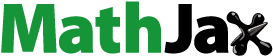
ABSTRACT
Joint variations of precipitation (P) and temperature (T) under the influence of climate variability are examined in this study. Individual and joint influences of Atlantic multidecadal oscillation (AMO) and El Niño Southern Oscillation (ENSO) on P and T variability in a tropical region (i.e. Florida) are investigated. A rank-based association measure, nonparametric hypothesis tests, and Latin hypercube bootstrap sampling are used for assessments of hydroclimatic data at 78 observation stations. Spatially non-uniform influences of these two oscillations on the P-T associations are evident. While P-T associations were stronger during La Niña compared to El Niño, relatively lower precipitation is observed during La Niña. Temperature range-specific variations in the frequency and magnitude of precipitation are noted in two phases of AMO and ENSO. Climate variability has a stronger influence than local meteorological conditions in generating higher precipitation. The changes in P-T associations due to climate variability are mostly elevation invariant.
Editor A. Fiori Associate editor S. Huang
1 Introduction
Fluctuations in the land surface temperature (T) directly influence the precipitation (P) variability across any terrestrial region. An increase in surface air temperature would increase the moisture-holding capacity of the air, which, in turn, produces intense precipitation due to enhanced moisture supply (Trenberth and Shea Citation2005). The observed changes in regional precipitation and temperature are often physically interrelated, and significant large-scale associations between them have been reported in multiple studies (Madden and Williams Citation1978, Trenberth and Shea Citation2005). In many regions, the precipitation patterns are also influenced by fluctuations in the sea surface temperature (SST), specifically over the tropics and sub-tropics (Trenberth Citation2011). SSTs have a stronger influence on the precipitable water (Trenberth et al. Citation2005), and changes in SSTs across the large pool of warm water in the tropical Pacific exerts considerable influence on the P-T variability. Such changes are often responsible for cyclones (or hurricanes) in the tropical regions, and their landfalls cause extreme precipitation events and catastrophic floods. Knowledge about specific influences of climate variability on occurrences of cyclones or extratropical storms and ensuing extreme precipitation events would be helpful for disaster mitigation preparedness (Murnane Citation2004). Also, studying spatial and temporal variations in P-T interactions is critical for the agricultural sector in the determination of crop yield, irrigation water supply, and ecological changes.
P-T relationships exhibit considerable latitudinal variations and are influenced by the type of regional climate. Utsumi et al. (Citation2011) reported a monotonic increase (decrease) in extreme daily precipitation with the temperature at high latitudes (over the tropics). The temperature fields demonstrate a rather strong spatio-temporal correlation, and their distribution functions show a symmetric nature about the mean (Berg and Haerter Citation2013). Jones et al. (Citation2010) reported a substantial decrease in maximum relative humidity across Australia where land surface temperatures were greater than 26°C. Mishra et al. (Citation2012) found a stronger P-T association for the regions (such as the southeastern US) where convection activity is predominant. Berg et al. (Citation2013) observed an overall decrease in relative humidity with temperature for convective precipitation compared to stratiform precipitation, indicating the sensitivity of convection to temperature changes. Sharma and Mujumdar (Citation2019) showed that peak values of daily rainfall extremes were observed in the temperature range 25–30°C, whereas a minor decrease in the same was reported at higher temperatures. Thus, the extreme precipitation variability at higher temperatures is predominantly driven by moisture availability (Trenberth and Shea Citation2005, Jones et al. Citation2010). Moreover, a negative relationship between extreme precipitation and the surface temperature has been observed across many regions, specifically for warmer climates (Zhao and Khalil Citation1993, Wasko et al. Citation2016, Ali and Mishra Citation2017). Ali and Mishra (Citation2017) stated that such a negative relationship indicates the response of surface air temperature to extreme precipitation rather than implying a cause–effect relationship between them. These correlations could also be explained by several physical mechanisms, such as shifts in precipitation, changes in cloud cover, and ensuing variations in the regional heat balance (Zhao and Khalil Citation1993). In general, the asymmetric nature of P-T relationships was observed globally (Wang et al. Citation2017). Also, climate variability could have a considerable influence on P-T relationships, which warrants region-specific investigations.
Linkages between climate anomalies that are separated by large distances are defined as teleconnections (Chiew et al. Citation1998). Several studies (Enfield et al. Citation2001, Kashid et al. Citation2010, Teegavarapu Citation2012, Teegavarapu et al. Citation2013, Goly and Teegavarapu Citation2014, Sun et al. Citation2015, Tian et al. Citation2016, Murgulet et al. Citation2017, Wang et al. Citation2017, Maleski and Martinez Citation2018, Sharma et al. Citation2020) have documented the influences of climate variability on hydroclimatic variables across the globe. However, these studies were primarily focused on analyzing the influence of climate variability on changes in hydroclimatic variables, while their specific influences on the regional variability of P-T associations were not explored. Also, the large-scale coupled oceanic–atmospheric circulations reportedly exert varied influences on hydroclimatic variability depending on the geographical location. Moreover, point-based analyses can better help unravel the complexity in P-T relationships compared with grid-based analyses, since the latter masks the spatial variability of P-T associations. However, comprehensive assessments of the influence of climate variability on P-T associations using point-based observations are not available from past studies. In this study, the individual and joint variations of point-based temperature and precipitation observations in space and time over a tropical region that is influenced by two major coupled oceanic–atmospheric oscillations, viz. the Atlantic Multidecadal Oscillation (AMO) and El Niño Southern Oscillation (ENSO), are of interest.
Enfield et al. (Citation2001) emphasized that since climate variability largely influences the regional precipitation variability, these could have implications for water resources and disaster management in Florida. Murgulet et al. (Citation2017) analyzed the individual and joint influences of various phases of ENSO, AMO, and the Pacific Decadal Oscillation (PDO) on precipitation and streamflow variability across South Texas. They reported stronger teleconnection influences of these oscillations on streamflow changes. A distinct spatial heterogeneity in the influences of AMO phases on precipitation extremes across Florida was reported (Teegavarapu et al. Citation2013). An increase in the occurrence of extreme events in the dry (wet) season during the AMO cool (warm) phase across Florida was reported (Teegavarapu et al. Citation2013, Goly and Teegavarapu Citation2014). The warm (cool) ENSO phase leads to an increase (decrease) in total and extreme precipitation across Florida (Goly and Teegavarapu Citation2014). These naturally occurring modes of climate variability impact the climate of Florida through modifications of the sub-tropical and polar jet streams (Kirtman et al. Citation2017).
Previous studies (Irizarry‐Ortiz et al. Citation2013, Teegavarapu et al. Citation2013, Goly and Teegavarapu Citation2014, Abiy et al. Citation2019, Misra and Bhardwaj Citation2020) have evaluated the influences of climate variability and change on precipitation extremes, specifically for the Florida region. However, these studies did not examine the influence of large-scale climate variability on joint P-T associations in the region. This study focuses on (i) evaluation of spatial and temporal variability of P-T associations in the region, (ii) assessment of the individual and joint influences of AMO and ENSO on P-T associations at multiple temporal scales (viz. daily, monthly, and multi-month), and (iii) examination of the changes in the P-T associations within various temperature intervals during different phases of oscillations using a variant of the Latin hypercube sampling approach.
This article is organized as follows. First, the methodology adopted for evaluating the P-T associations under the influence of climate variability is explained, and the nonparametric and parametric statistical methods employed in this study are discussed, in the methods of analysis section. The characteristics of the study region and various datasets adopted are elaborated next in the case study domain and data description section. Finally, the results from the analyses related to changes in P-T associations and their linkages to climate variability are reported along with a discussion and conclusions.
