ABSTRACT
The Kalu Ganga catchment is one of the largest in Sri Lanka, and is home to 5% of the national population. A first assessment is provided here of the sensitivity of Kalu Ganga runoff to a 2°C increase in global mean temperature – the supposed threshold for “dangerous” climate change. Runoff is simulated using the HBV-Light hydrological model and scenario data from seven general circulation models (GCMs). Precipitation is the strongest cause of change in runoff. Substantial inter-GCM differences in scenario precipitation lead to uncertainty in the direction of change in mean annual runoff from the baseline (range −25% to +19%). Scenario monthly runoff ranges from −41% to +124% of the baseline values at its most extreme (March); June is the only month with a consistent direction of change (range −17% to −65%) – thus indicating that climate change may lead to a substantially different hydrological regime in the Kalu Ganga catchment.
EDITOR A. CastellarinASSOCIATE EDITOR Q. Zhang
1 Introduction
Catchment-scale hydrological processes worldwide are expected to change due to the effects of climate change (Jiménez Cisneros et al. Citation2014). In general, a warmer world is expected to result in both changing mean states and in the frequency and magnitude of wet and dry extreme events, in part as a result of increased water holding capacity of the atmosphere (Held and Soden Citation2006). In consequence, there will likely be large scale, and in places substantial, changes in water resource availability (e.g. Gosling and Arnell Citation2016). Although climate change (and associated water resource changes) will bring positive as well as negative effects, the negatives are expected to overwhelm any benefits that may occur (IPCC Citation2014). In particular, changes to the hydrological cycle are expected to have negative impacts for crop yields, food security and livelihoods in many areas (e.g. Kelkar et al. Citation2008). Impacts on staple crops such as rice may lead to increases in food prices and the cost of living, further exacerbating existing rural poverty in developing countries (Skoufias et al. Citation2011).
Previous research has highlighted the need for further work on building understanding of the impacts of climate change (Hijioka et al. Citation2014), as the more understanding there is about the impacts of climate change the better the potential costs to humans can be estimated and effective mitigating and adaptive measures taken. Policy decisions on mitigation and adaptation strategies require understanding, quantification and a synthesis of climate change impacts, whilst taking into account their uncertainties (Piontek et al. Citation2014). Uncertainty is a particularly critical aspect of climate change impact assessment, owing to the cascade of uncertainty that develops from the greenhouse gas emissions scenario through general circulation model (GCM) sensitivity, impact (i.e. hydrological) model uncertainty, and through to management and user implications. Although it is not always possible to reduce such uncertainty, its identification is critical. This study focuses on GCM sensitivity, often one of the largest components of uncertainty in hydrological impact studies (Prudhomme and Davies Citation2009).
In Sri Lanka, there is already evidence for the occurrence of climatic change based on a statistically significant increase in decadal mean temperatures between 1869 and 2007 in six out of seven stations distributed across all national climatic zones (Costa Citation2017). Additionally, in all seven locations mean annual precipitation from 1990 to 2007 was lower than precipitation from 1950 to 1989 (Costa Citation2017). Similar patterns of change in observed temperature are generally present at the wider regional scale, but regional precipitation trends are highly variable (Jiménez Cisneros et al. Citation2014). The latter finding is in part due to the strong influence of the Asian monsoon on precipitation climate, and substantial spatial differences in the impact of this phenomenon combined with inter-decadal variability. Regional-scale projections of 21st century climate change indicate further warming, and for precipitation, increases that will exceed present day natural variability (IPCC Citation2013, Hijioka et al. Citation2014).
The Kalu Ganga is the second largest river by discharge volume in Sri Lanka, and the catchment is of particular importance to the country due to its agricultural productivity, high population density and growing population, but at the same time it is one of the most flood-prone rivers in the country (Eriyagama et al. Citation2010). A number of authors have suggested that catchment-scale impact assessments may bring some clarity to how Sri Lanka may be affected by a changing climate (e.g. Eriyagama et al. Citation2010, Esham and Garforth Citation2013). Although national-scale impacts on the balance between precipitation and potential evaporation (for irrigation requirements) have been studied (De Silva et al. Citation2007), with increased water for irrigation needed by the 2050s, to date there have been no climate change impact assessments focused on catchment-scale hydrology in Sri Lanka (to the authors’ knowledge). This research need is addressed here through an investigation of the possible impacts on Kalu Ganga river flow of a 2°C increase in global mean temperature – frequently assumed to be the threshold for “dangerous” climate change (Todd et al. Citation2011). The Kalu Ganga is simulated using the hydrological model HBV-Light, and seven GCMs are used to provide an indication of the possible changes in climate, and in turn, river flow, at the 2°C threshold.
2 Data and methods
2.1 The Kalu Ganga catchment
The catchment’s main river, the Kalu Ganga, has a length of 129 km and lies between approximately 6.2°N–6.5°N and 79.9°E–80.5°E (). The catchment has an area of 2765 km2 and flows from an elevation of 2250 m in Sri Lanka’s central highlands in a westerly direction along (and occasionally cutting through) narrow strike valleys, forming a typically rectangular drainage pattern (Wickramagamage Citation2011). In some places the river cuts through strike ridges. From the city of Ratnapura, the Kalu Ganga flows through relatively flat terrain from an elevation of 14 m for about 70 km before flowing into the Indian Ocean (). The gauging station (Putupaula) formed the downstream limit of this study, at which the catchment size is 2627 km2.
