ABSTRACT
Macro-scale river-routing schemes first emerged to channel runoff generated as a by-product from land surface models to oceans. In the past decade, as discharge of major rivers was identified as a suitable parameter to test the performance of the macro-scale land surface models, river-routing received significant attention, with development of multiple schemes. As resolution improves, the possibility of river-routing schemes connecting the global models with watershed issues has emerged as an option. Yet, even as results from these schemes become easily available, a comprehensive overview of their scope and limitation when considering regional or watershed-centric applications is lacking. To address this gap, 18 published river-routing schemes are compared by examining their structure, rationale and limitations. Due to the diverse nature of scheme implementations, a direct comparison of performance is not yet possible. However, features and studies geared towards watershed-scale applications are highlighted. Issues of global to local integration are discussed.
Editor R. Woods Associate editor G. Di Baldassarre
1 Introduction
Emerging water management challenges, stemming from the need to mitigate the impacts of climatic and anthropogenic forcing, cut across several scientific disciplines and multiple spatial scales. While at the fore are the local water resources availability and extreme event concerns, modified and shaped by social–ecological interaction, regional pressures and global changes impact the terrestrial water budgets that underscore the system. Traditionally, the modelling approaches for local and global scales of water resources have evolved separately (Archfield et al. Citation2015) with either simplifying assumptions or unidirectional forcing substituting for the processes at the other scale. Based on downscaling method pathways reviewed in Xu (Citation1999), shows the pathways that may be followed for calculating streamflow based on information flowing between global and local scales. Thus, for local-scale management, the emphasis has been on developing and applying distributed hydrological and hydraulic models for watersheds (Overgaard et al. Citation2006) that rely on downscaled climatic information from global or regional models to obtain climatic forcing datasets. While the local hydrological models can provide the most detailed assessment of the conditions in basins on both quantity and quality of water for decision makers, high data requirements and local technical expertise required to develop, run and maintain these models results in slow proliferation. On the other hand, initially, global climate models (GCMs) primarily relied on simple hydrological assumptions to compute runoff as a means of balancing the water budget. The focus has been on vertical rather than horizontal movement of water on the surface, with runoff routing primarily coming into place to close the water budgets. While, clearly, the water budgets derived from these global models are less than ideal for local-scale water resource management, given the technical and logistical requirements for developing, maintaining and sharing distributed hydrological and hydraulic models for watersheds, increasingly multilateral, bilateral, regional and local organizations turn towards macro-scale models, or their results available on web-based platforms (e.g. NASA, Giovanni Citation2018), for preliminary information about the local water cycle that is otherwise inaccessible to them, or generally unavailable.
Figure 1. Pathways of deriving river discharge information from GCMs. Based on downscaling method pathways reviewed in Xu (Citation1999).
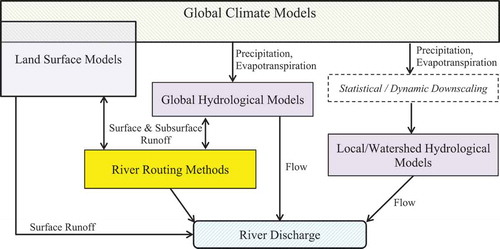
In this context, the improvement and evolution in modelling of land surface processes in GCMs (Pitman Citation2003, Overgaard et al. Citation2006) and development of macro-scale hydrological models (Sood and Smakhtin Citation2015) are important advances. In response to questions on global water security (Griffiths and Lambert Citation2013), its impact on the nutrient cycle (Rabalais et al. Citation2009, Fekete et al. Citation2010) and food security (Islam et al. Citation2007), a class of global models that are attempting to use globally and consistently collected remotely sensed data to address these issues at the global, regional and local scales has started to take shape. Besides precipitation and evapotranspiration, runoff and discharge have become variables of interest from these models and, over the past decades, their capability to route surface water has received significant attention from the research community. Although, at a global scale, river and streamflow may only form a small proportion of the water cycle, locally, they have a direct impact on human society (Li et al. Citation2015). Besides their role in transport of sediment and nutrient, maintenance of terrestrial and freshwater ecosystems, as well as cycles of floods and drought, rivers regulate the balance between water supply and demand. River-routing schemes and methods (RRMs) linked to global/regional models have thus emerged as possibly a key component in connecting the global and local-scale modelling approaches, as well as providing a pathway for assessing local surface water resources and water transport in the rivers of the world (Miller et al. Citation1994, Yamazaki et al. Citation2011) in a globally consistent manner. While Mizukami et al. (Citation2016) and Clark et al. (Citation2015), among others, have provided a brief overview of the types of schemes used for river-routing, as the model results from RRMs and maps generated therefrom become widely and easily available to influence decision making, a comprehensive analysis of the capabilities, features and assumptions used in RRMs is missing to help users make informed judgement of the skill of the models used to produce outputs. The focus of this review is to fill that gap, with Sections 2 and 3 looking at the evolution of the routing schemes, their underlying mechanics, as well as their structure, while Section 4 identifies gaps and opportunities for future developments in RRMs that would continue to make them relevant to the water resources challenges faced around the world.
2 Evolution of routing schemes
Over the past two decades a number of RRMs have been developed – some tightly coupled to particular land surface models or macro-scale hydrological models (referred to collectively hereafter as global models or GMs) – other, more general, open systems are used to route runoff at variable grid resolutions. This review was able to identify at least 18 RRM schemes published between 1996 and the present. This list is not exhaustive, but aims to cover the basic assumption, components and limitations of the current state of the art available for routing flow derived from GMs. provides a summary of the conceptual underpinnings of the 18 RRMs in chronological order, namely the main technical steps and set-up for performing routing. outlines the structural elements of the RRMs including scale/resolution and other processes, besides direct surface flow considered by the RRM. also tabulates any known application – especially for watershed-scale studies – and the GM that the RRM has been developed for or demonstrated with.
Table 1. Conceptual underpinnings of the river-routing models arranged in chronological order.
Table 2. Structural components and known applications of the river-routing models (RRM).