2 Methods of analysis
The methodology for the joint analysis of P and T data adopted in this study is shown in . Initially, the daily precipitation and temperature (maximum, minimum, and average) data at each station are analyzed and aggregated to a monthly time scale. The daily non-zero precipitation values and corresponding temperatures are also extracted. The datasets corresponding to various temporal windows, coinciding with individual and coupled AMO (warm and cool) and ENSO (El Niño and La Niña) phases, are then segregated. In this study, the temporal window refers to the length of time series of the observed hydroclimatic variable(s) corresponding to any given phase of ENSO and/or AMO oscillations. Procedures for the identification of temporal windows for different phases of oscillations are discussed in the case study domain and data description section. The joint variability of P and T is assessed using Spearman’s rank correlation (SRC) at each station for different temporal windows. Nonparametric statistical tests are then used to examine and draw inferences about statistically significant differences in the P-T associations in different temporal windows. Thereafter, the extent of spatial variability in the P-T relationship is assessed. To understand the variability of daily P-T correlation (or association) within various temperature ranges or intervals, a Latin hypercube bootstrap sampling (LHBS) procedure is adopted (shown in ). The LHBS procedure is elaborated in Section 2.2.
Figure 1. Methodology for evaluation of climate variability influences on individual and joint precipitation-temperature associations
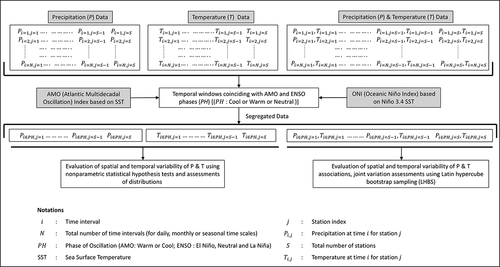
Figure 2. Latin hypercube bootstrap sampling (LHBS) procedure for evaluation of precipitation-temperature associations
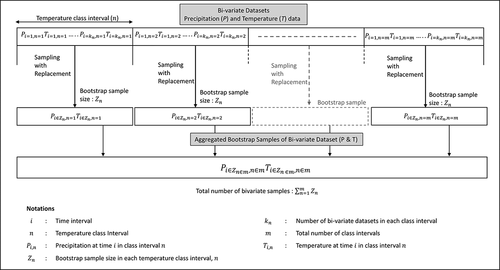
2.1 Nonparametric tests and smoothing methods
The nonparametric tests for evaluating the P-T associations and changes in such associations due to climate variability and smoothing methods are discussed in the following sections.
2.1.1 Rank-based association measure
The strength of the monotonic association between precipitation and temperature is quantified using a rank-order correlation measure, Spearman’s rank correlation, ρ (Rousseau et al. Citation2018), at each station. The SRC is estimated as:
where di represents the difference in ranking for the observation i and n is the number of bivariate observations. A statistical test with the null hypothesis (H0) suggesting no association between the two variables (i.e. ρ = 0), and an alternate hypothesis (H1) indicating the two variables are correlated (i.e. ρ ≠ 0), is conducted. The test is carried out at a 5% significance level (α).
2.1.2 Nonparametric test for change evaluation
A nonparametric test, the Mann-Whitney U test, is used to evaluate changes in two independent samples (i.e. precipitation and temperature, or joint variation metrics derived based on the two datasets) in two different temporal windows. In this test, two samples ( = 1 and
= 2) with
and
elements, respectively, are ranked from lowest to highest; and tied rank values are also reported, where appropriate. The test statistic (Z*) of the Mann-Whitney U test is estimated as (Teegavarapu et al. Citation2013, Corder and Foreman Citation2014):
The standard deviation (), the rank (
) from the sample
, and the mean value (
) are used to estimate the z score (
) or statistic for a normal approximation of the data. The null hypothesis (H0: the two population medians are equal) is rejected if the z score is greater than the critical value at a 5% significance level (α).
2.1.3 Density estimation
Kernel density estimates (KDEs) (Wilks Citation2011, Teegavarapu et al. Citation2013) are used to evaluate and compare probability densities of P-T associations characterized by SRCs in different phases of two oscillations (viz. AMO and ENSO). A Gaussian kernel is used to develop the smoothing function for KDE estimation. Kernel estimators center a kernel function () at each data point as given by EquationEquation (6)
(6)
(6) . The notations followed for explaining the KDE and corresponding equations are borrowed from Teegavarapu et al. (Citation2013). The contribution of data point
to the estimate at some point
depends on how far apart these two points are. The extent of this contribution is dependent upon the shape of the kernel function adopted and the bandwidth (
) assigned. The bandwidth parameter (h) controls the smoothness of the probability density curve. The estimated density at any point
is:
The variable is the kernel density estimate and n is the number of data points. The Gaussian kernel function (EquationEquation (7)
(7)
(7) ) is used for developing density estimates.
2.2 LHBS approach
A variant of the Latin hypercube sampling (LHS) approach (McKay et al. Citation1979), Latin hypercube bootstrap sampling (LHBS), is developed and implemented to reduce the inferential bias from unequal sample sizes. Unequal sample sizes are possible due to different temporal window lengths corresponding to different phases of the oscillations. As explained in , LHBS is employed to obtain samples of a specific size from each class interval (or bin) of one of the variables (temperature in this study). In the current study, the temperature dataset is divided or stratified into several class intervals (or ranges) , with a total of m intervals. In each interval, bootstrap samples of size
are generated. All these samples are aggregated to obtain
bivariate (P and T) samples. Based on this aggregated sample, a measure (i.e. SRC) quantifying the strength of the association can be obtained. This process is repeated NB number of times to obtain a large sample of association measures for evaluation using KDEs or nonparametric tests.
2.3 Equal slopes test
A parametric equal slopes test (Sachs Citation1982, Armitage et al. Citation2008) is employed in this study to assess the changes in slopes of fitted linear regression lines on two groups of data. The entire dataset is partitioned into two groups (i.e. groups I and II) based on elevation, and monthly P-T correlations are derived for the stations in each group. The P-T correlations for both groups are regressed with their corresponding elevations by fitting a least-squares linear regression model. Thereafter, the slopes of the linear regression models for each group are compared using the equal slopes test to check whether the P-T associations are influenced by the elevation changes or not. The null hypothesis () of this test considers that slopes from two independent populations are equal (i.e.
), while the alternate hypothesis is that these slopes are not equal (i.e.
) (Sachs Citation1982). Here,
are the population slope estimates, while
are the corresponding sample estimates. In this test, the samples (
,
) from populations are assumed to be independent and have equal residual variances
. The test statistic
is assessed by fitting a Student’s t-distribution, and the null hypothesis (
) is rejected when the value of
is greater than the critical value (i.e.
) at a given significance level α corresponding to
degrees of freedom. The test statistic
(Armitage et al. Citation2008) is derived as:
where and
are the regression slopes of the fitted P-T correlations against the elevations for group I and group II, having sample lengths
and
, respectively, and
is the standard error of the difference in slopes.
3 Case study domain and data description
The description of the case study region and its hydroclimatic characteristics along with the datasets analyzed in the study are discussed in the subsequent paragraphs.
3.1 Study area: Florida
The methods discussed in the previous section are used for the evaluation of changes in the individual and joint variation of P and T in the state of Florida. The state of Florida is in the southeastern United States, encompassing an area of 105 822 km2. Florida is surrounded by large water bodies on three sides, viz. the Atlantic Ocean, the Gulf of Mexico, and the Caribbean Sea to its east, west, and south respectively. The climatology of the state and the region (southeastern US) is influenced by AMO and ENSO along with frequent landfalls of hurricanes. Additional details of the case study region can be obtained from previous studies (Teegavarapu et al. Citation2013, Goly and Teegavarapu Citation2014). The climate in the study region is classified according to the Köppen-Geiger climate classification (Rubel and Kottek Citation2010) system as humid sub-tropical (Cfa) for most of the state with small areas identified as monsoon (Am), savanna (Aw), and rainforest (Af) climates in southern Florida. The mean annual precipitation across Florida ranges from 984 mm to 1638 mm (Teegavarapu et al. Citation2013). Peninsular Florida exhibits rather flat topography compared to the continental part (shown in ).
