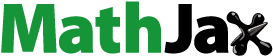
ABSTRACT
Recently, the land surface in the Haihe River basin has changed, influencing the flood processes in the basin. To quantify this impact, seven typical sub-catchments were selected from different hydrological regions of the Haihe River basin for study. The non-parametric Mann-Kendall test was used to analyse for trends, and the non-parametric Pettitt test was adopted to detect any change point in the flood time series. Then, a hydrological model was established to simulate the effects of each potential driving factor on flood peak and volume. It was shown that flood peak and volume time series had decreased significantly, and the change point was around the year 1980. Groundwater depletion was not the main contribution to flood peak (FP) and volume (FV) decrease. In the Shifokou, Mubi and Lengkou sub-catchments, small hydraulic structures are the main driving factors for FP and FV decreasing. In the Xitaiyu, Daomaguan and Fuping sub-catchments, both land-use change and hydraulic structures are the main driving factors. The decreasing percentage decreases with the increase of the flood magnitude. The results provide valuable information for flood simulation and control in the Haihe River basin.
Editor A. Castellarin
GUEST EDITOR Y. Chen
1 Introduction
Flooding is a frequent natural hazard, which has serious impacts on the economy, ecology, environment, lives and society. Flood warning and forecasting is an effective method to reduce such losses. The primary task is to identify the flood generation mechanism at the watershed scale, and this is still a challenge in flood hydrology, especially under changes in climate and land surface due to human activities.
With environmental change, hydrological processes are influenced and the hydrological components show increasing or decreasing trends over the long term. Flood characteristics, including peak discharge and volume, vary significantly based on many previous studies. The variation has often been identified by widely-used trend analysis methods, such as the non-parametric Mann-Kendall test and the linear regression method. Haddad and Rahman (Citation2011) employed the Mann-Kendall test for annual maximum flood peak (AMF) series in Tasmania, Australia, and a decreasing trend was identified. Fritsch (Citation2012) adopted the same method in AMF trend detection in 569 hydrological stations in the USA.
In addition to trend analysis, another aspect for flood variation is to identify a change point due to climate change or human impact. Numerous change-point detection methods have been presented in the past decades, and the non-parametric Pettitt test, the double-mass curve, and the R/S test are well-known methods in hydrology. Li and Feng (Citation2007) identified the year 1979 as a change point in annual runoff time series using the Pettitt test. This test was also used by other researchers in studying annual maximum peak discharge series (e.g. Villarini et al. Citation2011, Fritsch Citation2012).
However, the qualitative quantification of flood variation is inadequate for flood control purposes, as changes in flood peak and volume should be quantitatively evaluated. Statistical and hydrological modelling are two main methods to identify the contribution of environmental change to flood variations. Sahin and Hall (Citation1996) applied multi-linear regression to quantify the effects of land-use change on runoff. Sriwongsitanon and Taesombat (Citation2011) built a power function between runoff coefficients and flood peaks using flood data for the period 1988–2005 in 11 small catchments in Thailand, and estimated the impact of land-use change on runoff coefficients. In these statistical analysis, rainfall areal uniformity was assumed and non-uniformity cannot be considered. Hydrological models can take rainfall non-uniformity and the runoff generation mechanism into consideration, and have been widely applied to this issue. Niehoff et al. (Citation2002) simulated flood responses to land-use change under different rainfall patterns using the WaSiM-ETH model, a widely-known water-balance simulation model. They pointed out that land-use change significantly affected flooding that was generated by long-duration and high-intensity rainfall. Ott and Uhlenbrook (Citation2004) and Bronstert et al. (Citation2007) reached similar conclusions. Shu et al. (Citation2009) applied the WetSpa hydrological rainfall–runoff model to simulate the impact of afforestation on floods in the Liuxihe River basin, China, and showed land-use change had greater impact on smaller floods.
Influenced by water resources exploitation and land-use change, land surface in the Haihe River basin has changed significantly, leading to a decrease in flood peak and flood volume. However, this was only realized in some individual small catchments in the Haihe River basin, not on the scale of the whole river basin. Zhang and Feng (Citation2012) quantified the changes in flood depth and flood peaks in the Daqinghe catchment, a sub-catchment of the Haihe River basin, using a multiple regression method. Li and Feng (Citation2011), Han and Feng (Citation2010) and Li et al. (Citation2012) applied a hydrological model to quantify the effects of land-use change on floods in the Zijingguan catchment, a small sub-catchment of the Haihe River basin. However, they only considered the effect of land-use change on flood characteristics, and ignored hydraulic structures and groundwater depletion. The extent to which the flood volume and flood peak were affected by land surface change was not quantified, and this subsequently affected the design of flood modification strategies. In this context, it is necessary to analyse changes in flood characteristics and their driving factors in the whole Haihe River basin.
