ABSTRACT
Background Data commentary, in-text comments on the visual presentation of data, is acknowledged as a central aspect of academic writing in many engineering disciplines. At the same time, it is a feature that has been shown to be challenging for students. One of the genres in which data commentary plays a significant role in many engineering disciplines is the master’s thesis. Comparatively little research has been done on the process of master thesis supervision, and combining the study of data commentary and master’s thesis supervision is therefore particularly interesting.
Purpose This study explores the challenges of data commentary writing through interviews with master’s students and thesis supervisors of chemical engineering.
Sample and method Master’s students at a Swedish university were invited to participate in a workshop about the writing of data commentary. Nine master’s students and five supervisors were interviewed about what is difficult and important about writing data commentaries in their discipline as well as about decisions made in data commentaries written by the students. The interviews were divided into a semi-structured and a discourse-based part.
Results Our results indicate that data commentary comes with a variety of challenges. Among the most difficult and important aspects are selection of content and clarity. The study also indicates a close connection between data commentary and disciplinary learning in chemical engineering, suggesting that highlighting data commentary in the teaching of master’s thesis writing will be time well spent.
Conclusions In order to make the teaching and learning of data commentary effective in the context investigated, we propose that important measures are: the development of a shared metalanguage among students and supervisors, a genre approach, and collaboration between engineering and communication faculty.
Introduction and background
The in-text commentaries accompanying visual displays of results (tables, figures, etc.) in academic texts are often referred to as data commentaries (Swales and Feak Citation2012; 139). Data commentaries play a central role in many disciplinary genres, partly because the main argument often unfolds as the authors move between visual displays and running text. Poe, Lerner, and Craig (Citation2010, 115), for example, argue that ‘visual representations of data are the work horses of arguments’ in biotechnology writing, and Curry (Citation2014) demonstrates how three research groups from different engineering groups use visual material as their point of departure for identifying results and for conceptualising and drafting the scientific story of their research papers. In the end, Curry shows that visual material is essential in text and knowledge production in several engineering disciplines. The importance of visual material is also highlighted through studies of graphing, the ability to interpret and produce graphs. For instance, Nixon et al. (Citation2016) describe graphing as an essential aspect of thinking like a physicist.
The centrality of data commentary in science and engineering writing is also indicated by the fact that most technical communication as well as academic writing books today give basic guidance to data commentary and data visualisation. At the same time, several studies point out that text books do not provide comprehensive guidance for learners (Poe, Lerner, and Craig Citation2010; Wolfe Citation2015) and that data commentary remains problematic for novice writers. Curry (Citation2014), for instance, calls for a broader focus on multimodality in both research and writing instruction, the main reason being that visual material tends to play a crucial role in the organisation of the storyline, and Poe, Lerner, and Craig (Citation2010) argue that text books often place too much focus on surface features, such as language accuracy, and too little on argumentation and selection of data. Further, Sancho Guinda (Citation2012) discusses Spanish second-year engineering students’ difficulties when commenting on data in a number of pre-selected charts and chart types, and argues for a discipline-specific, moves-based approach to data commentary instruction (Sancho Guinda Citation2012, 373; cf. Swales Citation1990; Swales and Feak Citation2012), without going into detail about how to organise such instruction.
In addition to these studies, our own experience from teaching academic writing to PhD students at universities of technology tells us that the combination of visualisation and data commentary tends to be central when presenting and ordering results in certain disciplines. One such discipline is chemical engineering. Many of our PhD students in this discipline have described that the composition of an article often starts with decisions about what figures to include, how to order them and how to build an argument around the order chosen. However, this understanding and description of the role of data commentaries stand in quite sharp contrast to how some students present data commentaries in their master’s theses. For instance, in a corpus of master theses within chemical engineering collected for a previous study (Nordrum and Eriksson Citation2015), we found that some students consistently referred only generally to the results displayed without further commenting on them, as in the following example:
The Coefficient of Variance is calculated from a cross section 11 m from injection to compare the rheology models. The results from the simulations with a single injection point are given in Figures 3–17.
In this study, we investigate students’ and supervisors’ perceptions of data commentaries in master’s theses in chemical engineering. We start from the assumption that data commentary in results sections is a central subgenre within master’s theses and journal papers in chemical engineering (cf. Curry Citation2014; Poe, Lerner, and Craig Citation2010; Sancho Guinda Citation2012) and that it is therefore of particular rhetorical and disciplinary value. Our study explores three research questions:
What do students and supervisors of chemical engineering perceive to be difficult when working with data commentaries in master’s theses?
What do students and supervisors of chemical engineering perceive to be important when working with data commentaries in master’s theses?
Can any pedagogical challenges related to data commentary be found in the context investigated, and if so, what are those challenges?
Master’s thesis supervision
Comparatively little work has been done on master’s thesis supervision, as most work on supervision in higher education concerns doctoral students. Some of the aspects of master’s thesis supervision that have been studied are text negotiation and discipline (Dysthe Citation2002; Dysthe, Samara, and Westrheim Citation2006) and perceptions of feedback (de Kleijn et al. Citation2013, Citation2014). In a recent study, Harwood and Petrić (Citation2017) address supervisors’ and international students’ experience of the supervisory process in the UK. The study is set within the humanities and social sciences and highlights the considerable differences in approaches, attitudes and emotions that students and supervisors experienced and also the great variation in practices of individuals and policies of institutions. Harwood and Petrić (Citation2017) note that comparatively little work has been done on master’s thesis supervision, particularly on processes, which underlines the need for more research on issues such as what perceptions master’s students bring into the master’s thesis project.