3.2 Datasets
Data on the daily precipitation depth and minimum, average, and maximum temperature (viz. Tmin, Tavg, and Tmax) were collected for 78 monitoring sites (shown in) across the state from the Florida Climate Center (FCC) (FSU Citation2020). The FCC disseminates the hydroclimatic data collected from the United States National Weather Service (NWS) first-order weather stations, NWS cooperative stations, and Federal Aviation Administration (FAA) sites in Florida. These stations were originally selected based on criteria such as record longevity, percentage of missing values, and spatial coverage as well as the number of station moves and/or other changes that might influence the homogeneity of the data.
A two-step quality control process was performed on these datasets (Kunkel et al. Citation2005). In the first step, a set of objective screening criteria to flag outliers was enforced on each P and T data value, which included extreme limits and spatial comparisons with nearby stations. In the second step, the climatologists manually assessed each outlier and flagged it as valid, plausible, questionable, or invalid (Kunkel et al. Citation2005). Thus, the homogeneity of the data is ensured through rigorous quality control procedures. The length of the data differs from one station to another, with only a few stations having long data records from 1892 onward. Data at most sites are available until 2017, with missing observations noted at several stations. However, for the sake of consistency, a common analysis period from 1950 to 2017 is used in this study. To ensure data consistency, the stations are carefully chosen such that the total number of missing observations at each station does not constitute more than 5% of the record length. Stations with a larger proportion of missing records were not included in the analyses. Also, the monthly records with missing daily observations at a given station were eliminated from the analysis to remove any possible bias.
The influence of climate variability manifested through two coupled oceanic and atmospheric oscillations and their respective phases is evaluated. The temporal windows corresponding to warm and cool phases of AMO are identified based on previous studies (Enfield et al. Citation2001, Teegavarapu et al. Citation2013, Goly and Teegavarapu Citation2014). AMO phases can also be identified using the Kaplan SST dataset provided by the Physical Sciences Laboratory (PSL) (PSL Citation2020). The three-month running mean values of the Oceanic Niño Index (ONI), available from the National Centers for Environmental Protection (NCEP) (NCEP Citation2020; www.origin.cpc.ncep.noaa.gov/, accessed May 2020), are used to derive the phases of ENSO which are classified as El Niño, neutral and La Niña. The ONI values are derived based on SST anomalies of Niño region 3.4 (5°N–5°S, 120–170°W). The El Niño and La Niña events are defined when five consecutive ONI values equal or exceed the +0.5 and −0.5 threshold levels, respectively, while ONI values within the range (−0.5, 0.5) denote the neutral ENSO events. The ONI presents a simple way to represent ENSO conditions, based on SST anomalies in the east-central equatorial Pacific, where the ocean is strongly coupled with the atmosphere (Bamston et al. Citation1997, Huang et al. Citation2016). Hence, the ONI is adopted for the classification of ENSO phases. However, other indices can also be used for the determination of temporal windows associated with various ENSO phases for the evaluation of P-T variability.
3.3 Hydroclimatic variability across Florida
Spatial heterogeneity in precipitation distribution is observed across Florida, wherein higher precipitation is noted across continental Florida as compared to peninsular Florida (except in southeast Florida). Since the topographic variation in the region is minimal, the orographic mode of precipitation is rather absent. The frontal and convective modes of precipitation are known to be predominant in dry and wet seasons, respectively. In general, the dry and wet seasons for Florida extend from November to April and May to October, respectively (Teegavarapu Citation2012). Continental Florida generally receives higher precipitation compared to the rest of the region since it is influenced by two different precipitation-generating mechanisms, viz. frontal precipitation during winter (dry) months, and spatially varying convective precipitation during summer (wet) months. The frontal precipitation has a diminishing influence from north to south in Florida. During summer, the higher daytime surface temperatures enhance the convection activity, and thus, most of the precipitation occurs during the daylight hours, especially in the afternoon. Also, due to its unique geographical location, Florida experiences frequent cyclonic activities manifested as hurricanes. In the past hundred years (1901–2002), around 86 hurricanes (cyclones or typhoons) and 73 tropical storms have affected Florida (http://www.orange.wateratlas.usf.edu/, accessed May 2020). Landfalls of hurricanes are the most numerous across Florida during September.
The climatology of monthly maximum, minimum, and average temperatures reveal widespread spatial variability across the state. In general, Tmin shows a higher temperature range compared to Tmax and Tavg. Central and southern Florida experience higher Tmax compared to continental Florida. These higher temperatures, especially during the summer months, may result in higher evaporation from Lake Okeechobee in south-central Florida. However, the higher Tmin and Tavg values are observed near southern Florida only. Continental Florida experiences sub-zero temperatures during the winter months, although the peninsular part remains somewhat warmer.
4 Results and analysis
The analyses of P and T data are conducted at daily, monthly, and multi-month time scales by applying multiple nonparametric statistical tests, discussed in Section 2. The analysis at the multi-month temporal scale is based on the collection of daily records for the months constituting the dry and wet seasons in Florida. The hypothesis-based statistical tests are conducted at a significance level (α = 0.05) of 5% in this study. The individual and coupled influences of the oscillations (viz. AMO and ENSO) on the joint variability of P and T are discussed in the subsequent sections.
4.1 Influence of climate variability on joint P-T variability
The precipitation patterns in the study region are influenced by both AMO and ENSO. Previous studies have shown that AMO and ENSO warm phases usually result in higher precipitation across Florida compared to AMO and ENSO cool phases (Goly and Teegavarapu Citation2014). However, the interplay of P-T dynamics under the influences of AMO and ENSO has not been explored for the study region. A cross-correlation analysis for precipitation and temperature has been performed and KDEs of the same are evaluated and compared. The cross-correlation values between P and T at each station are spatially interpolated using an inverse distance weighting-based interpolation method to assess the variation of P-T associations across Florida.
4.1.1 Monthly P-T associations: influence of AMO
The spatial precipitation pattern during AMO warm and cool phases exhibits a coherent structure across the state ()–(b)). Western continental Florida receives higher precipitation during both AMO phases compared to the rest of Florida. However, the KDEs show slightly higher mean values of precipitation during the AMO warm phase than the AMO cool phase, whereas higher extreme rainfall occurrences are observed in the latter ()). During the AMO warm phase, twice the number of tropical storms reportedly mature into hurricanes as compared to the AMO cool phase (Misra et al. Citation2011). These hurricanes could result in heavy precipitation in the region, particularly near their points of landfall.
Figure 4. Spatial variation of monthly total precipitation and maximum temperature for AMO warm and cool phases and their distributions
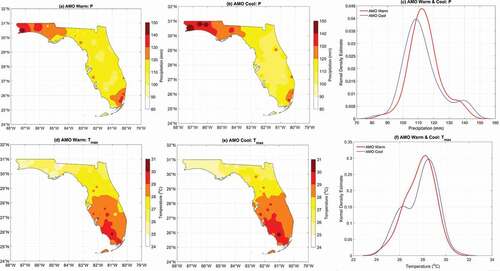
The spatial variations of maximum, minimum, and average temperatures during AMO warm and cool phases are found to be symmetric (–(e)). Central and southern Florida experiences a higher maximum temperature than continental Florida. The KDEs show marginally high maximum temperatures during the AMO cool phase compared to the AMO warm phase (). The KDEs for AMO cool exhibits bimodal characteristics. Similar variations are also observed for minimum and average temperatures. However, the changes in joint precipitation and temperature variations across the state during the two AMO phases are found to be statistically nonsignificant. Overall, the AMO warm phase contributes to wetter characteristics and milder temperatures compared to the AMO cool phase. The findings of Maleski and Martinez (Citation2018) for the southeastern United States contradict those from this study; they reported a decrease (increase) in precipitation and an increase (decrease) in mean temperature during the positive (negative) AMO phase. Also, Keellings and Waylen (Citation2015) reported an increase (decrease) in the frequency of heatwaves during the AMO warm (cool) phase across Florida. The findings of our study differ because our analyses are based on mean monthly climatic conditions as compared to the daily extremes.