The aims of this paper are to: (1) analyse the variations in flood series in the Haihe River basin; (2) quantify the impact of land surface change on flood characteristics; and (3) identify the main driving factors of flood variations. This study can provide valuable information for flood control and regulation.
2 Study area
The Haihe River basin is one of the seven main river basins in China, and is located between longitude 112°–120°E and latitude 35°–43°N. It has a drainage area of 318 000 km2, of which mountains account for 59% and plains for 41%. The main sub-watersheds are the Luanhe, Beisanhe, Yongdinghe, Daqinghe, Ziyahe, Zhangweinanhe, Tuhaihe and Majiahe, some of which are shown in .
Figure 1. Study area: the seven hydrological areas and the selected hydrological stations. I: Shifokou; II: Mubi; III: Xitaiyu; IV: Daomaguan; V: Fuping; VI: Dage; and VII: Lengkou.
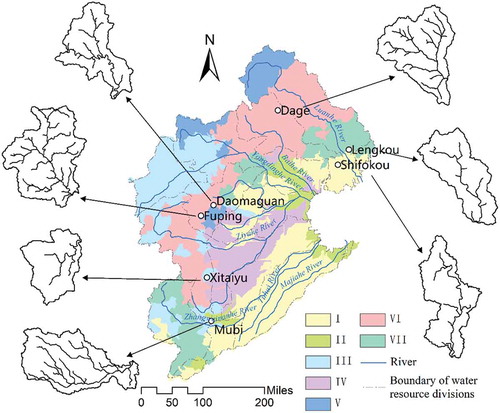
The river basin receives an average annual precipitation of 538 mm, about 80% of which falls during June–September. Floods are often caused by rainstorms during this period, and have the characteristics of high peak discharge and large flood volume. The average temperature is between 1.5–14°C, and the relative humidity is 50%–70%. The average potential evapotranspiration reaches 1100 mm/year.
The land use in the Haihe River basin has changed since the 1980s, when about 80 large reservoirs and 1400 small reservoirs were built that controlled around 85% of the mountainous area. In addition, afforestation was carried out which increased land cover by 40%. In this case, runoff yield decreased significantly, and the occurrence of floods also decreased. Taking Fuyanghe sub-catchment as an example, the amount of rainfall that occurred in August 1956 and August 1996 was almost the same, but the measured flood magnitude for the 1996 rainfall event was half that of the 1956 rainfall event. Therefore, it is necessary to quantify to what extent land surface change affected the flood characteristics of different return periods.
3 Data and methods
3.1 Data
The Haihe River basin has such a large drainage area, including several outlets to the Bohai Sea, that it is impossible to simulate the flood processes in the whole area. According to Feng et al. (Citation2013), the Haihe River basin may be delineated into seven hydrological areas; therefore, we chose a sub-catchment from each area to analyse the changes in flood characteristics. The hydrological areas and selected sub-catchments are shown in .
Hourly rainfall and flood data from the period 1956–2005 were provided by the Haihe River Water Conservancy Commission. Annual maximum flood peak discharge and volume were selected as the flood characteristics to analyse the changes. Remotely sensed land-use data of 1980 and 2000 were provided by the Chinese Academy of Sciences (). The land use was classified into six types: forest, grassland, agricultural land, urban area, water area and unused land.
3.2 Methods
3.2.1 Trend and change point detection
Analysis of changes in flood characteristics mainly includes trend test and change point detection of the annual maximum flood peak and volume for the seven selected typical sub-catchments. A widely-used non-parametric trend test method, the Mann-Kendall test (Mann Citation1945, Kendall Citation1975), was used to detect trends. The test statistic is U. The non-parametric Pettitt test (Pettitt Citation1979) was employed to detect the change point. All of these non-parametric tests were conducted at a significance level of α = 0.05. Readers are referred to the references in this section for more details.