Figure 1. Location and elevation of the Kalu Ganga catchment, river, meteorological and hydrological stations and other noteworthy features.
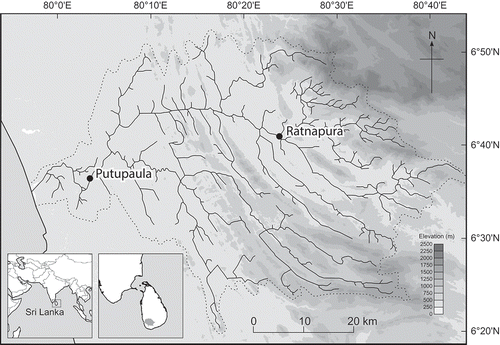
The Kalu Ganga catchment lies in the F-region according to the Domroes and Ranatunge (Citation1993) climatic regionalization of Sri Lanka. In this region, annual rainfall is typically greater than 3000 mm. In terms of seasonal distribution of rainfall, there are two monsoon seasons: the southwest monsoon (May–July) and the northeast monsoon (October–November), but with rainfall also occurring in the two inter-monsoon seasons. The Kalu Ganga catchment, with an estimated mean annual discharge of 4000 × 106 m3, is Sri Lanka’s second largest by discharge volume (Ampitiyawatta and Guo Citation2009). The mean annual temperature and precipitation (1981–2009) for the meteorological station at Ratnapura () are 27.5°C and 3798 mm, respectively.
Approximately 2.2 million people live in the Kalu Ganga catchment, with the lower floodplains densely populated (Ampitiyawatta and Guo Citation2009). The fertile floodplains and temperate climate have resulted in increasing human population encroachments into the flood plains, making the population more vulnerable to frequent floods (Samarasinghea et al. Citation2010). According to Nandalal (Citation2009), there is no substantial development of water resources in the Kalu Ganga basin apart from drinking water supply and minor irrigation schemes. According to the same author, the only notable hydropower development is on the Kukule Ganga tributary, where an 80-MW run-of-river hydropower scheme was opened in 2005. However, water extraction in the catchment is not tightly monitored. Unofficial water use in the catchment, such as small-scale irrigation, small-scale animal husbandry or small-scale industrial uses, might result in significant levels of water extraction (Prasantha et al. 2003), especially since a government subsidy scheme for brick and concrete lined wells was introduced in 1989 (FAO Citation2011).
Major land use in the catchment consists of natural and cultivated forest, as well as residential and agricultural cropland use (Samarasinghea et al. Citation2010). In the upper reaches, most tributaries to the Kalu Ganga originate in the Peak Wilderness Sanctuary in the northeast of the catchment, and the UNESCO world heritage rainforest Sinharaja in the southeast. The upper catchment area is also known for practices resulting in unstable slopes and regular landslides, such as chena cultivation, which is a form of shifting cultivation involving periodic tree felling and burning in preparation of the land for crop planting, gem mining and regular replanting of tea and rubber plantations (Prasantha et al. Citation2003). Prasantha et al. (Citation2003) suggest that the gem-mining activities may result in increased clay sedimentation in rivers and reservoirs, a lower groundwater table, unstable slopes, as well as contamination of and other adverse effects on arable land. The main land use in the lower part of the catchment consists of domestic gardens, rubber plantations and rice cultivation (Imbulana et al. Citation2006).
2.2 Data
Daily values of river discharge from just one gauging station, Putupaula (for 1980–2011) were made available from the Department of Irrigation, Colombo. This flow record formed the basis for evaluation of model performance (the HBV-Light model can only provide output for the catchment outlet). The HBV-Light simulation of river flow can only be based on a single temperature and precipitation input record; in this case the record from Ratnapura () for the period 1980–2011 was used (data source: Department of Meteorology, Colombo). These measurements were taken at the local office of the Department of Meteorology (6°41′N, 80°24′E), which lies at an elevation of 34 m a.s.l. and in the middle reaches of the Kalu Ganga catchment (). Elevation-dependent variation in precipitation from the lower to the upper catchment was accounted for using a lapse rate function within HBV-Light (a temperature lapse rate function is present too, but was not considered further as this is only relevant for the snow routine in the model). A single record for potential evapotranspiration is also required as input to HBV-Light. For this study, daily values for potential evapotranspiration were calculated (external to the model) from the Ratnapura temperature record using the Hargreaves-Samani method. This method is preferred when solar radiation, wind speed, relative humidity and other meteorological variables are absent (Droogers and Allen Citation2002). It is not possible to lapse potential evapotranspiration (PET) with altitude in the model, a potential limitation to this study.