The spatial P-T correlation patterns show variable responses for Tmax and Tmin, wherein the latter experiences higher correlations throughout central and southern Florida, while high correlations are confined within southern Florida in the former (– The spatial variation of correlation between P and Tavg is found to be rather uniform across AMO warm and cool phases –. Although continental Florida exhibits lower correlations, it receives relatively higher precipitation compared to peninsular Florida. This indicates that the convective mechanism is rather weak in precipitation generation across continental Florida, while frontal systems lead to precipitation events in this region. The KDEs of cross-correlation between P and Tmax,, Tmin, and Tavg are shown in .The KDEs indicate that the AMO cool phase exhibits higher correlations compared to the AMO warm phase.However, these correlations are not found to be statistically significant based on the Mann-Whitney U test evaluated at a 5% significance level. The KDEs associated with the AMO warm phase display higher densities compared to those of the AMO cool phase.
4.1.2 Monthly P-T associations: influence of ENSO
The spatial variation of precipitation across Florida, along with the KDEs, for different ENSO phases are shown in . The precipitation during El Niño is reported to be slightly higher than that in the neutral phase ()), whereas the spatial patterns for both phases are found to be symmetric (–(b)). Continental Florida receives marginally higher precipitation during the El Niño and neutral phases compared to the rest of Florida (except for a single patch in southeast Florida). Compared to El Niño and neutral phases, continental Florida receives much lower precipitation during La Niña (–(c))). Overall, the entire region receives considerably lower precipitation during La Niña. Thus, wetter conditions are prevalent during El Niño and neutral phases, while drier conditions are experienced during La Niña. Maleski and Martinez (Citation2018) reported, in general, an increase in precipitation during El Niño, while La Niña was associated with decreased precipitation and increased temperature across the southeastern United States. The findings of this study confirm the inferences drawn about P and T variability in the previous study.
Figure 7. Spatial variation of monthly total precipitation and maximum temperature for El Niño, ENSO Neutral, and La Niña and their distributions
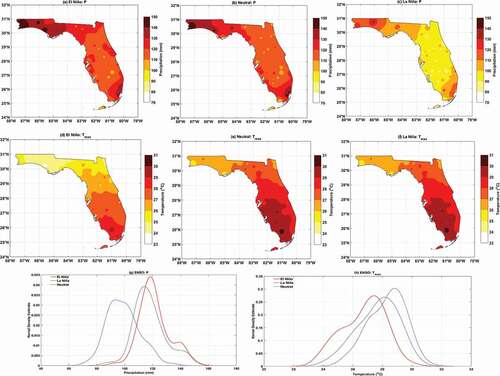
The maximum temperature across the region exhibits contrasting spatial variability to that observed for precipitation. Florida experiences lower Tmax during El Niño as compared to the neutral and La Niña phases ()–(f)). Also, continental Florida shows milder temperatures compared to peninsular Florida during all phases. Southwest peninsular Florida (i.e. the Everglades region) experiences fairly high temperatures during La Niña and neutral phases. The KDEs, however, indicate higher Tmax during the neutral phase than during La Niña ()). Overall, El Niño is associated with wet and warm conditions, whereas La Niña is associated with dry and hot conditions in the region. The neutral phase also demonstrates wet and hot tendencies across the state.
The spatial variation of these cross-correlations for El Niño, neutral, and La Niña phases is shown in . As shows, lower P-T correlations are noted across continental Florida as compared to peninsular Florida during all ENSO phases. This conforms to our earlier results, derived for the AMO phase. Overall, lower P-T correlations are observed during El Niño as compared to the neutral and La Niña phases (). On the other hand, P-T correlations during La Niña are much stronger in southern Florida, and they gradually weaken as we move northward (), (f), and (i)). Moreover, despite higher P-T correlations being observed during La Niña, the precipitation received during this phase is considerably lower than that during El Niño, irrespective of the higher temperatures observed during the former phase. This warrants further investigation regarding the dynamics governing the P-T relationship during ENSO phases. From the KDEs of P-T correlations, it is evident that El Niño exhibits lower correlations compared to neutral and La Niña phases ()–(f)). Strong correlations between precipitation and temperature (Tmax, Tmin, and Tavg) are observed during La Niña. The differences in P-T correlations amongst the ENSO phases are found to be statistically significant at α = 0.05.
4.1.3 Monthly P-T associations: coupled influences of AMO and ENSO
The study also evaluated the coupled influences of AMO and ENSO on the precipitation–temperature relationship across Florida. The joint occurrences of AMO and ENSO phases can be grouped into four possible combinations: (i) El Niño and AMO warm, (ii) El Niño and AMO cool, (iii) La Niña and AMO warm, and (iv) La Niña and AMO cool. The monthly data are extracted for the temporal windows coinciding with aforesaid combinations, and cross-correlations of P with Tmax, Tmin, and Tavg are, thereafter, estimated using SRC for all stations. The KDEs of these correlations are shown in . The KDEs indicate higher correlations during El Niño and AMO warm phase compared to El Niño and AMO cool phase ()–(c)). On the other hand, La Niña and AMO cool phase exhibit higher correlations than La Niña and AMO warm phase ()–(f)). Overall, it is evident that La Niña combinations demonstrate higher correlations than those of El Niño, an observation that provides support to our previous findings. Moreover, it is also seen that ENSO exerts a greater influence on the P-T relationships than AMO does. The Mann-Whitney U test, however, failed to reject the null hypothesis at a 5% significance level
for El Niño and La Niña combinations. The null hypothesis
is rejected for AMO warm and AMO cool combinations at a 5% significance level for all temperature series. Thus, the foregoing discussion indicates that the P-T relationship is considerably influenced by ENSO variability.
Figure 9. Variation in the distribution of correlations between precipitation and maximum, minimum, and average temperatures, for coupled AMO and ENSO combinations
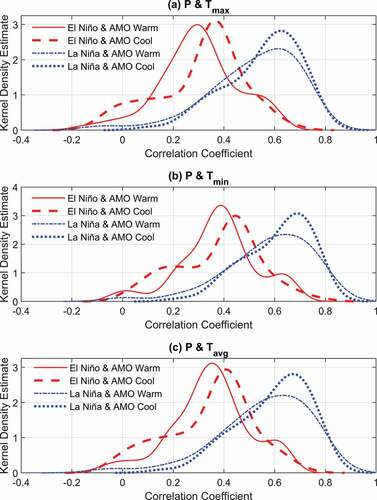
The spatial variations of P-Tmax correlations for AMO-ENSO combinations described earlier are shown in . Compared to El Niño ()–(b)), the La Niña ()–(d)) combinations display the prevalence of higher correlations across central and southern Florida. The highest correlations are observed during La Niña and AMO cool phase, wherein the whole of peninsular Florida (i.e. except the north)exhibits a stronger association (i.e. correlation coefficient greater than 0.5). However, the lowest correlation is evident across continental Florida compared to the rest of the region for all four combinations. This reinforces the fact that convection is not a major source of precipitation formation in continental Florida and frontal systems are rather dominant. Similar results are also noticed for P-Tmin and P-Tavg associations.
4.1.4 Monthly P-T associations: influence of topography
The influence of topographical changes on P-T associations is also explored using monthly data. From the geographical location attributes of the dataset, it is found that around 51% (49%) stations are located at elevations lower (higher) than 10 m. The assessment of the influence of elevation changes on the P-T association under climate variability is carried out by dividing the elevation range in the study region into two groups (i.e. groups I and II) along with their conditions (viz. (I) elevations > 10 m and (II) elevations < 10 m). The monthly P-T correlations between these two groups during different AMO and ENSO phases are compared. These P-T correlations for each group are fitted with linear regression models, and the significant difference in the slopes of the two groups is assessed using the equal slopes test described in Section 2.3. The results show that the monthly P-T correlations exhibit an inverse relationship with elevation for both groups, irrespective of the oscillation phase. The equal slopes test fails to reject the null hypothesis at a 5% significance level
for all cases during both ENSO and AMO phases (except for P-Tmax during El Niño). Further, to remove any plausible bias due to the grouping of the data, random sampling of sites with different elevations is employed to obtain two groups, with each group having a minimum sample length of 20 (i.e. at least 25% of the entire dataset). Multiple random sampling-based groups thus generated are evaluated using the equal slopes test. The results compiled from successful simulation runs suggest that the slopes test fails to reject the null hypothesis
at a 5% significance level (α) for most occasions, except for P-Tmax and P-Tavg correlations during El Niño, when the number of samples in group I are 48 or more. Overall, it can be inferred that the differences noted in P-T associations due to climate variability mostly remain unaffected by the changes in the topography of the region.
