ABSTRACT
The Natural Resource Conservation Service – Curve Number (NRCS-CN) methodology is a widely used tool for estimating surface runoff, which is of prime importance in hydrological engineering, agricultural planning and management, environmental impact assessment, flood forecasting, and others fields. This article reviews the methodology and associated hydrological models used for runoff estimation along with their advantages and limitations. Furthermore, discussion focuses on the potential applications of Remote Sensing (RS) and Geographical Information System (GIS) techniques for estimating hydrological variables, such as rainfall, soil moisture and CN required for the NRCS-CN methodology, as well as future research and opportunities for improved runoff estimation at the macro scale.
EDITOR D. Koutsoyiannis
ASSOCIATE EDITOR A. Efstratiadis
Abbreviations
ADI | = | Antecedent Discharge Index |
AGNPS | = | Agricultural Non-Point Source Pollution |
AMA | = | Antecedent Moisture Amount |
AMC | = | Antecedent Moisture Condition |
AMC I | = | AMC for dry |
AMC II | = | AMC for average |
AMC III | = | AMC for wet |
AMSR-E | = | Advanced Microwave Scanning Radiometer – Earth observing system |
AMSU | = | Advanced Microwave Sounding Unit |
ANN | = | Artificial Neural Network |
APEX | = | Agricultural Policy/Environmental Extender |
API | = | Antecedent Precipitation Index |
ARS | = | Agriculture Research Service (of the USDA) |
ASCAT | = | Advanced Scatterometer |
ASM | = | Antecedent Soil Moisture |
ASTER | = | Advanced Spaceborne Thermal Emission and Reflection |
AWC | = | Antecedent Wetness Condition |
CELTHYM | = | Cell-based Long-term Hydrological Model |
CMORPH | = | Climate Prediction Centre Morphing |
CN | = | Curve Number |
CN1 | = | Curve Number I |
CN2 | = | Curve Number II |
CN3 | = | Curve Number III |
CN4GA | = | Curve Number for Green-Ampt |
CREAMS | = | Chemicals, Runoff and Erosion from Agricultural Management Systems |
DEM | = | Digital Elevation Model |
DMSP | = | Defense Meteorological Satellite Program |
EPIC | = | Erosion Productivity Impact Calculator |
ERS | = | European Remote Sensing |
ESA | = | European Space Agency |
ESRI | = | Environmental Systems Research Institute |
ET | = | Evapotranspiration |
EVI | = | Enhanced Vegetation Index |
GEWEX | = | Global Water and Energy Cycle Experiment |
GIS | = | Geographical Information System |
GLCF | = | Global Land Cover Facility |
GLEAMS | = | Groundwater Loading Effects of Agriculture Management System |
GMS | = | Geostationary Meteorological Satellite |
GOES | = | Geostationary Operational Environmental Satellite |
GPM | = | Global Precipitation Mission |
GR4J | = | Génie Rural à 4 paramètres Journalier |
GRACE | = | Gravity Recovery and Climate Experiment |
HEC-HMS | = | Hydrologic Engineering Centre’s Hydraulic Modeling System |
HELP | = | Hydrologic Evaluation of Landfill Performance |
HSG | = | Hydrological Soil Group |
IRS | = | Indian Remote Sensing |
L-THIA | = | Long-term Hydrological Impact Assessment |
LTHS ASMA | = | Long-term Hydrological Simulation Advanced Soil Moisture Accounting Model |
LTHS-MICHEL | = | Long-term Hydrological Simulation – Michel Model |
LULC | = | Land use/Land cover |
MADRAS | = | Microwave Analysis and Detection of Rain and Atmospheric Structures |
MODIS | = | Moderate Resolution Imaging Spectroradiometer |
MW | = | Microwave |
NASA | = | National Aeronautics and Space Administration (USA) |
NASDA | = | National Space Development Agency (USA) |
NATSGO | = | National Soils Geographic Database (USA) |
NEH | = | National Engineering Handbook (USA) |
NLEAP | = | Nitrogen Leaching and Economic Analysis Package |
NOAA | = | National Oceanic and Atmospheric Administration (USA) |
NRCS-CN | = | Natural Resource Conservation Service – Curve Number |
PERSIANN | = | Precipitation Estimation from Remotely Sensed Information using Artificial Neural Networks |
PRZM | = | Pesticide Root Zone Model |
RS | = | Remote Sensing |
SAR | = | Synthetic Aperture Radar |
SCS-CN | = | Soil Conservation Service Curve Number |
SM | = | Soil Moisture |
SMA | = | Soil Moisture Accounting |
SMAP | = | Soil Moisture Active Passive Mission |
SMOS | = | Soil Moisture Ocean Salinity |
SPUR | = | Simulation of Production and Utilization of Rangelands |
SRTM | = | Shuttle Radar Topography Mission |
SSM/I | = | Special Sensor Microwave Imager |
SSURGO | = | Soil Survey Geographic Database |
STATSGO | = | State Soil Geographic Database |
SWAT | = | Soil and Water Assessment Tool |
SWI | = | Soil Wetness Index |
SWIM | = | Soil and Water Integrated Model |
SWOT | = | Surface Water and Ocean Topography |
SWRRB | = | Simulator for Water Resources in Rural Basins |
TDR | = | Time Domain Reflectometry |
TRMM | = | Tropical Rainfall Measuring Mission |
USDA | = | United States Department of Agriculture |
VIS and IR | = | Visible and Infrared |
VSA | = | Variable Source Area |
WSR 88-D | = | Weather Surveillance Radar 1988 Doppler |
1 Introduction
Estimation of the amount of rainfall that is transformed into surface runoff is of prime importance in hydrological engineering and watershed management. Among the various methods for runoff estimation developed over time, the NRCS-CN methodology (SCS Citation1993, USDA Citation1986), previously known as SCS-CN, is widely accepted and popular, as indicated from its frequent use in the literature. Since its inception, the method has been the focus of extended discussion and debate, although the actual intent of the procedure has often been ignored. In particular, it has attracted intensive and extensive exploration into its formation, rationality, applicability and extendibility, aspects of its pros and cons, and physical significance (e.g. Ponce and Hawkins Citation1996, Mishra and Singh Citation1999, Mishra et al. Citation2005, Citation2008b). The advantages of the NRCS-CN method are its simplicity, predictability, stability, applicability for ungauged watersheds, reliance on only two parameters – namely the CN that specifies the soil storage capacity (S) and initial abstraction (Ia), which is expressed as a percentage of S – its convenience of use and capability of incorporating easily accessible watershed characteristics, such as soil type, land use/treatment, hydrological conditions and AMC (Mishra et al. Citation2004, Citation2005, Sahu et al. Citation2007, Citation2012b, Jiao et al. Citation2015). Due to these many advantages, the method is still commonly used today among water resources practitioners (Singh et al. Citation2015) and enjoys fruitful applications across the globe.
Initially, the method was developed for computation of direct surface runoff from storm rainfall events in small agricultural watersheds in the USA, but very soon it was adopted for several regions, land uses and climatic conditions (Romero et al. Citation2007, Soulis and Valiantzas Citation2013). In the past two decades, runoff estimations and predictions have been strongly improved, after coupling of RS and GIS techniques within the NRCS-CN approach. Due to recent developments in sensor functionality at both temporal and spatial resolution, RS techniques can monitor large regions and provide images at short time intervals, on a repetitive basis. These advantages can be used in two different ways: (a) in terms of model input data and (b) within model parameter estimation. Remote sensing data can be acquired from various satellites (e.g. Landsat, IRS, NOAA, Meteosat, Megha Tropiques) and global missions, and can be used to provide reliable estimations of input data, such as rainfall, soil moisture and CN values. In addition, multi-temporal imagery offers unique opportunities to collect datasets on, for example, soil type, soil texture, LULC and its changes, and other properties that are required for rainfall–runoff modelling of large areas (Schultz Citation1988). Furthermore, visible, microwave and thermal spectral measurements have also proven to have major benefits for hydrological applications. Furthermore, the GIS technique has powerful capability to process spatial and non-spatial data and provide new platforms for data analysis, management and visualization. It also makes it feasible to simulate large areas in many widely used hydrological models.
In this perspective, the purpose of this article is to (a) provide a critical review of the NRCS-CN concept and associated models, its advantages and limitations; (b) discuss recent improvements in the CN concept; (c) discuss the applications of RS and GIS techniques for estimating model inputs and parameters; and (d) summarize the outcomes of this review for future courses of action in research.
2 NRCS-CN method: origins, derivation and limitations
2.1 Historical background
The NRCS-CN method was pioneered and developed by the USDA in 1954, for estimation of surface runoff from a given storm event and construction of design hydrographs in US agricultural watersheds of less than 250 km2. Since its first documentation (Section 3, NEH-4), it has been revised several times (SCS Citation1956, 1964, 1971, 1972, 1985, 1993, 2004). Its origin was based on Sherman’s proposal (Citation1942, Citation1949) for plotting direct runoff vs storm rainfall. The subsequent work of Mockus (Citation1949) focused on estimating surface runoff in ungauged watersheds using information on soil, land use, antecedent rainfall, storm duration and average annual temperature. Andrews (Citation1954) also developed a graphical procedure for estimating runoff from rainfall, for several combinations of soil texture and type, vegetation cover and conservation practices, which are referred to as the soil-cover complex. Finally, the empirical rainfall–runoff relationship of Mockus (Citation1949) and the soil-cover complex of Andrews (Citation1954) led to the foundation of the NRCS-CN methodology (USDA Citation1986).
2.2 Derivation of the traditional NRCS-CN method
The traditional NRCS-CN method is an event-based, lumped rainfall–runoff model. It is based on the combination of the water balance equation:
and the following two proportional equality hypotheses, expressed as:
and
where P is total rainfall, Ia is initial abstraction, F is cumulative infiltration excluding Ia, Q is direct surface runoff, S is potential maximum retention and λ is the initial abstraction coefficient. A combination of Equations (1) and (2) yields the popular form of the NRCS-CN method:
Parameter S is mapped to the CN using:
where S is expressed in mm and CN is a dimensionless runoff index varying in the range 0–100 and also varying with AMC, generally described using a 5-day antecedent rainfall.
This method assumes a long-term average soil moisture condition prior to the start of runoff, and a ratio of Ia to S of 0.2. The first assumption is not always the case for the period of interest in a given area and can result in unreasonable sudden jumps in estimated runoff (Michel et al. Citation2005, Sahu et al. Citation2010), while the second assumption has no scientific basis, is very ambiguous and has raised inconclusive arguments (Sahu et al. Citation2007, Shi et al. Citation2009, Wang et al. Citation2010). Later, Steenhuis et al. (Citation1995) stated that runoff generation from rainfall is not due only to infiltration excess, which, in fact, is consistent with both infiltration excess and saturation excess or VSA hydrology concepts. They derived the fraction of the watershed (Af) contributing runoff by differentiating Equation (4) as:
where Pe is the effective rainfall (cm) and P – Ia is the amount of water required to initiate runoff.
2.3 Limitations of the traditional NRCS-CN method
The NRCS-CN method has a number of limitations and misinterpretations due to the basic empirical structure, as described below:
It was derived from approximately 10 years of rainfall–runoff data collected exclusively from agricultural and rangeland. Therefore, it works best on agricultural sites, fairly on range sites and poorly on humid, semi-arid climatic and forested regions (Hawkins Citation1984, Mishra and Singh Citation2003b).
It was developed simply to estimate the daily runoff that could result from daily rain storms, without taking care of the effect of the antecedent moisture in its basic formulation.
It does not contain any expression for time and, as a result, ignores the impact of rain intensity and duration. In addition, there is no explicit provision for the spatial variability of rainfall. Therefore, in principle, it may not be appropriate for the sub-daily time resolution. Consequently, the use of this procedure in small catchments may be erroneous and prone to relatively large errors as it requires hourly or sub-hourly temporal resolution of the net rainstorm hyetograph (Garen and Moore Citation2005, Eli and Lamont Citation2010).
It is an event-based model, and not a continuous simulation model. Its advancements have significantly altered CN notions and vocabulary well beyond its original definition and validity (Woodward et al. Citation2010).
It works well at the watershed scale, not at the point, plot or field scale (Garen and Moore Citation2005). Thus, it is not specific to a streamflow generation process. In principle, it is incorrect to imply that the runoff is generated entirely from overland flow and not only by infiltration excess from the whole land surface.
It implicitly assumes that infiltration excess is the primary runoff mechanism, but it is also composed of the saturation excess mechanism.
It is not suitable for computation of infiltration during a storm event (Eli and Lamont Citation2010).
Its development took place before computers and GIS were in wide use; these now provide extensive spatial data on terrain, soils and vegetation.
3 Improvements in the NRCS-CN methodology
Despite several limitations and misinterpretations, as mentioned above, and even questionable credibility reported from time to time, the NRCS-CN method has been in continuous use due to its satisfactory performance at the field level. It has also gone through several phases of critical reviews (Rallison Citation1980, Chen Citation1982, Ponce and Hawkins Citation1996, Mishra and Singh Citation2003b, Citation2006, Garen and Moore Citation2005, Michel et al. Citation2005, Walter and Stephen Citation2005, Mishra et al. Citation2007, Singh et al. Citation2010).
In 1990, NRCS and ARS, both agencies of the USDA, formed a joint working group to assess the state of the CN procedure and to chart its future development. The primary task of the committee was to rewrite those portions of the NEH-4 where confusion and problems arose from incorrect and misleading statements or incomplete documentation. The group concluded that the added portion must agree with concepts expressed, references should be included, and the result must be technically defensible. As the work progressed, the vastly expanded database of hydrological soils classification and the capability of modern computers were considered. The use of initial abstraction, Ia, equal to 0.2S was also considered during re-evaluation. Finally, there was significant concern about the possibility of regional and seasonal variations of CNs (Hjelmfelt et al. Citation2001).
The joint working group recognized three distinct different modes of application for the CN method:
determination of runoff volume of a given return period and a given total event rainfall for that return period;
determination of direct runoff for individual events, explaining the variability from event to event, as used in continuous simulation models; and
determination of infiltration rates for short time intervals, as used within unit hydrograph development of flood hydrographs.
In addition to the above agencies, several researchers have also proposed improvements to overcome the limitations of the traditional NRCS-CN method and its modified version with respect to SMA (e.g. Williams and LaSeur Citation1976, Ponce and Hawkins Citation1996, Michel et al. Citation2005, Sahu et al. Citation2007, Wang et al. Citation2012b, Singh et al. Citation2015, Ajmal et al. Citation2015b, Citation2016a), infiltration (e.g. Gabellani et al. Citation2008, Grimaldi et al. Citation2013), as well as potential retention (e.g. Smith and Williams Citation1980, Wang et al. Citation2008, Sahu et al. Citation2010, Babu and Mishra Citation2012) and baseflow (e.g. Coustau et al. Citation2012) processes. Some modified versions of the NRCS-CN method that are applicable for continuous hydrological simulation have also been suggested (e.g. Huber et al. Citation1976, Williams and LaSeur Citation1976, Knisel Citation1980, Woodward and Gbuerek Citation1992, Mishra and Singh Citation2004a, Geetha et al. Citation2007, Kannan et al. Citation2008, Williams et al. Citation2012, Durbude et al. Citation2011, Jain et al. Citation2012), with less interest for ungauged watersheds, because several new parameters were included and, as a consequence, “convenience” and “simplicity” were reduced.
The implementation of the NRCS-CN method in a continuous simulation model within hybrid modelling schemes was also developed in order to represent the variability of rainfall and AMC (e.g. Camici et al. Citation2011, Grimaldi et al. Citation2012a, Citation2012b). In particular, Svoboda (Citation1991) used the CN concept to calculate the soil water content for deriving the rainfall contribution to direct runoff and groundwater. Steenhuis et al. (Citation1995) modified the NRCS-CN equation using the partial-area hydrology concept by simply assuming that runoff occurs from areas that are saturated and that the remaining watershed does not contribute any runoff, giving the expression of Af (Equation (6)). Furthermore, Lyon et al. (Citation2004) developed a modified approach called the CN-VSA method. They combined the traditional method with a distributed topographic index to estimate the saturation probability of a specific area of watershed for predicting distributed runoff volume. Thus, these modifications took into account saturation excess or VSA hydrology.
To overcome limitation 2 (Section 2.3), several improvements in the NRCS-CN method have been attempted and used with different degrees of success over many years, explicitly on the SMA procedure. In particular, Mishra and Singh (Citation2002a) incorporated a new parameter, antecedent moisture, Mc, which can be estimated from prior 5-day accumulated rainfall. Furthermore, Mishra et al. (Citation2006a) proposed a relationship for estimating Mc () to obviate sudden jumps in CN variations with AMC. Michel et al. (Citation2005) reviewed the SMA process and pointed out several structural inconsistencies in the traditional method. They found that these inconsistencies arise partly from the confusion between intrinsic parameter and initial condition, and partly from an incorrect use of the underlying SMA procedure. They also proposed two new parameters: soil moisture store level at the beginning of the event, V0, and the threshold moisture for runoff generation to compute the direct runoff, Sa. Later, Sahu et al. (Citation2007) amended the Michel et al. (Citation2005) model, suggesting that V0 be equal to the sum of pre-antecedent moisture level (V00) 5 days before the onset of rainfall and a fraction of the part of rainfall that is not transformed into runoff. Furthermore, the Sahu-Mishra-Eldho model (Sahu et al. Citation2012b) reformulated the Mishra and Singh (Citation2006) model and hypothesized that the ratio of Q to (P – Ia) is equal to that of the sum of cumulative infiltration after runoff starts and the antecedent soil moisture store level to the maximum retention S0, and that Ia is a fraction of the difference between S0 and antecedent soil moisture store level. The model assumes that the antecedent soil moisture store level can be estimated as a function of P5 and that a watershed of interest is always dry 5 days before the onset of a rainfall event. Geetha et al. (Citation2007) adjusted event CN values for AMCs by modifying the existing NRCS-CN method in two approaches: AMC and AMA. However, Woodward et al. (Citation2010) highlighted major abuses related to the use of CN values in continuous models proposed by Geetha et al. (Citation2007) that are only remotely linked to the NRCS-CN method. Williams et al. (Citation2012) revised the soil moisture index proposed by Williams and LaSeur (Citation1976) for a continuous method, instead of using 5-day antecedent rainfall. They also tested the ability of the soil moisture index to predict runoff over a range of soil properties and conditions with two different runoff models. Recently, Ajmal et al. (Citation2015b, Citation2016a) also proposed an advanced rainfall–runoff relationship for obviating sudden jumps based on both P and P5.
To overcome limitation 3 (Section 2.3), Grimaldi et al. (Citation2013) proposed a CN4GA semi-empirical model from the modified Green-Ampt (Green and Ampt Citation1911) infiltration equation and tested it on 100 observed rainfall–runoff events. This model is also applicable to runoff prediction in ungauged basins. In contrast, Kabiri et al. (Citation2013) found the runoff values computed from the SCS-CN method did not differ from those of the Green-Ampt method. Mockus, the developer of the original method, noted in his later years that the saturation- and infiltration-excess mechanisms are part of runoff generation. This means that landscape factors should also be accounted for in addition to land use, soil class and land management conditions when assigning the CN values. Recently, Bartlett et al. (Citation2016) proposed a theoretical framework (ProStor) to create an extended NRCS-CNx model. The framework describes a spatially lumped, event-based rainfall–runoff modelling to overcome spatial variability of runoff, one of the limitations of the traditional method. It may also be applied with different rainfall–runoff responses at a point and different spatial probability density functions for the watershed variables that are representative of watershed heterogeneities. Furthermore, the spatial distribution of runoff may be mapped to the watershed using the topographic index (e.g. Lyon et al. Citation2004).