Early land surface models (LSM) focused almost exclusively on the partitioning of energy over the land surface into latent and sensible heat and, thus, led to equations focused on a vertical solution scheme of energy transfer between ground and atmosphere, with no horizontal exchange between neighbouring cells of the LSM. Arnell (Citation1995) recognized that this partitioning capability can be validated against observed precipitation and streamflow data. However, this required the development of runoff routing schemes that could preserve the horizontal transfer time over the model domain to obtain discharge estimates in streams derived from LSM-generated runoff estimates. One of the first most widely adopted approaches in this direction was developed by Lohmann et al. (Citation1996). The underlying assumptions of the Lohmann routing scheme, which sets the basis of the RRMs that follow, is that all the nonlinear processes associated with flow generation can be resolved into the vertical solution scheme of the LSM – leaving the RRM to deal only with routing water over the surface. Lohmann et al. (Citation1996) then expressed the routing process as a linear and time-invariant transfer function modelled on unit hydrographs (also referred to as impulse response function, or IRFs) firstly within each grid and then connecting each grid through a river network. This is achieved by using a linear model of “fast” and “slow” components of the measured discharge as the basis for the in-grid flow’s IRF and linearized one-dimensional (1D) diffusive wave equation for the inter-grid flow’s IRF. While the model does not explicitly account for subsurface flow, the baseflow contribution from each grid cell is reflected via a “slow” flow component of the in-grid routing step. The main parameters of the routing scheme are the ratio between the “fast” and “slow” flow components, wave velocity and diffusivity estimates for each grid cell – fixed values for which are derived during an optimization procedure for the region/watershed being studied. As discharge at the river outlet (or main gauging stations) was the key output required from this scheme, IRFs proved to be a computationally efficient approach to adopt – leading to the development of more sophisticated “source-to-sink” schemes (Naden et al. Citation1999, Olivera et al. Citation2000, Gong et al. Citation2009).
Storage-based routing schemes demonstrate the first attempts to capture spatial distribution of flow. summarizes the governing equations employed by these models. These schemes relied on various simplifications of the 1D kinematic wave routing (KWR) equation without distinguishing between overland and channel flow. Oki and Sud (Citation1998) demonstrate a simple storage-based scheme (referred to as TRIP here) with a priori constant velocity for routing water between grids along the “TRIP network” – a global river channel network developed at 1-degree (1°) resolution. Under this scheme, flow is modelled based on 1D KWR, which reduces to a linear reservoir equation with the constant velocity assumption. The cell-by-cell calculation increases the computational load considerably compared to “source-to-sink” schemes; however, the formulation of the equations is simpler to implement and has been widely used in LSMs. (Pappenberger et al. Citation2010 identified at least 14 studies using variants of this scheme.) The river transport model (RTM; Branstetter Citation2001) – the standard routing method in the community land model (CLM) – uses a similar simplified 1D KWR approach; however, it allows for user-derived flow networks at finer scales resulting in more detailed channel networks. The imposed linearity, with lack of representation of spatio-temporal variability due to the calibrated or prescribed constant velocity approach, was replaced by a Manning’s equation-based approach developed by, among others, Ngo-Duc et al. (Citation2007) and Arora and George (Citation1999). Implemented in TRIP2 (Pappenberger et al. Citation2010), TRIP CHS (Decharme et al. Citation2010) and WaterGAP (Verzano et al. Citation2012), the temporally variable routing velocity is now estimated using Manning’s equation dependent on channel width and roughness parameters. The channel width is, in turn, estimated by geomorphological functions assuming a rectangular (TRIP2 and TRIP CHS) or trapezoidal (WaterGAP) cross-section. TRIP2 and TRIP CHS also introduced an element of groundwater dynamics by incorporating a linear reservoir to represent exchange with the subsurface in each grid. Gong et al. (Citation2009) strongly critiqued storage-based schemes due to their inability to account for convective time delay over river reaches and scale dependency inherent to slope and reach length calculation being dependent on spatial resolution used for channel network derivation. The ARTS model (Getirana et al. Citation2014) attempts to incorporate a time delay parameter, τ, for a grid cell’s flow release from storage while using a nonlinear Muskingum-Cunge (MC) method for inter-grid routing. The parameters for the nonlinear MC routing method attempt to account for the physical constraints of the flow network and are re-calculated for each reach and discharge; however, the time delay parameter, τ, which attempts to characterize the physically-based process occurring at the subgrid-scale routing (Getirana et al. Citation2012), including convective time delay, is calibrated as a single value for the entire model. The G2G model (Bell et al. Citation2007) attempts to use hyper-resolution (1-km resolution grid) – albeit with a pre-assigned wave celerity assumption – to improve flow representation and account for convective time delay. G2G is among the first schemes to distinguish overland and channel flow in its set-up (with complementary groundwater storage) and solves a 2D KWR using finite difference with different celerities for overland and channel flow. However, this comes at a high computational cost, requiring a 15-min time step throughout the domain. Scale dependency remains an issue for simple storage-based cell-by-cell routing schemes. Nevertheless, the spatial distribution of flow (and, in some cases, depth and velocity) provided by them has opened up possibilities for regional and local application in water resource management.
Table 3. Governing equations for storage-based routing schemes.
All the above schemes for flow routing are tightly coupled to the scale and grid, with the GM providing the runoff input. Considering recognition of scale dependency, improving computational efficiency and demonstration of the potential to obtain spatially-distributed flow directly from GMs has led to the gradual decoupling of routing schemes from GMs in two ways. The first is in terms of the grid and scale they operate on. These routing methods using a different grid from the GM to route flow, incorporating flow length distributions, sub-basin polygons, stream vectors, terrain raster, etc. to capture flow and channel dynamics in more detail, while being able to provide routing information an any point within the basin. Secondly, these methods explicitly account for at least two different routing processes – overland flow and channel flow – solving different sets of equation for each at the grid/inter-grid level. The MOSART (Li et al. Citation2013), KWR CLM (Ye et al. Citation2013) and DRTR models (Wu et al. Citation2014) are examples of routing schemes using these approaches while still primarily using the 1D KWR equations for routing flow, however, now solving these equations at various levels. The KWR CLM and DRTR models solve the 1D KWR at two levels: the hillslope and the main channel for KWR CLM, and tributaries and the main channel for the DRTR model. The MOSART model applies the 1D KWR at three levels: hillslopes, tributaries and main channel. All three schemes rely on higher-resolution data to determine the characteristics used to solve the 1D KWR, such as delineating sub-basins (for KWR CLM), calculating average hillslope, average length of tributaries and main channel, etc. The MOSART and DRTR models rely on a hierarchical dominant river tracing (DRT) algorithm (Wu et al. Citation2011, Citation2012) to generate spatial scale-consistent river network generation. The novelty of the DRT algorithm is in its attempt to utilize information on global and local drainage patterns from available hydrography at finer spatial scales/resolution to determine flow directions, basin area, basin shape and river lengths at coarser scales. The original baseline hierarchical drainage structure is preserved by tracing the entire flow path from headwater to river mouth at fine scale while prioritizing successively higher-order basins and rivers for tracing, allowing consistent sub-grid parameterization of RRMs. The routing scheme by Wen et al. (Citation2012) – referred to here as “Wen routing” – introduces a novel element for the overland flow component by using a higher-resolution DEM to derive statistical distributions for overland flow paths within each LSM grid cell using a multidirectional flow algorithm. Wen routing then uses 1D KWR for in-grid overland flow alongside the distributions, where the range of flow lengths derived from the distribution helps account for different arrival times to the channel while keeping the computational load low.