4.1.5 Seasonal P-T associations
As discussed in Section 4.1.1, the AMO warm phase exhibits wetter characteristics and milder temperatures compared to the AMO cool phase. Also, wetter and warmer conditions are observed during El Niño, whereas drier and hotter conditions are prevalent during La Niña. However, the P-T correlations were positive and stronger in La Niña (AMO cool) than El Niño (AMO warm). Thus, a paradoxical outcome is visible, wherein despite higher P-T correlations in La Niña and AMO cool phase, it receives comparatively lower rainfall. To understand this paradox, the P-T relationship is further investigated at multi-month (or seasonal) time scales. In Florida, the dry (i.e. winter) season ranges from November to April, whereas the wet (i.e. summer) season spans from May to October. The daily non-zero precipitation and corresponding temperatures are extracted for dry and wet seasons and their long-term means are estimated at each station.
The spatial variation of precipitation during dry and wet seasons for AMO and ENSO phases across Florida is shown in and(see the Appendix), respectively. As shows, the region experiences higher precipitation during the AMO warm phase in the wet season compared to the dry season (except western continental Florida) (shown in (a)–(c)). Also, higher precipitation is reported during the AMO warm phase than the AMO cool phase in the wet season ((a)–(b)). Considerably higher precipitation is observed in the dry season over continental Florida in the AMO cool phase ((d)). The Mann-Whitney U test rejected (failed to reject) the null hypothesis for wet- (dry-) season precipitation during AMO warm and cool phases at a 5% significance level (α).
As shows, El Niño and La Niña exhibit minimal deviations in precipitation distribution during wet months. Continental Florida receives marginally higher precipitation than the rest of Florida in the wet season ((a)–(b)). On the other hand, there is a marked difference between precipitation during El Niño and La Niña in the dry season ((c)–(d)). During dry El Niño, widespread heavy precipitation is witnessed across entire Florida, whereas comparatively much lower precipitation is recorded during dry La Niña. Also, the Mann-Whitney U test indicated a rejection of the null hypothesis at a 5% significance level for dry season precipitation during El Niño and La Niña phases. The variability in the response of precipitation between dry and wet seasons is attributed to the presence of stronger ENSO teleconnections across the southeastern US and Florida in the winter months than in the summer months (Kirtman et al. Citation2017).
The P-T correlations in the wet season are found to be higher for the AMO cool phase compared to the AMO warm phase, as shown in )–(c). The Mann-Whitney U test rejected the null hypothesis for correlations between P and Tmax and P and Tavg at a 5% significance level
for the wet season. On the other hand, the AMO warm phase exhibits higher P-T correlations than the AMO cool phase in the dry season ()–(f)). The Mann-Whitney U test showed statistically significant variations (at a 5% significance level (α)) in the P-T associations for all cases between AMO warm and cool phases in the dry season.
Figure 11. Variation in the distribution of correlations between daily precipitation and maximum, minimum, and average temperatures during wet and dry AMO phases
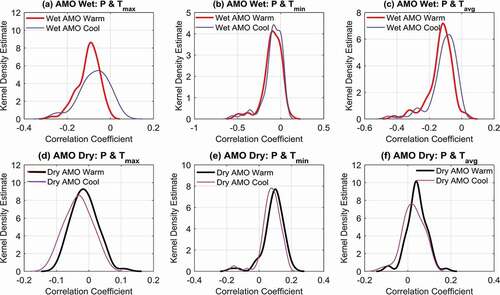
The P-T correlations are found to be lower for dry El Niño compared to dry La Niña conditions (except for P and Tmin), while marginally higher P-T correlations are noticed during the wet La Niña phase than the wet El Niño phase (). The P-T associations during El Niño and La Niña are found to exhibit statistically nonsignificant variations in both wet and dry seasons, based on the results of the Mann-Whitney U test. However, asymmetric intra-seasonal differences in P-T correlations during El Niño and La Niña, especially in the dry season, have resulted in the deflation of overall P-T correlations for the El Niño phase. Also, it can be inferred that during the wet season, when higher temperatures are prevalent, convection plays a major role in precipitation occurrence. However, during the dry season, which is comparatively cooler, El Niño significantly enhances the precipitation. Thus, climate variability exerts a predominant influence in the dry season compared to the wet season. O’Brien et al. (Citation1999) also indicated that prolonged drought extends into the warm season in the case of La Niña compared to El Niño.
4.1.6 Daily P-T associations
The P-T associations are further examined at the daily time scale. The non-zero precipitation days and corresponding temperature data are mined from the entire dataset. The zero values were removed since the presence of many such values tends to inflate the correlations. The temperatures are binned into several classes (equal intervals), and the precipitation occurrences under each class during various ENSO and AMO phases have been worked out. The probability density of the daily precipitation occurrences during ENSO and AMO phases within each temperature bin is shown in . As ) shows, a nearly symmetrical precipitation distribution is observed for both AMO warm and cool phases. Also, occurrences of extreme precipitation can be noted in both phases. On the other end, occurrences of extreme precipitation are more frequent during El Niño than during the La Niña phase ()). The percentage of extreme precipitation days (i.e. P ≥ 100 mm) among the total days corresponding to different ENSO phases have been separately worked out for wet and dry seasons at each station and compiled. The percentage of extreme precipitation days for El Niño and La Niña during the wet season are found to be 0.24% and 0.21%, respectively, while the corresponding values for the dry season are estimated as 0.12% and 0.07% over the entire study region. Thus, a considerable difference in the occurrence of extreme precipitation is noted between El Niño and La Niña phases in the dry season compared to the wet season.
Figure 13. Distribution of daily precipitation depths within various maximum temperature intervals for (a) AMO and (b) ENSO phases
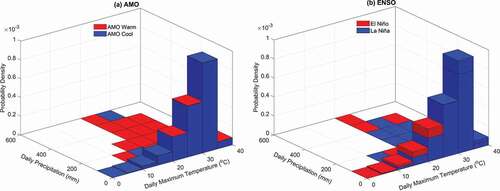
To investigate the inconsistencies between the P-T correlations and their occurrence, an investigation of precipitation and temperature variability for different temperature intervals is attempted. While extracting the daily non-zero precipitation and corresponding temperature values for ENSO and AMO phases, a higher frequency of observations is usually found in El Niño (AMO warm) datasets compared to La Niña (AMO cool), which could potentially induce bias while attempting to derive meaningful inferences. To overcome this bias due to indifferent sample sizes, computations using the LHBS approach are executed. In this study, the maximum temperature values from the dataset for each ENSO/AMO phase are initially stratified into equal intervals of 5°C, and corresponding precipitation values are extracted. But since the interval 0–5°C contained very few samples, it has been merged with the next higher interval (i.e. 5–10°C), ultimately resulting in seven intervals or strata. The population size for deriving bootstrap sampling varied for each temperature interval depending upon the minimum number of values in either phase; however, equal population size is adopted for El Niño (AMO warm) and La Niña (AMO cool) within each interval. After deriving bootstrap samples for each interval, 2000 realizations of those samples were generated for both phases, and their associations are compared.
The changes in the distribution of P-T correlations within each interval for AMO warm and cool phases are shown in . The results indicate minimal variability in the KDEs of AMO warm and cool phases for temperature intervals below 20°C ()–(c)). However, for temperature intervals 20–25°C and 25–30°C, the AMO warm phase exhibits a significant shift in the distribution compared to the AMO cool phase, wherein predominantly positive correlations are observed in the former ()–(e)). In contrast, the temperature intervals above 30°C demonstrate higher tendencies of positive correlation during the AMO cool phase compared to the AMO warm phase ()–(g)). The fluctuations observed in correlations within different temperature intervals for AMO yield nonsignificant variations in their overall correlations between AMO warm and cool phases, as described in Sections 4.1.1 and 4.1.5.