To move beyond the limitations of the NRCS-CN method in the context of using advance GIS techniques: Patil et al. (Citation2008) developed a GIS interface to estimate runoff by adopting the typical NRCS-CN method and three modifications: (a) modified CN-I, considering Ia = 0; (b) modified CN-II, considering Ia = λS and λ = αtp, where tp is time of ponding and α is the Horton constant; and (c) modified CN-III by expressing cumulative infiltration, F, as a sum of static (Fc) and dynamic (Fd) components, and substituting them against appropriate parameters of the traditional NRCS-CN method. They tested the above modifications using recorded data for the Banha watershed in Jharkhand, India. Their results showed that modified CN-I performed best, followed by modified CN-III, while modified CN-II performed worst for all AMCs. Moreover, under AMC-based conditions, modified CN-I also performed best for AMC II, while modified CN-II performed worst under all AMCs.
Thus, the above-mentioned recent contributions have provided significant improvements in the understanding of the NRCS-CN method and, consequently, its potential for a wide range of applications. Although primarily developed for event-based rainfall–runoff modelling and flood design on ungauged watersheds, it has been successfully applied in the broader domain of hydrology, watershed management and environmental engineering. The method has also been successfully applied to sediment yield modelling (Mishra et al. Citation2006b, Singh et al. Citation2008, Tyagi et al. Citation2008, Bhunya et al. Citation2009), subsurface flow estimation (Yuan et al. Citation2001, Mishra and Singh Citation2004b, Jain et al. Citation2012), urban hydrology (Kibbler Citation1982, Pandit and Gopalakrishnan Citation1996), water quality (Ojha Citation2012, Narain et al. Citation2015), rainwater harvesting (Kadam et al. Citation2012, Singh et al. Citation2013, Zende and Atal Citation2015) and estimation of ET (Mishra et al. Citation2014b). It is also used to determine soil erosion, and nutrients and pesticides transported from fields and into streams (Garen and Moore Citation2005). This vast applicability clearly proves the prominence of the NRCS-CN technique among prevailing hydrological models.
The widespread application of the NRCS-CN method has led to its inclusion in continuous hydrological simulation models for runoff estimation, such as CREAMS (Knisel Citation1980), AGNPS (Young et al. Citation1989), HEC-I and HEC-HMS (US Army Corps of Engineers), SPUR (Wight and Skiles Citation1987), GLEAMS (Leonard et al. Citation1987), EPIC (Sharpley and Williams Citation1990), SWRRB (Arnold et al. Citation1990), HELP (Schroeder et al. Citation1994), L-THIA (Harbor Citation1994), PRZM (Carsel et al. Citation1997), NLEAP (Shaffer et al. Citation1991), SWIM (Krysanova et al. Citation2000), APEX (Williams et al. Citation2000), CELTHYM (Choi et al. Citation2002) and SWAT (Neitsch et al. Citation2002, Gassman et al. Citation2007). In addition, the capabilities of RS and GIS have encouraged and improved the expanded use of these models worldwide. High-resolution satellite data products from various sensors appear poised to capture the spatial variations in the hydro-meteorological variables and high temporal resolution sufficient to represent the dynamics of the hydrological processes, while GIS tools have proved to be highly useful for the generation of precise and updated information for characterizing drainage basin parameters (Grohmann Citation2004, Korkalainen et al. Citation2007, Hlaing et al. Citation2008, Javed et al. Citation2009, Pankaj and Kumar Citation2009, Singh et al. Citation2014). The published literature highlights the successful application of the NRCS-CN method in distributed watershed modelling (White Citation1988, Moglen Citation2000). Hong et al. (Citation2007) demonstrated the potential for using this simple method when computing runoff values from satellite rainfall data for the globe and for medium to large river basins.
4 Recent improvements in the CN concept
The CN is a dimensionless watershed index. Originally, it was determined using only three inputs, namely soil type, land cover and land management conditions, which are published in SCS NEH-4 tables. The NEH-4 tables provide three CN values: those for the upper and lower bounds and the median, for various typical land–soil complexes. However, the tables do not provide guidance for selecting other CN values throughout the expected range and even its origin seems to be lost; the lack of scientific testing of the results raises some doubts when very accurate results are needed (Hawkins Citation1984).
The CN values determined from look-up tables are usually treated as fixed parameters, although they are random variables that vary across different sources of spatio-temporal variability, such as the effects of storm intensity or of antecedent rainfall and associated soil moisture (Ponce and Hawkins (Citation1996). In addition, CN values also change with regional rainfall–runoff behaviour, quality of the measured data, hydrological soil groups, and seasonal and land-use condition. The CN value theoretically varies from 0 to 100, but, in practice, as validated by experience, it lies between 40 and 98 (Van Mullem Citation1991) when considering Ia = 0.2S. Owens et al. (Citation2003) reported that CN ranged from 97 under bare conditions to 57 under full cover on a hard-setting Sodosol with low surface infiltration rates under bare conditions. For a heavy clay soil with varying levels of cover (25–90%), Foerster and Milne-Home (Citation1995) similarly found optimized CN ranging between 56 and 96 for runoff plots under wheat for different tillage systems in Gunnedah, Australia. Later, Efstratiadis et al. (Citation2014) found that basins such as karst with negligible Ia showed overestimated CN values, in fact much lower, of about 30–40. Therefore, the CN represented a much wider range than values for similar land use and HSGs in NEH tables, thus resulting in significant uncertainty in the determination of CN values.
However, several studies have already indicated that many parameters in addition to soil type and LULC, such as 5-day antecedent rainfall, stage of vegetation growth, soil moisture and interception, explain the variation in individual CN for a watershed with storms. Therefore, it seems necessary to account for these additional parameters to reduce the uncertainty of modelling results and promote more common use of adjusted CN in practice. Soulis et al. (Citation2009) and Steenhuis et al. (Citation1995) noted that variation of CN values according to AMC category alone cannot justify the observed variability of CN values in every case. Yuan et al. (Citation2014) reported that runoff estimation can be very sensitive to initial abstraction coefficient, λ, especially for relatively low rainfall amount and for semi-arid watersheds.
In the following sections, we explain how these parameters affect CN.
4.1 Factors affecting CN
The CN is primarily affected by major runoff-associated watershed characteristics, such as soil type, type of vegetation, land use and treatment, hydrological condition, AMC and climatic regime (Singh Citation1992, Mishra and Singh Citation2003b, Sahu et al. Citation2007). These characteristics mainly affect the infiltration/retention capacity of a watershed. This is elaborated below.
4.1.1 Soil type
Musgrave (Citation1955) described a hydrological classification of soils depending on their infiltration rate. He grouped all soils into four basic groups (A–D) depending on the minimum infiltration capacity. Soils in groups A, B, C and D exhibit high, moderate, low and very low rates of infiltration, respectively. Hence, the CN increases as the soil type changes from HSG A to HSG D. This hydrological classification system was a major stepping stone for the NRCS-CN method (Woodward et al. Citation2002).
4.1.2 Land use/land cover
The SCS defined three major classes of LULC as urban, cultivated, and woods and forests. These classes were further categorized into various subclasses on the basis of land treatment practices, such as contoured, terraced, straight row, bare, etc.
4.1.3 Hydrological conditions
The SCS defines the hydrological condition of a watershed in terms of the percentage area of vegetation cover. A watershed is in good hydrological condition if it is lightly grazed and more than 75% of the area is covered with vegetation. If a watershed is not heavily grazed and 50–75% of the area is covered with vegetation, it is referred to as being in “fair” hydrological condition, whereas “poor” hydrological condition of a watershed is considered if it is heavily grazed and there is vegetation cover on less than 50% of the area. Watersheds in “good” hydrological condition have high CNs and vice versa.
4.1.4 Antecedent soil moisture conditions
The AMC refers to the soil moisture present in the soil profile before the start of an event. On the basis of P5, the NEH categorized AMC into three levels: AMC I, AMC II and AMC III, which refer, respectively, to dry, average or wet conditions and correspond statistically to 90, 50 and 10% cumulative probability of exceedence of runoff depth for a given rainfall event (Hjelmfelt et al. Citation1982). The higher the soil moisture, the higher the CN and vice versa.
4.1.5 Climate and initial abstraction
The water held by initial abstraction in the form of surface detention, infiltration and interception finally goes back to the atmosphere through evaporation and transpiration. These processes are mainly governed by climatic factors, such as humidity, radiation, temperature and wind. The NRCS-CN accounts for the climatic effect in terms of Ia. Moreover, temperature also affects the viscosity of water, which may slightly affect the infiltration rate (Lin et al. Citation2003).
4.1.6 Rainfall duration and intensity
For a given rainfall amount, the storm duration and rainfall intensity are inversely related. The greater the rainfall intensity, the shorter will be the storm duration and vice versa. A storm of shorter duration allows less time for rainwater to stay on the land surface, which leads to smaller infiltration and consequently greater surface runoff. The reverse also holds true.
4.1.7 Slope
The CN2 values mentioned in the NEH-4 tables are appropriate for slopes of around 5% (Sharpley and Williams Citation1990), because these reference values were identified from small agricultural watersheds in Texas, where slope does not play a major role. However, this relationship was never thoroughly tested. According to Hawkins et al. (Citation2009), some researchers suggest employing negative slope adjustments for steeper slopes, which could be related to the erosion of potential surface seals before they can form.
4.2 Determination of representative CN
As mentioned above, the CN can vary within a significant range for a given watershed (Hjelmfelt Citation1991, McCuen Citation2002, Tedela et al. Citation2012). Hawkins (Citation1993) reported that the runoff calculated from the NRCS-CN method was much more sensitive to the CN chosen than to rainfall depth. Thus, several approaches are available to estimate representative CN for a watershed (Hawkins et al. Citation2009), and these can be categorized into two broad groups: (a) hydrological soil-cover complex number procedure, and (b) derivation of CN from field data.
4.2.1 Hydrological soil-cover complex number procedure
This procedure consists of two main approaches: (i) a composite (area-weighted) CN approach, and (ii) a weighted-Q approach.
4.2.1.1 Composite CN approach
Standard CN tables of NEH-4 (SCS Citation1956, 1964, 1971, 1972, 1985, 1993, 2004) are used to estimate CN at fine spatial scales (e.g. grid-cell scale), on the basis of land-use type and HSG combinations. The CN values of the respective hydrological soil-cover complex are multiplied by the respective percentage areal coverage of the complex. The area-weighted CN is computed using:
where CNn and An are CN value and area of the nth watershed, respectively, with n the total number of segments in the watershed and A the total area of the watershed.
Narayana (Citation1993) recommended the use of the composite CN technique in India, with Ia = 0.1S for black clay soil and 0.3S for the remaining soils. In contrast, Grove et al. (Citation1998), Soulis and Valiantzas (Citation2012) and Jena et al. (Citation2012) found that significant errors were produced in runoff estimates using single composite CN values instead of weighted Q. This may be due to the nonlinear form of the NRCS-CN formula. Lantz and Hawkins (Citation2001) also discussed possible errors caused by the use of a single composite CN value.
In the past decade, this approach has been employed with the aid of RS and GIS techniques in distributed watershed modelling. Pandey et al. (Citation2003), Tejaswini et al. (Citation2011), Dhawale (Citation2013), Joycee and Santhi (Citation2015) and many others used RS and GIS to estimate representative CN for a watershed. Randusová et al. (Citation2015) compared different approaches of CN determination using natural and rank ordered data and highlighted the uncertainties of tabulated CN values.
4.2.1.2 Weighted-Q approach
In this approach, runoff (Q) is computed for each sub-area of a watershed using Equations (1) and (5), utilizing the CN value derived for each respective hydrological soil-cover complex of the sub-area from standard CN tables of NEH-4. Finally, the area-weighted Q is computed. Obviously, the weighted-Q approach is superior to the weighted-CN method, as the former is more rational than the latter for water balance reasons. However, the weighted-CN is easier to work with if the watershed has many complexes or for a series of storms. Mishra and Singh (Citation2003b) pointed out that the runoff computed using the hydrological soil-cover complex CN approach would deviate significantly for a wide range of CN values for various complexes in a watershed.
4.2.2 CN from field data
Several researchers have highlighted the difficulty in selecting accurate CN and suggested that CN values could be determined from recorded (P–Q) data. This involves transformation of S, which varies in the range 0 ≤ S ≤ ∞. A value of S can be calculated as follows (Equation (8)), if λ = 0.2 (Hawkins Citation1993) and CN is later computed via Equation (5):
Furthermore, the above equation is transformed (Equation (9)) to determine S, characterizing the watershed, and CN is later computed via Equation (5):
However, CN values calculated using Equations (8) and (9) vary systematically with rainfall depth. In this case, a single asymptotic CN value observed for very high rainfall depths is preferred. In addition, different approaches are also available in the literature to determine CN values from field data, as given below.
4.2.2.1 Asymptotic (rank order) approach
Hjelmfelt (Citation1980) suggested a rank-ordered approach, in which P–Q data are sorted and rearranged so as to have equal return periods. This technique is also referred to as “frequency matching”. The CNs derived from a rank-ordered P–Q dataset give a secondary systematic correlation that always emerges in the watershed as three different behaviours: complacent, standard and violent. The standard and violent cases lead to a constant CN with increasing storm size, whereas complacent behaviour does not lead to a stable determination of CN (Hawkins Citation1993). The standard and violent relationships can be expressed by Equations (10) and (11), respectively:
where is constant as
and k is a fitting constant.
Several researchers have used this approach (e.g. Hawkins and Ward Citation1998, Hjelmfelt et al. Citation2001, Van Mullem et al. Citation2002). Hawkins (Citation1993) suggested that a single asymptotic CN value can be safely determined from measured data for a standard watershed, whereas the CN value cannot be determined for a complacent watershed. A similar suggestion was also made by Hjelmfelt et al. (Citation2001). Bonta (Citation1997) proposed an improvement to the Hawkins (Citation1993) method for the asymptotic determination of CN from measured data in violent and standard watersheds using derived distributions. Rietz and Hawkins (Citation2000) applied this approach for estimating CN for each land use in a watershed at three scales, i.e. local, regional and national. Schneider and McCuen (Citation2005) developed a new lognormal frequency distribution method to estimate CN from measured P–Q data.
However, all the above methodologies for estimating a single asymptotic CN are only applicable for high rainfall depths and they also fail to determine a final CN value in complacent watersheds. More recently, Soulis and Valiantzas (Citation2012) observed a correlation between calculated CN values and rainfall depths in standard and complacent watersheds by considering the spatial variability of soils and land cover; they introduced a two-CN heterogeneous system for calculating watershed runoff and found that, for any watershed characterized by heterogeneous land use, runoff determined with this approach was very similar to the recorded runoff. They also proposed two CN system approaches for runoff calculation, for high rainfall (P > 3000 mm) and low rainfall, and found them more accurate for runoff evaluation as compared to a single asymptotic CN value approach.
4.2.2.2 Median CN approach
Originally, CNs were developed using daily P–Q data corresponding to maximum annual flows from gauged watersheds, for which information on soil-cover complex was available (SCS Citation1972, 1985). The P–Q data were plotted on arithmetic paper having a grid of plotted CN (). The value corresponding to the curve that parted one half of the plotted data from the other half was taken as the median CN for the watershed, and assigned to AMC II (CN2). The upper and lower enveloping curves were taken as representative of AMC III (CN3) and AMC I (CN1), respectively. Schneider and McCuen (Citation2005) stated that the mean or median of the storm-event CNs is the best estimate of CN for the watershed. The median is more suitable for small samples, since it reduces the influence of outliers (Schneider and McCuen Citation2005) and it is more reliable in an operational setting (Hjelmfelt Citation1991).
Figure 1. Determination of CN for AMC I, AMC II and AMC III using the original SCS-CN method. Source: Mishra and Singh (Citation2003b).
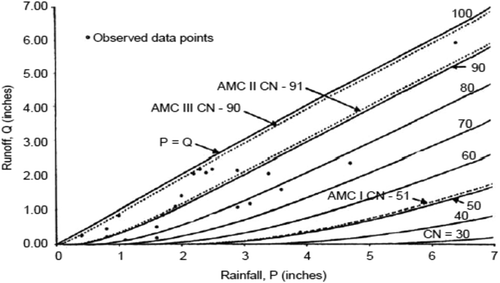
4.2.2.3 Geometric mean approach
This approach has been used to determine a representative CN value if individual values calculated using measured P–Q data from storm events are lognormally distributed. Yet, no one seems to have established the lognormality of CN distributions. The major strength of this approach is quantification of uncertainty with the standard deviation and confidence intervals. The geometric mean is obtained by calculating the average of the series logS, and then estimating the geometric mean maximum potential retention . Then, the geometric mean CN is computed via:
where α = 254 mm. Tedela et al. (Citation2012) and Gundalia and Dholakia (Citation2014) compared this approach of CN estimation with other approaches in their studies.
4.3 CN variation with AMC
The runoff depth estimated using the NRCS-CN method was found to be critically influenced by three main factors: total rainfall, rainfall intensity and AMC (Roberts and Crane Citation1997, Menziani et al. Citation2001, Pfister et al. Citation2003, Weiler et al. Citation2003, Brocca et al. Citation2008). Although AMC is usually considered the most significant factor (Mishra et al. Citation2004), several researchers have suggested that the catchment’s initial conditions are not critical, particularly in the case of large events (Merz and Plate Citation1997, Castillo et al. Citation2003, Brocca et al. Citation2009b, Gamage et al. Citation2015). For any change in AMC (say from AMC II to AMC I or AMC III) in a given catchment, a sudden jump in CN value (i.e. from CN2 to CN1 or CN3) occurs, thus giving the impression of a discrete nature of the CN–AMC relationship.
The CN2, which corresponds to AMC II, depends on the cumulative 5-day precipitation (P5) and is converted to CN1 and CN3 using analytical formulae (SCS Citation1972, McCuen Citation1989, Ponce Citation1989, Singh Citation1992, Mishra and Singh Citation2003b). shows conversion expressions developed by Sobhani (Citation1975), Hawkins et al. (Citation1985), Chow et al. (Citation1988), Neitsch et al. (Citation2002) and Mishra et al. (Citation2008a), and directly from the NEH-4 table. The formula by Sobhani (Citation1975) was found suitable for CN1 conversion and that by Hawkins (Citation1985) for CN3 conversion. Mishra et al. (Citation2008a) developed AMC-dependent conversion formulae that have been compared with the existing ones, using field data taken from the USDA-ARS database. The results showed that their formulae provided the best performance and those of Neitsch et al. (Citation2002) formulae the poorest.
Table 1. AMC-dependent CN conversion formulae.
4.4 Slope adjustment to CN
provides some recently proposed formulae for adjusting slope to CN. Sharpley and Williams (Citation1990) first presented a slope-corrected CN equation. In another attempt, Williams and Izaurralde (Citation2005) developed a relationship for adjusting CN2 for slopes of more than 5%. However, the initial approach led by Sharpley and Williams (Citation1990) has not been intensively tested in the field. Hence, Huang et al. (Citation2006) adopted a simplified approach and developed a relationship for slope-corrected CN2 for slope gradients ranging from 0.14 to 1.4. Recently, Ajmal et al. (Citation2016b) modified their approach for slope adjustment accompanied by a lower λ value and validated its efficacy using a dataset of rainfall–runoff events for South Korean watersheds.