Double-mass analysis of runoff and precipitation over the study period suggest that the discharge record may contain inhomogeneities, and initial runs of HBV-light support this finding. Cumulative precipitation measured at Ratnapura shows no major long-term change in accumulation rate of daily precipitation, whereas cumulative runoff shows some instability across time (). In personal communications with the Department of Irrigation, it was indicated that riverbed modifications due to sand mining may have changed the stage–discharge relationship, causing the rating curve to change periodically. This meant that a conventional division of the 30-year observed period into calibration and validation periods was not possible, with shorter periods of similar approximate runoff accumulation rates used instead. The longest stable period occurs from March 1989 to November 1993, and so was used as the basis for hydrological model calibration. Earlier (shorter) stable periods, from February 1981 to October 1983 and September 1984 to July 1987, served as the periods for model validation.
2.3 The hydrological model
The catchment model used for this study is the conceptual Hydrologiska Byråns Vattenbalansavdelning (HBV) model. The model version HBV-Light is used here (Seibert and Vis Citation2012). This version is very similar to the HBV-6 model (Bergstrom Citation1992), but with a user-defined model warm-up period added for model state variables to reach appropriate values, and non-integer values allowed for the routing parameter (Seibert Citation1999). Although the HBV model was initially developed for use in northern Europe, HBV-Light has been used successfully to model catchments in a wide range of locations, from montane tropical (Plesca et al. Citation2012) to lowland and moderate elevation temperate catchments (Steele-Dunne et al. Citation2008, Driessen et al. Citation2010, Koedyk and Kingston Citation2016). The last three studies have also shown that HBV-Light can be used successfully for climate change impact assessments.
The HBV-Light model is a conceptual rainfall–runoff model based on a series of numerical relationships that approximate real-world processes in the hydrological system of a catchment. Its design stands in contrast to more complex distributed models (such as MIKE-SHE: Graham and Butts Citation2005), which simulate spatial variation in catchment hydrological processes at far higher spatial resolution and with greater physical basis to the representation of those hydrological processes. However, complex models also require substantial input data, whereas HBV-Light requires only a single climate and discharge record, making it a pragmatic choice of hydrological model in the situation of limited data availability.
The HBV-Light model has four parts, namely a snow routine, a soil moisture routine, a recharge routine and a routing routine, each of which can be adjusted by one to four parameters. In the HBV-Light simulation, water from rain or snowmelt enters either the “soil box” (from which it is evaporated) or the recharge routine. In the latter, water is released for runoff via two mechanisms: a fast-responding and a slow-responding water store (forming representations of quickflow and baseflow, respectively). HBV-Light includes some representation of spatial variability in catchment characteristics, but in a relatively simple way (i.e. without use of a GIS base to the model). Firstly, different elevation zones can be defined within the model. In combination with user-specified lapse rates for precipitation and temperature, this allows for spatial variation in precipitation totals, and the accumulation and melt of snow in the catchment (although the latter is not relevant for the Kalu Ganga). Secondly, each elevation zone can be subsequently divided into “vegetation” zones, which allows for representation of spatial variation in the process of division of water from rain or snowmelt into either the soil box or recharge routine.
2.4 Calibration and validation
The data period for calibration covers March 1989–November 1993, which is the longest stable period in the record. Here, the best performing parameter set was determined on the basis of 250 000 Monte-Carlo simulations that subsequently were modified iteratively to achieve greater model performance. For validation of the model the same optimum set of parameters determined by the calibration process were applied to a dataset which had not been part of the calibration period. Parameter values of the final model version are given in .
Table 1. Calibrated values of HBV-Light parameters for simulation of Kalu Ganga river flow.
The performance for the calibration period (1989–1993) resulted in a daily Nash-Sutcliffe efficiency (NS) of 0.85, on the margin between “excellent” and “very good” according to the classification used by Henriksen et al. (Citation2003), and in the “very good” category of Moriasi et al. (Citation2007). The model performance for the validation periods (1980–1983, 1983–1987), with NS values of 0.67 to 0.73 respectively, is “very good” according to Henriksen et al. (Citation2003) and “good” following Moriasi et al. (Citation2007). Percent bias of daily runoff is <4% for all three periods, which is in the “very good” category of Moriasi et al. (Citation2007). Spearman Rank correlation of modelled and observed daily runoff ranges between 0.84 and 0.89, again suggesting realistic simulation of the Kalu Ganga. Examination of mean monthly observed and simulated river flow () indicates a very good year-round fit during the calibration period. A slightly weaker monthly fit is present during the validation period, with runoff slightly too high for December–March, and slightly too low for April–June. Notwithstanding these relatively minor biases, model performance appears to provide a realistic and stable simulation of Kalu Ganga river runoff.
Figure 3. Mean monthly simulated and observed river runoff for (a) the calibration period, (b) combined validation period and (c) monthly simulated and observed runoff for all three periods (vertical lines indicate gap between the first and second validation periods, and subsequently between the second validation and calibration periods).