Figure 14. Variation in the distribution of correlations within different temperature intervals for AMO warm and cool phases using the LHBS approach
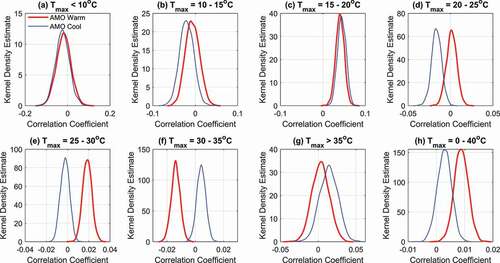
The changes in the distribution of P-T correlations within each interval for El Niño and La Niña phases are shown in . In , lower correlations can be observed during El Niño than in La Niña for all temperature intervals (except in 10–15°C and above 35°C). However, these two intervals exhibit relatively fewer peak densities for both phases as compared to the intervals 20–25°C, 25–30°C, and 30–35°C ()–(e)). In the aforementioned three intervals, higher densities of negative (positive) correlations are seen during El Niño (La Niña). Moreover, a distinct shift in the KDEs can be noted within both phases, and these are found to be statistically significant at α = 0.05. Thus, the predominance of negative correlations during El Niño in these three intervals deflates its overall correlation compared to La Niña, which also holds when viewed at coarser temporal scales.
Figure 15. Variation in the distribution of correlations within different temperature intervals for El Niño and La Niña using the LHBS approach
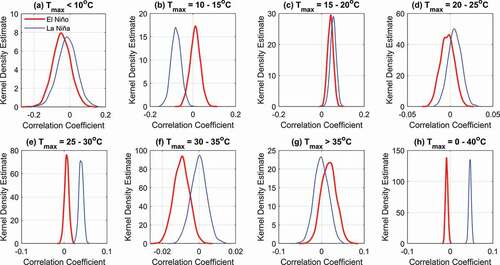
The percentage of precipitation days and mean precipitation depths within each interval are further estimated for El Niño and La Niña phases only, since they exhibit considerable variation compared to those for AMO phases. Here, precipitation days refer to days with positive values for precipitation (i.e. P > 0 mm). The percentages of precipitation days relative to the total days during El Niño and La Niña for the wet season are estimated to be 39.3% and 38.9%, respectively, while the corresponding values for the dry season are reported to be 25.7% and 19.4% over the entire study region. Further, the number of precipitation days is estimated for each interval and divided by the total number of days within that interval for El Niño and La Niña. The distribution of the percentage of precipitation days within various temperature intervals and as one entity is shown in (see the Appendix). As shows, the percentage of precipitation days during El Niño and La Niña monotonically increases with temperature up to 35°C; however, beyond that it shows a decreasing tendency. The region experiences a significant and consistently higher percentage of precipitation days during El Niño than La Niña. But for temperatures greater than 35°C, this difference becomes negligible. The mean daily precipitation depths for various temperature intervals were also estimated and are shown in (see the Appendix). From , it can be noted that for lower temperatures (i.e. Tmax < 30°C), significantly higher mean precipitation depths are evident during El Niño than La Niña, but a reversal occurs for higher temperatures (i.e. Tmax > 30°C), where higher precipitation tendency is seen for La Niña compared to El Niño. Thus, higher precipitation occurs at lower temperatures during the El Niño phase, while at higher temperatures the influence of climate variability is inconsequential, and local hydroclimatic conditions likely predominate.
From the foregoing analyses, a better understanding of the decrease in precipitation during La Niña was derived by evaluating storms that result in precipitation other than convective or convergent storms during the warm season, common in peninsular Florida. While a stronger association (quantified by SRC) was found between temperature and precipitation in La Niña compared to El Niño, the higher temperatures prevalent during La Niña do not necessarily result in higher precipitation totals. El Niño conditions facilitate increased precipitation in the temperature range of 0–30°C compared to La Niña. These anomalously high precipitation amounts occurring at lower temperatures reduce the strength of the P-T association, and the lack of such precipitation variability during La Niña inflates the overall association.
4.2 Physical basis of P-T variability
The AMO warm and cool phases have contributed to the formation of low-pressure anomalies over North America, and to low- and high-pressure anomalies over the sub-tropical North Atlantic and the eastern sub-tropical Pacific, respectively (Hu et al. Citation2011). These changes in pressure patterns due to the AMO were identified as one of the mechanisms leading to climatic shifts in precipitation over Florida on a multi-decadal time scale. The jet streams, which guide fronts and storms, are more frequently observed to pass near Florida during El Niño than during La Niña (O’Brien et al. Citation1999). Therefore, the pressure and wind disturbances resulting in frequent storm events could be attributed to the increase in precipitation during the El Niño phase. This means that the presence of strong jet streams during El Niño enhances the moisture fluxes over the land surface and results in higher precipitation. In contrast, a poleward displacement of the mid-latitude jet was observed during La Niña, which led to zonal airflow and drying over the region (Ropelewski and Halpert Citation1986). The jet streams are weaker during La Niña, wherein the localized convection exerts a predominant influence on the precipitation occurrence, thus overshadowing the climatic variability (O’Brien et al. Citation1999). Also, a strong (weak) circulation response was observed during winter (summer) El Niño and La Niña, which was found to be consistent with the strong response in temperature and precipitation patterns during winter (Kirtman et al. Citation2017).
The presence of numerous wetlands and a large water body (i.e. Lake Okeechobee) in south-central Florida and higher temperatures would lead to increased evaporation rates, which enhances the convection in the region. The regional increase in summer temperatures and decrease in precipitation were attributed to the conversion of wetlands into agricultural and urbanized areas during the 20th century (Pielke et al. Citation1999, Marshall et al. Citation2003, Volk et al. Citation2017) in peninsular Florida.
4.3 General remarks
The lack of long-term gap-free chronologically continuous daily precipitation and temperature data is one of the limitations in conducting a comprehensive analysis of individual and joint temporal variations of P and T in the region. Missing data in the P and T time series also contributed to unequal sample sizes in temporal windows that are associated with different phases of oscillations. The LHBS approach adopted in this study reduced the bias associated with such sample size variability. However, there is a possibility that due to missing data, characteristics of the P and T data that explain variations in extremes and relationships between them may not have been fully assessed. The assessment of the spatial variability of the two variables in this study is limited by the low spatial density of raingauges in the region, although the observation sites (i.e. stations) are uniformly located across the region. Gridded datasets that uniformly cover the entire region and infilling approaches to eliminate the gaps in the data could be used to improve the analysis. However, these datasets may deflate the variability of P and T in the region compared to point data (i.e. station data) and may not provide an accurate assessment of changes in these variables over time or due to climate variability and climate change. This study provides insights into P-T associations across Florida under the influence of climate variability; however, attribution studies need to be done to gain more insights into the local hydroclimatic conditions influencing P-T dynamics. Future studies can explore the role of meteorological and atmospheric variables such as vapour pressure, relative humidity, precipitable water, and others on precipitation–temperature associations, which could improve the region-specific knowledge and attribution.
5 Conclusions
A comprehensive analysis of precipitation and temperature data on various temporal scales (daily, monthly, and multi-month) for a tropical region that is influenced by AMO and ENSO is carried out in this study. A spatially non-uniform influence of these two oscillations on the P-T associations is noted across the region. The results indicate that the warm (cool) AMO phase is associated with wet and cool (dry and hot) conditions, and likewise the warm (cool) ENSO phase is associated with wet and cool (dry and hot) conditions.However, the cross-correlations between precipitation and temperature are higher and stronger during La Niña as compared to El Niño. On the other hand, the AMO cool phase exhibits marginally higher correlations than the AMO warm phase. The coupled influences of AMO and ENSO reveal that the spatial extent of stronger positive correlation is higher for La Niña combinations (with AMO phases) compared to El Niño combinations, which indicates the greater influence of ENSO than AMO on the P-T relationship. The P-T association at the seasonal scale displayed significant differences in precipitation patterns across Florida between El Niño and La Niña in the dry season (November to April), wherein the former receives significantly higher precipitation. On the other hand, nominal variations are seen in P-T associations between the ENSO phases for the wet season (May to October). Despite higher P-T correlations noted in La Niña, precipitation totals in La Niña were considerably lower than those during El Niño in the study region.