Table 2. Correction formulae for CN2 against slope.
Chaudhary et al. (Citation2013) and Jha et al. (Citation2014) experimentally investigated the effect of watershed slope on runoff generation and resulting CN for a given soil (HSG-C) and land use of sugarcane. They found that a 5% slope yielded the largest runoff and the derived CN values were fairly close to NEH-4 values. Shrestha et al. (Citation2013) found an increment in CN values with an increase in slope and vice versa. Mishra et al. (Citation2014a) and Lal et al. (Citation2015) also reported that slope-corrected CN values provided significantly improved runoff.
4.5 Impacts of rainfall characteristics
As highlighted by Smith (Citation1997) and Yu (Citation1998), among others, the NRCS-CN method does not consider the impact of rainfall intensity or storm duration. Several studies (Hawkins Citation1978, Smith Citation1978) proposed a CN-based infiltration relationship and found that CN decreases as storm duration increases. Rallison (Citation1980) attributed the runoff variation to varying infiltration rates at the soil surface strongly affected by rainfall intensity. Smith (Citation1997) proposed accounting for not only rainfall intensity but also its pattern, since in NRCS-CN methodology a particular storm can produce different runoff hydrographs, depending on rainfall intensity. Jain et al. (Citation2006) and Sahu et al. (Citation2012a) incorporated the storm duration and presented enhanced versions of the traditional NRCS-CN model. Mishra et al. (Citation2008b) developed a rain-duration-dependent procedure to estimate CN for the computation of direct surface runoff from long-duration rains. Mishra and Singh (Citation2002b) presented a NRCS-CN-based time-distributed runoff model. Finally, Mishra and Singh (Citation2004b) established the criterion for the applicability of the NRCS-CN method and extended the concept to derive a time-distributed runoff model and infiltration model.
4.6 Advances in the Ia–S relationship
The Ia–S relationship has been a topic of wide research for a variety of practical applications. Plummer and Woodward (Citation2002) stated that Ia was not accounted for in the original version of the NRCS-CN method during its initial formulation, but, with the continuation of developmental phases, it was assumed to be in a constant ratio of 0.2Ia to S (Equation (3)) for all watersheds. It was described as pre-empting a regionalization based on the geological and climate setting. The validity of the constant 0.2 is also matter of debate and discussion (Woodward et al. Citation2003).
Since its introduction in 1954, this linear relationship has been used to avoid an independent estimation of Ia (NRCS Citation2001). Nevertheless, more representative values have been derived that seem to have less uncertainty for some watersheds. For example, the Central Unit for Soil Conservation (Citation1972) recommended a λ value of 0.3 for all regions of India, except for the black cotton region, for which λ = 0.1 is applied under AMC II and III conditions. Aron et al. (Citation1977) suggested λ ≤ 0.1 and Golding (Citation1979) provided values for urban watersheds depending on CN with λ ≤ 0.075 for CN ≤ 70, λ ≤ 0.10 for CN ≤ 80 and λ ≤ 0.15 for CN ≤ 90. Springer et al. (Citation1980) found that the most commonly used value of λ = 0.2 is not appropriate for either humid or arid watersheds and cautioned against its use for other watersheds.
Several studies indicated that the typical value of λ = 0.2 is strongly overestimated. For instance, Jacobs and Srinivasan (Citation2005) obtained higher correlation coefficients with λ = 0.05 and 0.1 than with 0.2. Lim et al. (Citation2006) computed Q utilizing λ = 0.05 in urbanized areas with HSG-D and also obtained better agreement than those with λ = 0.2 when compared with the observed ones. In a later study, Baltas et al. (Citation2007) determined λ of a small watershed in Attica, Greece. They found that the average ratio of the entire watershed was equal to 0.014, whereas the corresponding ratio at a sub-watershed was 0.037. They attributed this difference to the different spatial distribution of land uses and geological formations at the watershed extent. Beck et al. (Citation2009) computed observed S in 186 Australian watersheds by means of P–Q pairs with a proposed λ = 0.05. Shi et al. (Citation2009) also reported a similar study using the event rainfall–runoff data from the Three Gorges area of China. Their results indicated that λ ranged from 0.010 to 0.154, with mean and median values of 0.053 and 0.048, respectively. They recommended a modified λ value equal to 0.05, for better prediction of runoff from large storm events. Yuan et al. (Citation2014) reported that runoff estimation can be very sensitive to λ, especially for relatively low rainfall amount and for semi-arid watersheds. They found a variation of the Ia/S ratio of between 0.01 and 0.53 for different Walnut Gulch (USA) catchments. A similar study carried out in India by Lal et al. (Citation2015) reported that median and mean λ values were, respectively, 0 and 0.034for original P–Q data, and 0.033 and 0.108 for ordered P–Q data.
Hjelmfelt (Citation1991) revealed that several factors related to storm and landscape interact to explain the initial abstraction. In this context, numerous studies have been carried out across the globe (SCS Citation1956, 1964, 1971, 1972, 1985, 1993, 2004, Springer et al. Citation1980, Ramasastri and Seth Citation1985, Bosznay Citation1989) and values with a variation of λ within the range (0, 0.3) have been reported. However, as the initial abstraction concept accounts for short-term losses, such as interception, depression storage and infiltration before runoff commences, λ can theoretically take any non-negative value, even higher than 1 (Mishra and Singh Citation1999). Ponce and Hawkins (Citation1996) advocated the treatment of λ as a regional parameter to enhance the model responsiveness to a diversity of geological and climatic settings. Mishra and Singh (Citation1999, Citation2003a) also suggested λ to be implicitly related to both S and P. In this context, Jain et al. (Citation2006) proposed a nonlinear Ia–S relationship, incorporating P. Mishra et al. (Citation2006a) modified this relationship by including antecedent moisture, M, since Ia largely depends on it. These relationships were tested using a large dataset of the USDA ARS water database. The results revealed enhanced performance of the nonlinear relationship as compared to the existing ones. Finally, Babu and Mishra (Citation2012) highlighted the dependencies of Ia on AMC and S that vary from event to event, and they related Ia with potential maximum retention (Sabs) and M linearly, which gave improved performance.
4.7 Impact of vegetation on CN
The spatial and temporal variability of vegetation is important because it affects hydrological processes (Ishidaira et al. Citation2008, Temimi et al. Citation2010, Gonzalez et al. Citation2015). Processes such as infiltration, evapotranspiration and water balance are nonlinearly related to soil moisture. Therefore, it is essential to accurately monitor the spatial and temporal variation of vegetation to improve the performance of the NRCS-CN method. Ponce and Hawkins (Citation1996) suggested the necessity of adjusting CN to account for the changing canopy over time. Hawkins and Ward (Citation1998) examined the behaviour of CN and vegetation cover for several bush and grassland sites in New Mexico (USA). Elhakeem and Papanicolaou (Citation2009) estimated a range of CN values in agricultural fields by means of runoff simulators, and proposed a modification to the original CN equations for autumn and summer seasons. Gonzalez et al. (Citation2015) proposed adjusted CNs using the greenness fraction (GA) as proxy for vegetation density to improve the runoff estimation by means of the NRCS-CN method.
4.8 Overparameterization
The scientific community always prefers the simplest hydrological models with a small number of parameters required and superior performance (Merritt et al. Citation2003). This is because overparameterization in hydrological modelling increases the number of processes considered and associated parameters run the risk of having a high degree of uncertainty associated with the method inputs that are translated through to the method outputs. The ultimate factor determining a method’s value is its simplicity relative to its explanatory power (Steefel and Van Cappellen Citation1998, Skaugen et al. Citation2015). Researchers have also demonstrated that overparameterization prevents the possibility of locating a better calibrated value for unidentifiable parameters (Jakeman and Hornberger Citation1993, Grimaldi et al. Citation2013).
In this context, some researchers (e.g. Michel et al. Citation2005, Sahu et al. Citation2007, Babu and Mishra Citation2012, Ajmal et al. Citation2015b) also simplified their improved models to a single-parameter model as the traditional NRCS-CN method for field applications (). However, all simplifications achieved by optimization of the selected variables might make these models less appealing for runoff prediction in ungauged watersheds in other climatic settings (Ajmal et al. Citation2015b).
Table 3. Equations used in NRCS-CN-based hydrological simulation models.
5 Modified NRCS-CN-based hydrological model
A short review on long-term hydrological models based on the NRCS-CN method was presented by Mishra and Singh (Citation2004a). This section provides an updated list of the empirical models and discusses their assumptions, limitations and advantages. The governing equations of these models are given in and their advantages and limitations are discussed in .
Table 4. Advantages and limitations of reviewed models.
5.1 Advanced model
5.1.1 Williams and LaSeur (Citation1976) model
Williams and LaSeur (Citation1976) developed a continuous simulation model by relating the retention parameter, S, of the traditional NRCS-CN model to soil moisture, M, for the computation of direct surface runoff. They were the first to incorporate the antecedent moisture, M, in the traditional methodology (Equation (13)), as it is depleted continuously between storms by ET and deep storage. The parameter M was assumed to vary with lake evaporation, as given by Equation (14). For applying the model in ungauged watersheds, they calibrated the average CN value of the watershed using Equation (15).
5.1.2 Hawkins (Citation1978) model
Hawkins (Citation1978) formulated a continuous SMA procedure by linking ET and CN for use in continuous hydrological simulation modelling. This model uses the volumetric concept to account for the site moisture on a continuous basis. Daily simulations are implemented by using Equation (16), which shows that, as P → ∞, the maximum possible water loss is equal to St (total space available for water storage), which is equal to 1.2S. The maximum water loss at a higher time level, t + ∆t, for storm duration equal to ∆t, can be derived from the moisture balance, given in Equation (17), by coupling these water loss relationships, and through substitution of Equation (6) into the resulting expression, as given in Equation (18) (Mishra and Singh Citation2004a). For known values of ET, P and Q within ∆t, and the computed value of CNt, the next value of CN(t+∆t) is calculated using Equation (18).
5.1.3 Smith and Williams (Citation1980) model
Smith and Williams (Citation1980) improved the NRCS-CN method by modifying the retention parameter, S. As shown in Equation (19), they introduced the weighting factor, Wf (Equation (20)), to account for the initial moisture in the soil profile and related it to the retention parameter. They reduced CN2, corresponding to AMC II, to CN1 (i.e. CN for AMC I) to identify the maximum retention, Smax (Equation (21)), and then adjusted it to the moisture distribution in the soil profile using the weighting technique.
5.1.4 Mishra et al. (Citation1998) model
Mishra et al. (Citation1998) suggested a NRCS-CN-based model for long-term hydrological simulations. They defined AMC based on the NEH-4 table and varied CN with time, as given in Equations (22) and (23). They used a linear regression approach similar to the unit hydrograph scheme to convert rainfall excess into direct runoff, which is given in Equation (24).
5.1.5 Mishra and Singh (Citation2002a) model
Mishra and Singh (Citation2002a) assumed that C = Sr, where C = Q/(P – Ia) is the runoff coefficient, and S is the degree of saturation. Using this concept, they modified the equation of direct runoff by incorporating the antecedent moisture, M (Equation (25)). They also formulated Equation (26), for estimating M.
5.1.6 Choi et al. (Citation2002) model
Choi et al. (Citation2002) proposed a cell-based long-term hydrological model, CELTHYM, for estimating direct runoff from small agricultural watersheds at daily time steps. The direct runoff at the grid scale (qdr) is computed using the traditional NRCS-CN method. Grid runoff is further averaged together to get the sub-watershed direct runoff, Qsub (Equation (27)). Finally, the total direct runoff from the main watershed, Qdr, is obtained by the area-weighted average of the sub-watershed direct runoff, using Equation (28). Choi et al. (Citation2002) calculated the retention parameter using adjusted CN, CNadj, as given in Equation (29). The CN is assumed to vary linearly from CN1 at AMC I to CN2 at AMC II and CN3 at AMC III (Equations (30)–(33), respectively), to allow for the representation of varying soil moisture conditions. In this model, AMC II is assumed at 50% of maximum available soil moisture, i.e. 0.5ASMmax, AMC I is considered the soil moisture at the wilting point, and AMC III is the soil moisture at the field capacity, because the soil moisture varies from wilting point to field capacity after drainage of excess soil water by deep percolation processes.
5.1.7 Mishra and Singh (Citation2003b) model
Both Mishra and Singh (Citation2002a) and Mishra and Singh (Citation2003b) modified the traditional NRCS-CN formula by accounting for the static portion of infiltration, Fc, and antecedent moisture, M. The modified method assumed that, once the soil reaches saturation, a constant rate of infiltration will continue, which accounts for the seepage and percolation losses from the soil; this final constant rate of infiltration is referred to as Fc. At saturation, S = 0, so Q is the amount of rainfall after deducting Fc. The resulting modified expression for Q proposed by Mishra and Singh (Citation2003b) is given in Equation (34).
5.1.8 Mishra and Singh (Citation2004a) model
Mishra and Singh (Citation2004a) proposed a versatile NRCS-CN model for long-term hydrological simulations. They described the water balance equations of Mishra and Singh (Citation2002a) by discretizing them for time t, using Equation (36), and modified the runoff equation of Mishra and Singh (Citation2002a) by means of Equation (37). They computed a dynamic infiltration, Fd, during ∆t using Equation (38), which represents an increase in amount of soil moisture, M, in the soil profile during ∆t. The soil moisture budget is expressed by Equation (39), which yields AMA for the next storm to modify S(t) for the next storm. The sum Sabs = S + M represents the absolute potential maximum retention, which corresponds to the completely dry condition of the soil and represents the maximum possible available void space in the soil profile ranging from zero to the upper bound of S. They also expressed ET(t) in terms of S(t) and Sabs, as shown in Equation (40).
5.1.9 Michel et al. (Citation2005) model
Michel et al. (Citation2005) developed a sounder methodology, hypothesizing that the NRCS-CN model is valid not only at the end of the storm but also at any instant during a storm. Their findings were based on an analysis of the continuous SMA implied by the NRCS-CN equation. They used the SMA process, which was based on the notion that high moisture storage in the soil will lead to higher rainfall conversion into runoff. Finally, they developed a procedure that is more consistent from the SMA viewpoint, by introducing two new variables: V0, representing the initial soil moisture store level (Equation (41)) and Sa (= Ia + V0); here Ia can be either negative or positive and is not a fraction of S, whereas the traditional NRCS-CN method is valid for Ia > 0 only. Based on the above hypothesis and Equation (42), Michel et al. (Citation2005) showed several limitations in the traditional NRCS-CN procedure, due in part to confusion between intrinsic parameters and initial conditions, and partly from an incorrect use of the underlying SMA procedure. The generalized model, with new insight into the SMA process, is expressed through Equations (42)–(44).
5.1.10 Jain et al. (Citation2006) model
Jain et al. (Citation2006) proposed a new model formulation to enhance the NRCS-CN methodology, by incorporating the storm duration and a nonlinear Ia–S relationship, expressed by Equation (45). The modified Ia–S relationship relies on the adjusted rainfall Pc and S (Equation (46)). Jain et al. (Citation2006) also developed a relationship to estimate antecedent moisture content using P5 (48).
5.1.11 Mishra et al. (2006) model
Mishra et al. (Citation2006a) experienced the high dependency of initial abstraction, Ia, on antecedent soil moisture, M, which is very close to reality because the larger the antecedent moisture, the smaller will be the initial abstraction, and vice versa. In this respect, they modified the Ia–S relationship, as given in Equation (49), and used it in the model of Mishra and Singh (Citation2002a) for computing runoff.
5.1.12 Sahu et al. (Citation2007) model
Sahu et al. (Citation2007) proposed an expression for V0 of the Michel et al. (Citation2005) model, primarily based on the assumption that V0 depends not only on P5, but also on S. Considering Sa = αS, consistent with Michel et al. (Citation2005), and using other suitable hydrological assumptions (Equation (51)), they derived Equations (52)–(54) for the three AMC conditions.
5.1.13 Geetha et al. (Citation2007) model
Geetha et al. (Citation2007) modified the existing NRCS-CN method by attributing CN to AMA in place of AMC, to eliminate the sudden jumps in CN values. They considered the current space available for retention, St, which is inversely proportional to CNt, assuming CNt = CN0 or St = S0 for the first 5 days (Equations (55–57)). For following days, St is calculated by Equation (58) and antecedent rainfall is computed using Equation (58). The AMA is a fraction of the antecedent 5-day rainfall, which is expressed through Equation (59).
5.1.14 Kim and Lee (Citation2008) model
Kim and Lee (Citation2008) developed a temporally weighted average CN method for daily runoff simulation, considering daily rainfall and ASM conditions. The model is based on the notion that existing approaches use daily time steps to provide estimations of streamflow, and a rainfall event is defined as the total sum of all rainfall that occurs during the calendar day, ignoring the number of storms and distribution of rainfall within a day. They developed Equation (60) for the computation of the weighted average CN on the current rainy day, by introducing a temporal weight that represents the degree of the effect of increase in soil moisture during the current rainy day.
5.1.15 Wang et al. (Citation2008) model
Wang et al. (Citation2008) proposed a modified curve number (MoCN) model which overcomes limiting the accuracy of Equation (4) by computing S as the difference between S1 and a continuous variable M that represents a decrease in retention due to increase in soil moisture. While Ia is computed using Equation (46) (Jain et al. Citation2006), the other parameter M is represented as a power function of soil moisture, θ (Equation (61)).
5.1.16 Sahu-Mishra-Eldho (2010) model
Sahu et al. (Citation2010) analysed the limitations of the Mishra and Singh (Citation2002a) model and presented a hydrologically more sound procedure, by defining S = S0 – M (here S0 is the absolute maximum retention capacity). They modified Q and Ia estimations through Equations (62) and (63), respectively, and also introduced Equation (64) to calculate M. It may be noted that Equation (64) assumes Ia to be explicitly dependent on M, which is close to reality, given that the higher the antecedent moisture, the lower the initial abstraction, and vice versa.
5.1.17 Durbude et al. (Citation2011) model
Durbude et al. (Citation2011) modified the Michel et al. (Citation2005) model to avoid the unrealistic sudden jumps in V0 by incorporating a conceptual SMA procedure and using daily CN variation based on AMA rather than AMC. Between these two modified models, the long-term hydrological simulation LTHS MICHEL model used V0 for AMC, as proposed by Michel et al. (Citation2005), whereas the LTHS ASMA (advanced SMA) model re-conceptualized the Michel et al. (Citation2005) SMA procedure to avoid the unrealistic sudden jumps in CN, and further in V0, by incorporating an expression for V0 based on AMA. They modified Equations (42)–(44) as Equations (65)–(67), respectively, to compute runoff by using expressions for V0, V00, AMA and SM (Equations (68)–(71)).
5.1.18 Babu and Mishra (Citation2012) model
Babu and Mishra (Citation2012) modified the Jain et al. model (Citation2006) by including a new parameter, Sabs, to overcome most of the limitations existing in the NRCS-CN method and developed a new model consisting of five parameters, α, β, γ, λ and Sabs, as given in Equations (72)–(74). They assumed Sabs to be the sum of S and M (here S is a variable quantity from storm to storm and inversely varies with M). Furthermore, to make the model more realistic, M was modified as a function of P5 as well as Sabs, which is represented as Equation (74). The model was developed with the same existing Ia–S relationship, but considering Sabs in place of S in its expression (Equation (72)).