Two recent models, mizuRoute (Mizukami et al. Citation2016) and HYPERstream (Piccolroaz et al. Citation2016), attempt to provide “general” flow routing models that can couple to any GM runoff results available. Additionally, they can leverage both raster and vector data with the computationally efficient IRF type methods of the early noted “source-to-sink” methods. Discharge values can be reported for river segments (mizuRoute) or points defined by the user (HYPERstream). Both models use an IRF-based method to route flow from hillslope/overland to the channel over units (sub-basins or grids) derived from the highest quality terrain/network data available. While HYPERstream uses a constant velocity assumption to aggregate flows along the channel network, mizuRoute allows for a Lohmann type 1D diffusive wave approximation based IRF or a kinematic wave tracking (KWT) method. The KWT method computes wave celerity for each sub-basin as the basis of routing. However, Mizukami et al. (Citation2016) reported that the KWT component’s sensitivity to parametrization was substantial. Another significant attempt to use a vector river network with an added component of parameter estimation was through RAPID (David et al. Citation2011). The RAPID model uses over 20 000 gauges situated across river basins in the contiguous USA to automatically optimize Muskingum parameters for the RRM and perform discharge computations.
The work by Paiva et al. (Citation2011) on coupling a full 1D hydrodynamic model to a large-scale hydrological model, and the 1D diffusive equation of CaMa-Flood (Yamazaki et al. Citation2011) represent attempts to move beyond the simpler representation of flow hydrodynamics seen so far and expand the application in the direction of flood inundation mapping. These models require significant data and computational resources. However, CaMa-Flood has been successfully applied for flood risk assessment on major basins of the world by Hirabayashi et al. (Citation2013), albeit without accounting for human intervention in river systems. On this front, HydroROUT (beta version discussed in Lehner and Grill Citation2013) hopes to build with it the resources on inundation areas, dams and reservoirs, etc. into a global database that can be harnessed for detailed global-scale modelling.
3 Comparison of features
3.1 Resolution and scale
One of the main distinctions between RRMs, besides the routing equations employed, is the grid used for routing the flow. summarizes the key aspects of this. The spatial resolutions of the routing schemes are derived as a combination of the resolution of the GM that drives it and the river network description used to route the flow. Nine of the 18 schemes reviewed have a fixed spatial resolution reported with the scheme developed around a pre-defined network. Five of these nine schemes run at resolutions greater than or equal to 0.5°. The remaining schemes are able to run at multiple spatial scales depending on the requirements of the case study (global, regional or basin), or the forcing data available. While most schemes currently use a grid-based format for routing flow, vector data have also been demonstrated in at least four schemes – HydroROUT, RAPID, mizuRoute and HYPERstream. Use of vector data has allowed these schemes to post results at 1-km resolution and finer. Only one grid-based method, G2G, reports a spatial resolution of up to 1 km.
Closely linked to spatial resolution is the river network description used to route the flow. In the coarse spatial resolution models, TRIP or TRIP-like eight-directional drainage networks derived from the underlying terrain model are used. The HydroSHEDS river network, derived from SRTM data, has been used in three of the schemes. Nine of the 18 schemes use their own method of deriving river flow networks from the terrain data available, ranging from using SRTM and HYDRO1K terrain models as a base to GMs underlying terrain information. The MOSART and DRTR models propose the use of a hierarchical dominant river tracing (DRT) algorithm that aims to maintain a scale-consistent upscaling of the river network (described briefly in Section 2). The HYPERstream and mizuRoute models allow users to provide the best available topographic data – a hybrid of grid and vector layers – to derive the river-routing network.
Almost all the schemes are able to report discharges at the outlet at a daily time step. Nine of the 18 schemes reported capability of producing outputs at a sub-daily time step – typically hourly or 3-hourly. Also, almost all the reviewed schemes are able to report discharge at multiple points within the watersheds, albeit limited by the constraints of spatial resolution. At least 10 of the 18 schemes are able to calculate variable flow velocity within their domain and a further four – TRIP CHS, DRTR, Paiva routing and CaMa-Flood – attempt to estimate some measure of water depth or area of flood inundation as part of their schemes. Eight of the routing schemes reviewed have been applied at the global scale, with Lohmann routing, TRIP and RTM available as standard routing methods in variable infiltration capacity (VIC), interaction soil–biosphere–atmosphere (ISBA) and community land model (CLM) LSMs, respectively. The TRIP2, TRIP CHS, MOSART and DRTR models have been used at the global scale by coupling with the European Centre for Medium-Range Weather Forecasts (ECMWF) model, ISBA, CLM4 and VIC. Six of the schemes have been demonstrated on a regional multi-basin level, with G2G in the British Isles, WaterGAP routing over Europe, HydroROUT and KWR CLM over China, mizuRoute and RAPID over the contiguous United States and the CaMa-Flood over 29 major basins around the world. The three remaining schemes have been demonstrated on single, or parts of, large watersheds, such as the Amazon Basin by Paiva routing.
3.2 Subsurface and lateral flows
Beyond routing of surface flow, a few of the RRMs attempt to incorporate a more complete description of surface–subsurface dynamics (). Nine schemes have some representation of subsurface dynamics within the grids that will impact the generation of flow into the channel. IRF-based methods, such as Lohmann routing, use a calibrated “slow” component to capture the time delay in subsurface flow releasing water into the channel. TRIP, RTM and DRTR allow the deep drainage/baseflow values from their LSM to flow into the surface, while TRIP2, TRIP CHS and ARTS add another linear reservoir to represent groundwater storage that links to the LSM’s drainage. This linear reservoir then releases baseflow into the surface routing schemes. G2G attempts to use a subsurface 2D kinematic flow with constant pre-defined velocity below overland and river cells that can mimic two-directional exchange of water between surface and subsurface.