The daily P-T relationship has been explored for various temperature intervals for AMO and ENSO phases using the LHBS approach to reduce bias due to unequal sample sizes of temporal windows for different oscillations. The AMO phases exhibit fluctuations in correlations within different temperature intervals, which nullifies their overall correlation variability. However, significantly higher densities of negative P-T correlation are seen during El Niño within the intervals 20–25, 25–30, and 30–35°C, which deflates the overall P-T correlation reported for El Niño. Also, the mean precipitation depth (percentage of precipitation days) exhibits a monotonic rise for temperatures up to 30°C (35°C) for both ENSO phases, wherein El Niño shows considerably higher values compared to La Niña at lower temperatures. Thus, higher precipitation occurs at lower temperatures during the El Niño phase. The results from the LHBS approach in this study suggest stronger climate variability influence in generating higher precipitation during El Niño compared to La Niña than the regional precipitation contributing processes (i.e. convective and convergence) for this region. The P-T associations under the influence of climate variability are found to be mostly elevation invariant. Improved understanding of the P-T relationships across a tropical region (i.e. Florida) under the influence of climate variability can benefit different aspects of water resource and disaster management.
Data availability
The data used in this study are available in the public domain and are provided by the Florida Climate Center (https://climatecenter.fsu.edu/climate-data-access-tools/downloadable-data). The monthly time series of the Atlantic Multidecadal Oscillation (AMO) Index and Oceanic Niño Index (ONI) of the ENSO for the Niño 3.4 region were downloaded from the NOAA website at https://psl.noaa.gov/data/timeseries/AMO/ (accessed on 30 May 2020) and https://psl.noaa.gov/gcos_wgsp/Timeseries/Niño34/ (accessed on 30 May 2020), respectively.
Acknowledgements
The authors thank the Florida Climate Center (FCC) for providing the precipitation and temperature datasets, and the anonymous reviewers for their constructive comments and suggestions.
Disclosure statement
No potential conflict of interest was reported by the authors.
References
- Abiy, A.Z., et al., 2019. Rainfall trend and variability in Southeast Florida: implications for freshwater availability in the Everglades. PloS One, 14 (2), e0212008. doi:https://doi.org/10.1371/journal.pone.0212008
- Ali, H. and Mishra, V., 2017. Contrasting response of rainfall extremes to increase in surface air and dewpoint temperatures at urban locations in India. Scientific Reports, 7 (1), 1–15. doi:https://doi.org/10.1038/s41598-017-01306-1
- Armitage, P., Berry, G., and Matthews, J.N.S., 2008. Statistical methods in medical research. New York (NY): John Wiley and Sons.
- Bamston, A.G., Chelliah, M., and Goldenberg, S.B., 1997. Documentation of a highly ENSO‐related SST region in the equatorial Pacific: research note. Atmosphere-Ocean, 35 (3), 367–383. doi:https://doi.org/10.1080/07055900.1997.9649597
- Berg, P. and Haerter, J.O., 2013. Unexpected increase in precipitation intensity with temperature - A result of mixing of precipitation types? Atmospheric Research, 119, 56–61. doi:https://doi.org/10.1016/j.atmosres.2011.05.012
- Berg, P., Moseley, C., and Haerter, J.O., 2013. Strong increase in convective precipitation in response to higher temperatures. Nature Geoscience, 6 (3), 181–185. doi:https://doi.org/10.1038/ngeo1731
- Chiew, F.H., et al., 1998. El Nino/Southern Oscillation and Australian rainfall, streamflow and drought: links and potential for forecasting. Journal of Hydrology, 204 (1–4), 138–149. doi:https://doi.org/10.1016/S0022-1694(97)00121-2
- Corder, G.W., and Foreman, D.I., 2014. Nonparametric statistics: a step-by-step approach. 2nd ed. New Jersey: Wiley.
- Enfield, D.B., Mestas‐Nuñez, A.M., and Trimble, P.J., 2001. The Atlantic Multidecadal Oscillation and its relation to rainfall and river flows in the continental U.S. Geophysical Research Letters, 28 (10), 2077–2080. doi:https://doi.org/10.1029/2000GL012745
- FSU, 2020. https://climatecenter.fsu.edu/climate-data-access-tools/downloadable-data [ Accessed April 2020].
- Goly, A. and Teegavarapu, R.S.V., 2014. Individual and coupled influences of AMO and ENSO on regional precipitation characteristics and extremes. Water Resources Research, 50 (6), 4686–4709. http://www.orange.wateratlas.usf.edu/ [ Accessed 30 May 2020].
- Hu, Q., Feng, S., and Oglesby, R.J., 2011. Variations in North American summer precipitation driven by the Atlantic Multidecadal Oscillation. Journal of Climate, 24 (21), 5555–5570. doi:https://doi.org/10.1175/2011JCLI4060.1
- Huang, B., et al., 2016. Ranking the strongest ENSO events while incorporating SST uncertainty. Geophysical Research Letters, 43 (17), 9165–9172. doi:https://doi.org/10.1002/2016GL070888
- Irizarry‐Ortiz, M.M., et al., 2013. Historical trends in Florida temperature and precipitation. Hydrological Processes, 27 (16), 2225–2246. doi:https://doi.org/10.1002/hyp.8259
- Jones, H.R., Westra, S., and Sharma, A., 2010. Observed relationships between extreme sub‐daily precipitation, surface temperature, and relative humidity. Geophysical Research Letters, 37 (22). doi:https://doi.org/10.1029/2010GL045081
- Kashid, S.S., Ghosh, S., and Maity, R., 2010. Streamflow prediction using multi-site rainfall obtained from hydroclimatic teleconnection. Journal of Hydrology, 395 (1–2), 23–38. doi:https://doi.org/10.1016/j.jhydrol.2010.10.004
- Keellings, D. and Waylen, P., 2015. Investigating teleconnection drivers of bivariate heat waves in Florida using extreme value analysis. Climate Dynamics, 44 (11–12), 3383–3391. doi:https://doi.org/10.1007/s00382-014-2345-8
- Kirtman, B.P., et al. 2017. Florida climate variability and prediction. In: E.P. Chassignet, et al., eds. Florida’s climate: changes, variations, and impacts. Gainesville: Florida Climate Institute, 511–532. doi:https://doi.org/10.17125/fci2017.ch17
- Kunkel, K.E., et al., 2005. Quality control of pre-1948 cooperative observer network data. Journal of Atmospheric and Oceanic Technology, 22 (11), 1691–1705. doi:https://doi.org/10.1175/JTECH1816.1
- Madden, R.A. and Williams, J., 1978. The correlation between temperature and precipitation in the United States and Europe. Monthly Weather Review, 106 (1), 142–147. doi:https://doi.org/10.1175/1520-0493(1978)106<0142:TCBTAP>2.0.CO;2
- Maleski, J.J. and Martinez, C.J., 2018. Coupled impacts of ENSO AMO and PDO on temperature and precipitation in the Alabama–Coosa–Tallapoosa and Apalachicola–Chattahoochee–Flint river basins. International Journal of Climatology, 38, e717–e728. doi:https://doi.org/10.1002/joc.5401
- Marshall, C.H., Pielke, R.A., and Steyaert, L.T., 2003. Crop freezes and land-use change in Florida. Nature, 426 (6962), 29–30. doi:https://doi.org/10.1038/426029a
- McKay, M.D., Beckman, R.J., and Conover, W.J., 1979. Comparison of three methods for selecting values of input variables in the analysis of output from a computer code. Technometrics, 21 (2), 239–245. doi:https://doi.org/10.2307/1268522
- Mishra, V., Wallace, J.M., and Lettenmaier, D.P., 2012. Relationship between hourly extreme precipitation and local air temperature in the United States. Geophysical Research Letters, 39 (16). doi:https://doi.org/10.1029/2012GL052790
- Misra, V., et al., 2011. The influence of the Atlantic Warm Pool on the Florida panhandle sea breeze. Journal of Geophysical Research: Atmospheres, 116 (D21). doi:https://doi.org/10.1029/2010JD015367
- Misra, V. and Bhardwaj, A., 2020. Understanding the seasonal variations of peninsular Florida. Climate Dynamics, 54 (3), 1873–1885. doi:https://doi.org/10.1007/s00382-019-05091-7
- Murgulet, D., et al., 2017. Relationships between sea surface temperature anomalies in the Pacific and Atlantic Oceans and South Texas precipitation and streamflow variability. Journal of Hydrology, 550, 726–739. doi:https://doi.org/10.1016/j.jhydrol.2017.05.041
- Murnane, R.J., 2004. Climate research and reinsurance. Bulletin of the American Meteorological Society, 85 (5), 697–708. doi:https://doi.org/10.1175/BAMS-85-5-697
- NCEP, 2020. National centers for environmental protection (NCEP). https://origin.cpc.ncep.noaa.gov/products/analysis_monitoring/ensostuff/ONI_v5.php [ Accessed 15 April 2020].