5.1.19 Modified Sahu-Mishra-Eldho (2012a) model
Sahu et al. (Citation2012a) modified the earlier Sahu-Mishra-Eldho (Sahu et al. Citation2010) model by considering the effect of cumulative infiltration, Fc, on runoff. They separated it from total infiltration, F, and excluded it as it was similar to Ia. They developed an improved equation for computing surface runoff (Equations (75) and (76)) and incorporated the storm duration for computing the static infiltration, Fc, as given in Equation (77).
5.1.20 Wang et al. (2012b) model
Wang et al. (Citation2012b) proposed a more robust modified rational equation (MoRE) by coupling the NRCS-CN model and the rational equation (Qp = CiA, where Qp is peak discharge in m3 s−1, C is a runoff coefficient, i is the average rainfall intensity in mm h−1 for the time of concentration, and A is the area of the watershed in ha). The method includes four intrinsic parameters: CN; permanent wilting point, ψ; field capacity, θ; and saturated soil moisture, Ssat, but excludes Ia. It was found to be an improved version of the traditional NRCS-CN method and the methods of Michel et al. (Citation2005), Sahu et al. (Citation2007, Citation2012b) and Wang et al. (Citation2008). The proposed formula for runoff computation is given in Equations (80)–(81).
5.1.21 Singh et al. (Citation2015) model
Singh et al. (Citation2015) used the C = Sr concept given by Mishra and Singh (Citation2002a) and a modified basic hypothesis of the NRCS-CN methodology by incorporating V0 in it. They formulated a set of equations, as given in Equations (82)–(84), and developed an enhanced version of the model. For the computation of initial soil moisture (V0) and threshold soil moisture (Sa) required for surface runoff generation, they used well-tested functions, as given in Equations (85) and (88), respectively.
5.1.22 Jiao et al. (Citation2015) model
Jiao et al. (Citation2015) introduced the potential initial abstraction (Id), which determines the maximum rainfall storage before runoff and the threshold of daily effective rainfall, and a decay constant, K, which describes the dynamic depletion of antecedent daily effective rainfall induced by ET and seepage. They employed an exponential function to estimate the water depletion and included it for computing the initial abstraction (Equation (89)).
5.2 Single-parameter approaches
5.2.1 Pandit and Gopalakrishnan (Citation1996) model
Pandit and Gopalakrishnan (Citation1996) developed a continuous simulation model for estimating the annual runoff and extended it for determining the annual pollutant loads. This model is useful for urban areas characterized by a given percentage imperviousness, which shows application of the NRCS-CN method in urban hydrology. It involves the following steps (Mishra and Singh Citation2004a):
Determine the pervious CN for AMC II.
Determine the directly connected impervious area of the urban watershed.
Estimate the daily runoff depth for both pervious and impervious areas separately, using Equation (5).
Determine the actual AMC based on P5 and modify CN (using Equations (90) and (91)).
5.2.2 Mishra-Singh (2002) model
Mishra and Singh (Citation2002a) replaced the λ value with 0.2, because it was highly accepted among researchers, and proposed a simplified version of their model for field application. The formulations are given in Equations (92)–(93).
5.2.3 Michel et al. (Citation2005) model
Michel et al. (Citation2005) simplified Equations (42)–(44) by letting Sa be a set fraction of S, i.e. Sa = 0.33S. Additionally, the parameter V0 was replaced by a fraction of S corresponding to lower, median and upper deciles as representative values for AMC I, AMC II and AMC III, respectively. They finally suggested single-parameter models to compute the runoff for these three AMCs, as given in Equations (94)–(96), which retain the original definition of the SCS-CN method.
5.2.4 Mishra et al. (2006a) model
Mishra et al. (Citation2006a) applied their model to 84 small agricultural watersheds in the USA. On the basis of their results, they replaced the parameter α with their mean value (0.72) and parameter λ with their median value (0.08) and proposed a single-parameter model for field application (Equation (97)).
5.2.5 Kannan et al. (Citation2007) model
Kannan et al. (Citation2007) used the expression given by Williams et al. (2000) for ET estimation and developed a simple one-parameter continuous SMA procedure to use within the NRCS-CN methodology for continuous hydrological simulation. The retention parameter at the present time step and an initial value of S are estimated using Equation (98) and the existing value of CN2. Furthermore, they converted Equation (100) for two different conditions in order to estimate retention (Equations (99) and (100)). Equation (101) can be used when soil moisture depletes at a faster rate, whereas Equation (102) can be used when soil moisture depletes at a much slower rate.
5.2.6 Sahu et al. (Citation2007) model
Sahu et al. (Citation2007) applied their advanced models (Equations (52)–(54)) on 82 watersheds, which resulted in a number of optimized values of α and β coefficients. The median values among them were taken as 0.1 and 0.4 for α and β, respectively, and these simplifications yielded a single-parameter model, as described by the set of Equations (103)–(105), with V0 defined in Equation (106).
5.2.7 Ajmal et al. (Citation2015a) model
Ajmal et al. (Citation2015a) considered the high dependency of runoff on the rainfall event and developed a new model for runoff computation. They assumed that Ia is 2% of the rainfall amount, which resulted in the runoff equation described in Equation (107).
5.2.8 Ajmal et al. (Citation2015b) model
Ajmal et al. (Citation2015b) developed a single-parameter model combining the concept of the traditional NRCS-CN model and the SMA procedure from Michel et al. (Citation2005), which was used in the formulation of an event-based empirical GR4J model. They also applied the typical assumption Ia = 0.2S and V0 values suggested by Michel et al. (Citation2005) for the three different AMCs (i.e. V0 = 0.33S, 0.61S and 0.87S for AMC I, AMC II and AMC III, respectively). The continuous variation of S was adjusted on the basis of both P and P5 and replaced using the expression in place of S, in order to avoid sudden jumps in runoff prediction. The runoff equation is given in Equation (108).
5.2.9 Ajmal et al. (Citation2016a) model
Considering continuous Ia after ensembling the traditional NRCS-CN model and the GR4J model, Ajmal et al. (Citation2016a) proposed a single-parameter lumped model by replacing Mc of Equation (44) (Mishra et al. Citation2006a) with V0 (Michel et al. Citation2005) and taking Ia for the three AMCs (i.e. Ia = 0.15S, 0.124S and 0.107S for AMC I, AMC II and AMC III, respectively) to avoid the problem of sudden jumps in runoff prediction. Furthermore, different transformed values of S were used, as in the Ajmal et al. (Citation2015b) model. The proposed runoff equation is given in Equation (109).
6 Use of RS and GIS techniques
The RS technology is now being widely used by the hydrological community, because it considers two important characteristics of hydrological processes, i.e. variability over time and variability in space. Temporal variation has always been accounted for in hydrological measurements, but spatial variation has not been treated adequately with traditional techniques. The spatial distribution of variables is a unique feature of RS, which simplifies their incorporation into GIS. This potential makes several hydrological models more transparent and enables the fast communication of their operations and results to a large group of users. The growing literature on the integration of GIS within hydrological modelling confirms the recognition of such coupled benefits (e.g. DeVantier and Feldman Citation1993, Maidment Citation1996, Kherde and Sawant Citation2013). Numerous studies have also reported the use of GIS in hydrological modelling (e.g. Zhang et al. Citation1990, Bhaskar et al. Citation1992, Srinivasan and Engel Citation1994, Nourani et al. Citation2009, Fry et al. Citation2013).
Furthermore, with the advancement in satellite imagery and digital data, topography, LULC and HSG data are now available in digital format. Given the accessibility of digital databases, NRCS-CN application to distributed hydrological modelling has become very popular in the past two decades. Before describing the extended application of NRCS-CN methodology coupled with RS and GIS, it is appropriate to provide a brief account of the availability of digital databases for predicting CN to distributed hydrological modelling.
6.1 Availability of digital data
6.1.1 Topography
Spatially distributed topographical details are available in the form of DEMs. These models have been used widely to derive hydrological features which serve as inputs to various hydrological models. Currently, elevation data are available from several sources and at different spatial resolutions. Globally, DEMs can be downloaded from either the Shuttle Radar Topography Mission (SRTM) at http://srtm.usgs.gov/(90-m resolution, except for USA with 30-m resolution), or ASTER (30-m resolution). Using a GIS, the process of determining any of the required quantities from a DEM is largely an automated process.
6.1.2 Land use
Land use is an important characteristic of the runoff process that affects infiltration, erosion and evapotranspiration. In digital format, land use is often described by a series of polygons over a region, with each polygon indicating a region of assumed homogeneous land use. There are several sources available to describe land use at different resolutions. Landsat Thematic Mapper (TM) (30 m resolution) images represent valuable and continuous records of the Earth’s surface over the past three decades. Moreover, the entire Landsat archive is now available freely to the scientific community, which helps in identifying and monitoring changes in manmade and physical environments. The satellite data covering a study area can be downloaded from the Global Land Cover Facility (GLCF; http://glcfapp.glcf.umd.edu:8080/esdil) and the USGS Earth Explorer site (http://earthexplorer.usgs.gov/).
6.1.3 Soil data
Soil hydrodynamics information is one of the crucial inputs needed to assess the impacts of existing and alternative land-use management practices on runoff. Thus, it is important to evaluate the effects of the spatial scale at which the soil database is developed. The NRCS maintains spatially different soil databases in three GIS formats: (a) STATSGO (mapping scale: 1:250 000); (b) SSURGO (mapping scale: from 1:12 000 to 1:31 680); and (c) NATSGO, which can be used to derive soil water parameters such as saturation soil moisture, wilting point and field capacity needed for runoff estimation. The STATSGO database contains data as polygon coverage and is available throughout the USA. SSURGO is a more detailed soils database; however, not all areas are covered in digital format. However, NATSGO data describe variations in soil type from a multi-county to state scale (USA). Mednick (Citation2010) studied the effects of spatial resolution (STATSGO vs SSURGO) on runoff across Wisconsin, USA, using a synthetic rainfall dataset and concluded that STATSGO-based estimates under-predicted the impact of rainfall intensity.
6.2 Coupling with NRCS-CN methodology
Integration of GIS and RS in hydrological modelling can enhance the quality of a hydrological model. Brooner et al. (Citation1987) described many hydrologically significant parameters that can be obtained through RS, including land cover, vegetation properties, near surface soil moisture, surface roughness, ET, thermal and moisture indices and imperviousness.
Event-based hydrological simulations can be implemented through modification of the CN based on ASM, which can be retrieved using RS data. The most practiced approach for determining AMC is through API, by means of 5-day precipitation (SCS Citation1971), which can be retrieved using RS data. Blanchard et al. (Citation1981) and Rosenthal et al. (Citation1982) also related API to remotely sensed surface soil-moisture content. In contrast, long-term hydrological simulation models have a comprehensive structure, which requires a number of components, for example, infiltration, ET, percolation and subsurface flow. Among these, ET and surface runoff can also be estimated using remotely sensed data (Karimi and Bastiaanssen Citation2015).
GIS utilities have been exploited for the pre- and post-processing of spatially distributed hydrological data. Mancini and Rosso (Citation1989) examined the spatial variability of the SCS-CN using a raster GIS and a distributed hydrological model. Choi et al. (Citation2002) developed the CELTHYM model which can be integrated with a GIS to predict continuous streamflow from small agricultural watersheds on a daily time step. They used the NRCS-CN method considering soil moisture on a daily basis for surface runoff estimation (Savvidou et al. Citation2016). Therefore, the GIS is found to be an acceptable alternative, greatly simplifying data preparation and processing.
There are many articles that discuss the role of RS techniques for any particular application, namely, estimation of rainfall (Petty and Krajewski Citation1996, Kidd and Levizzani Citation2011), land surface evaporation (Kalma et al. Citation2008), water quality (Ritchie et al. Citation2003) and runoff (Schultz Citation1996). Several studies are also available evaluating the multidimensional applications of RS in hydrology and water resources (Kite and Pietroniro Citation1996, Kumar and Reshmidev Citation2013). In this article, we emphasize the role of RS and GIS in estimating and representing input data (e.g. rainfall, soil moisture) and watershed properties (e.g. LULC), as well as model parameters (e.g. CN) that are used within NRCS-CN methodology.
6.2.1 Rainfall
Rainfall exhibits high space–time variability and requires frequent observations to ensure adequate representation. Such observations are not possible through surface-based measurements over large areas, particularly in inaccessible regions (Huffman et al. Citation2007). Thus, observations through high-resolution satellite sensors have massive potential for use in hydrological modelling, particularly in developing countries, as an alternative to conventional raingauges, which are typically sparse. Satellite-based rainfall estimation techniques can be grouped broadly into three categories: (a) VIS and IR; (b) active and passive MW; and (c) a combination of both. The VIS and IR sensors offer data at very high temporal resolution but with crude estimation of rainfall, whereas MW sensors (e.g. AMSU, MADRAS) provide more accurate estimation of rainfall but at coarse temporal resolution. Therefore, the third category is the best for rainfall estimation, as recognized by numerous researchers (Adler et al. Citation1993, Jobard and Desbois Citation1994, Vicente and Anderson Citation1994, Mishra et al. Citation2009, Prakash et al. Citation2009, Behrangi et al. Citation2011). Furthermore, soft computing techniques, such as fuzzy logic and ANN, make use of IR and MW at various degrees of complexity and targeting different rainfall regimes (Sorooshian et al. Citation2000, Todd et al. Citation2001).
Over the past four decades, satellite rainfall products have been used widely for runoff estimation (Hong et al. Citation2006, Su et al. Citation2008, Ashenafi and Hailu Citation2014). Yilmaz et al. (Citation2005) investigated the use of a satellite product called PERSIANN in streamflow forecasting, using a lumped hydrological model over several medium-size basins in the southeastern USA. Their analysis showed the improved performance of remotely sensed rainfall data in hydrological modelling when the model was calibrated with satellite data. Hossain and Lettenmaier (Citation2006) described a number of issues that must be addressed prior to the adoption of global satellite rainfall datasets in hydrological models. Artan et al. (Citation2007) demonstrated the enhanced performance of remotely sensed rainfall data in hydrological modelling by using satellite data instead of gauged rainfall to recalibrate the GeoFSM hydrological model for four sub-basins of the Mekong and Nile rivers. Milewski et al. (Citation2009) used TRMM (3B42.v6) rainfall data for estimating runoff and observed that the regionalization approach is not a substitute for traditional methodologies that rely on extensive field datasets from raingauge and streamflow networks. Yet, they claimed that these can provide first estimates for rainfall and runoff over large sectors of the arid world lacking adequate coverage with spatial and temporal precipitation and field data. Sagintayev et al. (Citation2011) also reported similar results and suggested the use of extensive field datasets. Their methodology was found useful for less-studied watersheds of the world, especially in areas where field data are inadequate or accessibility is limited.
In recent years, several applications using satellite-derived rainfall within CN-based hydrological models have also been reported. For instance, Jacobs and Srinivasan (Citation2005) evaluated several versions of the NRCS-CN method for estimating near real-time runoff for naturalized flow using high-resolution radar (WSR-88D) rainfall data for watersheds in various agro-climatic regions of Texas, USA. They found that the use of the CN1 value and a reduced initial abstraction ratio (equal to 0.05) produced the most statistically significant comparison between observed and estimated runoff. Hong et al. (Citation2007) presented a global runoff model applying the NRCS-CN method. They used three RS global datasets: (a) rainfall data (TRMM 3B42), (b) LULC data (MODIS) and (c) soil data (TERRASTAT database). Their model calculated pixel-based CN values and runoff depths using the standard approach that has been practiced in hydrology for several decades. Their results showed that calculated runoff volume was larger than observed runoff for the flat and larger watersheds. Yilmaz et al. (Citation2010) evaluated the global runoff model developed by Hong et al. (Citation2007) and found that the model was able to simulate the onset of flood events produced by heavy precipitation; however, the simulation performance declined in the later stages, which pointed out the need for an improved routing component. Global-scale analysis showed that the model is able to detect 38% of the observed floods; however, it suffers from region-dependent bias. Curtis et al. (Citation2007) also used satellite-derived rainfall and gauged runoff data to estimate CN for basins in eastern North Carolina, USA. Harris and Hossain (Citation2008) found that the NRCS-CN method is more robust than more complex schemes in terms of handling uncertainty that exist in satellite rainfall data products. Collischonn et al. (Citation2008) noticed that TRMM-driven daily simulations performed well for representing low flows, but peak flows tended to be overestimated. Finally, Coustau et al. (Citation2012) developed a distributed rainfall–runoff model, coupled with the NRCS-CN method, and assessed its performance using radar rainfall data. The calibration results were found satisfactory by using hourly time steps, and the radar rainfall inputs significantly improved the simulations of the initial conditions for five events at the beginning of autumn (mostly in September and October), but were less efficient for the events at the end of autumn.
6.2.2 Soil moisture
The RS techniques, by virtue of their large area coverage and frequent revisit capability enabling repeated estimates on regular basis, are highly suitable for soil moisture estimation. Runoff estimations have been strongly improved after the addition of remotely sensed AWC data (Aubert et al. Citation2003, Jacobs et al. Citation2004, Crow and Ryu Citation2009, Brocca et al. Citation2010). These data can be retrieved from different RS methods, which can be classified into three main groups: (a) active, (b) passive and (c) a combination of both. The combination of high spatial resolution information from active sensors with high temporal resolution information from passive sensors on an extended area provides more accurate AWC. Microwave combined algorithms, statistical analysis techniques and neural network applications with both active and passive data are also highlighted in the literature.
Numerous researchers have used remotely sensed soil moisture products for hydrological modelling and found promising results (Goodrich et al. Citation1994, Wagner et al. Citation1999, Tischler et al. Citation2007, Wang et al. Citation2011, Tramblay et al. Citation2012, Brocca et al. Citation2013, Massari et al. Citation2014, Citation2015). In particular, in the context of CN-based applications, Hong et al. (Citation2007) used API as a proxy of AMC to estimate time-varying CN values determined by 5-day normalized API. Their results demonstrated the potential of satellite rainfall data for AMC estimations, to then be used for runoff estimation. Beck et al. (Citation2009) showed that CN-based runoff estimates at 186 Australian catchments were improved after inclusion of proxy soil moisture estimations based on remotely sensed data (AMSR-E and TRMM). In particular, the largest improvement was detected in drier catchments with low EVI and topographical slope. Brocca et al. (Citation2009a) investigated the potential of the ERS scatterometer for assessment of wetness conditions in three sub-catchments of central Italy. They derived SWI to estimate the retention parameter of the NRCS-CN method and compared it with AWC derived from selected flood events. The results showed the great reliability of SWI for estimation of wetness conditions at the catchment scale and it was highly correlated with observed maximum potential retention. The computed runoff depth estimation provided good performance with an error of less than 25% for 80% of the investigated rainfall–runoff events. In another study, Brocca et al. (Citation2009b) examined the relationship between soil moisture measurements of in situ and satellite sensors and soil potential maximum retention parameter. An inverse linear relationship was found, with the coefficient of determination decreasing with catchment area, but significant for the largest catchment. Tramblay et al. (Citation2010) used TDR observations of soil moisture to estimate the potential retention of a small headwater catchment in France, and they found good correlation between TDR predictor and calibrated values of S. Tramblay et al. (Citation2012) evaluated the ability of RS data (ASCAT and AMSR-E) to produce AWC for use in the NRCS-CN method. They used three AWC estimators (ADI, API and SMA) based on rainfall and ET. The results showed that both satellite products were able to reproduce daily soil moisture dynamics simulated by the SMA model with satisfactory accuracy. Finally, Meier et al. (Citation2011) developed a forecasting model, based solely on RS data, providing soil moisture and rainfall estimates for prediction of discharge and applied it to three different sub-basins of the Zambezi River basin (Africa). The application of this model in a real-time framework yielded good results in watersheds where soil storage is an important factor.