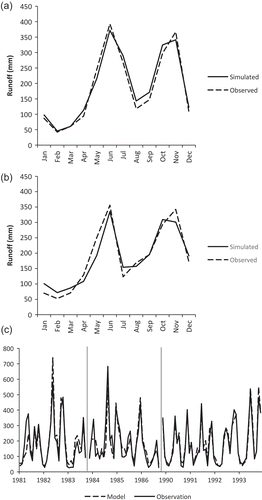
2.5 Downscaling and the 2°C scenario
The hydrological model was forced with projections of Sri Lankan climate in scenarios where global mean temperature is 2°C above the 1961–1990 mean. The 2°C threshold represents a particularly interesting scenario, as it is often presumed to be the threshold for dangerous climate change, and because it represents the increase in global mean temperature the international community has committed the world to (UNFCCC Citation2015).
Climate change scenarios were generated using the pattern-scaling technique, as described by Todd et al. (Citation2011) and Osborn et al. (Citation2016). Pattern scaling can be a particularly useful method to investigate climate change impacts, as it allows scaling of global mean temperatures to specific thresholds (such as the 2°C increase simulated here). This method has been used by a series of previous studies to explore catchment- and global-scale impacts of climate change on river flow at the 2°C threshold (e.g. Gosling et al. Citation2011, Todd et al. Citation2011, Thompson et al. Citation2015), and the current investigation adds to this series of case studies.
Pattern-scaled climate change scenarios were generated for seven Coupled Model Intercomparison Stage 3 (CMIP-3) GCMs using the ClimGen programme (Osborn Citation2009). Only seven GCMs were examined in light of the time constraints of this study, and nature of the CMIP-3 GCMs as an arbitrary “ensemble of opportunity” rather than spanning the full range of GCM uncertainty (Tebaldi and Knutti Citation2007). These comprise the GCMs CCCMA GCGM 3.1, CSIRO Mk2, IPSL CM4, MPI ECHAM5, NCAR CCSM30, UKMO HadCM3 and UKMO HadGEM1, full details of which can be found in Meehl et al. (Citation2007). These GCMs were selected on the basis of previous (subjective) assessment of model quality, use of the GCMs in previous impact assessments, and that they spanned a range of different precipitation futures (Gosling et al. Citation2011, Todd et al. Citation2011). Following Todd et al. (Citation2011), the GCM scenario data are interpolated statistically to a spatial resolution of 0.5° latitude/longitude and applied to the CRU TS 3.0 historical climate dataset. The Kalu Ganga falls within a single grid cell at this resolution, so to produce scenario river flow simulations GCM data for the grid cell corresponding to the catchment location were extracted and the baseline-scenario difference applied to the observed time series – again following the methodology outlined in Todd et al. (Citation2011).
3 Results
3.1 Changes in catchment climatology
Changes in climate from the 1961–1990 baseline to the 2°C scenario were not consistent between the seven GCMs (). Each GCM differs in its simulation of global temperature change meaning that while the global mean temperature change is the same between GCMs, regional patterns vary somewhat. For example, the rise in mean annual air temperature ranges from 1.2°C for the NCAR GCM to 1.6°C for the CSIRO GCM. Differences between GCMs are much more apparent for precipitation compared to temperature. Projected change in mean annual precipitation over the Kalu Ganga catchment varies from a 13% increase (MPI) to a 15% decrease (CSIRO) ().
Table 2. Simulation results for baseline scenario mean annual precipitation, temperature and runoff. Baseline values are in mm for precipitation and runoff, and °C for temperature. Scenario values are in % change from baseline for precipitation and runoff, and °C increase from baseline for temperature.
On a monthly basis, changes in climate are more variable than at the annual resolution (both within and between GCMs) – for example all GCMs show a mixture of increasing and decreasing precipitation over the course of the year (). There is little clarity as to the overall changes in southwest monsoon precipitation (May–September), with some GCMs projecting a seasonal peak for April and wide disagreement around precipitation in July. There is, however, consistency in the direction of change for June and August precipitation. Simulated mean monthly precipitation for June ranges from – 31% (HadCM3) to – 60% (HadGEM1). Simulations of mean monthly precipitation for August range from +5% (HadGEM1) to +46% (MPI). Although GCM projections appear more clustered (with one or two outliers) in the second inter-monsoon period (October–November), there is still uncertainty in the direction of change. In addition to uncertainty in wet season precipitation, there is very little agreement between GCMs as to whether precipitation will decrease or increase during the driest period from December to February, which comprises the northeast monsoon season. Despite uncertainty in the direction of change at various points of the year, all simulations suggest a change from a bi-modal to a tri-modal precipitation regime, with a relatively small third peak in rainfall occurring in July, caused by substantially reduced June totals ().
Figure 4. (a) Baseline and scenario precipitation, (b) temperature and (c) precipitation to PET ratio.
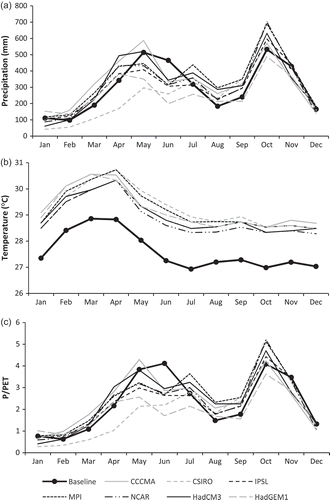
In contrast to precipitation, temperature increases in all months, and is relatively consistent between GCMs, with increases ranging from a minimum of 1.1°C in February and March (IPSL, NCAR, HadCM3) to 2.1°C in June (CSIRO) (). Across all months and GCMs, the mean increase in monthly temperature is 1.5°C. Although not great in magnitude, there are some consistent differences between GCMs: CCCMA has the largest increases in temperature for November–January, and CSIRO for May–September; and the NCAR GCM results in the lowest increases in temperature for May–September.