- O’Brien, J.J., et al., 1999. El Niño, La Niña and Florida’s climate: effects on agriculture and forestry. Tallahassee, FL: The Florida Consortium: The Florida State University, the University of Florida, University of Miami.
- Pielke, R.A., Sr, et al., 1999. The influence of anthropogenic landscape changes on weather in south Florida. Monthly Weather Review, 127 (7), 1663–1673. doi:https://doi.org/10.1175/1520-0493(1999)127<1663:TIOALC>2.0.CO;2
- PSL, 2020. Physical sciences laboratory (PSL). https://psl.noaa.gov/data/correlation//amon.us.long.data [ Accessed April 2020].
- Ropelewski, C.F. and Halpert, M.S., 1986. North American precipitation and temperature patterns associated with the El Niño/Southern Oscillation (ENSO). Monthly Weather Review, 114 (12), 2352–2362. doi:https://doi.org/10.1175/1520-0493(1986)114<2352:NAPATP>2.0.CO;2
- Rousseau, R., Egghe, L., and Guns, R., 2018. Becoming metric-wise: a bibliometric guide for researchers. Kidlington: Elsevier-Chandos.
- Rubel, F. and Kottek, M., 2010. Observed and projected climate shifts 1901–2100 depicted by world maps of the Köppen-Geiger climate classification. Meteorologische Zeitschrift, 19 (2), 135–141. doi:https://doi.org/10.1127/0941-2948/2010/0430
- Sachs, L., 1982. Applied statistics: a handbook of techniques. New York: Springer Science & Business Media, 440–442.
- Sharma, P.J., Patel, P.L., and Jothiprakash, V., 2020. Hydroclimatic teleconnections of large-scale oceanic-atmospheric circulations on hydrometeorological extremes of Tapi Basin, India. Atmospheric Research, 235, 104791. doi:https://doi.org/10.1016/j.atmosres.2019.104791
- Sharma, S. and Mujumdar, P.P., 2019. On the relationship of daily rainfall extremes and local mean temperature. Journal of Hydrology, 572, 179–191. doi:https://doi.org/10.1016/j.jhydrol.2019.02.048
- Sun, X., et al., 2015. A global analysis of the asymmetric effect of ENSO on extreme precipitation. Journal of Hydrology, 530, 51–65. doi:https://doi.org/10.1016/j.jhydrol.2015.09.016
- Teegavarapu, R.S.V., 2012. Floods in a changing climate: extreme precipitation. London: Cambridge University Press.
- Teegavarapu, R.S.V., Goly, A., and Obeysekera, J., 2013. Influences of Atlantic Multi-decadal Oscillation on regional precipitation extremes. Journal of Hydrology, 495, 74–93. doi:https://doi.org/10.1016/j.jhydrol.2013.05.003
- Tian, Q., Prange, M., and Merkel, U., 2016. Precipitation and temperature changes in the major Chinese river basins during 1957–2013 and links to sea surface temperature. Journal of Hydrology, 536, 208–221. doi:https://doi.org/10.1016/j.jhydrol.2016.02.048
- Trenberth, K.E., 2011. Changes in precipitation with climate change. Climate Research, 47 (1–2), 123–138. doi:https://doi.org/10.3354/cr00953
- Trenberth, K.E., Fasullo, J., and Smith, L., 2005. Trends and variability in column-integrated atmospheric water vapor. Climate Dynamics, 24 (7–8), 741–758. doi:https://doi.org/10.1007/s00382-005-0017-4
- Trenberth, K.E. and Shea, D.J., 2005. Relationships between precipitation and surface temperature. Geophysical Research Letters, 32 (14). doi:https://doi.org/10.1029/2005GL022760
- Utsumi, N., et al., 2011. Does higher surface temperature intensify extreme precipitation? Geophysical Research Letters, 38 (16). doi:https://doi.org/10.1029/2011GL048426
- Volk, M.I., et al., 2017. Florida land use and land cover change in the past 100 years. In: Florida’s climate: changes, variations, and impacts, 51–82. Gainesville, FL: Florida Climate Institute. https://doi.org/https://doi.org/10.17125/fci2017.ch02.
- Wang, G., et al., 2017. The peak structure and future changes of the relationships between extreme precipitation and temperature. Nature Climate Change, 7 (4), 268–274. doi:https://doi.org/10.1038/nclimate3239
- Wasko, C., Parinussa, R.M., and Sharma, A., 2016. A quasi‐global assessment of changes in remotely sensed rainfall extremes with temperature. Geophysical Research Letters, 43 (24), 12–659. doi:https://doi.org/10.1002/2016GL071354
- Wilks, D.S., 2011. Statistical methods in the atmospheric sciences (Vol. 100). Cambridge, MA: Academic press.
- Zhao, W. and Khalil, M.A.K., 1993. The relationship between precipitation and temperature over the contiguous United States. Journal of Climate, 6 (6), 1232–1236. https://www.jstor.org/stable/26197265
Appendix
Figure A1. Spatial variation of mean daily precipitation during wet and dry AMO (Atlantic multidecadal oscillation) phases
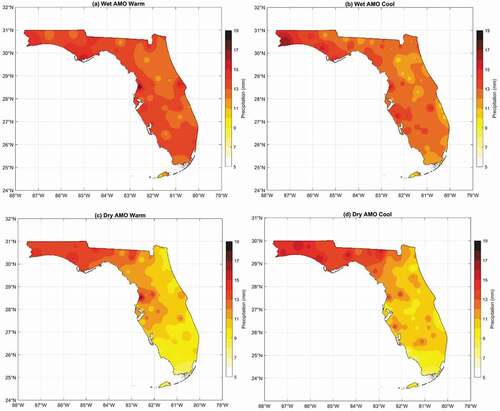
Figure A2. Spatial variation of mean daily precipitation during wet and dry ENSO (El Niño Southern Oscillation) phases
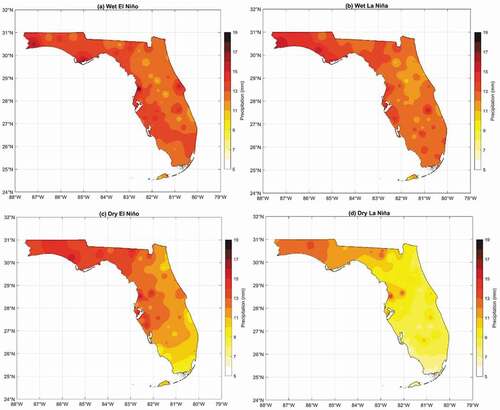