6.2.3 Curve number
The CN depends on soil and land-use characteristics, the spatial variability of which is better represented through RS (Ragan and Jackson Citation1980). The use of RS also helps in updating land-use changes, particularly in areas where the land-use pattern is highly dynamic, causing significant variation in the hydrological processes. Then, an RS map can be integrated with HSGs (A-D) of the watershed using GIS, which gives more reliable CN values.
White (Citation1988) applied the NRCS-CN method for runoff estimation by combining watershed information with daily rainfall data, using GIS. Stuebe and Johnston (Citation1990) used GIS to predict the runoff volume for six basins. Tauer and Humborg (Citation1992) used RS data and GIS to determine potential sites for water harvesting. Gumbo et al. (Citation2002) found that the CN method worked well in the GIS environment because of its relatively simple formulation. Akhondi (Citation2001) pointed out that increasing watershed area decreases correlation between observed and estimated discharge using the CN method. Jasrotia et al. (Citation2002) determined the rainfall–runoff relationship for the Tons watershed (India), by deriving CN through RS and GIS techniques. Sandholt et al. (Citation2003) also investigated the use of RS data in distributed hydrological modelling.
Zhan and Huang (Citation2004) developed the ArcCN runoff tool, an extension of ESRI ArcGIS software, which can be applied to determine CNs and calculate runoff for a storm event within a watershed. They also suggested that the implementation of a precipitation time series and consideration of factors such as dry and wet AMCs would improve the runoff predictions. Kamuju (Citation2015) compared runoff estimated by the ArcCN runoff tool and ArcSWAT and found that the first gives 90% of rainfall in the form of runoff, while 75% of rainfall becomes surface runoff from ArcSWAT.
Nayak and Jaiswal (Citation2003) found a good correlation between measured and estimated runoff depth using GIS with CN, and concluded that GIS is an efficient tool for preparation of most of the input data required for the NRCS-CN method. Many researchers applied this technology to calculate runoff curve numbers using Landsat, IRS-1A and IRS-1B data. Ebrahihimian et al. (Citation2012), Fan et al. (Citation2013) and many others have estimated runoff using the CN of a basin and RS data in a GIS environment. Ebrahimian et al. (Citation2012) and Garg et al. (Citation2013) tested the effect of slope on runoff using the NRCS-CN method and RS and GIS. Many others used NRCS-CN coupled with RS and GIS technologies for runoff estimation (e.g. Sharma and Singh Citation1992, Chakraborti Citation1993, Tiwari et al. Citation1997, Chatterjee et al. Citation2001, Tripathi et al. Citation2002, Ashish et al. Citation2003, Bhanumurty et al. Citation2003, Gupta and Panigrahy Citation2008, Ramakrishnan et al. Citation2009, Gajbhiye and Mishra Citation2012, Dhawale et al. Citation2013, Nagarajan and Poongothai Citation2012, Nayak et al. Citation2012, Sindhu et al. Citation2013, Thakuriah and Ranjan Citation2014, Zende et al. Citation2014, Topno et al. Citation2015). Finally, Hernández-Guzmán and Ruiz-Luna (Citation2013) developed a vector-based interface to estimate CN and runoff volume for hydrological evaluations. The interface allows users to select different AMC and λ to fit the local conditions.
6.2.4 Land use/land cover (LULC)
Pandey and Sahu (Citation2002) pointed out that LULC, which is used for the determination of CN, is an important input of the NRCS-CN method. Ragan and Jackson (Citation1980) showed that urban land uses, as well as various categories of agriculture and forest cover, can be determined accurately through RS, and can then be used to estimate CN values. Still and Shih (Citation1984, Citation1985, Citation1991) used Landsat data to develop a basin-wide runoff index and successfully demonstrated how RS data can be used to track the changes in runoff that occur in a basin due to land-use change. Sharma et al. (Citation2001) digitally analysed multi-date satellite imagery for improving accuracy in mapping LULC in a multi-crop cultivated watershed.
7 Advantages and limitations of RS and GIS-based approaches
The rising accessibility of high-resolution satellite data products is providing hydrologists and water resource practitioners with ample opportunities to improve their simulations, especially in developing countries and inaccessible regions where in situ measurement networks are sparse (Anagnostou Citation2004, Hong et al. Citation2006, Hossain and Lettenmaier Citation2006, Ebert et al. Citation2007, Gourley et al. Citation2010, Yong et al. Citation2010, Bitew and Gebremichael Citation2011, Wang et al. Citation2012a, Xue et al. Citation2013, Awadallah et al. Citation2016). As indicated so far, the success of NRCS-CN methodology is often governed by the precision of CN and Ia parameters, which are very sensitive. Typically, these are assumed constant over space and time. However, even for the same location, these values are highly variable due to changes in land use, crop cover, crop growth, land treatment etc. Therefore, RS and GIS techniques provide inputs more efficiently, as well as changing parameters (i.e. CN) to NRCS-CN methodology, as discussed in previous sections.
Despite the multiple advantages offered by RS and GIS techniques in hydrological modelling, they have some limitations. For example, in most satellite systems the revisit time can be a critical problem in studies involving rapidly changing conditions, such as surface soil moisture, and, especially, if a constant viewing angle is important, the revisit time can be much longer. Thus, optimizing the time and frequency of coverage is a critical problem for measuring soil moisture. Microwave remote sensing offers unique advantages over other spectral regions. However, major difficulties exist because the MW signal is influenced by various terrestrial factors (e.g. surface roughness, vegetation) and the resolution in space (passive MW) is too coarse for many hydrological tasks. AMSR may be the optimal option for mapping soil moisture in regions of low vegetation cover (<1 kg m−2 vegetation water content), because it operates at high frequency, i.e. 6.9 GHz (Njoku et al. Citation2003).
In the case of precipitation, there are many RS possibilities, including ground-based radar, VIS and thermal IR satellite imagery and MW satellite data. Radar systems provide high-resolution precipitation data over space and time. However, their spatial extent is inadequate to detect global precipitation and to evaluate hydrological models at global and continental scales. This limitation highlights the importance of satellite-based global precipitation data for hydrological and other studies. Over the past decade, the use of precipitation data observed by satellites in hydrology has advanced greatly with the launch of various satellite series (i.e. GOES, DMSP) and research platforms and with the development of more sophisticated retrieval algorithms (e.g. TRMM 3B43, CMORPH, PERSIANN). Despite the significant developments in satellite observation of precipitation, the coarse spatial and temporal resolution of the data are still inappropriate for most hydrological and climate studies. In this respect, there exist a number of challenges, such as: (a) the development of hybrid solutions to combine the benefits of satellites and ground observations (e.g. ground radars and gauge networks); (b) the use of advanced multi-spectral algorithms to improve the accuracy and spatial resolution of satellite precipitation; (c) improving computational efficiency; (d) enhancing the ability to detect liquid and solid precipitation, (e) the development of IR-based algorithms for capturing extreme precipitation more reliably; and (f) understanding and communicating uncertainties of precipitation estimates and forecasts.
8 Future research and opportunities
In general, future research and opportunities are related to present and upcoming information requirements over large areas, and the expected evolution of the associated data and methodology. In all these areas, the NRCS-CN methodology has received a strong boost. The methodology still has issues requiring further improvement, which can be achieved by incorporating the effects of spatial and temporal variations of rainfall, soil moisture and LULC parameters, treating λ as a regional and climatic parameter, estimating CN using RS and GIS techniques, transferring model parameters to regions with similar hydro-climatic and physiographic characteristics (e.g. slope), and refining the Ia–S relationship for different regions and different soil, land-use and climate conditions.
In addition to the above suggestions, another major inconsistency of the method is that it implicitly seems based on infiltration excess and similarity of the Horton-based approach. Saturation excess, however, is dependent on landscape factors that are rarely or never used to defined CN values. Thus, there is a need to develop methods using saturation excess as an important hydrological process.
Recent development in RS techniques and in current satellites and sensors provide many of the necessary data to supplement conventional data, to expand the NRCS-CN methodology in new directions. Ongoing development and future satellite missions, as well as innovative GIS techniques, will offer entirely new data types and forms that will improve the functioning of the method, which will also help hydrologists tackle previously unsolved problems. In addition, combinations of RS data from different sources, as well as combinations with spatially distributed in situ measurements (e.g. digital maps, digital terrain models), provide very promising approaches for the purpose of CN-based runoff computations in the future.
Current satellites/sensors and missions also offer new opportunities for rainfall and soil moisture estimation, in general, and hopefully for MW applications, in particular. Rainfall can be estimated from geostationary satellites. It is also hoped that MW data (passive or active) may be used for the determination of soil moisture in the upper layer (i.e. few centimetres), as well as in deeper layers (i.e. up to several decimetres). If such information is available, a breakthrough in hydrological modelling could be expected.
Future research on data assimilation techniques, error properties in different climate regions, storm regimes and seasons, sources of error (i.e. satellite error) are also necessary to evaluate the quality and reliability of satellite data estimates across different regions and climate conditions. This task will require improved retrieval algorithms, uncertainty models and bias adjustment techniques.
For high resolution and reliable precipitation or rainfall data, future research should also focus on: (a) evaluation of extremes using ground reference measurements (e.g. ground radars and gauges) to understand to what extent one can observe extremes reliably; (b) multi-spectral and multi-satellite retrieval techniques; (c) improvements in GPM datasets by combining downscaling and artificial intelligence techniques; and (d) developing data assimilation techniques in order to integrate assimilation as a part of the modelling and algorithm development approach. For soil moisture estimation, future research is highly desired in integrating the space-borne measurements from multiple sensors, especially high-resolution SAR images, with radiometric data (e.g. SMAP mission). In the context of global climate modelling and regional weather forecasting, large-scale hydrological modelling is of increasing relevance. Here, runoff computations are of particular importance for calibrating such combined atmospheric and hydrological models, and the use of RS data for these purposes at large scales is gaining relevance (Schulz Citation1988). The GPM mission as an international network of satellites, which is available globally, is perhaps an extensive use of RS data for large-scale hydrological modelling. Furthermore, ground validation ensures that the satellite estimates are statistically accurate.
9 Conclusions
This article presents a critical review of NRCS-CN-based approaches for runoff estimation, coupled with RS and GIS applications. In view of the above discussion, it can be realized that in recent years the methodology has been improved by using SMA procedures, by incorporating AMA, storm duration and slope adjustment, as well as linear and nonlinear Ia–S relationships, by linking the dependency of initial abstraction on initial moisture amount, and by accounting for absolute retention, static and dynamic infiltration, ET, baseflow computation, etc. Despite several modifications, there is still need for further refinement in the critical components of the methodology, such as the SMA procedure for water balance study, simulation of peak of hydrograph, use of spatially distributed data, and GIS interfaces for initial data processing.
Thus, further research and investigation are needed in the context of model development and validation. Both RS and GIS techniques and the data derived using RS methods can and should play a pivotal role in future research. The capability of high spatial resolution satellites/sensors and missions for global data has been found to be advantageous in many hydrological applications, including the NRCS-CN methodology. Ongoing research for deriving high-accuracy parameter and input data, based on RS and GIS, can be expected to ensure more accurate runoff estimations. In particular, by 2020, there will be an unprecedented stream of concurrent observations from the GPM, SMAP, GRACE and GRACE-FO missions, and eventually the SWOT and ECO stress missions. As we look ahead, these satellites will soon provide routine global monitoring of all stocks and fluxes for continental- to regional-scale hydrology.
Acknowledgements
The authors are thankful to Associate Editor, Dr Andreas Efstratiadis, and the anonymous reviewers for their thoughtful comments, which helped to improve the article.
Disclosure statement
No potential conflict of interest was reported by the authors.
References
- Adler, R.F., et al., 1993. Estimation of monthly rainfall over Japan and surrounding waters from a combination of low-orbit microwave and geosynchronous IR data. Journal of Applied Meteorology, 32, 335–356. doi:10.1175/1520-0450(1993)0322.0.CO;2
- Ajmal, M., et al., 2015a. Investigation of SCS-CN and its inspired modified models for runoff estimation in South Korean watersheds. Journal of Hydro-Environment Research, 9 (4), 592–603. doi:10.1016/j.jher.2014.11.003
- Ajmal, M., et al., 2015b. Evolution of a parsimonious rainfall–runoff model using soil moisture proxies. Journal of Hydrology, 530, 623–633. doi:10.1016/j.jhydrol.2015.10.019
- Ajmal, M., et al., 2016b. Runoff estimation using the NRCS slope-adjusted curve number in mountainous watersheds. Journal of Irrigation and Drainage Engineering, 141, 04014058. doi:10.1061/(ASCE)IR.1943-4774.0000805
- Ajmal, M., Khan, T.K., and Kim, T.-W., 2016a. A CN-based ensembled hydrological model for enhanced watershed runoff prediction. Water, 8, 20. doi:10.3390/w8010020
- Akhondi, S., 2001. An investigation of curve number model in flood estimation using Geographical Information System. Thesis (MSc). Tarbiat Modares University, Tehran, Iran.
- Anagnostou, E.N., 2004. Overview of overland satellite rainfall estimation for hydro-meteorological applications. Surveys in Geophysics, 25 (5–6), 511–537. doi:10.1007/s10712-004-5724-6
- Andrews, R.G., 1954. The use of relative infiltration indices in computing runoff. In: V.P. Singh, ed. Rainfall–runoff relationship. Littleton, CO: Water Resources Publications.
- Arnold, J.G., et al., 1990. SWRRB-A basin scale simulation model for soil and water resources management. College Station, TX: A&M University Press.
- Aron, G., Miller, A.C., and Lakatos, D.F., 1977. Infiltration formula based on SCS curve numbers. Journal of Irrigation and Drainage Division ASCE, 103 (4), 419–427.
- Artan, G., et al., 2007. Users’ manual for the geospatial stream flow model (GeoSFM). Reston, VA: US Geological Survey, Open-File Report 2007–1440.
- Ashenafi, Y. and Hailu, D., 2014. Assessment of the use of remotely sensed rainfall products for runoff simulation in the Upper Blue Nile basin of Ethiopia. Journal of EEA, 31, 1–9.
- Ashish, P., et al., 2003. Estimation of run-off for agricultural watershed using SCS curve number and Geographical Information System. Available from: http://www.gisdevelopment.net.
- Aubert, D., Loumagne, C., and Oudin, L., 2003. Sequential assimilation of soil moisture and streamflow data in a conceptual rainfall runoff model. Journal of Hydrology, 280, 145–161. Available from http://www.wcc.nrcs.usda.gov/water/quality/common/techpaper/don1.pdf
- Awadallah, A.G., et al., 2016. Reliability assessment of water structures subject to data scarcity using the SCS-CN model. Hydrological Sciences Journal, 61 (4), 696–710. doi:10.1080/02626667.2015.1027709
- Babu, P.S. and Mishra, S.K., 2012. An improved SCS-CN inspired model. Journal of Hydrologic Engineering, ASCE, 17 (11), 1164–1172. doi:10.1061/(ASCE)HE.1943-5584.0000435
- Baltas, E.A., Dervos, N.A., and Mimikou, M.A., 2007. Determination of the SCS initial abstraction ratio in an experimental watershed in Greece. Hydrology and Earth System Sciences, 11, 1825–1829. doi:10.5194/hess-11-1825-2007
- Bartlett, M.S., et al., 2016. Beyond the SCS-CN method: A theoretical framework for spatially lumped rainfall–runoff response. Water Resources Research, 52, 4608–4627. doi:10.1002/2015WR018439
- Beck, H.E., et al., 2009. Improving curve number based storm runoff estimates using soil moisture proxies. IEEE Journal of Selected Topics in Applied Earth Observations and Remote Sensing, 2 (4), 250–259. doi:10.1109/JSTARS.2009.2031227
- Behrangi, A., et al., 2011. Hydrologic evaluation of satellite precipitation products over a mid-size basin. Journal of Hydrology, 397, 225–237. doi:10.1016/j.jhydrol.2010.11.043
- Bhanumurty, V., et al., 2003. Application of remote sensing and GIS in water resources development. Water and Energy International, 60 (2), 38–50.
- Bhaskar, N.R., Wesley, P.J., and Devulapalli, R.S., 1992. Hydrologic parameter estimation using geographic information systems. Journal of Water Resources Planning and Management, 118, 492–512. doi:10.1061/(ASCE)0733-9496(1992)118:5(492)
- Bhunya, P.K., et al., 2009. A simple conceptual model of sediment yield. Water Resources Management, 24 (8), 1697–1716. doi:10.1007/s11269-009-9520-4
- Bitew, M.M. and Gebremichael, M., 2011. Evaluation of satellite rainfall products through hydrologic simulation in a fully distributed hydrologic model. Water Resources Research, 47 (6), W06526. doi:10.1029/2010WR009917
- Blanchard, B.J., et al., 1981. Estimation of soil moisture with API algorithms and microwave emission. Water Resources Bulletin, 17, 767–774. doi:10.1111/j.1752-1688.1981.tb01296.x
- Bonta, J.V., 1997. Determination of watershed curve number using derived distributions. Journal of Irrigation and Drainage Engineering, ASCE, 123 (1), 28–36. doi:10.1061/(ASCE)0733-9437(1997)123:1(28)
- Bosznay, M., 1989. Generalization of SCS curve number method. Journal of Irrigation and Drainage Engineering, 115 (1), 139–144. doi:10.1061/(ASCE)0733-9437(1989)115:1(139)
- Brocca, L., et al., 2009b. Assimilation of observed soil moisture data in storm rainfall–runoff modelling. Journal of Hydrologic Engineering, ASCE, 14 (2), 153–165. doi:10.1061/(ASCE)1084-0699(2009)14:2(153)
- Brocca, L., et al., 2010. Improving runoff prediction through the assimilation of the ASCAT soil moisture product. Hydrology and Earth System Sciences, 14, 1881–1893. doi:10.5194/hess-14-1881-2010
- Brocca, L., et al., 2013. Detecting threshold hydrological response through satellite soil moisture data. Die Bodenkultur, 64, 7–12.
- Brocca, L., Melone, F., and Moramarco, T., 2008. On the estimation of antecedent wetness conditions in rainfall–runoff modelling. Hydrological Processes, 22, 629–642. doi:10.1002/(ISSN)1099-1085
- Brocca, L., Melone, F., and Moramarco, T., 2009a. Antecedent wetness conditions based on ERS scatterometer data in support to rainfall–runoff modeling. Journal of Hydrology, 364, 73–87. doi:10.1016/j.jhydrol.2008.10.007
- Brooner, W.G., et al., 1987. Remote sensing technologies and spatial data applications relevant to HEC programs and mission. Davis, CA: USACE, Final report, contract no. DACWO5-87-C-0012, US Army Corps of Engineers.