Seven schemes report capability to incorporate some effects of lateral flow from other surface storage features that will impact flow dynamics, such as reservoirs, wetlands, lakes and flood plains. WaterGAP uses linear and nonlinear storage to represent lakes, reservoirs and wetland (further described in Döll et al. Citation2003), which connects to its routing scheme. HydroROUT also intends to account for lake volume and obstruction to flow caused by dams and reservoirs. TRIP CHS and DRTR have a floodplain reservoir that allows a two-way interaction with the LSM – where the water entering the floodplain can impact the evapotranspiration and infiltration over the surface. However, this capability has been reported as “not activated” in DRTR at the current stage. CaMa-Flood and Paiva routing allow for overflow from the channel into the floodplain (without interacting with the LSM), thus allowing for floodplain inundation dynamics. Only HydroROUT reports attempts to couple a contaminant fate model to the routing scheme – an application that can open a new avenue of application for routing schemes, as discussed in Section 4.
4 Gaps, opportunities and trends for regional application
With advances in the routing methods documented in the previous two sections, the prospect of applying them in regional and local management of water resources – in the context of (1) filling gaps in information where none exists, and (2) bridging local, regional and global forcing data on the water resources – has emerged as a distinct possibility. In the domain of flood risk management, GFMS (Global Flood Monitoring System) and Aqueduct Global Flood Analyzer are examples of web-based dissemination platforms that provide access to processed flood risk data derived from RRMs routing runoff obtained from GMs. Ward et al. (Citation2015) gave an illustrative example of how these results have been used to engage stakeholders and identify local regions at high risk that require further detailed study. It is clear that these routing schemes are not yet capable of providing actionable input on interventions for changing or modifying the scale of flood protection (e.g. height of dykes required); nevertheless, these developments are encouraging as they have the ability to highlight challenges in disaster risk management.
Therefore, considering their potential to provide timely information for more holistic local and regional freshwater management, three areas are identified where continued integration and development in RRM and linked tools may lead to actionable and relevant information.
4.1 Improving local controls on flow and validation
With basins representing 59% of global rivers having large dams (Grill et al. Citation2015) and many more having smaller regulating structures on their stream network, reservoir storage and operation directly influence instream flow (Vogel et al. Citation2007), and are a significant factor in shaping the overall behaviour of the hydrological regime. While Lohmann routing implicitly is able to incorporate some influence of these interventions, none of the newer and more detailed methods report their inclusion; instead (if at all) they rely on the underlying GMs to account for their influence. Of the 12 macro-scale hydrological models that Sood and Smakhtin (Citation2015) reviewed, 50% had some capability to include reservoir dynamics. Even when applied in the global models, due to varying levels of operation information available at the global level, the representation can be reduced to simple calibrated parameters. Nazemi and Wheater (Citation2015b) provided a detailed review of algorithms used for water supply and allocation modelling in GMs and noted considerable limitations in representing streamflow in regulated catchments. For local and regional application, moving towards the ability to (1) represent interventions, and (2) incorporate local knowledge on their operation is the key opportunity for RRMs to make them relevant for long-term water resource management and planning.
In general, most studies rely on goodness of fit of model prediction and observed discharge at the outflow to validate the GM–RRM coupling. Some studies extend this and use an instance of a model calibrated to discharge from a regulated watershed as a proxy for representing current conditions. As Vogel and Sankarasubramanian (Citation2003) demonstrated, fitness can be influenced by model error and the advocated using a model’s ability to reproduce the statistical characteristics of the input and output data as the quantitative method to accept or reject a model’s performance rather than a goodness of fit validation which may produce misleading results. A simple water balance model coupled to a storage-based hydrological routing model (to mimic the GM–RRM coupling), with and without basic reservoir operations for the Dongjiang River Basin (China), is used here to test the difference in ability to represent hydrological processes by the approach advocated by Vogel and Sankarasubramanian (Citation2003). This illustrative set-up for Dongjiang ()) uses the HydroBASIN Level 8 product (Lehner and Günther 2013) to describe the drainage network – where each sub-basin is analogous to a cell/HRU/sub-basin used in GMs. The water balance component of the set-up uses the “abcd” model (Thomas Citation1981) for each sub-basin. The runoff thus generated for each sub-basin is then routed through the storage-based routing method along the stream network, transferring flow to the basin outlet. In the setup “with basic reservoir operation”, the release from the two main reservoirs in the basin is modelled based on a policy of “fixed target release”. This requires the reservoirs to release a fixed volume per month to fulfil downstream demand, however, with the ability for overspill or reduced discharge depending on reservoir storage status. In the set-up “without basic reservoir operation”, no steps are taken to represent the impact of the reservoirs on the flow.
Figure 2. Dongjiang basin and validation of model with and without basic reservoir representation for the two main reservoirs based on discharge at downstream gauge at Boluo.
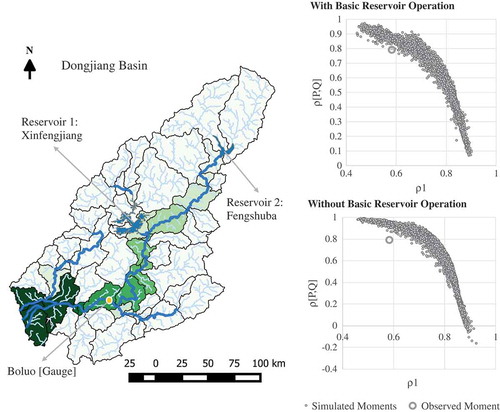
The model set-ups are then evaluated in their ability to reproduce the serial correlation between discharge (ρ1 of Q) and the cross-correlation between precipitation (P) and discharge (Q). The ability to maintain these statistical characteristics reflects on the model’s fit for long-term water resource planning. Using 10 000 Monte Carlo experiments, which generate parameter values from the range documented in , model simulations are realized and the moments plotted in ). As seen in , the goodness of fit achieved between discharge observed at Boluo and a calibrated simulation using the “without basic reservoir operation” set-up appears fairly similar to the other set-up; however, based on its failure to reproduce the observed moment, the set-up is rejected. Alongside developing the idea of locally-sensible control representation, the analysis advises caution on using calibration and validation on runoff estimates produced directly from GMs at a daily time step or lower, without adequately considering any routing scheme to derive discharge from runoff. While the “fit” observed through the calibration process may be satisfactory, this analysis indicates that that may not necessarily be due to the skill of the model to simulate the basin hydrology.