3.2.2 Description of the hydrological model
As pointed out in previous studies (Cheng et al., Citation2015, Xu Citation2007), in the mountainous area of the Haihe River basin, the runoff generation mechanism consists of coupled infiltration-excess and saturation-excess. The distributed hydrological model considering land use and land cover (the ZWHM-LUCC model), based on this runoff generation mechanism, as presented by Xie et al. (Citation2014), is used in this study. The model can be established with the following procedure:
Step 1. The entire watershed is delineated into several sub-watersheds.
Step 2. The land-use types in the watershed are identified first, e.g. forest, agricultural land, grassland, urban area, unused land and water bodies; then, the area of each land-use type in the sub-watersheds is calculated in ArcGIS.
Step 3. In the forest, grassland, agricultural land and unused land, coupled infiltration-excess and saturation-excess runoff generation is employed to calculate the surface runoff, sub-surface runoff and baseflow in each sub-watershed. The infiltration-excess runoff generation is employed for water bodies and urban area as these are considered as impermeable.
Step 4. The unit hydrograph is adopted to calculate hillslope surface flow to the channel. Before the calculation of surface flow routing, it should satisfy the storage of the small hydraulic structures and check dams. The linear reservoir method is applied for interflow and groundwater flow routing, and the Muskingum method is used for the flow in channels. Finally, the flood hydrograph at the outlet of the watershed is obtained. More details of this process can be found in Xie et al. (Citation2014) and Li et al. (Citation2014).
Step 5. Relative error (RE) and the Nash-Sutcliffe efficiency criterion (NSE) were selected as the indices for model calibration and validation. If the RE between the simulated and observed flood peak or flood volume approaches zero, and the NSE approaches 1, the model performance is good. The graphical description of the ZWHM-LUCC model, as well as its parameters, are shown in .
3.2.3 Identification of potential driving factors of flood variation
The potential driving factors of flood variation include rainstorm, land-use change, hydraulic structures, groundwater variation, geography and soil. The geography and soil are considered to be unchanged in the short period of time of the study. Therefore, the other factors need to be analysed.
Annual maximum 1-h and 24-h rainfall time series were selected to analyse the trend and change point using the above-mentioned Mann-Kendall test and non-parametric Pettitt test. If the time series show a significant trend or change point, then changes in rainfall may be considered as a factor leading to flood changes.
Using the remotely sensed land-use data of 1980 and 2000, the area of each land-use type was obtained by ArcGIS software, and changes in land use were analysed.
Groundwater exploitation during the period 1980–2000 was obtained from Ren (Citation2007) and the Haihe River Water Conservancy Commission (Citation2013). The over-exploitation of groundwater has led to groundwater table depletion, and increased the deep soil moisture storage capacity.
Many small hydraulic structures have been built in these sub-catchments for soil and water conservation and irrigation, and their storage capacity was also investigated. Taking the Fuping sub-catchment as an example, 11 small hydraulic structures were identified, among them (Haiyan, Mapeng, Nanyu and Tagou reserviors). The total storage capacity of these hydraulic structures is 5.915 × 106 m3, amounting to 2.7 mm of runoff in the entire sub-catchment.
3.2.4 Quantification of the effects of driving factors on flood characteristics
The hydrological model was used to quantitatively simulate the effects of potential driving factors on flood characteristics.
The 1980 and 2000 land-use data were input into the hydrological model, and for the flood events before the change point, the other driving factors were input using the data from before the change point, and for the flood events after the change point, the other driving factors were input using the data from after the change point. According to the modelling results of each flood event for the period 1956–2005 under different land-use conditions, the relative changes in flood peak and flood volume due to land-use change can be quantified. Taking flood peak as an example, the relative change is calculated by:
where RC is relative change of flood peak (%); and Qsim,1 and Qsim,2 are the simulated flood peak before 1980 and after 1980 land surface conditions, respectively.
Similarly, the hydrological model was run for each flood event in the period 1956–2005 without and with hydraulic structures, to represent the conditions before and after 1980, respectively. Thus, the effect of hydraulic structures on flood peak and flood volume could be assessed. The deep soil moisture before and after the change point was input into the hydrological model to simulate each flood event for the period 1956–2005. By comparing the simulated flood processes, the effect of groundwater table depletion on flood peak and flood volume could be obtained.
