observations from different radar stations in regions of overlap. (See Crisologo et al. (Citation2014) for progress in radar-based QPE for a C-band radar that also covers the Marikina Basin.)
Although the implementation of those measures will reduce the errors in deterministic forecasts, considerable uncertainty is likely to remain. In this context, the parametric uncertainty post-processor outlined in Section 5 should be compared against alternative statistical approaches such as quantile regression. Also, the parameters of any statistical post-processor need to be verified/updated when new hindcasts become available. Finally, more effort must be put into the generation of case-specific estimates of predictive uncertainty from all operationally available information, e.g. by means of generalized linear models.
Extension of the hydrological forecast range via rainfall nowcasting will become a priority task as soon as significant progress has been made at short lead times.
Acknowledgements
All meteorological and hydrological observation data were provided by EFCOS (Effective Flood Control Operation and Services). Special thanks go to the Environment Monitoring Laboratory (EML) members for providing equipment and assistance for field campaigns.
Disclosure statement
No potential conflict of interest was reported by the authors.
Additional information
Funding
References
- Abon, C.C. , David, C.P.C. , and Pellejera, N.E.B. , 2011. Reconstructing the tropical storm Ketsana flood event in Marikina River, Philippines. Hydrology and Earth System Sciences , 15 (4), 1283–1289. doi:10.5194/hess-15-1283-2011
- Abon, C.C. , et al., 2015. Evaluating the potential of radar-based rainfall estimates for streamflow and flood simulations in the Philippines. Geomatics, Natural Hazards and Risk . doi:10.1080/19475705.2015.1058862
- Arnaud, P. , et al., 2011. Sensitivity of hydrological models to uncertainty in rainfall input. Hydrological Sciences Journal , 56 (3), 397–410. doi:10.1080/02626667.2011.563742
- Beven, K. and Binley, A. , 1992. The future of distributed models: model calibration and uncertainty prediction. Hydrological Processes , 6 (3), 279–298. doi:10.1002/(ISSN)1099-1085
- Bronstert, A. , et al., 2012. Potentials and constraints of different types of soil moisture observations for flood simulations in headwater catchments. Natural Hazards , 60, 879–914. doi:10.1007/s11069-011-9874-9
- Brown, J.D. and Seo, D.-J. , 2013. Evaluation of a nonparametric post-processor for bias correction and uncertainty estimation of hydrologic predictions. Hydrological Processes , 27 (1), 83–105. doi:10.1002/hyp.v27.1
- Bürger, G. , Reusser, D. , and Kneis, D. , 2009. Early flood warnings from empirical (expanded) downscaling of the full ECMWF ensemble prediction system. Water Resources Research , 45, W10443. doi:10.1029/2009WR007779
- Carnell, R. , 2012. lhs: Latin Hypercube Samples. R package version 0.10 . Available from: http://CRAN.R-project.org/package=lhs
- Crisologo, I. , et al., 2014. Polarimetric rainfall retrieval from a c-band weather radar in a tropical environment (The Philippines). Asia-Pacific Journal of Atmospheric Sciences , 50 (S1), 595–607. doi:10.1007/s13143-014-0049-y
- De Bruin, H.A.R. , 1987. From Penman to Makkink. In: J.C. Hooghart , Ed. Evaporation and Weather: Proceedings and information . Vol. 39. The Hague: TNO Committee on Hydrological Research.
- De Roo, A.P.J. , Wesseling, C.G. , and Van Deursen, W.P.A. , 2000. Physically based river basin modelling within a gis: the lisflood model. Hydrological Processes , 14 (11–12), 1981–1992. doi:10.1002/(ISSN)1099-1085
- Demargne, J. , et al., 2014. The science of NOAA’s operational hydrologic ensemble forecast service. Bulletin American Meteorological Social , 95 (1), 79–98. doi:10.1175/BAMS-D-12-00081.1
- Ehret, U. , et al., 2008. Radar-based flood forecasting in small catchments, exemplified by the Goldersbach catchment, Germany. International Journal of River Basin Management , 6 (4), 323–329. doi:10.1080/15715124.2008.9635359
- Engeland, K. and Gottschalk, L. , 2002. Bayesian estimation of parameters in a regional hydrological model. Hydrology and Earth Systems Sciences , 6 (5), 883–898. doi:10.5194/hess-6-883-2002
- Feddes, R.A. , 1987. Crop factors in relation to Makkink reference-crop evapotranspiration. In: J.C. Hooghart , Ed. Evaporation and Weather: proceedings and information . Vol. 39. The Hague: TNO Committee on Hydrological Research.