Table 4. Range of parameters of the abcd model for Monte Carlo simulations.
Figure 3. Average monthly discharge at Boluo with Pearson correlation coefficient and NSE between monitored data and simulated results.
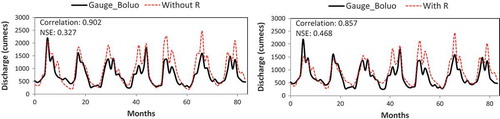
Figure 4. High-resolution tracking of surface water dynamics using remotely sensed optical imagery, demonstrated using 30-m resolution LandSAT imagery over the Pearl River Estuary and Lower Dongjiang River Basin. Map extracted from results of an algorithm tracking change of Water Occurrence Change Intensity between 1984 and 2015 developed by Pekel et al. (Citation2016).
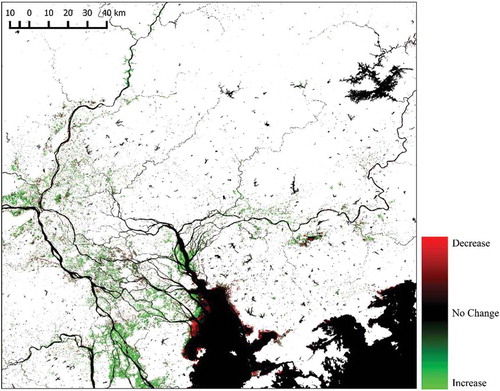
Thus, a dynamic link between global runoff generation and local discharge routing will likely produce the best results. Development and proliferation of cloud-based computing platforms opens the option where GMs relying on global data sources to generate runoff estimates are given a spatially-bounded interface for local user input on the regulation of the stream network which can influence and improve the streamflow estimates derived from RRMs. The web-based adoption of METRIC, in the form of EEflux (Kilic et al. Citation2016) for calculating actual evapotranspiration, using the Google Earth Engine as the computing platform and some user input for calibration, in some respects can serve as a template for the future direction of RRM development that incorporates local input into a global dataset.
4.2 Continuing integration with remotely sensed data
With data assimilation (DA) algorithms being applied in a number of GMs for improved model prediction by integrating available observations of the state of the system, refining the estimates of river flow is one of the key areas in which GMs/RRMs can leverage and benefit from the developments in remote sensing of freshwater systems. While no published study was found on attempts to use DA with RRMs, the number of applications demonstrating the DA algorithm applied on hydraulic models with remotely sensed data has been growing over the past decade (Grimaldi et al. Citation2016). Among these, remotely sensed observations of extent and level of water – derived from optical imagery, radar altimeters, LiDAR, SAR and other active/passive microwave imagery – are assimilated in models using filtering approaches. The selection of the filtering algorithms is based on (a) the filter’s ability to account for uncertainty of the remotely sensed data, and (b) the physical dimensions of the model domain. The ensemble Kalman filter (EnKF) or particle filter (PF), or variants thereof, have emerged as prominent algorithms (see the review by Grimaldi et al. Citation2016). Both global and local formulations of weighting are possible in the filter-based DA algorithms. For RRMs, a global filter is the more likely mode of application where, in general terms, the likelihood of model realization is based on its ability to correctly predict state variables (water level, depth, velocity) over the entire river domain. In the applications of DA reviewed, the updating process of the model consists of either (i) updating state variables only or, state variable and input conditions, at the time step when new information is made available by remotely sensed data; (ii) constant error correction of state variables only or, state variable and input conditions, based on an error forecast model; or (iii) parameter updating of the simulating model to improve performance. Among these, error correction models appear to have had the most success in improving predictions, with just state/input updates at time of availability of new information dampened out after a few time steps and parameter updates not having performed as well as expected (with the limited number of case studies carried out so far).
Studies by Durand et al. (Citation2008) and Biancamaria et al. (Citation2011), among others, have demonstrated significant improvements in the root mean square error (RMSE) of simulated discharge from river hydraulic models assimilating remotely sensed river elevation and slope data. These studies used a synthetic version of radar-based (Ka-band, ~35 GHz) satellite altimetry data of surface water height – of the form that can be expected to be available globally once the Surface Water and Ocean Topography (SWOT) mission launches in 2020 (Biancamaria et al. Citation2016). Even with its limitations on stream width for stream elevation measurements (at least 100 m width) and orbital measurements every ~11 days, the estimated high vertical elevation and water-surface slope accuracy (1 cm/km2 and 0.1 cm/km river length, respectively) will open up avenues for monitoring freshwater systems continually and in most weather conditions.
Other approaches for obtaining discharge estimates from remotely sensed data revolve around using SAR and optical imagery. Here, from the data, “effective channel width” can be established and, with estimated hydraulic geometry relationships, discharge estimates can be derived from width alone. Gleason and Smith (Citation2014) outlined such an approach, named “At-Many-Station-Hydraulic Geometry” (AMGH), and demonstrated the process for three rivers using LandSAT imagery, obtaining RMSE of discharge estimates in the 20–40% range. With a number of optical instrument-carrying satellites (such as LandSAT, SPOT, SENTINEL 2) monitoring land surface at high resolution, the change in wetted area is trackable and becoming more easily accessible (Fig. 4; source: Pekel et al. Citation2016) for future development and incorporation in DA algorithms.
4.3 Evaluating impact on coupled human and natural systems
Even with the limitations in reservoir and other regulating infrastructure representation in GMs (discussed in Section 4.1), a number of attempts have been made to use these models for estimating water supply and demand for human, agricultural and industrial consumption. Nazemi and Wheater (Citation2015a, Citation2015b) provided a detailed overview of the algorithms and approaches that have been developed for this, highlighting their potential as well as challenges for a greater role in determining water supply from ecosystems. They concluded that the current capability of GMs to account for water demand is limited and estimates of water supply uncertain due to limitations of data, model uncertainty and propagation of bias across integrated models. However, they identified that considering water resources jointly in terms of demand and supply was the right direction to take, with availability of regional-scale data for model development, diagnosis and validation the next steps needed in this domain of research.