4 Results
4.1 Trend and change point of flood characteristics
The trends in the flood volume and flood peak series were detected by the non-parametric Mann-Kendall test at the significance level α = 0.05, and the critical value Uα/2 = ±1.96, where “+” means an increasing trend, and “–” means a decreasing trend. The values of statistic U are listed in . It can be seen that both flood volume and peak discharges in Shifokou, Fuping, Dage and Lengkou sub-catchments show a significant decreasing trend. The flood volume in Mubi and the peak discharge in the Daomaguan sub-catchment show significant decreasing trends too. The flood characteristics in the Xitaiyu sub-catchment show a decreasing trend, but are not significant at the 0.05 significance level.
Table 1. Trend and change point test results of flood characteristics for the seven study sub-catchments. Bold indicates significant trend and change point. Numbers indicate sub-catchments in . FV: flood volume.
The Pettitt test was used to detect the change point of the flood time series at a 5% significance level. The change points were all around the year 1980 (), and only the flood volume time series in the Daomaguan was not significant.
4.2 Changes in potential driving factors
Rainfall is the source of flood events and is the primary factor that should be considered for flood variation. Annual maximum 1-h and 24-h rainfall amounts, which may contribute to the peak discharge and total flood volume, respectively, were selected to analyse trends and change points. No significant trend or change point were detected in the sub-catchments.
The land-use data of 1980 and 2000 for the seven typical sub-catchments were obtained from the remotely sensed land use of the Haihe River basin, and are listed in . The main land-use types in the sub-catchments are agricultural land, forest land and grassland, except for the Mubi sub-catchment where agricultural land is the main land-use type. The land-use change patterns are very different in each sub-catchment. Whether the area of each land-use type increased or decreased will influence the runoff yield.
Table 2. Area (km2) of each land-use type in 1980 and 2000 for each sub-catchment.
To investigate of groundwater exploitation, the corresponding increased deep soil moisture storage capacity was estimated, as listed in . The increased values range from 13.7 to 27.3 mm, which will result in decreased runoff yield through greater storage of infiltrated rainfall.
Table 3. Increased storage capacity of small hydraulic structures (converted to runoff depth) and deep soil moisture.
Some small hydraulic structures were built for irrigation after 1980, and check dams were also built for soil and water conservation. The total storage capacity of these water storage projects was estimated and it was found that they can store surface runoff volume amounting to 1.2–6.4 mm runoff depth in the sub-catchments.
4.3 Hydrological model calibration and validation
Several flood events were selected from the seven typical sub-catchments to calibrate the hydrological model, and other flood events were selected for model validation. A set of numerical experiments was conducted to identify the sensitivity of the flood to changes in different parameters of the model before calibration. It was concluded that the parameters WUM and FC are most important, as these control saturation-excess runoff volume and infiltration-excess runoff volume, respectively. The values of less sensitive parameters were determined by Li et al. (Citation2014). The sensitive parameters were adjusted to make the simulated hydrograph fit the observed hydrograph. To save space, only the calibration main parameters in the Shifokou sub-catchment are given in and the simulation results are presented in . Two simulated flood events in Shifokou sub-catchment are shown in .
Table 4. Calibrated values of the main parameters for the Shifokou sub-catchment.
Table 5. Comparison of simulated and observed flood events in Shifokou sub-catchment. FVO and FVS: observed and simulated flood volume, respectively. FPO and FPS: observed and simulated mean flood peak, respectively. RE: relative error; NSE: Nash-Sutcliffe efficiency criterion.
Figure 4. Simulated (Δ) and observed (•) flood processes in Shifokou sub-catchment for flood events: (a) 670820 and (b) 700903.
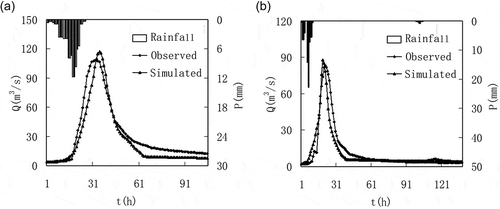
Comparing the measured with the simulated hydrographs, the range of RE was –18.9% to 14.5% for FV and –18.1% to 8.4% for the selected 11 calibrated flood events. For the validation period, the RE of flood volume and flood peak for all the flood events are within 20% except for the 980713 flood event. The NSE coefficient explains the performance of the model in terms of simulating flood processes. Only one flood event (690902) had a relatively low NSE of 0.40, while the other flood events had NSE values larger than 0.60, especially events 670820 and 770720, where the NSE value reached 0.90. shows that the simulated hydrograph fits the observed hydrograph very well in the Shifokou sub-catchment, which illustrates that the model performance is acceptable to simulate the flood process.