- Frederick, R.H. , Myers, V.A. , and Auciello, E.P. , 1977. Storm depth-area relations from digitized radar returns. Water Resources Research , 13 (3), 675–679. doi:10.1029/WR013i003p00675
- Golding, B.W. , 1998. Nimrod: a system for generating automated very short range forecasts. Meteorological Applications , 5 (1), 1–16. doi:10.1017/S1350482798000577
- Goudenhoofdt, E. and Delobbe, L. , 2009. Evaluation of radar-gauge merging methods for quantitative precipitation estimates. Hydrology Earth Systems Sciences , 13, 195–203. doi:10.5194/hess-13-195-2009
- Gourley, J.J. , et al., 2011. Hydrologic evaluation of rainfall estimates from radar, satellite, gauge, and combinations on Ft. Cobb Basin, Oklahoma. Journal of Hydrometeorology , 12, 973–988. doi:10.1175/2011JHM1287.1
- Gourley, J.J. and Vieux, B.E. , 2005. A method for evaluating the accuracy of quantitative precipitation estimates from a hydrologic modeling perspective. Journal of Hydrometeorology , 6, 115–133. doi:10.1175/JHM408.1
- Heistermann, M. , et al., 2015. The emergence of open source software for the weather radar community. Bulletin of the American Meteorological Society , 96, 117–128. doi:10.1175/BAMS-D-13-00240.1
- Heistermann, M. , et al., 2013a. Brief communication “Using the new Philippine radar network to reconstruct the Habagat of august 2012 monsoon event around Metropolitan Manila”. Natural Hazards and Earth System Sciences , 13 (3), 653–657. doi:10.5194/nhess-13-653-2013
- Heistermann, M. , Jacobi, S. , and Pfaff, T. , 2013b. Technical note: an open source library for processing weather radar data (wradlib). Hydrology and Earth System Sciences , 17 (2), 863–871. doi:10.5194/hess-17-863-2013
- Heistermann, M. and Kneis, D. , 2011. Benchmarking quantitative precipitation estimation by conceptual rainfall–runoff modeling. Water Resources Research , 47, W06514. doi:10.1029/2010WR009153
- Jolliffe, I.T. and Stephenson, D.B. , Eds., 2003. Forecast verification, a practitioner’s guide in atmospheric science . Chichester: Wiley.
- Kitanidis, P.K. and Bras, R.L. , 1980. Real-time forecasting with a conceptual hydrologic model: 1. Analysis of uncertainty. Water Resources Research , 16 (6), 1025–1033. doi:10.1029/WR016i006p01025
- Kitchen, M. and Blackall, R.M. , 1992. Representativeness errors in comparisons between radar and gauge measurements of rainfall. Journal of Hydrology , 134 (1–4), 13–33. doi:10.1016/0022-1694(92)90026-R
- Kneis, D. , 2012a. Eco-Hydrological Simulation Environment (ECHSE) - Documentation of model engines . University of Potsdam, Institute of Earth and Environmental Sciences. Available from: http://echse.github.io/downloads/documentation/echse_engines_doc.pdf
- Kneis, D. , 2012b. Eco-Hydrological Simulation Environment (ECHSE) - Documentation of Pre- and Post-Processors . University of Potsdam, Institute of Earth and Environmental Sciences. Available from: http://echse.github.io/downloads/documentation/echse_tools_doc.pdf
- Kneis, D. , 2015. A lightweight framework for rapid development of object-based hydrological model engines. Environmental Modelling & Software , 68, 110–121. doi:10.1016/j.envsoft.2015.02.009
- Kneis, D. , Bürger, G. , and Bronstert, A. , 2012. Evaluation of medium-range runoff forecasts for a 50 km2 watershed. Journal of Hydrology , 414-415, 341–353. doi:10.1016/j.jhydrol.2011.11.005
- Kneis, D. , Chatterjee, C. , and Singh, R. , 2014. Evaluation of TRMM rainfall estimates over a large Indian river basin (Mahanadi). Hydrology and Earth System Sciences , 18, 2493–2502. doi:10.5194/hess-18-2493-2014
- Kneis, D. and Heistermann, M. , 2009. Bewertung der Güte einer Radar-basierten Niederschlagsschätzung am Beispiel eines kleinen Einzugsgebiets (Quality assessment of radar-based precipitation estimates with the example of a small catchment; in German). Hydrologie und Wasserbewirtschaftung , 53 (3), 160–171.