Besides water supply and demand, sediment and water quality regulation remain two key areas where routing methods along with their GMs can make significant contributions in improving management. These are also the areas where, generally, local expertise in modelling is limited. Of the reviewed literature, only one of the RRMs mentioned attempts to build in capability for stream water quality projections. The main challenge with water quality, similar to reservoir operation, is that the forcing datasets are context dependent and in many cases highly localized. However, a generic framework to track sources and transport patterns in a river basin may be possible using the information available globally. The simple water balance model coupled to a storage-based hydrological routing model used in Section 4.1 has been extended to include a water quality module based on the InVEST ecosystem services model (Tallis et al. Citation2013) for non-conservative pollutants. The loadings for this model are generated based on population density (SEDAC global population layer; CIESIN – Columbia University Citation2015), agricultural land (land cover map; ESA Climate Change Initiative – Land Cover project 2014) and per capita load estimates (Wu and Chen Citation2013). depicts the estimated load from each sub-basin for total phosphorus (TP) and its routing through the river network. The critical drawback of this approach is the gap between the granularity at which the adsorption, absorption and decay processes work and the scale of the routing model. Hence, these processes are controlled by a “decay-rate” parameter, which may require to be calibrated to local data – although general patterns of water quality will likely be primarily driven by magnitude of input loads. Clearly, this remains an area that will need more effort in the coming years. Two modelling approaches that have used hydrological data from GMs to make inroads into this domain should be considered at this point. The first is a stream temperature model by Yearsley (Citation2012), which applies a 1D semi-Lagrangian model using time-dependent equations for conservation of thermal energy on flow estimates derived from VIC. The presented case studies show potential in analysing the impact of climate change on stream temperatures despite significant bias in simulated stream temperatures due to uncertainty in estimation of headwater temperature and magnitude of stream speed during low-flow periods. The second approach is WorldQual (Voß et al. Citation2012), which couples with WaterGap to gauge both conservative and non-conservative pollutants. Applied for estimates of in-stream biological oxygen demand (BOD) and faecal coliform for Latin America, Africa and Asia (WWAP, Citation2017), the WorldQual model attempts to use simple equations that are reflective of data availability at continental scales to gauge impacts on river water quality in response to anthropogenic loading and flow dilution. Voß et al. (Citation2012) reported overall good fit of the spatial/temporal water quality pattern based on observed and simulated values in Europe, despite the model parameters for water quality not having been calibrated for local conditions.
5 Conclusions
The growing sophistication of process representation in land surface models and macro-scale hydrological models, higher accuracy of atmospheric data available to force them, alongside improving the resolution of the models, have highlighted their potential in local and regional water management. River-routing methods that were first introduced as a validation approach for the global and macro-scale models based on discharge comparisons for major rivers have continued to evolve over the past two decades, improving the representation of river flow dynamics. Structurally, the models differ in channel routing methods, resolution and characterization of the drainage network, as well as in the way they account for and integrate surface and subsurface drainage from the global models.
Most of the routing methods have been applied globally, or at least regionally, and validated against local discharge. Almost all the approaches produce flows at least at a daily time step and have the ability to provide discharge not just at the basin outlet, but at any point within the drainage network. However, as the analysis based on the method of Vogel and Sankarasubramanian (Citation2003) suggests, the calibration–validation approach alone may not be sufficient to establish the fitness of the modelling set-up to represent flow dynamics, especially in the absence of representation of regulating structures in the model or routing schemes.
Incorporating representation of regulating structures and continuing integration of remotely sensed data in global models with DA algorithms can help improve the flow representation, to be locally and regionally more suitable for water supply, demand and extreme event analysis and risk management. While improvement in the model output using DA is something that can be carried out centrally, to truly make the representation of regulating structures relevant to local management, approaches that allow tiered input into these set-ups will be required, where local stakeholders can modify the operation rules to reflect ground conditions and requirements. Emergence of programmable GIS platforms such as Google Earth Engine and the tools being developed on them sets a precedent of how a hybrid global-to-local set-up can be achieved.
Moving beyond routing of flows to include other issues related to delivery of ecosystem services by water, such as regulation of water quality and sediment transport, can be envisioned to be the next step in the development of these schemes, with initial attempts in this direction already hinted at in published literature. These developments will help fill some critical gaps in local and regional management of water resources.
Disclosure statement
No potential conflict of interest was reported by the author.
Additional information
Funding
References
- Archfield, S.A., et al. 2015. Accelerating advances in continental domain hydrologic modeling. Water Resources Research, 51 (12), 10078–10091. doi:10.1002/2015WR017498
- Arnell, N.W., 1995. River runoff data for the validation of climate simulation models. In: H.R. Oliver and S.A. Oliver, eds. The role of water and the hydrological cycle in global change. Berlin Heidelberg: Springer, 349–371.
- Arora, V.K. and George, J.B., 1999. A variable velocity flow routing algorithm for GCMs. Journal of Geophysical Research: Atmospheres, 104 (D24), 30965–30979. doi:10.1029/1999JD900905
- Bell, V.A., et al., 2007. Development of a high resolution grid-based river flow model for use with regional climate model output. Hydrology and Earth System Sciences, 11 (1), 532–549. doi:10.5194/hess-11-53two-2007
- Biancamaria, S., et al., 2011. Assimilation of virtual wide swath altimetry to improve Arctic river modeling. Remote Sensing of Environment, 115 (2), 373–381.
- Biancamaria, S., Lettenmaier, D.P., and Pavelsky, T.M., 2016. The SWOT mission and its capabilities for land hydrology. Surveys in Geophysics, 37 (2), 307–337. doi:10.1007/s10712-015-9346-y
- Branstetter, M.L., 2001. Development of a parallel river transport algorithm and applications to climate studies PhD Dissertation. University of Texas.