In other sub-catchments, the RE of flood volume and flood peak are acceptable, most of them being lower than 20%. However, in the Fuping sub-catchment, there are two simulated flood events with NSE < 0. The main reason is that there is a 2-h lag in the simulated flood peak. Overall, the established model may be used to simulate flood events in the Haihe River basin, and to analyse the effects of driving factors on changes in flood characteristics.
4.4 Effects of potential driving factors on flood characteristics
No significant changes in annual maximum rainfall time series were found and, therefore, we did not analyse the effect of changes in rainfall on flood variations. For the same rainfall event, the flood event was simulated under 1980 and 2000 conditions, so the simulated flood events do not include the effect of rainfall changes.
Several observed flood events were selected, and they were classified into three categories according to return periods of: >10 years, 5–10-years and <5 years. Each flood event was simulated by the hydrological model under the corresponding environmental conditions, and then only one driving factor (i.e. groundwater depletion, land-use change, or small hydraulic structures) was changed to simulate the effects of this driving factor on flooding. The average ratios of variations due to each driving factor were calculated and are presented in .
Table 6. Effects of driving factors – groundwater depletion, land-use change and small hydraulic structures – on flood characteristics. FP: flood peak; FV: flood volume.
As may be seen from , Groundwater depletion has a small negative influence on flood peak (FP) and flood volume (FV), with the change percentages in the range −0.8 to −2.9 and −0.3 to −2.5, respectively.
Changes in land use have a negative impact too, and the decreasing percentages vary largely in different sub-catchments and for floods of different magnitudes (). In the Shifokou, Mubi and Lengkou sub-catchments, the decreasing percentages range from −1.3 to −9.2 for FP and from −0.8 to −4.4 for FV, which shows a relatively low impact of land-use change in these sub-catchments. In the other four sub-catchments, the decreasing percentages are in the ranges −2.6 to −30.0 for FP and −4.4 to −22.5 for FV, which shows a relatively high impact of land-use change in these sub-catchments. In addition, the decreasing percentage decreases with the increase in flood magnitude (return period).
Small hydraulic structures store part of the surface runoff and have a large negative impact on both FP and FV. The Dage sub-catchment has the smallest change ratio, ranging from −6.7 to −7.1 for FP and from −4.9 to −6.5 for FV. In this sub-catchment, land-use change is the main driving factor for the decrease in flood magnitude. In the Shifokou, Mubi and Lengkou sub-catchments, small hydraulic structures are the main driving factors for decreases in FP and FV. In the Xitaiyu, Daomaguan and Fuping sub-catchments, both land-use change and hydraulic structures are driving factors.
Li et al. (Citation2014) analysed the effects of land-use change in the Fuping sub-catchment, and found relative changes in the ranges −1.4 to −3.8% and −0.3 to −6.4% for flood peak and flood volume, respectively. However, in this study the effects of land-use change in the Fuping sub-catchment are considerably higher, with ranges of −2.6 to −27.0% and −8.9 to −15.9%, respectively. In addition, Li et al. (Citation2014) did not take the hydraulic structures into account, whereas in this study, the effect of small hydraulic structures is −5.5 to −26.6% and −5.4 to −13.3% for flood peak and volume, respectively, for the Fuping sub-catchment. This suggests that the flood events were simulated according to the actual runoff generation in this research, and the results are reasonable.
5 Discussion
Flood events can be expressed by characteristics such as flood peak, volume and duration, and the shape of flood hydrograph (Nied et al. Citation2017). Flood peak and volume were selected as flood characteristics, and were shown to decrease significantly in the Haihe River basin, with the changes of all the factors that influence runoff generation contributing to the decrease. In other previous studies, floods have been reported as increasing due to environmental change (e.g. Kundzewicz et al. Citation2012, Stevens et al. Citation2014).