Potential evapotranspiration (PET) increases in all months, with similar magnitudes of change between months and between GCMs (and largely following the pattern of scenario temperature). The mean monthly aridity index (i.e. P/PET) shows that during February mean monthly PET exceeds precipitation for the baseline and all scenarios, which indicates that this month will remain a period of a meteorological water deficit (). The same is true for January for all but the HadGEM1 GCM. According to all simulations dryness increases in June, but not to the level of a meteorological deficit. According to all simulations the month of August becomes wetter with precipitation increasing relative to changing evapotranspiration.
3.2 Changes in runoff and catchment hydrology
Simulations forced by changes in both precipitation and evapotranspiration show substantial disparity between GCMs with little consistency to either direction or magnitude on a monthly and annual scale (, ). Scenario annual runoff ranges from an increase of 19.4% (MPI) to a decrease of 25.1% (CSIRO). On an annual level the CSIRO- and HadGEM1-driven simulations indicate substantial reductions in mean annual runoff as opposed to a slight reduction (IPSL) or increases for the remaining four GCMs. Hydrological model results are similar whether forced by precipitation only or changing precipitation and evapotranspiration: differences between the precipitation-only and precipitation and evaporation scenarios range from 1% to 3% in most months and peak differences of up to 5% occurring for March or May depending on GCM-simulation.
There is large variation in magnitude and direction between the different GCM-driven simulations of mean monthly runoff. Scenario monthly runoff ranges for example from 36 mm (–46%, CSIRO) to 135 mm (+102%, CCCMA) in March and from 246 mm (–24%, HadGEM1) to 451 mm (+38%, MPI) in October (). There is some level of clustering of GCMs, with all but the CSIRO-driven simulation indicating a positive change in the months of March and April and all but the HadGEM1-driven simulation showing a positive change in October. Simulations for runoff during the Northeast Monsoon season (December–February) show an increase or decrease depending on the GCM driving the simulation. Simulations range from 114 mm (–14%, CCCMA) to 151 mm (15%, MPI) in December and 63 mm (–36%, CSIRO) to 118 mm (+20%, HadGEM1) in January. Simulations for runoff during the Southwest Monsoon season (May–September) show an increase or decrease depending on the GCM driving the simulation with only one month having cross-GCM agreement as to the direction of change. Simulations range across over 100 percentage points (243 mm) in May and in July by 62% or 156 mm. June simulations for mean monthly runoff however agree in the direction of change and range from 132 mm (–10%, CCCMA) to 310 mm (–62%, CSIRO).
Under the baseline climate, baseflow is the most important component of runoff from January–March, peaking in February at 90% of runoff (). For all other months, quickflow is more important, peaking just below 90% of runoff in June, October and November. As with runoff overall, there is little agreement as to the direction or magnitude of monthly changes between the different GCMs. Also following total runoff, there is a consistent direction of change in June, with the proportion of runoff originating from quickflow (baseflow) decreasing (increasing) across all scenarios.
4 Discussion
The scenario results depend overwhelmingly on the respective GCM driving the simulation. The results indicate uncertainty in the direction of change of runoff at both the mean annual and monthly resolution, with projections of mean annual runoff varying from a 19% increase to a 25% decrease. Uncertainty at the monthly scale is more variable. Direction of simulated change varies between GCMs in all but one month, with the largest disagreement in percentage terms for March, April and May (149, 139 and 100%, respectively), and in absolute terms for May and October (over 200 mm for both; ). Generally, the different GCM scenarios tend to cluster during the months of February, March and April as well as August and September. Frequently it is CSIRO or HadGEM1 GCMs that appear markedly different to the other GCMs, resulting in relatively low scenario runoff. The CCCMA GCM generally results in the highest scenario runoff during the first half of the year, and IPSL during the second half, although there is no clear separation between these and the other GCMs (unlike for HadGEM1 and CSIRO). The month of June shows agreement across all GCMs in terms of direction of simulated change and this month is among the four lowest in terms of percentage difference between the highest and lowest GCM scenario.
Running the hydrological model with baseline PET and scenario precipitation provides almost identical results to those shown in . Together with comparison of the precipitation climate change signal (), it appears that the vast majority of uncertainty in the Kalu Ganga runoff climate change signal results from differences in GCM scenario precipitation. This finding largely mirrors many previous studies of climate change impacts on river flow (e.g. Prudhomme and Davies Citation2009, Todd et al. Citation2011, Jiménez Cisneros et al. Citation2014). Although there appears to be a clustering of GCMs (for both precipitation and runoff) in a number of months, this is not a complete sample of available GCMs, so any clustering cannot be considered statistically meaningful. There also have not been any similar studies in this region with which results could be compared (to the authors’ knowledge). However, assessment of changes in scenario precipitation for Sri Lanka from a far greater number of GCMs in the IPCC Fifth Assessment Report (IPCC Citation2013) has indicated little consistency in seasonal 50th and 75th percentile precipitation between models. Notwithstanding the dominance of precipitation in the runoff climate change signal, it is important to note that the only month with a consistent direction of change in runoff (June) also has the greatest increase in temperature.