- Camici, S., et al., 2011. Design soil moisture estimation by comparing continuous and storm-based rainfall–runoff modelling. Water Resources Research, 47, W05527. doi:10.1029/2010WR009298
- Carsel, R., et al., 1997. PRZM 3.1 user’s manual, national exposure research lab, office of research and development. Athens, GA: US Environmental Protection Agency.
- Castillo, V., Gomez-Plaza, A., and Martınez-Mena, M., 2003. The role of antecedent soil water content in the runoff response of semiarid catchments: a simulation approach. Journal of Hydrology, 284 (1–4), 114–130. doi:10.1016/S0022-1694(03)00264-6
- Central Unit for Soil Conservation (Hydrology and Sedimentation), 1972. Handbook of hydrology. India: Soil Conservation Division, Ministry of Agriculture, Govt. of India.
- Chakraborti, A.K., 1993. Strategies for watershed management planning using remote sensing techniques. Journal of the Indian Society of Remote Sensing, 21 (2), 87–97. doi:10.1007/BF02996346
- Chatterjee, C., et al., 2001. Runoff curve number estimation for a basin using remote sensing and GIS. Asian Pacific Remote Sensing and GIS Journal, 14, 1–8.
- Chaudhary, A., Mishra, S.K., and Pandey, A., 2013. Experimental verification of effect of slope on runoff and curve numbers. Journal of Indian Water Resources Society, 33 (1), 40–46.
- Chen, C.L., 1982. Infiltration formulas by curve number procedure. Journal of Hydraulics Division, ASCE, 108 (7), 823–829.
- Choi, J.-Y., Engel, B.A., and Chung, H.W., 2002. Daily streamflow modelling and assessment based on the curve-number technique. Hydrological Processes, 16 (16), 3131–3150. doi:10.1002/(ISSN)1099-1085
- Chow, V.T., Maidment, D.R., and Mays, L.W., eds., 1988. Applied hydrology. New York: Mc-Graw-Hill.
- Collischonn, B., Collischonn, W., and Tucci, C.E.M., 2008. Daily hydrological modeling in the Amazon basin using TRMM rainfall estimates. Journal of Hydrology, 360 (1–4), 207–216. doi:10.1016/j.jhydrol.2008.07.032
- Coustau, M., et al., 2012. Flood modelling with a distributed event-based parsimonious rainfall–runoff model: case of the karstic Lez river catchment. Natural Hazards and Earth System Sciences, 12, 1119–1133. doi:10.5194/nhess-12-1119-2012
- Crow, W.T. and Ryu, D., 2009. A new data assimilation approach for improving runoff prediction using remotely-sensed soil moisture retrievals. Hydrology and Earth System Sciences, 13, 1–16. doi:10.5194/hess-13-1-2009
- Curtis, S., Crawford, T.W., and Lecce, S.A., 2007. A comparison of TRMM to other basin-scale estimates of rainfall during the 1999 Hurricane Floyd flood. Natural Hazards, 43, 187–198. doi:10.1007/s11069-006-9093-y
- DeVantier, B.A. and Feldman, A.D., 1993. Review of GIS applications in hydrologic modeling. Journal of Water Resources Planning and Management, ASCE, 119, 246–261. doi:10.1061/(ASCE)0733-9496(1993)119:2(246)
- Dhawale, A.W., 2013. Runoff estimation for Darewadi watershed using RS and GIS. International Journal of Recent Technology and Engineering, 1 (6), 46–50.
- Durbude, D.G., Jain, M.K., and Mishra, S.K., 2011. Long-term hydrologic simulation using SCS-CN based improved soil moisture accounting procedure. Hydrological Processes, 25 (4), 561–579. doi:10.1002/hyp.7789
- Ebert, E., Janowiak, J.E., and Kidd, C., 2007. Comparison of near-real-time precipitation estimates from satellite observations and numerical models. Bulletin of the American Meteorological Society, 88, 1–18. doi:10.1175/BAMS-88-1-47
- Ebrahimian, M., et al., 2012. Runoff estimation in step slope watershed with standard and slope-adjusted Curve Number Methods. Polish Journal Environmental Studies, 21 (5), 1191–1202.
- Efstratiadis, A., et al., 2014. Flood design recipes vs. reality: can predictions for ungauged basins be trusted? Natural Hazards Earth System Sciences, 14, 1417–1428. doi:10.5194/nhess-14-1417-2014
- Elhakeem, M. and Papanicolaou, A., 2009. Estimation of the runoff curve number via direct rainfall simulator measurements in the state of Iowa, USA. Water Resources Management, 23 (12), 2455–2473. doi:10.1007/s11269-008-9390-1
- Eli, R.N. and Lamont, S.J., 2010. Curve numbers and urban runoff modelling – application limitations. In: S. Struck and K. Lichten, eds.. Low impact development 2010: redefining water in the city (Proceedings of the 2010 International Low Impact Development Conference, San Francisco, CA, April 11-14, 2010). ASCE, 405–418. doi:10.1061/9780784410998
- Fan, F.L., et al., 2013. Estimating composite curve number using an improved SCS-CN method with remotely sensed variables in Guangzhou, China. Remote Sensing, 5, 1425–1438. doi:10.3390/rs5031425
- Foerster, J. and Milne-Home, W.A., 1995. Application of AGNPS to model nutrient generation rates under different farming management practices at the Gunnedah Research Centre catchment. Australian Journal of Experimental Agriculture, 35, 961–967. doi:10.1071/EA9950961
- Fry, L., et al., 2013. Identifying stream gage networks for maximizing the effectiveness of regional water balance modeling. Water Resources Research, 49 (5), 2689–2700. doi:10.1002/wrcr.20233
- Gabellani, S., et al., 2008. General calibration methodology for a combined Horton-SCS infiltration scheme in flash flood modelling. Natural Hazards Earth System Sciences, 8, 1317–1327. doi:10.5194/nhess-8-1317-2008
- Gajbhiye, S. and Mishra, S.K., 2012. Application of NRSC-SCS curve number model in runoff estimation using RS and GIS. In: IEEE International conference on advances in engineering, science and management (ICAESM 2012). IEEE, 346–352. Available from: http://ieeexplore.ieee.org/document/6216286/ [Accessed 20 May 2017].
- Gamage, S.H.P.W., Hewa, G.A., and Beecham, S., 2015. Modelling hydrological losses for varying rainfall and moisture conditions in South Australian catchments. Journal of Hydrology. Regional Studies, 4, 1–21.
- Garen, D. and Moore, D.S., 2005. Curve number hydrology in water quality modeling: uses, abuses, and future directions. Journal of the American Water Resources Association, 41 (2), 377–388. doi:10.1111/jawr.2005.41.issue-2
- Garg, V., et al., 2013. Assessment of the effect of slope on runoff potential of a watershed using NRCS-CN method. International Journal of Hydrology Science and Technology, 3, 141–159. doi:10.1504/IJHST.2013.057626
- Gassman, P.W., et al., 2007. The soil and water assessment tool: historical development, applications, and future research directions. Transaction of the American Society of Agriculture Engineering, 50 (4), 1211–1250.
- Geetha, K., et al., 2007. Modifications to SCS-CN method for long term hydrologic simulation. Journal of Irrigation and Drainage Engineering, ASCE, 133 (5), 475–486. doi:10.1061/(ASCE)0733-9437(2007)133:5(475)
- Golding, B.L., 1979. Discussion of Runoff curve number with varying site moisture” by Hawkins, R. H. Journal of Irrigation and Drainage Division, ASCE, 105 (IR4), 441–442.
- Gonzalez, A., Temimi, M., and Khanbilvardi, R., 2015. Adjustment to the curve number (NRCS-CN) to account for the vegetation effect on hydrological processes. Hydrological Sciences Journal, 60 (4), 591–605. doi:10.1080/02626667.2014.898119
- Goodrich, D.C., et al., 1994. Runoff simulation sensitivity to remotely sensed initial soil water content. Water Resources Research, 30, 1393–1405. doi:10.1029/93WR03083
- Gourley, D.C., et al., 2010. Impacts of polarimetric radar observations on hydrologic simulation. Journal of Hydrometeorology, 11, 781–796. doi:10.1175/2010JHM1218.1
- Green, W. H., and Ampt, G., 1911, Studies on soil physics, 1. The flow of air and water through soils. The Journal of Agricultural Science, 4, 1–24.
- Grimaldi, S., Petroselli, A., and Romano, N., 2013. Green-Ampt Curve-Number mixed procedure as an empirical tool for rainfall–runoff modelling in small and ungauged basins. Hydrological Processes, 27 (8), 1253–1264. doi:10.1002/hyp.9303
- Grimaldi, S., Petroselli, A., and Serinaldi, F., 2012a. A continuous simulation model for design-hydrograph estimation in small and ungauged watersheds. Hydrological Sciences Journal, 57, 1035–1051. doi:10.1080/02626667.2012.702214
- Grimaldi, S., Petroselli, A., and Serinaldi, F., 2012b. Design hydrograph estimation in small and ungauged watersheds: continuous simulation method versus event-based approach. Hydrological Processes, 26, 3124–3134. doi:10.1002/hyp.v26.20
- Grohmann, C.H., 2004. Morphometric analysis in geographic information systems: applications of free software GRASS and R. Computers & Geosciences, 30, 1055–1067. doi:10.1016/j.cageo.2004.08.002
- Grove, M., Harbor, J., and Engel, B., 1998. Composite vs. Distributed curve numbers: effects on estimates of storm runoff depths. Journal of the American Water resources Association, 34, 1015–1023. doi:10.1111/jawr.1998.34.issue-5
- Gumbo, B., et al., 2002. Coupling of digital elevation model and rainfall–runoff model in storm drainage network design. Physics and Chemistry of the Earth, Parts A/B/C, 27 (11–22), 755–764. doi:10.1016/S1474-7065(02)00063-3
- Gundalia, M. and Dholakia, M., 2014. Impact of monthly curve number on daily runoff estimation for Ozat catchment in India. Open Journal of Modern Hydrology, 4, 144–155. doi:10.4236/ojmh.2014.44014
- Gupta, P.K. and Panigrahy, S., 2008. Predicting the spatio-temporal variation of run-off generation in India using remotely sensed input and Soil Conservation Service curve number model. Current Science, 95 (11), 1580–1587.
- Harbor, J.M., 1994. A practical method for estimating the impact of land use change on surface runoff, groundwater recharge, and wetland hydrology. Journal of the American Planning Association, 60 (1), 95–108. doi:10.1080/01944369408975555
- Harris, A. and Hossain, F., 2008. Investigating the optimal configuration of conceptual hydrologic models for satellite-rainfall–based flood prediction. IEEE Geoscience and Remote Sensing Letters, 5 (3), 532–536. doi:10.1109/LGRS.2008.922551
- Hawkins, R.H., 1978. Runoff curve number with varying site moisture. Journal of Irrigation and Drainage Engineering, ASCE, 104 (4), 389–398.
- Hawkins, R.H., 1984. A comparison of predicted and observed runoff curve numbers. In: Proceeding of ASCE, Irrigation and Drainage Division, special conference. Flagstaff AZ: ASCE, 702–709.
- Hawkins, R.H., et al., 1985. Runoff probability storm depth and curve numbers. Journal of irrigation and drainage division. Asce, 111 (4), 330–340.
- Hawkins, R.H., 1993. Asymptotic determination of runoff curve numbers from data. Journal of Irrigation and Drainage Engineering, ASCE, 119 (2), 334–345. doi:10.1061/(ASCE)0733-9437(1993)119:2(334)
- Hawkins, R.H., et al., 2009. Curve number hydrology: state of the practice. Reston, VA: American Society of Civil Engineers, 106. ISBN 978-0-7844-1044-2.
- Hawkins, R.H., Hjelmfelt Jr., A.T., and Zevenbergen, A.W., 1985. Runoff probability, storm depth and curve numbers. Journal of Irrigation and Drainage Engineering, 111 (4), 330–340. doi:10.1061/(ASCE)0733-9437(1985)111:4(330)
- Hawkins, R.H. and Ward, T.J., 1998. Site and cover effects on event runoff, Jornada experimental range, New Mexico. Rangeland management and water resources. In: Proceedings from American Water Resources Association (AWRA). Reno, NV, 361–370.
- Hernández-Guzmán, R. and Ruiz-Luna, A., 2013. SARA – an enhanced curve number-based tool for estimating direct runoff. Journal of Hydroinformatics, 15, 881–887. doi:10.2166/hydro.2013.145
- Hjelmfelt Jr., A.T., 1980. Empirical investigation of curve number technique. Journal of Hydraulics and Engineering Division, ASCE, 106 (HY9), 1471–1476.
- Hjelmfelt Jr., A.T., 1991. Investigation of curve number procedure. Journal of hydraulic Engineering, 117 (6), 725–737. doi:10.1061/(ASCE)0733-9429(1991)117:6(725)
- Hjelmfelt, A.T., et al., 2001. Curve numbers, recent developments. In: XXIX IAHR Congress Proceedings, Beijing, China. IAHR: International Association for Hydro-Environment Engineering and Research.
- Hjelmfelt Jr., A.T., Kramer, K.A., and Burwell, R.E., 1982. Curve numbers as random variables. In: Proceedings of the international Symposium of Rainfall–Runoff Modeling. Littleton, CO: Water Resource Publications, 365–373.
- Hlaing, K.T., Haruyama, S., and Aye, M.M., 2008. Using GIS-based distributed soil loss modeling and morphometric analysis to prioritize watershed for soil conservation in Bago river basin of lower Myanmar. Frontiers of Earth Science in China, 2, 465–478. doi:10.1007/s11707-008-0048-3
- Hong, Y., et al., 2006. Uncertainty quantification of satellite precipitation estimation and Monte Carlo assessment of the error propagation into hydrologic response. Water Resources Research, 42, W08421. doi:10.1029/2005WR004398
- Hong, Y., et al., 2007. A first approach to global runoff simulation using satellite rainfall estimation. Water Resources Research, 43 (8), W080502. doi:10.1029/2006WR005739
- Hossain, F. and Lettenmaier, D.P., 2006. Flood prediction in the future: recognizing hydrologic issues in anticipation of the global precipitation measurement mission. Water Resources Research, 42, W11301. doi:10.1029/2006WR005202
- Huang, M., et al., 2006. A modification to the Soil Conservation Service curve number method for steep slopes in the Loess plateau of China. Hydrological Processes, 20 (3), 579–589. doi:10.1002/hyp.5925
- Huber, W.C., et al., 1976. Environmental resources management studies in the Kissimmee River basin. Gainsville, FL: Department of Environmental Engineering and Science, University of Florida, Report ENV-05–76-3.
- Huffman, G.J., et al., 2007. The TRMM multi-satellite precipitation analysis: quasi global, multiyear, combined sensor precipitation estimates at fine scale. Journal of Hydrometeorology, 8 (1), 38–55. doi:10.1175/JHM560.1
- Ishidaira, H., et al., 2008. Estimating the evolution of vegetation cover and its hydrological impact in the Mekong River basin in the 21st century. Hydrological Processes, 22, 1395–1405. doi:10.1002/hyp.6948
- Jacobs, J.H. and Srinivasan, R., 2005. Effects of curve number modification on runoff estimation using WSR-88D rainfall data in Texas watersheds. Journal of Soil and Water Conservation, 60 (5), 274–279.
- Jacobs, J.M., et al., 2004. SMEX02: field scale variability, time stability and similarity of soil moisture. Remote Sensing of Environment, 92, 436–446. doi:10.1016/j.rse.2004.02.017
- Jain, M.K., et al., 2006. Enhanced runoff curve number model incorporating storm duration and a non-linear Ia-S relation. Journal of Hydrologic Engineering, ASCE, 11 (6), 631–635. doi:10.1061/(ASCE)1084-0699(2006)11:6(631)
- Jain, M.K., Durbude, D.G., and Mishra, S.K., 2012. Improved CN-based long-term hydrologic simulation model. Journal of Hydrologic Engineering, ASCE, 17, 1204–1220. doi:10.1061/(ASCE)HE.1943-5584.0000592
- Jakeman, A. J., and Hornberger, G. M., 1993. How much complexity is warranted in a rainfall-runoff model? Water Resources Research, 29(8), 2637-2649.
- Jasrotia, A.S., Dhiman, S.D., and Aggarwal, S.P., 2002. Rainfall–runoff and soil erosion modeling using remote sensing and GIS technique – A case study of Tons watershed. Journal of the Indian Society of Remote Sensing, 30 (3), 167–180. doi:10.1007/BF02990649
- Javed, A., Khanday, M.Y., and Ahmed, R., 2009. Prioritization of sub-watersheds based on morphometric and land use analysis using remote sensing and GIS techniques. Journal of the Indian Society of Remote Sensing, 37, 261–274. doi:10.1007/s12524-009-0016-8
- Jena, S.K., et al., 2012. RS and Geographical Information System–based evaluation of distributed and composite curve number techniques. Journal of Hydrologic Engineering, 17 (11), 1278–1286. doi:10.1061/(ASCE)HE.1943-5584.0000651
- Jha, R.K., Mishra, S.K., and Pandey, A., 2014. Experimental verification of effect of slope, soil, and AMC of a fallow land on runoff curve number. Journal of Indian Water Resources Society, 34 (2), 40–47.
- Jiao, P., et al., 2015. Improved SCS-CN method based on storage and depletion of antecedent daily precipitation. Water Resources Management, 29 (13), 4753–4765. doi:10.1007/s11269-015-1088-6
- Jobard, I. and Desbois, M., 1994. Satellite estimation of the tropical precipitation using the METEOSAT and SSM/I data. Atmospheric Research, 34 (1–4), 285–298. doi:10.1016/0169-8095(94)90097-3
- Joycee, C.S.D. and Santhi, M.H., 2015. Assessment of surface runoff from sub basin of Kodayar using NRCS-CN model with GIS. Indian Journal of Science and Technology, 8 (13). doi:10.17485/ijst/2015/v8i13/60403
- Kabiri, R., Chan, A., and Bai, R., 2013. Comparison of SCS and Green-Ampt methods in surface runoff-flooding simulation for Klang watershed in Malaysia. Open Journal of Modern Hydrology, 3 (3), 102–114. doi:10.4236/ojmh.2013.33014
- Kadam, A.K., et al., 2012. Identifying potential rainwater harvesting sites of a semi-arid, basaltic region of western India, using SCS-CN method. Water Resources Management, 26 (9), 2537–2554. doi:10.1007/s11269-012-0031-3
- Kalma, J.D., McVicar, T.R., and McCabe, M., 2008. Estimating land surface evaporation: a review of methods using remotely sensed surface temperature data. Surveys in Geophysics, 29 (4–5), 421–469. doi:10.1007/s10712-008-9037-z
- Kamuju, N., 2015. Rainfall–runoff estimation and comparative analysis using advanced geospatial digital hydrological modelling tools, ArcCN-runoff and ArcSWAT. SSRG International Journal of Geo Informatics and Geological Science, 2 (4), 1–6.
- Kannan, N., et al., 2007. Development of a continuous soil moisture accounting procedure for curve number methodology and its behavior with different evapotranspiration methods. Hydrological Processes, 22, 2114–2121. doi:10.1002/hyp.6811
- Kannan, N., Santhi, C., and Arnold, J.G., 2008. Development of an automated procedure for estimation of the spatial variation of runoff in large river basins. Journal of Hydrology, 359, 1–15. doi:10.1016/j.jhydrol.2008.06.001
- Karimi, P. and Bastiaanssen, W.G.M., 2015. Spatial evapotranspiration, rainfall and land use data in water accounting – part 1: review of the accuracy of the remote sensing data. Hydrology and Earth System Sciences, 19, 507–532. doi:10.5194/hess-19-507-2015
- Kherde, R.V.K. and Sawant, P.H., 2013. Integrating geographical information systems (GIS) with hydrological modeling-applicability and limitations. International Journal of Engineering and Technology, 5 (4), 3374–3381.