- Krause, P. , Boyle, D.P. , and Bäse, F. , 2005. Comparison of different efficiency criteria for hydrological model assessment. Advances in Geosciences , 5, 89–97. doi:10.5194/adgeo-5-89-2005
- Krzysztofowicz, R. , 1999. Bayesian theory of probabilistic forecasting via deterministic hydrologic model. Water Resources Research , 35 (9), 2739–2750. doi:10.1029/1999WR900099
- Krzysztofowicz, R. , 2001. The case for probabilistic forecasting in hydrology. Journal of Hydrology , 249, 2–9. doi:10.1016/S0022-1694(01)00420-6
- Kuzmin, V. , Seo, D.-J. , and Koren, V. , 2008. Fast and efficient optimization of hydrologic model parameters using a priori estimates and stepwise line search. Journal of Hydrology , 353, 109–128. doi:10.1016/j.jhydrol.2008.02.001
- Liu, Y. , et al., 2012. Advancing data assimilation in operational hydrologic forecasting: Progresses, challenges, and emerging opportunities. Hydrology and Earth Systems Sciences , 16, 3863–3887. doi:10.5194/hess-16-3863-2012
- Liu, Z. , Martina, M.L.V. , and Todini, E. , 2005. Flood forecasting using a fully distributed model: Application of the TOPKAPI model to the Upper Xixian Catchment. Hydrology and Earth Systems Sciences , 9, 347–364. doi:10.5194/hess-9-347-2005
- Ludwig, K. and Bremicker, M. , Eds., 2006. The water balance model LARSIM - design, content and application. Vol. 22 of Freiburger Schriften zur Hydrologie . Freiburg, Germany: University of Freiburg, Institute of Hydrology.
- Montanari, A. and Brath, A. , 2004. A stochastic approach for assessing the uncertainty of rainfall–runoff simulations. Water Resources Research , 40 (1), W01106. doi:10.1029/2003WR002540
- Nash, J.E. and Sutcliffe, J.V. , 1970. River flow forecasting through conceptual models part I - A discussion of principles. Journal of Hydrology , 10 (3), 282–290. doi:10.1016/0022-1694(70)90255-6
- Pierce, C. , et al., 2004. The nowcasting of precipitation during Sydney 2000: an appraisal of the QPF algorithms. Weather and Forecasting , 19 (1), 7–21. doi:10.1175/1520-0434(2004)019<0007:TNOPDS>2.0.CO;2
- Plate, E.J. , 2009. HESS Opinions “Classification of hydrological models for flood management“. Hydrology and Earth Systems Sciences , 13, 1939–1951. doi:10.5194/hess-13-1939-2009
- Refsgaard, J.C. , 1997. Validation and intercomparison of different updating procedures for real-time forecasting. Nordic Hydrology , 28, 65–84.