A coupled infiltration-excess and saturation-excess runoff generation mechanism was applied to simulate the flood hydrograph, as presented by Xie et al. (Citation2014), and used by other researchers (Li et al. Citation2014). The difference between our study and previous studies is the consideration of the storage capacity of small hydraulic structures in the selected sub-watersheds of the Haihe River basin, which cannot be neglected. In previous studies, the storage capacity of hydraulic structures was assumed as part of the soil moisture storage capacity, which would influence the sub-surface flow generation. Therefore, the improved model is shown to better reflect the flood processes in the Haihe River basin.
In this study, rainfall was not considered as a driving factor of flood decrease as there were no significant changes in rainfall in the study period. However, insignificant trends of rainfall may lead to significant changes in floods because of the nonlinear relationship between rainfall and runoff. To date, there is still not an effective method to take this issue into account. The accuracy of land-use data will affect the simulation of flood events. In addition, the increased deep soil moisture storage capacity, as well as that of hydraulic structures, were estimated but not measured directly, which also bring model uncertainty (Kapangaziwiri et al. Citation2012, Kasiviswanathan et al. Citation2018). Therefore, we did not compare the simulated with the observed flood events to quantify the effect of driving factors on flood decrease, but made comparisons between the simulated results under different conditions.
The significant decrease of flood peak and volume illustrates the nonstationarity of the flood series, and may affect the existing design flood in the Haihe River basin. Some previous studies have documented the necessity of refining the design floods (Gilroy and McCuen Citation2012, Gu et al. Citation2017), and the corresponding flood control measures might be modified.
6 Conclusions
Seven typical sub-catchments of the Haihe River basin were selected to analyse changes in flood characteristics and the driving factors were identified using hydrological modelling techniques. Some conclusions can be drawn, and the results are summarized as follows.
The maximum flood peak and flood volume time series were constructed using observed hourly flood data. The non-parametric Mann-Kendall test was adopted to analyse the trend of the time series, which showed significant decreasing trend for most of the sub-catchments. The Pettitt test was employed to detect the change point of the time series, which was around the year 1980.
Combined with the land surface conditions in the Haihe River basin, a hydrological model was established considering the groundwater depletion, land-use change and the storage of small hydraulic structures. The model was calibrated and validated in the seven sub-catchments, and performed very well in flood peak, volume and process simulation.
The changes in potential driving factors were analysed, including depletion of groundwater, land-use change from 1980 to 2000, and the construction of small hydraulic structures. The impacts of these potential driving factors on flood peak and volume were simulated. It was shown that land-use change and/or small hydraulic structures are the main driving factors, and groundwater depletion has a small impact on the decrease in flooding.
Acknowledgements
The authors are grateful to the Haihe River Water Conservancy Commission for providing the hydrological data.
Disclosure statement
No potential conflict of interest was reported by the authors.
Additional information
Funding
References
- Bronstert, A., et al., 2007. Multi-scale modelling of land use change and river training effects on floods in the Rhine basin. River Research and Applications, 23, 1102–1125. doi:10.1002/rra.1036
- Cheng, S.H., Hu, C.Q., and Ma, C.H., 2015. Application of spatially distributed unit hydrograph to rainfall-flood model of Hebei province. Journal of Yangtze River Scientific Research Institute, 32, 11–14.
- Feng, P., Wei, Z.Z., and Li, J.Z., 2013. Hydrologic type regions delineation of Haihe River basin based on remote sensing data of underlying surface cover. Journal of Natural Resources, 28, 1350–1360.
- Fritsch, C.E., 2012. Evaluation of Flood Risk in Response to Climate Variability. Michigan: Michigan Technological University.
- Gilroy, K.L. and McCuen, R.H., 2012. A nonstationary flood frequency analysis method to adjust for future climate change and urbanization. Journal of Hydrology, 414–415, 40–48. doi:10.1016/j.jhydrol.2011.10.009
- Gu, X., et al., 2017. Nonstationarity-based evaluation of flood risk in the Pearl River basin: changing patterns causes and implications. Hydrological Sciences Journal, 62, 246–258. doi:10.1080/02626667.2016.1183774
- Haddad, K. and Rahman, A., 2011. Selection of the best fit flood frequency distribution and parameter estimation procedure: a case study for Tasmania in Australia. Stochastic Environmental Research and Risk Assessment, 25, 415–428. doi:10.1007/s00477-010-0412-1
- Haihe River Water Conservancy Commission. 2013. Land surface changes in Haihe River basin. Report. Haihe River Water Conservancy Commission.