- Kibbler, D.F., 1982. Recommended hydrologic procedures for computing urban runoff from small developing watersheds in Pennsylvania. University Park, PA: Institute for Research on Land and Water Resources, The Pennsylvania State University.
- Kidd, C. and Levizzani, V., 2011. Status of satellite precipitation retrievals. Hydrology and Earth System Sciences, 15, 1109–1116. doi:10.5194/hess-15-1109-2011
- Kim, N.W. and Lee, J., 2008. Temporally weighted average curve number method for daily runoff simulation. Hydrological Processes, 22 (25), 4936–4948. doi:10.1002/hyp.v22:25
- Kite, G.W. and Pietroniro, A., 1996. Remote sensing applications in hydrological modelling. Hydrological Sciences Journal, 41 (4), 563–591. doi:10.1080/02626669609491526
- Knisel, W.G., 1980 CREAMS: a field-scale model for chemical, runoff and erosion from agricultural management systems. Washington, DC: US Department of Agriculture, USDA Conservation Research Report. No. 26.
- Korkalainen, T.H.J., Lauren, A.M., and Kokkonen, T.S., 2007. A GIS based analysis of catchment properties within a drumlin field. Boreal Environment Research, 12, 489–500.
- Krysanova, V., Wechsung, F., and Arnold, J.G., 2000. SWIM user manual. Potsdam, Germany: Potsdam Institute for Climate Impact Research (PIK).
- Kumar, D.N. and Reshmidev, T.V., 2013. Remote sensing applications in water resources. Journal of the Indian Institute of Science, 93 (2), 163–187.
- Lal, M., Mishra, S.K., and Pandey, A., 2015. Physical verification of the effect of land features and antecedent moisture on runoff curve number. Catena, 133, 318–327. doi:10.1016/j.catena.2015.06.001
- Lantz, D. G., and Hawkins, R. H., 2001. Discussion of long-term hydrologic impact of urbanization: a tale of two models. Journal of Water Resources Planning and Management, ASCE. 127, 13–19. doi:10.1061/(ASCE)0733
- Leonard, R.A., Knisel, W.G., and Still, D.A., 1987. GLEAMS: groundwater loading effects of agricultural management systems. Transactions of the ASAE, 30 (5), 1403–1418. doi:10.13031/2013.30578
- Lim, K.J., et al., 2006. Effects of initial abstraction and urbanization on estimated runoff using CN technology. Journal of the American Water Resources Association, 42, 629–643. doi:10.1111/j.1752-1688.2006.tb04481.x
- Lin, C., Greenwald, D., and Banin, A., 2003. Temperature dependence of infiltration rate during large scale water recharge into soils. Soil Science Society of America Journal, 67, 487–493. doi:10.2136/sssaj2003.4870
- Lyon, S.W., et al., 2004. Using a topographic index to distribute variable source area runoff predicted with the SCS curve-number equation. Hydrological Processes, 18, 2757–2771. doi:10.1002/hyp.v18:15
- Maidment, D.R., 1996. GIS and hydrological modeling - an assessment of progress. In: Paper presented at The Third International Conference on GIS and Environmental Modeling, Santa Fe, NM. Available from: maidment/GISHydro/meetings/santafe/santafe.htm.
- Mancini, M. and Rosso, R., 1989. Using GIS to assess spatial variability of SCS curve number at the basin scale. Wallingford, UK:International Association of Hydrological Sciences, New direction for surface water modeling, IAHS Publ. No. 181, 435–444.
- Massari, C., et al., 2014. Using globally available soil moisture indicators for flood modelling in Mediterranean catchments. Hydrology and Earth System Sciences, 18, 839–853. doi:10.5194/hess-18-839-2014
- Massari, C., et al., 2015. The use of H-SAF soil moisture products for operational hydrology: flood modelling over Italy. Hydrology, 2, 2–22. doi:10.3390/hydrology2010002
- McCuen, R.H., 1989. Hydrologic analysis and design. Englewood Cliffs, NJ: Prentice Hall.
- McCuen, R.H., 2002. Approach to confidence interval estimation for curve numbers. Journal of Hydrologic Engineering, 7 (1), 43–48. doi:10.1061/(ASCE)1084-0699(2002)7:1(43)
- Mednick, A.C., 2010. Does soil data resolution matter? State soil geographic database versus soil survey geographic database in rainfall–runoff modeling across Wisconsin. Journal of Soil and Water Conservation, 65 (3), 190–199. doi:10.2489/jswc.65.3.190
- Meier, P., Frömelt, A., and Kinzelbach, W., 2011. Hydrological real-time modelling in the Zambezi river basin using satellite-based soil moisture and rainfall data. Hydrology and Earth System Sciences, 15 (3), 999–1008. doi:10.5194/hess-15-999-2011
- Menziani, M., et al., 2001. TDR soil moisture measurements at the Lago Maggiore MAP target area: preliminary results. Physics and Chemistry of the Earth, Part B: Hydrology, Oceans and Atmosphere, 26 (5–6), 431–436. doi:10.1016/S1464-1909(01)00031-4
- Merritt, W.S., Letcher, R.A., and Jakeman, A.J., 2003. A review of erosion and sediment transport models. Environmental modelling & Software, 18, 761–799. doi:10.1016/S1364-8152(03)00078-1
- Merz, B. and Plate, E.J., 1997. An analysis of the effects of spatial variability of soil and soil moisture on runoff. Water Resources Research, 33 (12), 2909–2922. doi:10.1029/97WR02204
- Michel, C., Vazken, A., and Charles, P., 2005. Soil Conservation Service curve number method: how to mend a wrong soil moisture accounting procedure? Water Resources Research, 41 (2), W02011. doi:10.1029/2004WR003191
- Milewski, A., et al., 2009. A remote sensing solution for estimating runoff and recharge in arid environments. Journal of Hydrology, 373 (1–2), 1–14. doi:10.1016/j.jhydrol.2009.04.002
- Mishra, A., et al., 2009. Study of intense rainfall events over India using Kalpana-IR and TRMM-precipitation radar observations. Current Science, 97, 689–695.
- Mishra, S.K., et al., 1998. An SCS-CN-based long-term daily flow simulation model a hilly catchment. In: Proc. of international symposium on hydrology of ungauged streams in hilly regions for small hydro power development. New Delhi, India: AHEC, 59–81.
- Mishra, S.K., et al., 2005. Long-term hydrologic simulation using storage and source area concepts. Hydrological Processes, 19 (14), 2845–2861. doi:10.1002/hyp.5735
- Mishra, S.K., et al., 2006a. An improved Ia–S relation incorporating antecedent moisture in SCS-CN methodology. Water Resources Management, 20, 643–660. doi:10.1007/s11269-005-9000-4
- Mishra, S.K., et al., 2006b. SCS-CN based modeling of sediment yield. Journal of Hydrology, 324, 301–322. doi:10.1016/j.jhydrol.2005.10.006
- Mishra, S.K., et al., 2008a. Comparison of AMC-dependent CN-conversion formulae. Water Resources Management, 22, 1409–1420. doi:10.1007/s11269-007-9233-5
- Mishra, S.K., et al., 2008b. A rain duration and modified AMC dependent SCS-CN procedure for long duration rainfall– runoff events. Water Resources Management, 22 (7), 861–876. doi:10.1007/s11269-007-9196-6
- Mishra, S.K., et al., 2014a. Experimental verification of the effect of slope and landuse on SCS runoff curve number. Water Resources Management, 28 (11), 3407–3416. doi:10.1007/s11269-014-0582-6
- Mishra, S.K., et al., 2014b. Relationship between runoff curve number and PET. Journal of Hydrologic Engineering, 19 (2), 355–365. doi:10.1061/(ASCE)HE.1943-5584.0000780
- Mishra, S.K., Babu, P.S., and Singh, V.P., 2007. SCS-CN method revisited. In: V.P. Singh, ed. Advances in hydraulics and hydrology. Colorado: Water Resources Publication (WRP), 36 p.
- Mishra, S.K., Jain, M.K., and Singh, V.P., 2004. Evaluation of the SCS-CN-based model incorporating antecedent moisture. Water Resources Management, 18 (6), 567–589. doi:10.1007/s11269-004-8765-1
- Mishra, S.K. and Singh, V.P., 1999. Another look at SCS-CN method. Journal of Hydrologic Engineering, ASCE, 4 (3), 257–264. doi:10.1061/(ASCE)1084-0699(1999)4:3(257)
- Mishra, S.K. and Singh, V.P., 2002a. SCS-CN method: part-I: derivation of SCS-CN based models. Acta Geophysica Polonica, 50 (3), 457–477.
- Mishra, S.K. and Singh, V.P., 2003a. Derivation of SCS-CN parameter S from linear Fokker-Planck equation. Acta Geophysica Polonica, 51 (2), 180–202.
- Mishra, S.K. and Singh, V.P., 2003b. Soil Conservation Service curve number (SCS-CN) methodology. Dordrecht, The Netherlands: Kluwer.
- Mishra, S.K. and Singh, V.P., 2004a. Long-term hydrological simulation based on the Soil Conservation Service curve number. Hydrological Processes, 18 (7), 1291–1313. doi:10.1002/(ISSN)1099-1085
- Mishra, S.K. and Singh, V.P., 2004b. Validity and extension of the SCS-CN method for computing infiltration and rainfall–excess rates. Hydrological Processes, 18 (17), 3323–3345. doi:10.1002/(ISSN)1099-1085
- Mishra, S.K. and Singh, V.P., 2006. A relook at NEH-4 curve number data and antecedent moisture condition criteria. Hydrological Processes, 20 (13), 2755–2768. doi:10.1002/(ISSN)1099-1085
- Mishra, S.K. and Singh, V.P., 2002b. SCS-CN based hydrologic simulation package. In: V.P. Singh and D.K. Frevert, eds. Mathematical models in small watershed hydrology. Littleton, CO: Water Resources Publications, 391–464.
- Mockus, V., 1949. Estimation of total (peak rates of) surface runoff for individual storms. Exhibit A of Appendix B, Interim Survey Rep. Washington, DC: Grand (Neosho) River Watershed, USDA.
- Moglen, G.E., 2000. Effect of orientation of spatially distributed curve number in runoff calculations. Journal of American Water Resources Association, 36 (6), 1391–1400. doi:10.1111/j.1752-1688.2000.tb05734.x
- Musgrave, G.W., 1955. How much of the rain enters the soil?, Yearbook of agriculture 1955: water. Washington, DC: USDA.
- Nagarajan, N. and Poongothai, S., 2012. Spatial mapping of runoff from a watershed using SCS-CN method with remote sensing and GIS. Journal of Hydrologic Engineering, 17 (11), 1268–1277. doi:10.1061/(ASCE)HE.1943-5584.0000520
- Narain, S., et al., 2015. SCS-CN-based simulation of pollutants removal. Journal of Civil and Environmental Engineering, 5 (2), 168.
- Narayana, V.V.D., 1993. Soil and water conservation research in India. New Delhi, India: Indian Council of Agricultural Research.
- Natural Resources Conservation Service (NRCS), 2001. National engineering handbook Section 4: hydrology. Washington, DC: USDA.
- Nayak, T., Verma, M.K., and Hema Bindu, S., 2012. SCS curve number method in Narmada basin. International Journal of Geomatics and Geosciences, 3 (1), 219–228.
- Nayak, T.R. and Jaiswal, R.K., 2003. Rainfall–runoff modeling using satellite data and GIS for Bebas River in Madhya Pradesh. Journal of Institution of Engineers, 84, 47–50.
- Neitsch, S.L., et al., 2002. Soil and water assessment tool (SWAT): theoretical documentation, version 2000. College Station, TX: Texas Water Resources Institute, TWRI Report TR-191.
- Njoku, E.G., et al., 2003. Soil moisture retrieval from AMSR-E. IEEE Transactions on Geoscience and Remote Sensing, 41 (2), 215–229. doi:10.1109/TGRS.2002.808243
- Nourani, V., Singh, V.P., and Delafrouz, H., 2009. Three geomorphological rainfall–runoff models based on the linear reservoir concept. Catena, 76, 206–214. doi:10.1016/j.catena.2008.11.008
- Ojha, C., 2012. Simulating turbidity removal at a river bank filtration site in India using SCS-CN approach. Journal of Hydrologic Engineering, ASCE, 17 (11), 1240–1244. doi:10.1061/(ASCE)HE.1943-5584.0000498
- Owens, J. S., et al., 2003. Cover-runoff equations to improve simulation of runoff in pasture growth models. Australian Journal Of Soil Research, 41, doi:10.1071/SR03047-0004-9573/03/0801467
- Pandey, A., et al., 2003. Estimation of runoff for agricultural watershed using SCS curve number and Geographic Information System. Available from: htpp://www.GISdevelopment.net.
- Pandey, A. and Sahu, A.K., 2002. Generation of curve number using remote sensing and geographic information system. In: Water resources, Map India Conference. Available from: http://[email protected]/.
- Pandit, A. and Gopalakrishnan, G., 1996. Estimation of annual storm runoff coefficients by continuous simulation. Journal of Irrigation and Drainage Engineering, ASCE, 122 (4), 211–220. doi:10.1061/(ASCE)0733-9437(1996)122:4(211)
- Pankaj, A. and Kumar, P., 2009. GIS-based morphometric analysis of five major sub-watersheds of Song River, Dehradun District, Uttarakhand with special reference to landslide incidences. Journal of the Indian Society of Remote Sensing, 37, 157–166. doi:10.1007/s12524-009-0007-9
- Patil, J.P., et al., 2008. Evaluation of modified CN methods for watershed runoff estimation using a GIS-based interface. Biomass Engineering, 100, 137–146.
- Petty, G. and Krajewski, W.F., 1996. Satellite estimation of precipitation over land. Hydrological Sciences Journal, 41, 433–451. doi:10.1080/02626669609491519
- Pfister, L., et al., 2003. Predicting peak discharge through empirical relationships between rainfall, groundwater level and basin humidity in the Alzette River basin Grand-Duchy of Luxembourg. Journal of Hydrology and Hydromechanics, 51 (3), 210–220.
- Plummer, A. and Woodward, D.E., 2002. The origin and derivation of Ia/S in the runoff curve number system. Available from: https://www.nrcs.usda.gov/Internet/FSE_DOCUMENTS/stelprdb1044210.pdf
- Ponce, V.M., 1989. Engineering hydrology: principles and practice. Englewood Cliffs, NJ: Prentice-Hall.
- Ponce, V.M. and Hawkins, R.H., 1996. Runoff curve number: has it reached maturity? Journal of Hydrologic Engineering, ASCE, 1 (1), 11–19. doi:10.1061/(ASCE)1084-0699(1996)1:1(11)
- Prakash, S., et al., 2009. Combined use of microwave and IR data for the study of Indian monsoon rainfall. In: ISPRS archives XXXVIII-8/W3 workshop proceedings: impact of climate change on agriculture. Ahmedabad, India, 227–230.
- Ragan, R.M. and Jackson, T.M., 1980. Runoff synthesis using Landsat and SCS model. Journal of Hydraulics Division, ASCE, 106 (5), 667–678.
- Rallison, R.E., 1980. Origin and evaluation of the SCS runoff equation. In: Proceeding of symposium Watershed Management. Idaho: ASCE, 912–924.
- Ramakrishnan, D., Bandyopadhyay, A., and Kusuma, K.N., 2009. SCS-CN and GIS based approach for identifying potential water harvesting sites in the Kali watershed, Mahi river basin, India. Journal of Earth System Sciences, 118 (4), 355–368. doi:10.1007/s12040-009-0034-5
- Ramasastri, K.S. and Seth, S.M., 1985. Rainfall–runoff relationships. Roorkee, UP: National Institute of Hydrology, Rep. RN – 20.
- Randusová, B., et al., 2015. Comparison of CN estimation approaches. International Journal of Engineering Research and Science, 1 (7), 34–40.
- Rietz, P.D. and Hawkins, R.H., 2000. Effects of landuse on runoff curve number. In: Watershed management 2000, ASCE, Proceedings of the Watershed Management Symposium. Fort Collins, CO, CD ROM, 11 p.
- Ritchie, J.C., Zimba, P.V., and Everitt, J.H., 2003. Remote sensing techniques to assess water quality. Photogrammetric Engineering & Remote Sensing, 69, 695–704. doi:10.14358/PERS.69.6.695
- Roberts, G. and Crane, S.B., 1997. Temporal variations in near surface soil moisture at two contrasting sites in the Wye catchment and their control on storm streamflow generation. Hydrology and Earth System Sciences, 1 (3), 453–461. doi:10.5194/hess-1-453-1997
- Romero, P., et al., 2007. Curve number values for oliveorchards under different soil management. Journal of Soil and Water Conservation, 71 (6), 1758–1769.
- Rosenthal, W.D., Harlan, J.C., and Blanchard, B.J., 1982. Case study: estimating antecedent precipitation index from Head Capacity Mapping Mission day thermal infrared data. IAHS Bulletin, 27 (4), 415–426.
- Sagintayev, Z., et al., 2011. A remote sensing contribution to hydrologic modeling in arid and inaccessible watersheds, Pishin Lora basin, Pakistan. Hydrological Processes, 26, 85–99. doi:10.1002/hyp.8114
- Sahu, R.K., et al., 2007. An advanced soil moisture accounting procedure for SCS curve number method. Hydrological Processes, 21 (21), 2827–2881. doi:10.1002/hyp.6503
- Sahu, R.K., Mishra, S.K., and Eldho, T.I., 2010. An improved AMC-coupled runoff curve number model. Hydrological Processes, 24 (20), 2834–2839. doi:10.1002/hyp.v24:20
- Sahu, R.K., Mishra, S.K., and Eldho, T.I., 2012a. Improved storm duration and antecedent moisture condition coupled SCS-CN concept-based model. Journal of Hydrologic Engineering, ASCE, 17 (11), 1173–1179. doi:10.1061/(ASCE)HE.1943-5584.0000443
- Sahu, R.K., Mishra, S.K., and Eldho, T.I., 2012b. Performance evaluation of modified versions of SCS curve number method for two watersheds of Maharashtra, India. ISH Journal of Hydraulic Engineering, 18 (1), 27–36. doi:10.1080/09715010.2012.662425
- Sandholt, I., et al., 2003. Integration of earth observation data in distributed hydrological models: the Senegal River basin. Canadian Journal of Remote Sensing, 29 (6), 701–710. doi:10.5589/m03-045
- Savvidou, E., et al., 2016. A curve number approach to formulate hydrological response units within distributed hydrological modelling. Hydrology and Earth System Sciences Discussions, 1–34. doi:10.5194/hess-2016-627
- Schneider, L.E. and McCuen, R.H., 2005. Statistical guidelines for curve number generation. Journal of Irrigation and Drainage Engineering, 131 (3), 282–290. doi:10.1061/(ASCE)0733-9437(2005)131:3(282)
- Schroeder, P.R., et al., 1994. The hydrologic evaluation of landfill performance (HELP) model: engineering documentation for version 3. Washington, DC: US Environmental Protection Agency Office of Research and Development, EPA/600/R-94/168b.