A comparison with simulated baseflow indicates that quickflow is a far more important component of river runoff for nine months of the year. The dominance of quickflow is unsurprising in a tropical catchment with relatively high rainfall totals, such as the Kalu Ganga, where field capacity would be expected to be reached more often than in catchments in drier climatic zones. Inter-GCM uncertainty makes it unclear as to whether the total relative share of runoff consisting of quickflow will increase or decrease in all months but June. The ratio of quickflow to total runoff drops in June across all simulations, indicating that baseflow makes relative gains in hydrological importance in June as its fraction of total runoff increases. For all other months the direction of change in this ratio depends on which GCM is driving the simulation.
All but the CSIRO-driven simulation project an increase in precipitation and runoff in April, meaning a forward shift in the southwest monsoon season (May–September), changes that may have a detrimental impact on agriculture. In particular, an earlier southwest monsoon with less water available in mid-season and more precipitation toward its end on average may disrupt traditional growing schedules. June lies in the early April–early September cultivation season and the most popular variety of rice (Bg 300) would be in the middle of its three-month maturing period (Wang et al. Citation2012). Given inter-GCM uncertainty, it is not clear whether reductions in June precipitation will threaten rice production; however, extreme events such as floods have been shown to substantially impact rice production in the area (Wang et al. Citation2012).
The projected climate and hydrological changes found here may also influence hydropower generation. The 80 MW scheme in the Kukule Ganga tributary of the Kalu Ganga provides about 3.4% of national electricity, and there is substantial generating capacity in neighbouring catchments (immediately north of the Kalu Ganga, the Kelani Ganga catchment has 335 MW installed capacity). There have previously been concerns the effect changing precipitation may have on the operation of the Kukule Ganga station (Sonoda Citation2007). During the wet season, approximately 10% of river flow has to be spilled from the dam currently. It is unlikely therefore that increases in discharge in the second wet season could be used for additional generation. Decreases in the first wet season are greater than 10% for many scenarios, meaning a reduction in generating capacity is probable, but is countered by the increases in dry season runoff (in most scenarios). The CSIRO scenario would lead to by far the most dramatic drop in year-round generating capacity.
Notwithstanding the findings presented here, climate change is only one of the factors that influence the hydrology of the Kalu Ganga catchment. Global simulations by Fung et al. (Citation2011) have shown that, in a +2°C world, the population effect on water availability is greater than the water stress due to climate change. Particularly for the GCMs indicating a more moderate change in climate at the 2°C threshold (e.g. IPSL, NCAR), the findings of Fung et al. (Citation2011) may hold true for the Kalu Ganga, indicating that a 2°C rise in global mean temperature is not necessarily “dangerous” in itself for water resources in this location (but the combination of population pressure and climate change may be). However, Fung et al. (Citation2011) have shown that towards a world with a +4°C anomaly, the climate change influence becomes amplified and increasingly consistent between models, leaving climate change as the dominant source of water stress.
5 Conclusion
This study has shown that there is a high degree of uncertainty in the possible impacts of climate change on Kalu Ganga runoff at the supposed 2°C threshold of dangerous climate change. Scenario runoff ranges between increases and decreases from the baseline, and from relatively small to relatively large impacts at both the monthly and annual time scales. A consistent direction of change is observed in only one month of the year (June), where runoff is projected to decrease. Scenario runoff is shown to be highly sensitive to scenario precipitation, reflecting both that this is a precipitation-dominated catchment, and the relatively high degree of consistency between GCM projections of temperature. Further research is required to better constrain this initial assessment of uncertainty.
Bearing in mind the inconsistency between GCMs and the range of runoff scenarios from slight to more severe change, it is difficult to understand whether a 2°C increase in global mean temperature will actually have substantial impacts, at least for river runoff in the Kalu Ganga. Notwithstanding the definition of “dangerous”, all of the projected changes in climate simulated here are likely to impact agriculture and hydropower in some way. As such, these results provide important initial information on the possible range of uncertainty in future river flow for the Kalu Ganga, on which future studies of climate change impacts on water resources (and associated policy and management implications) can build.
Acknowledgements
Chris Garden (University of Otago Department of Geography) produced . Comments from the anonymous review process contributed to the manuscript.
Disclosure statement
No potential conflict of interest was reported by the authors.
Additional information
Funding
References
- Ampitiyawatta, A.D. and Guo, S., 2009. Precipitation trends in the Kalu Ganga Basin in Sri Lanka. The Journal of Agricultural Science, 4, 10–18.
- Bergstrom, S., 1992. The HBV model: its structure and applications. Norrköping: Swedish Meteorological and Hydrological Institute (SMHI), Hydrology, 35 pp.