- Han, R.G. and Feng, P., 2010. Effects of sublayer and land cover change on flood in Daqinghe River basin. Journal of Arid Land Resources and Environment, 24, 27–30.
- Kapangaziwiri, E., Hughes, D.A., and Wagener, T., 2012. Incorporating uncertainty in hydrological predictions for gauged and ungauged basins in southern Africa. Hydrological Sciences Journal, 57, 1000–1009. doi:10.1080/02626667.2012.690881
- Kasiviswanathan, K.S., Sudheer, K.P., and He, J., 2018. Probabilistic and ensemble simulation approaches for input uncertainty quantification of artificial neural network hydrological models. Hydrological Sciences Journal, 63, 101–113. doi:10.1080/02626667.2017.1393686
- Kendall, M.G., 1975. Rank correlation measures. London: Charles Griffin.
- Kundzewicz, Z.W., Pinskwar, I., and Brakenridge, G.R., 2012. Large floods in Europe, 1985–2009. Hydrological Sciences Journal, 58, 1–7. doi:10.1080/02626667.2012.745082
- Li, J.Z. and Feng, P., 2007. Runoff variations in the Luanhe River basin during 1956–2012. Journal of Geophysical Sciences, 17, 339–350.
- Li, J.Z. and Feng, P., 2011. The effects of underlying surface change on floods in Zijingguan watershed. Geographical Research, 30, 921–930.
- Li, J.Z., Feng, P., and Chen, F.L., 2014. Effects of land use change on flood characteristics in mountainous area of Daqinghe watershed, China. Natural Hazards, 70, 593–607. doi:10.1007/s11069-013-0830-8
- Li, Z.J., et al., 2012. Study on impact of basin cushion changes on river floods by hydrological model. Journal of Hydroelectric Engineering, 31, 5–10.
- Mann, H.B., 1945. Nonparametric test against trend. Econometrica, 13, 245–259. doi:10.2307/1907187
- Nied, M., et al., 2017. What are the hydro-meteorological controls on flood characteristics? Journal of Hydrology, 545, 310–326. doi:10.1016/j.jhydrol.2016.12.003
- Niehoff, D., Fritsch, U., and Bronstert, A., 2002. Land use impacts on storm-runoff generation: scenarios of land-use change and simulation of hydrological response in a meso-scale catchment in SW-Germany. Journal of Hydrology, 267, 80–93. doi:10.1016/S0022-1694(02)00142-7
- Ott, B. and Uhlenbrook, S., 2004. Quantifying the impact of land use changes at the event and seasonal time scale using a process oriented catchment model. Hydrology and Earth System Sciences, 8, 62–78. doi:10.5194/hess-8-62-2004
- Pettitt, A.N., 1979. A non-parametric approach to the change-point problem. Journal of the Royal Statistical Society, 28, 126–135.
- Ren, X.S., 2007. Assessment of water resources in Haihe River basin. Beijing: China Water&Power Press.
- Sahin, V. and Hall, M.J., 1996. The effects of afforestation and deforestation on water yields. Journal of Hydrology, 410, 226–238.
- Shu, X.J., Chen, Y.B., and Xu, H.J., 2009. Application of Wetspa model in simulation of Liuxihe reservoir inflow flood. Journal of Yangtze River Scientific Research Institute, 26, 17–20.
- Sriwongsitanon, N. and Taesombat, W., 2011. Effects of land cover on runoff coefficient. Journal of Hydrology, 410, 226–238. doi:10.1016/j.jhydrol.2011.09.021
- Stevens, A.J., Clarke, D., and Nicholls, R.J., 2014. Trends in reported flooding in the UK: 1998–2013. Hydrological Sciences Journal, 61, 50–63. doi:10.1080/02626667.2014.950581
- Villarini, G., et al., 2011. Examining flood frequency distributions in the Midwest U.S. Journal of the American Water Resources Association, 47, 447–463. doi:10.1111/j.1752-1688.2011.00540.x
- Xie, P., et al., 2014. Runoff response with distributed hydrological model based on river structure and LUCC. Mountain Research, 32, 154–162.
- Xu, X., 2007. Research on the Trends of Runoff Generation and Convergence Characteristics of Panjiakou Reservoir Basin. Tianjin: Tianjin University.
- Zhang, D.D. and Feng, P., 2012. Multivariate statistical analysis of influence of underlying surface change on flood. Water Resources and Power, 30, 37–40.