- Schultz, G.A., 1988. Remote sensing in hydrology. Journal of Hydrology, 100 (1–3), 239–265. doi:10.1016/0022-1694(88)90187-4
- Schultz, G.A., 1996. Remote sensing applications to hydrology: runoff. Hydrological Sciences Journal, 41, 453–475. doi:10.1080/02626669609491520
- SCS, 1956, 1964, 1971, 1972, 1985, 1993, 2004. National engineering handbook section 4: hydrology, chapter 4. Soil Conservation Service. Washington, DC: USDA.
- Shaffer, M.J., Halvorson, A.D., and Pierce, F.J., 1991. Nitrate leaching and economic analysis package (NLEAP): model description and application. In: R.F. Follett, et al., eds. Managing nitrogen for groundwater quality and farm profitability. Madison, WI: Soil Science Society of America, 285–322.
- Sharma, K.D. and Singh, S., 1992. Runoff estimation using Landsat Thematic Mapper data and the SCS model. Hydrological Sciences Journal, 37 (1), 39–52. doi:10.1080/02626669209492560
- Sharma, T., et al., 2001. Hydrologic response of a watershed to landuse changes: A remote sensing and GIS approach. International Journal of Remote Sensing, 22 (11), 2095–2108. doi:10.1080/01431160117359
- Sharpley, A.N. and Williams, J.R., 1990. EPIC—erosion/productivity impact calculator: 1. Model documentation. Washington, DC: US Government Printing Office, USDA Technical Bulletin No. 1768.
- Sherman, L.K., 1949. Streamflow from rainfall by the unit graph method. Engineering News Record, 108, 501–505.
- Sherman, L.K., 1942. Hydrograph of runoff. In: O.E. Meinzer, ed. Physics of the earth, vol. 9, hydrology. New York: McGraw-Hill.
- Shi, Z.-H., et al., 2009. Research on the SCS-CN initial abstraction ratio using rainfall–runoff event analysis in the Three Gorges area, China. Catena, 77, 1–7. doi:10.1016/j.catena.2008.11.006
- Shirmohammadi, A., et al., 1997. Evaluation of curve number procedures to predict runoff in GLEAMS. Journal of the American Water Resources Association, 33, 1069–1076. doi:10.1111/jawr.1997.33.issue-5
- Shrestha, R.K., Mishra, S.K., and Pandey, A., 2013. Curve number affected by slope of experimental plot having maize crop. Journal of Indian Water Resources Society, 33 (2), 42–50.
- Sindhu, D., Shivakumar, B.L., and Ravikumar, A.S., 2013. Estimation of surface runoff In Nallur Amanikere watershed using SCS-CN method. International Journal of Research in Engineering and Technology, 2 (13), 404–409. doi:10.15623/ijret.2013.0213076
- Singh, P., Gupta, A., and Singh, M., 2014. Hydrological inferences from watershed analysis for water resource management using remote sensing and GIS techniques. The Egyptian Journal of Remote Sensing and Space Science, 17, 111–121. doi:10.1016/j.ejrs.2014.09.003
- Singh, P.K., et al., 2008. A sediment graph model based on SCS-CN method. Journal of Hydrology, 349, 244–255. doi:10.1016/j.jhydrol.2007.11.004
- Singh, P.K., et al., 2010. An updated hydrological review on recent advancements in soil conservation service curve-number technique. Journal of Water and Climate Change, 1 (2), 118–134. doi:10.2166/wcc.2010.022
- Singh, P.K., et al., 2013. SCS-CN based quantification of potential of rooftop catchments and computation of ASRC for rainwater harvesting. Water Resources Management, 27, 2001–2012. doi:10.1007/s11269-013-0267-6
- Singh, P.K., et al., 2015. Development of a modified SMA based MSCS-CN model for runoff estimation. Water Resources Management, 29 (11), 4111–4127. doi:10.1007/s11269-015-1048-1
- Singh, V.P., 1992. Elementary hydrology. Englewood Cliffs, NJ: Prentice Hall.
- Skaugen, T., Peerebom, I.O., and Nilsson, A., 2015. Use of a parsimonious rainfall–runoff model for prediction hydrological response in ungauged basins. Hydrological Processes, 29, 1999–2013. doi:10.1002/hyp.10315
- Smith, R.E., 1978. A proposed infiltration model for use in simulation of field-scale watershed hydrology. In: Presented at U.S. Dep. Agric. Agric. Res. Serv. Nonpoint Pollution Modeling Workshop.
- Smith, R.E., 1997. Discussion of runoff curve number: has it reached maturity? by Ponce, V.M., and Hawkins, R.H. Journal of Hydrologic Engineering, 2 (3), 145–148. doi:10.1061/(ASCE)1084-0699(1997)2:3(145)
- Smith, R.E. and Williams, J.R., 1980. Simulation of surface water hydrology. In: W.G. Knisel, eds. CREAMS: a field scale model for chemicals. Conservation Research Report No.26. Washington, DC: USDA, 643.
- Sobhani, G., 1975. A review of selected small watershed design methods for possible adoption to Iranian conditions. MS Thesis, Utah State University, Logan, UT.
- Sorooshian, S., et al., 2000. Evaluation of PERSIANN system satellite-based estimates of tropical rainfall. Bulletin of the American Meteorological Society, 81, 2035–2046. doi:10.1175/1520-0477(2000)0812.3.CO;2
- Soulis, K.X., et al., 2009. Investigation of the direct runoff generation mechanism for the analysis of the SCS-CN method applicability to a partial area experimental watershed. Hydrology and Earth System Sciences, 13, 605–615. doi:10.5194/hess-13-605-2009
- Soulis, K.X. and Valiantzas, J.D., 2012. SCS-CN parameter determination using rainfall–runoff data in heterogeneous watersheds -the two-CN system approach. Hydrology and Earth System Sciences, 16, 1001–1015. doi:10.5194/hess-16-1001-2012
- Soulis, K.X. and Valiantzas, J.D., 2013. Identification of the SCS-CN parameter spatial distribution using rainfall–runoff data in heterogeneous watersheds. Water resources Management, 27, 1737–1749. doi:10.1007/s11269-012-0082-5
- Springer, E.P., et al., 1980. Curve numbers from watershed data. In: Symposium on “Watershed Management”. New York, NY: Boios, ID: ASCE, 938–950.
- Srinivasan, R. and Engel, B.A., 1994. A spatial decision support system for assessing agricultural nonpoint source pollution. Journal of the American Water Resources Association, 30 (3), 441–452. doi:10.1111/jawr.1994.30.issue-3
- Steefel, C.I. and Van Cappellen, P., 1998. Reactive transport modeling of natural systems. Journal of Hydrology, 209, 1–7.
- Steenhuis, T.S., et al., 1995. SCS runoff equation revisited for variable-source runoff areas. Journal of Irrigation and Drainage Engineering, ASCE, 121 (3), 234–238. doi:10.1061/(ASCE)0733-9437(1995)121:3(234)
- Still, D.A. and Shih, S.F., 1984. Using Landsat data to estimate runoff. ASAE Summer Meeting. Paper no. 80-2018. St. Joseph, MI.
- Still, D.A. and Shih, S.F., 1985. Using Landsat to classify land use for assessing the basin-wide runoff index. Journal of American Water Resources Association, 21 (6), 931–940. doi:10.1111/j.1752-1688.1985.tb00188.x
- Still, D.A. and Shih, S.F., 1991. Satellite data and geographic information system in runoff curve number prediction. In: Proceeding of the International Conference on Computer Application in Water Resources, Taipei, Taiwan, 1014–1021.
- Stuebe, M.M. and Johnston, D.M., 1990. Runoff volume estimation using GIS technique. Water Resources Bulletin, 26 (4), 611–620. doi:10.1111/j.1752-1688.1990.tb01398.x
- Su, F.G., Hong, Y., and Lettenmaier, D.P., 2008. Evaluation of TRMM multisatellite precipitation analysis (TMPA) and its utility in hydrologic prediction in the La Plata Basin. Journal of Hydrometeorology, 9 (4), 622–640. doi:10.1175/2007JHM944.1
- Suresh., Babu, P., and Mishra, S. K., 2012. Improved SCS-CN inspired model. Journal of Hydrologic Engineering, 17 (11), 1164-1172. doi: 10.1061/(ASCE)HE.1943-5584.0000435
- Svoboda, A., 1991. Changes in flood regime by use of the modified curve number method. Hydrological Sciences Journal, 36 (5), 461–470. doi:10.1080/02626669109492531
- Tauer, W. and Humborg, G., 1992. Runoff irrigation in the Sahel zone: remote sensing and GIS for determining potential sites. Technical Centre for Agriculture and Rural Co-operation. Netherlands: GIS publishers.
- Tedela, N.H., et al., 2012. Runoff curve number for 10 small forested watersheds in the mountains of the Eastern United States. Journal of Hydrologic Engineering, 17, 1188–1198. doi:10.1061/(ASCE)HE.1943-5584.0000436
- Tejaswini, N.B., Shetty, A., and Hegde, V.S., 2011. Land use scenario analysis and prediction of runoff using SCS‐CN method: A case study from the Gudgudi tank, Haveri District, Karnataka, India. International Journal of Earth Sciences and Engineering, 4 (5), 845–853.
- Temimi, M., et al., 2010. A combination of remote sensing data and topographic attributes for the spatial and temporal monitoring of soil wetness. Journal of Hydrology, 388 (1–2), 28–40. doi:10.1016/j.jhydrol.2010.04.021
- Thakuriah, G. and Ranjan, S., 2014. Estimation of surface runoff using NRCS curve number procedure in Buriganga Watershed, Assam, India – A geospatial approach. International Research Journal of Earth Sciences, 2 (5), 1–7.
- Tischler, M., et al., 2007. A GIS framework for surface-layer soil moisture estimation combining satellite radar measurements and land surface modeling with soil physical property estimation. Environmental Modelling & Software, 22, 891–898. doi:10.1016/j.envsoft.2006.05.022
- Tiwari, K.N., et al., 1997. Watershed parameters extraction using GIS and remote sensing for Hydrologic modelling. Journal of Asian-Pacific Remote Sensing and GIS, 10 (1), 43–52.
- Todd, M., et al., 2001. A combined satellite infrared and passive microwave technique for estimation of small-scale rainfall. Journal of Atmospheric and Oceanic Technology, 18, 742–755. doi:10.1175/1520-0469(2001)0582.0.CO;2
- Topno, A., Singh, A.K., and Vaishya, R.C., 2015. SCS-CN Runoff estimation for Vindhyachal region using remote sensing and GIS. International Journal of Advanced Remote Sensing and GIS, 4 (1), 1214–1223. doi:10.23953/cloud.ijarsg.108
- Tramblay, Y., et al., 2010. Assessment of initial soil moisture conditions for event-based rainfall–runoff modelling. Journal of Hydrology, 380 (3–4), 305–317. doi:10.1016/j.jhydrol.2009.11.006
- Tramblay, Y., et al., 2012. Estimation of antecedent wetness conditions for flood modelling in northern Morocco. Hydrology and Earth System Sciences, 16, 4375–4386. doi:10.5194/hess-16-4375-2012
- Tripathi, M.P., et al., 2002. Runoff modelling of a small watershed using satellite data and GIS. Journal of the Indian Society of Remote Sensing, 30 (1–2), 39–52. doi:10.1007/BF02989975
- Tyagi, J.V., et al., 2008. SCS-CN based time distributed sediment yield model. Journal of Hydrology, 352 (3–4), 388–403. doi:10.1016/j.jhydrol.2008.01.025
- USDA, 1986. Urban hydrology for small watersheds. Washington, DC: United States Department of Agriculture, Technical Release 55 (TR-55).
- Van Mullem, J., et al., 2002. Runoff curve number method: beyond the handbook. In: Second Federal Interagency Hydrologic Modeling Conference, Las Vegas, NV, 1–10. Available from: https://acwi.gov/hydrology/mtsconfwkshops/conf_proceedings/second_fihmc_nevada.pdf
- Van Mullem, J.A., 1991. Runoff and peak discharges using Green-Ampt infiltration model. Journal of Hydraulic Engineering, ASCE, 117 (3), 354–370. doi:10.1061/(ASCE)0733-9429(1991)117:3(354)
- Vicente, G.A. and Anderson, J.R., 1994. A new rain retrieval technique that combines geosynchronous IR and MW polar orbit data for hourly rainfall estimates. Case study: Kwajalein and TOGA-COARE. In: American Meteorological Society, ed. Proceeding of the 7th conference on satellite meteorology and oceanography. AMS, 34–37.
- Wagner, W., Lemoine, G., and Rott, H., 1999. A method for estimating soil moisture from ERS scatterometer and soil data. Remote Sensing of Environment, 70, 191–207. doi:10.1016/S0034-4257(99)00036-X
- Walter, M.T. and Stephen, B.S., 2005. Discussion of: “Curve number hydrology in water quality modeling: uses, abuses, and future directions” by David C. Garen and Daniel S. Moore. Journal of American Water Resources Association, 41 (6), 1491–1492. doi:10.1111/jawr.2005.41.issue-6
- Wang, S.G., et al., 2011. Estimation of surface soil moisture and roughness from multi-angular ASAR imagery in the Watershed Allied Telemetry Experimental Research (WATER). Hydrology and Earth System Sciences, 15 (5), 1415–1426. doi:10.5194/hess-15-1415-2011
- Wang, T., et al., 2012a. Climate WNA – high-resolution spatial climate data for western North America. Journal of Applied Meteorology and Climatology, 51, 16–29. doi:10.1175/JAMC-D-11-043.1
- Wang, X., et al., 2008. Simulation of an agricultural watershed using an improved curve number method in SWAT. Transactions of the American Society of Agricultural and Biological Engineering, 51 (4), 1323–1339.
- Wang, X., et al., 2010. Simulated wetland conservationrestoration effects on water quantity and quality at watershed scale. Journal of Environmental Management, 91, 1511–1525. doi:10.1016/j.jenvman.2010.02.023
- Wang, X., Liu, T., and Yang, W., 2012b. Development of a robust runoff-prediction model by fusing the rational equation and a modified SCS-CN method. Hydrological Sciences Journal, 57, 1118–1140. doi:10.1080/02626667.2012.701305
- Weiler, M., et al., 2003. How does rainfall become runoff? A combined tracer and runoff transfer function approach. Water Resources Research, 39 (11), 1315. doi:10.1029/2003WR002331
- White, D., 1988. Grid-based application of runoff curve numbers. Journal of Water Resources Planning and Management, ASCE, 114 (6), 601–612. doi:10.1061/(ASCE)0733-9496(1988)114:6(601)
- Wight, J.R. and Skiles, J.W., 1987. SPUR: simulation of production and utilization of rangelands. Documentation and users guide. Washington, DC: US Department of Agriculture -Agricultural Research Service, 372.
- Williams, J. R., Arnold, J. G., and Srinivasan, R., 2000. The APEX model. Temple, TX: Texas A&M Blackland Research Center, BRC Report No. 00–06.
- Williams, J.R., et al., 2012. Evolution of the SCS runoff curve number method and its application to continuous runoff simulation. Journal of Hydrologic Engineering, 17(11),1221–1229.
- Williams, J.R. and Izaurralde, R.C., 2005. The APEX model. Temple, TX: Texas A&M Blackland Research Center, BRC Report no. 2005-2.
- Williams, J.R. and LaSeur, V., 1976. Water yield model using SCS curve numbers. Journal of Hydraulic Engineering, 102 (9), 1241–1253.
- Woodward, D.E., et al., 2002. Curve number method: origins, applications and limitations. Second Federal Interagency Hydrologic Modeling Conference, Las Vegas, NV, 1–10. Available from: http://www.wcc.nrcs.usda.gov/water/quality/common/techpapers/curve.html.
- Woodward, D.E., et al., 2003. Runoff curve number method: examination of the initial abstraction ratio. In: Paul Bizier and Paul DeBarry, eds.World Water & Environ. Resources Congress 2003 and related symposia, 23–26 June 2003. Philadelphia, PA: EWRI, ASCE, 23–26.
- Woodward, D.E., et al., 2010. Discussion of “modifications to SCS-CN method for long-term hydrologic simulation” by K. Geetha, S. K. Mishra, T. I. Eldho, A. K. Rastogi, and R. P. Pandey. Journal of Irrigation and Drainage Engineering, 136 (6), 444–446. doi:10.1061/(ASCE)IR.1943-4774.0000231
- Woodward, D.E. and Gbuerek, W.J., 1992. Progress report ARS/SCS curve number work group. In: Proceedings ASCE, Water Forum, 92. New York: ASCE, 378–382.
- Xue, X., et al., 2013. Statistical and hydrological evaluation of TRMM-based multi-satellite precipitation analysis over the Wangchu basin of Bhutan: are the latest satellite precipitation products 3B42V7 ready for use in ungauged basins? Journal of Hydrology, 499, 91–99. doi:10.1016/j.jhydrol.2013.06.042
- Yilmaz, K., et al., 2010. Evaluation of a satellite based global flood monitoring system. International Journal of Remote Sensing, 31, 3763–3782. doi:10.1080/01431161.2010.483489
- Yilmaz, K.K., et al., 2005. Intercomparison of rain gauge, radar and satellite-based precipitation estimates with emphasis on hydrologic forecasting. Journal of Hydrometeorology, 6 (4), 497–517. doi:10.1175/JHM431.1
- Yong, B., et al., 2010. Hydrologic evaluation of multi satellite precipitation analysis standard precipitation products in basins beyond its inclined latitude band: A case study in Laohahe basin, China. Water Resources Research, 46 (7), W07542. doi:10.1029/2009WR008965
- Young, R.A., et al., 1989. AGNPS: a nonpoint-source pollution model for evaluating agricultural watersheds. Journal of Soil and Water Conservation, 44 (2), 168–173.
- Yu, B., 1998. Theoretical justification of SCS method for runoff estimation. Journal of Irrigation and Drainage Engineering, 124 (6), 306–310. doi:10.1061/(ASCE)0733-9437(1998)124:6(306)
- Yuan, Y., et al., 2001. Modified SCS curve number method for predicting sub surface drainage flow. Transactions of the ASCE, 44 (6), 1673–1682.
- Yuan, Y., et al., 2014. Initial abstraction and curve numbersfor semiarid watersheds in south eastern Arizona. Hydrological Processes, 28, 774–783. doi:10.1002/hyp.9592
- Zende, A.M. and Atal, K.R., 2015. Identification of rainwater harvesting structure for Yerala river using remote sensing and GIS. In: E-proceedings of the 36th IAHR World Congress. The Hague, The Netherlands: IAHR.
- Zende, A.M., Nagarajan, R., and Atal, K.R., 2014. Analysis of surface runoff from Yerala River Basin using SCS-CN and GIS. International Journal of Geomatics and Geosciences, 4 (3), 508–516.
- Zhan, X. and Huang, M.-L., 2004. ArcCN-runoff: an ArcGIS tool for generating curve number and runoff maps. Environmental Modelling & Software, 19 (10), 875–879. doi:10.1016/j.envsoft.2004.03.001
- Zhang, H., Haan, C.T., and Nofziger, D.L., 1990. Hydrologic modeling with GIS: an overview. Applied Engineering in Agriculture, 6 (4), 453–458. doi:10.13031/2013.26413