- Center for International Earth Science Information Network - CIESIN - Columbia University, 2015. Gridded population of the world, version 4 (GPWv4): population density, beta release. Palisades, NY: NASA Socioeconomic Data and Applications Center (SEDAC). doi:10.7927/H46T0JKB
- Clark, M.P., et al., 2015. Improving the representation of hydrologic processes in earth system models. Water Resources Research, 1–28. doi:10.1002/2015WR017096.Received
- David, C.H., et al., 2011. River network routing on the NHDPlus dataset. Journal of Hydrometeorology, 12 (5), 913–934. doi:10.1175/2011JHM1345.1
- Decharme, B., et al. 2010. Global evaluation of the ISBA-TRIP continental hydrological system. Part II: uncertainties in river routing simulation related to flow velocity and groundwater storage. Journal of Hydrometeorology, 11 (3), 601–617. doi:10.1175/2010JHM1212.1
- Decharme, B., et al., 2012. Global off-line evaluation of the ISBA-TRIP flood model. Climate Dynamics, 38 (7–8), 1389–1412. doi:10.1007/s0038two-011-1054-9
- Döll, P., Kaspar, F., and Lehner, B., 2003. A global hydrological model for deriving water availability indicators: model tuning and validation. Journal of Hydrology, 270 (1), 105–134. doi:10.1016/S0022-1694(02)00283-4
- Durand, M., et al., 2008. Estimation of bathymetric depth and slope from data assimilation of swath altimetry into a hydrodynamic model. Geophysical Research Letters, 35 (20). doi:10.1029/2008GL034150
- Fekete, B.M., et al., 2010. Millennium ecosystem assessment scenario drivers (1970–2050): climate and hydrological alterations. Global Biogeochemical Cycles, 24, 4. doi:10.1029/2009GB003593
- Getirana, A.C.V., et al., 2012. The hydrological modeling and analysis platform (HyMAP): evaluation in the Amazon basin. Journal of Hydrometeorology, 13(6), 1641–1665. doi:10.1175/JHM-D-1221.1
- Getirana, A.C.V., Boone, A., and Peugeot, C., 2014. Evaluating LSM-based water budgets over a west african basin assisted with a river routing scheme. Journal of Hydrometeorology, 15 (6), 2331–2346. doi:10.1175/JHM-D-14-0012.1
- Gleason, C.J. and Smith, L.C., 2014. Toward global mapping of river discharge using satellite images and at-many-stations hydraulic geometry. Proceedings of the National Academy of Sciences of the United States of America, 111 (13), 4788–4791. doi:10.1073/pnas.1317606111
- Gong, L., et al., 2009. Large-scale runoff routing with an aggregated network-response function. Journal of Hydrology, 368 (1), 237–250. doi:10.1016/j.jhydrol.2009.02.007
- Griffiths, J. and Lambert, R., 2013. Free flow: reaching water security through cooperation. Paris: UNESCO. Available from: http://unesdoc.unesco.org/images/0022/002228/222893e.pdf
- Grill, G., et al., 2015. An index-based framework for assessing patterns and trends in river fragmentation and flow regulation by global dams at multiple scales. Environmental Research Letters, 10 (1), 15001. doi:10.1088/1748-9326/10/1/015001
- Grimaldi, S., et al., 2016. Remote sensing-derived water extent and level to constrain hydraulic flood forecasting models: opportunities and challenges. Surveys in Geophysics, 37 (5), 977–1034. Springer Netherlands. doi:10.1007/s1071two-016-9378-y
- Guo, J., Liang, X., and Leung, L.R., 2004. A new multiscale flow network generation scheme for land surface models. Geophysical Research Letters, 31 (23). doi:10.1029/2004GL021381
- Hirabayashi, Y., et al., 2013. Global flood risk under climate change. Nature Climate Change, 3 (9), 816–821. Nature Publishing Group. doi:10.1038/nclimate1911
- Horizon Systems Corporation, 2007. National Hydrography Dataset Plus: Documentation. Available from: http://www.horizon-systems.com/nhdplus/[Accessed January 2018].
- Islam, M.S., et al., 2007. A grid-based assessment of global water scarcity including virtual water trading. Water Resources Management, 21 (1), 19. doi:10.1007/s11269-006-9038-y.
- Kilic, A., et al., 2016. METRIC-EEFLUX. Available from: http://eeflux-level1.appspot.com/ [Accessed January 2017].
- Lehner, B. and Grill, G., 2013. Global river hydrography and network routing: baseline data and new approaches to study the world’s large river systems. Hydrological Processes, 27 (15), 2171–2186. doi:10.1002/hyp.9740
- Li, H., et al., 2013. A physically based runoff routing model for land surface and earth system models. Journal of Hydrometeorology, 14 (3), 808–828. doi:10.1175/JHM-D-1two-015.1
- Li, H., et al., 2015. Evaluating global streamflow simulations by a physically based routing model coupled with the community land model. Journal of Hydrometeorology, 16 (2), 948–971. doi:10.1175/JHM-D-14-0079.1
- Lohmann, D., NolteHolube, R., and Raschke, E., 1996. A large-scale horizontal routing model to be coupled to land surface parametrization schemes. Tellus Series a-Dynamic Meteorology and Oceanography. doi:10.1034/j.1600-0870.1996.t01-3-00009.x
- Lohmann, D., et al., 1998. Regional scale hydrology: I. Formulation of the VIC-2l model coupled to a routing model. Hydrological Sciences Journal, 43 (1), 131–141. doi: 10.1080/02626669809492107
- Masutomi, Y., et al. 2009. Development of highly accurate global polygonal drainage basin data. Hydrological Processes, 23 (4), 57two–584. doi:10.1002/hyp.7186
- Miller, J.R., Russell, G.L., and Caliri, G., 1994. Continental-scale river flow in climate models. Journal of Climate, 7 (6), 914–928. doi:10.1175/1520-0442(1994)007<0914:CSRFIC>2.0.CO;2
- Mizukami, N., et al., 2016. mizuRoute version 1: a river network routing tool for a continental domain water resources applications. Geoscientific Model Development, 9, 2223–2238. doi:10.5194/gmd-9-2223-2016
- Naden, P., et al. 1999. River routing at the continental scale: use of globally-available data and an a priori method of parameter estimation. Hydrology and Earth System Sciences Discussions, 3 (1), 109–123. doi:10.5194/hess-3-109-1999
- NASA Giovanni, 2018. Giovanni: The bridge between Data and Science. Available from: https://giovanni.gsfc.nasa.gov/giovanni/. [Accessed January 2018].