- Costa, W.A.J.M., 2017. Climate change in Sri Lanka: myth or reality? evidence from long‐term meteorological data. Journal of the National Science Foundation of Sri Lanka, 36, 63–88. doi:10.4038/jnsfsr.v36i0.8048
- De Silva, C.S., et al., 2007. Predicting the impacts of climate change – a case study of paddy irrigation water requirements in Sri Lanka. Agricultural Water Management, 93, 19–29. doi:10.1016/j.agwat.2007.06.003
- Domroes, M. and Ranatunge, E., 1993. A statistical approach towards a regionalization of daily rainfall in Sri Lanka. International Journal of Climatology, 13 (7), 741–754. doi:10.1002/(ISSN)1097-0088
- Driessen, T.L.A., et al., 2010. The hydrological response of the Ourthe catchment to climate change as modelled by the HBV model. Hydrology and Earth System Sciences, 14, 651–665. doi:10.5194/hess-14-651-2010
- Droogers, P. and Allen, R.G., 2002. Estimating reference evapotranspiration under inaccurate data conditions. Irrigation and Drainage Systems, 16 (1), 33–45. doi:10.1023/A:1015508322413
- Eriyagama, N., et al., 2010. Impacts of climate change on water resources and agriculture in Sri Lanka: a review and preliminary vulnerability mapping. (IWMI Research Report 135), Colombo, Sri Lanka: International Water Management Institute, 51. doi:10.5337/2010.211.
- Esham, M. and Garforth, C., 2013. Climate change and agricultural adaptation in Sri Lanka: a review. Climate and Development, 5, 66–76. doi:10.1080/17565529.2012.762333
- FAO, 2011. Aquastat: Sri Lanka, Water Report 37. Geography, climate and population. http://www.fao.org/nr/water/aquastat/countries_regions/LKA/ [ Accessed June 2017].
- Fung, F., Lopez, A., and New, M., 2011. Water availability in +2°C and +4°C worlds. Philosophical Transactions of the Royal Society: Mathematical, Physical and Engineering Sciences, 369 (1934), 99–116. doi:10.1098/rsta.2010.0293
- IPCC (Intergovernmental Panel on Climate Change), 2013. Annex I: atlas of global and regional climate projections. In: G.J. van Oldenborgh, et al., eds. Climate change 2013: the physical science basis. Contribution of working group I to the fifth assessment report of the intergovernmental panel on climate change (T.F. Stocker et al., eds.). Cambridge, UK: Cambridge University Press.
- Gosling, S.N., et al., 2011. A comparative analysis of projected impacts of climate change on river runoff from global and catchment-scale hydrological models. Hydrology and Earth System Sciences, 15 (1), 279–294. doi:10.5194/hess-15-279-2011
- Gosling, S.N. and Arnell, N.W., 2016. A global assessment of the impact of climate change on water scarcity. Climatic Change, 134, 371–385. doi:10.1007/s10584-013-0853-x
- Graham, D.N., and Butts, M.B., 2005. Flexible, integrated watershed modelling with MIKE SHE. In: V.P. Singh and D.K. Frevert, eds. Watershed models. Boca Raton, FL: CRC Press, 245–272.
- Held, I.M. and Soden, B.J., 2006. Robust responses of the hydrological cycle to global warming. Journal of Climate, 19, 5686–5699. doi:10.1175/JCLI3990.1
- Henriksen, H.J., et al., 2003. Assessment of exploitable groundwater resources of Denmark by use of ensemble resource indicators and a numerical groundwater – surface water model. Journal of Hydrology, 348 (1–2), 224–240. doi:10.1016/j.jhydrol.2007.09.056
- Hijioka, Y., et al., 2014. Chapter 24. Asia. In: C. Field, et al., eds. Climate change 2014: impacts, adaptation, and vulnerability. Contribution of working group II to the fifth assessment report of the intergovernmental panel on climate change. Cambridge, UK: Cambridge University Press, IPCC.
- Imbulana, K.A.U.S., Wijesekara, N.T.S., and Neupane, B.R., 2006. Sri Lanka National Water Development Report. Colombo: Paris and New Delhi.
- Jiménez Cisneros, B.E., et al., 2014. Freshwater resources. In: C.B. Field et al., eds. Climate change 2014: impacts, adaptation, and vulnerability. Part A: global and sectoral aspects. Contribution of working group II to the fifth assessment report of the intergovernmental panel on climate change. Cambridge, UK: Cambridge University Press, 229–269.
- Kelkar, U., et al., 2008. Vulnerability and adaptation to climate variability and water stress in Uttarakhand State, India. Global Environmental Change, 18, 564–574. doi:10.1016/j.gloenvcha.2008.09.003
- Koedyk, L.P. and Kingston, D.G., 2016. Potential evapotranspiration method influence on climate change impacts on river flow: a mid-latitude case study. Hydrology Research, 47, 951–963. doi:10.2166/nh.2016.152
- Meehl, G.A., et al., 2007. The WCRP CMIP3 Multimodel Dataset: a new era in climate change research. Bulletin of the American Meteorological Society, 88, 1383–1394. doi:10.1175/BAMS-88-9-1383
- Moriasi, D.N., et al. 2007. Model evaluation guidelines for systematic quantification of accuracy in watershed simulations. American Society of Agricultural And Biological Engineers, 50, 885−900.