- Robinson, J.S. , Sivapalan, M. , and Snell, J.D. , 1995. On the relative roles of hillslope processes, channel routing, and network geomorphology in the hydrologic response of natural catchments. Water Resources Research , 31 (12), 3089–3101. doi:10.1029/95WR01948
- Rodriguez-Iturbe, I. and Mejía, J.M. , 1974. On the transformation of point rainfall to areal rainfall. Water Resources Research , 10 (4), 729–735. doi:10.1029/WR010i004p00729
- Sadegh, M. and Vrugt, J.A. , 2013. Bridging the gap between GLUE and formal statistical approaches: approximate Bayesian computation. Hydrology and Earth System Sciences , 17 (12), 4831–4850. Available from: http://www.hydrol-earth-syst-sci.net/17/4831/2013/
- Schmitz, G.H. and Cullmann, J. , 2008. PAI-OFF: A new proposal for online flood forecasting in flash flood prone catchments. Journal of Hydrology , 360 (1–4), 1–14. doi:10.1016/j.jhydrol.2008.07.002
- Sebastianelli, S. , et al., 2013. On precipitation measurements collected by a weather radar and a rain gauge network. Natural Hazards and Earth Systems Sciences , 13, 605–623. doi:10.5194/nhess-13-605-2013
- Seo, B.-C. and Krajewski, W.F. , 2010. Scale dependence of radar rainfall uncertainty: initial evaluation of NEXRAD’s new super-resolution data for hydrologic applications. Journal of Hydrometeorology , 11, 1191–1198. doi:10.1175/2010JHM1265.1
- Seo, D.-J. , Herr, H.D. , and Schaake, J.C. , 2006. A statistical post-processor for accounting of hydrologic uncertainty in short-range ensemble streamflow prediction. Hydrology and Earth Systems Sciences Discussions , 3, 1987–2035. doi:10.5194/hessd-3-1987-2006
- Solomatine, D.P. and Ostfeld, A. , 2008. Data-driven modelling: some past experiences and new approaches. Journal of Hydroinformatics , 10 (1), 3–22. doi:10.2166/hydro.2008.015
- Tarboton, D.G. and Luce, C.H. , 1996. Utah energy balance snow accumulation and melt model (UEB). Technical report. Utah State University and USDA Forest Service.
- Todini, E. , 1996. The ARNO rainfall–runoff model. Journal of Hydrology , 175, 339–382. doi:10.1016/S0022-1694(96)80016-3
- Todini, E. , 2008. A model conditional processor to assess predictive uncertainty in flood forecasting. International Journal of River Basin Management , 6 (2), 123–137. doi:10.1080/15715124.2008.9635342
- Todini, E. , 2013. From HUP to MCP: analogies and extended performances. Journal of Hydrology , 477, 33–42. doi:10.1016/j.jhydrol.2012.10.037
- Toth, E. , Brath, A. , and Montanari, A. , 2000. Comparison of short-term rainfall prediction models for real-time flood forecasting. Journal of Hydrology , 239, 132–147. doi:10.1016/S0022-1694(00)00344-9
- Vieux, B. and Imgarten, J. , 2012. On the scale-dependent propagation of hydrologic uncertainty using high-resolution x-band radar rainfall estimates. Atmospheric Research , 103, 96–105. doi:10.1016/j.atmosres.2011.06.009
- Weerts, A.H. , Winsemius, H.C. , and Verkade, J.S. , 2011. Estimation of predictive hydrological uncertainty using quantile regression: examples from the national flood forecasting system (England and Wales). Hydrology and Earth System Sciences , 15 (1), 255–265. doi:10.5194/hess-15-255-2011
- Werner, M. and Cranston, M. , 2009. Understanding the value of radar rainfall Nowcasts in flood forecasting and warning in flashy catchments. Meteorological Applications , 16, 41–55. doi:10.1002/met.v16:1
- Zhao, R.-J. , et al., 1980. The Xinanjiang model. In: Hydrological forecasting, Proceedings of the Oxford Symposium. Vol. 129 of IAHS-AISH Publ . Wallingford, UK: IAHS Press, 351–356.