- Nazemi, A. and Wheater, H.S., 2015a. On inclusion of water resource management in earth system models -part 1: problem definition and representation of water demand. Hydrology and Earth System Sciences, 19 (1), 33–61. doi:10.5194/hess-19-33-2015
- Nazemi, A. and Wheater, H.S., 2015b. On inclusion of water resource management in earth system models part 2: representation of water supply and allocation and opportunities for improved modeling. Hydrology and Earth System Sciences, 19 (1), 63–90. doi:10.5194/hess-19-63-2015
- Ngo-Duc, T., Oki, T., and Kanae, S., 2007. A variable streamflow velocity method for global river routing model: model description and preliminary results. Hydrology and Earth System Sciences Discussions, 4 (6), 4389–4414. doi:10.5194/hessd-4-4389-2007
- Oki, T. and Sud, Y.C., 1998. Design of total runoff integrating pathways (TRIP)—A global river channel network. Earth Interactions, 2 (1), 1. doi:10.1175/1087-3562(1998)002<0001:DoTRIP>2.0.CO;2
- Olivera, F., Famiglietti, J., and Asante, K., 2000. Global-scale flow routing using a source- to-sink algorithm. Water Resources Research, 36 (8), 2197–2207. doi:10.1029/2000WR900113
- Overgaard, J., Rosbjerg, D., and Butts, M.B., 2006. Land-surface modelling in hydrological perspective? a review. Biogeosciences, 3 (2), 229–241. doi:10.5194/bg-3-229-2006
- Paiva, R.C.D., Collischonn, W., and Tucci, C.E.M., 2011. Large scale hydrologic and hydrodynamic modeling using limited data and a GIS based approach. Journal of Hydrology, 406 (3), 170–181. doi:10.1016/j.jhydrol.2011.06.007
- Pappenberger, F., et al., 2010. Global runoff routing with the hydrological component of the ECMWF NWP system. International Journal of Climatology, 30 (14), 2155–2174. doi:10.1002/joc.2028
- Pekel, J.-F., et al., 2016. High-resolution mapping of global surface water and its long-term changes. Nature, 540, 418–422. doi:10.1038/nature20584
- Piccolroaz, S., et al., 2016. HYPERstream: a multi-scale framework for streamflow routing in large-scale hydrological model. Hydrol Earth Systems Sciences, 20, 2047–2061. doi:10.5194/hess-20-2047-2016
- Pitman, A.J., 2003. The evolution of, and revolution in, land surface schemes designed for climate models. International Journal of Climatology, 23 (5), 479–510. doi:10.1002/joc.893
- Pokhrel, Y., et al. 2012. Incorporating anthropogenic water regulation modules into a land surface model. Journal of Hydrometeorology, 13 (1), 255–269. doi:10.1175/JHM-D-11-013.1
- Rabalais, N.N., et al. 2009. Global change and eutrophication of coastal waters. ICES Journal of Marine Science: Journal Du Conseil, 66 (7), 1528–1537. doi:10.1093/icesjms/fsp047
- Sood, A. and Smakhtin, V., 2015. Global hydrological models: a review. Hydrological Sciences Journal, 60 (4), 549–565. doi:10.1080/02626667.2014.950580
- Tallis, H.T., et al., 2013. InVEST 2.5. 3 user’s guide. The natural capital project. Stanford. Available from: http://data.naturalcapitalproject.org/invest-releases/documentation/2–5–3/. [Accessed March 2018].
- Thomas, H.A., 1981. Improved methods for national water assessment. Report WR15249270. Washington, DC: US Water Resource Council.
- Verzano, K., et al. 2012. Modeling variable river flow velocity on continental scale: current situation and climate change impacts in Europe. Journal of Hydrology, 424–425, 238–251. Elsevier B.V. doi:10.1016/j.jhydrol.2012.01.005
- Voß, A., et al., 2012. Continental scale modelling of in‐stream river water quality: a report on methodology, test runs, and scenario application. Hydrological Processes, 26 (16), 2370–2384. doi:10.1002/hyp.9445
- Vogel, R.M., et al., 2007. Relations among storage, yield, and instream flow. Water Resources Research, 43 (5), 1–12. doi:10.1029/2006WR005226
- Vogel, R.M. and Sankarasubramanian, A., 2003. Validation of a watershed model without calibration. Water Resources Research, 39, 10. doi:10.1029/2002WR001940
- Ward, P.J., et al., 2015. Usefulness and limitations of global flood risk models. Nature Climate Change, 5 (8), 712–715. Nature Publishing Group. doi:10.1038/nclimate2742
- Wen, Z., Liang, X., and Yang, S., 2012. A new multiscale routing framework and its evaluation for land surface modeling applications. Water Resources Research, 48 (8). doi:10.1029/2011WR011337
- Wu, H., et al., 2011. Automated upscaling of river networks for macroscale hydrological modeling.”. Water Resources Research, 47, 3. doi:10.1029/2009WR008871
- Wu, H., et al. 2014. Real‐time global flood estimation using satellite‐based precipitation and a coupled land surface and routing model. Water Resources Research, 50 (3), 2693–2717. doi:10.1002/2013WR014710
- Wu, H., et al., 2012. A new global river network database for macroscale hydrologic modeling. Water Resources Research, 48, 9. doi:10.1029/2012WR012313
- Wu, Y. and Chen, J., 2013. Investigating the effects of point source and nonpoint source pollution on the water quality of the east river (Dongjiang) in South China. Ecological Indicators, 32, 294–304. Elsevier Ltd. doi:10.1016/j.ecolind.2013.04.002
- WWAP (World Water Assessment Programme of the United Nations), 2017. The United Nations world water development report 2017. Wastewater: the untapped resource. Paris: UNESCO.
- Xu, C.-Y., 1999. From GCMs to river flow: a review of downscaling methods and hydrologic modelling approaches. Progress in Physical Geography, 23 (2), 229–249. doi:10.1191/030913399667424608
- Yamazaki, D., et al., 2011. A physically based description of floodplain inundation dynamics in a global river routing model. Water Resources Research, 47 (4), 1–21. doi:10.1029/2010WR009726
- Yamazaki, D., et al., 2012. Analysis of the water level dynamics simulated by a global river model: a case study in the Amazon River. Water Resources Research, 48 (9), 1–15. doi:10.1029/2012WR011869
- Ye, A., et al., 2013. Improving kinematic wave routing scheme in community land model. Hydrology Research 44 (January 2016): 886, 44, 886. doi:10.2166/nh.2012.145
- Yearsley, J., 2012. A grid-based approach for simulating stream temperature. Water Resources Research, 48 (3), 1–15. doi:10.1029/2011WR011515