- Nandalal, K.D.W., 2009. Use of a hydrodynamic model to forecast floods of Kalu River in Sri Lanka. Journal of Flood Risk Management, 2, 151–158. doi:10.1111/jfrm.2009.2.issue-3
- Osborn, T.J., 2009. A user guide for ClimGen: a flexible tool for generating monthly climate data sets and scenarios. Climatic Research Unit. Norwich: University of East Anglia, 17.
- Osborn, T.J., et al., 2016. Pattern scaling using ClimGen: monthly-resolution future climate scenarios including changes in the variability of precipitation. Climatic Change, 134, 353–369. doi:10.1007/s10584-015-1509-9
- Piontek, F., et al. 2014. Multisectoral climate impact hotspots in a warming world. Proceedings of the National Academy of Sciences of the United States of America, 111 (9), 3233–3238. doi:10.1073/pnas.1222471110
- Plesca, I., et al., 2012. Model intercomparison to explore catchment functioning: results from a remote montane tropical rainforest. Ecological Modelling, 239, 3–13. doi:10.1016/j.ecolmodel.2011.05.005
- Prasantha, H.A., Zhijia, L.I., and Albert WOLFGANG, F.A., 2003. Application of the SWAT Model for River Flow Forecasting in Sri Lanka. Journal of Lake Sciences, 15, 147–154. doi:10.18307/2003.sup18
- Prudhomme, C. and Davies, H., 2009. Assessing uncertainties in climate change impact analyses on the river flow regimes in the UK. Part 2: future climate. Climatic Change, 93, 197–222. doi:10.1007/s10584-008-9461-6
- IPCC (Intergovernmental Panel on Climate Change), 2014. Climate change 2014: synthesis report. In: R.K. Pachauri and L.A. Meyer, eds. Contribution of working groups I, II and III to the fifth assessment report of the intergovernmental panel on climate change. Core Writing Team. Geneva, Switzerland: IPCC.
- Samarasinghea, S.M.J.S., et al., 2010. Application of remote sensing and GIS for flood risk analysis: a case study at Kalu-Ganga River, Sri Lanka. International Archives of the Photogrammetry, Remote Sensing and Spatial Information Science, XXXVIII Part, 8, 110–115.
- Seibert, J., 1999. Regionalisation of parameters for a conceptual rainfall–runoff model. Agricultural and Forest Meteorology, 98–99, 279–293. doi:10.1016/S0168-1923(99)00105-7
- Seibert, J. and Vis, M.J.P., 2012. Teaching hydrological modeling with a user-friendly catchment-runoff-model software package. Hydrology and Earth System Sciences, 16 (9), 3315–3325. doi:10.5194/hess-16-3315-2012
- Skoufias, E., Rabassa, M., and Olivieri, S., 2011. The poverty impacts of climate change: a review of the evidence. Policy Research Working Paper 5622, Poverty Reduction and Equity Unit, Poverty Reduction and Economic Management Network, Washington, DC: The World Bank, 35.
- Sonoda, H., 2007. Evaluation of Kukule Ganga Hydroelectric Power Project, Japan International Cooperation Agency, Available from: http://www.jica.go.jp/english/our_work/evaluation/oda_loan/post/2008/pdf/e_project07_full.pdf [ Accessed 07 August 2015].
- Steele-Dunne, S., et al., 2008. The impacts of climate change on hydrology in Ireland. Journal of Hydrology, 356, 28–45. doi:10.1016/j.jhydrol.2008.03.025
- Tebaldi, C. and Knutti, R., 2007. The use of the multi-model ensemble in probabilistic climate projections. Philosophical Transactions of the Royal Society A: Mathematical, Physical and Engineering Sciences, 365, 2053–2075. doi:10.1098/rsta.2007.2076
- Thompson, J., Crawley, A., and Kingston, D.G., 2015. GCM related uncertainty for river flows and inundation under climate change: the Inner Niger Delta. Hydrological Sciences Journal. doi:10.1080/02626667.2015.1117173
- Todd, M.C., et al., 2011. Uncertainty in climate change impacts on basin-‐scale freshwater resources – preface to the special issue: the QUEST‐GSI methodology and synthesis of results. Hydrology and Earth System Sciences, 15, 1035–1046. doi:10.5194/hess-15-1035-2011
- UNFCCC, 2015. The Paris Agreement. UNFCCC Secretariat. Available from: http://unfccc.int/resource/docs/2015/cop21/eng/l09r01.pdf [ Accessed 12 July 2016].
- Wang, H., et al., 2012. Patterns of varietal adoption and economics of rice production in Asia. Manila: International Rice Research Institute.
- Wickramagamage, P., 2011. Evolution of the Kalu Ganga – Bolgoda Ganga flood plain system, Sri Lanka. Journal of the Geological Society of Sri Lanka, 14, 41–53.