An important aspect of master’s thesis writing is that, during the writing of the thesis, the negotiation of writing does not necessarily involve a writing expert. Instead, this is typically a negotiation between students and disciplinary experts. Research has shown that disciplinary experts may struggle to explicate their knowledge about writing (Blåsjö Citation2011; Geisler Citation1994; Jacobs Citation2007), and that there can be a discrepancy between how students perceive comments from disciplinary experts and the intentions of the disciplinary experts (Pelger and Sigrell Citation2016). For example, in a study of supervisors’ comments on 44 biology and molecular biology master’s theses from a major Swedish university, Pelger and Sigrell (Citation2016) show that while students perceived feedback as mainly addressing language and style, disciplinary experts intended to emphasise both content and discourse organisation. We therefore argue that it is necessary to know more about how students and supervisors perceive and talk about the writing of their disciplinary genres in order to isolate both writing and content-related challenges.
Teaching and learning of genre writing in science and engineering
One of the most common approaches to analysing and accounting for writing in a specific discipline is a moves-based analysis (Swales Citation1990; Swales and Feak Citation2012), which is referred to as a ‘genre approach’ in this study. Such an analysis investigates and describes what communicative functions are used by writers in a particular genre. In the analysis, texts are divided up into sections on the basis of what communicative function (or move) the section performs. This type of research stems from Swales’ (Citation1990) work on the introduction section of scientific journal articles. In this work, Swales identified three main moves in introductions across disciplines: (1) establish a territory, (2) establish a niche and (3) occupy the niche. To exemplify how these functions are represented in text, the second move often involves the authors identifying what type of research is missing or incomplete within the discipline and, in the third move, the authors introduce their own research and how it, in one way or another, addresses what is regarded to be missing. As an extension of Swales’ work, numerous scholars have developed discipline-specific moves analyses for various disciplines, for example computer science (Posteguillo Citation1999), biochemistry (Kanoksilapatham Citation2005) and mathematics (Kuteeva and McGrath Citation2015). Although these studies do not aim specifically at pedagogical application, the pedagogical value of the developed framework tends to be stressed.
Genre analyses inform numerous academic writing courses in higher education. Often, such courses are run by writing experts, but there are also examples of how genre analyses are used in projects involving collaboration between writing experts and disciplinary specialists. One of the best examples of such an approach is the Write like a chemist project by Stoller, Robinson and colleagues (Robinson and Stoller Citation2007; Robinson, Stoller, and Jones Citation2008; Robinson et al. Citation2008; Stoller et al. Citation2005, Citation2006; Stoller and Robinson Citation2013). The key task of this project was to develop moves structures for a number of academic genres in chemistry (journal articles, abstracts, proposals and posters) (Stoller and Robinson Citation2013, 46) and use this structure to develop pedagogical materials (Robinson et al. Citation2008).
The Write like a chemist project is of particular interest for the present study because it involves analyses of results sections in journal articles in chemistry (Stoller and Robinson Citation2013). However, the moves and sub-moves structure was primarily developed to give students a good overview and starting point and did therefore not account for all variation between subfields of chemistry (Stoller and Robinson Citation2013, 48–50). In a study from 2015, we presented a slightly more detailed moves structure model of data commentaries in results sections of journal articles and master’s theses in chemical engineering (Nordrum and Eriksson Citation2015), and this structure informed the workshop on data commentary reported on in this study (see the section Workshop design for details).
Method and material
This study is based on interviews with master’s students and their supervisors collected before and after a workshop about data commentary writing for master’s students in chemical engineering. The workshop was given by the authors. Here, we first provide details about the participants and the timing of the workshop, next we explain the interview procedure for student as well as supervisor interviews, then we give an overview of the workshop design, and finally we present the data analysis.
Participants
The students participating in this study were master’s students of chemical engineering who were in the process of finishing their master’s thesis at a Swedish research university. Most students were completing their last term on a five-year programme towards a degree of Master of Science of Engineering, Chemical Engineering, while some had studied the first three years at other universities and were working towards a Master of Science. Studies in chemical engineering typically involve topics such as the modelling and design of chemical processes, fluid mechanics and heat transfer. In the context investigated, there is often also some overlap between chemical engineering and applied chemistry, as students for instance study analytical chemistry. After completion of the master’s programme, students can take up a career within academia or within industry.
The students were recruited through an invitation to master’s students of chemical engineering to attend a workshop on academic writing with a focus on data commentary. The invitation was sent by one of the chemical engineering supervisors to students writing their master’s thesis at the department of chemical engineering during the spring of 2015 and the spring of 2016. The student data were collected on two separate occasions: one workshop was delivered in 2015 with seven students attending, and one in 2016 with four students attending. Nine of these eleven participants could attend all activities (the workshop + three interviews, see the section Interview procedures) and were included in the study.Footnote1 Because students were invited to participate, the sample of students is not necessarily a representative sample of the student cohort. Nevertheless, the students who participated belong to the group of students we wanted to approach, and the student sample is therefore well suited for an exploratory study. In 2015, 25 students were registered as master’s thesis students of applied chemistry, and in 2016, 31 students were registered. Some of these students did their master’s thesis abroad or in other cities, and it is therefore not fully clear exactly how many students could potentially have attended the workshops.
According to a background record filled in prior to the workshop, the students had experience of writing in their discipline, primarily through lab reports and their bachelor’s thesis, but they had not had any specific training in disciplinary writing via technical communication courses or communication modules embedded in engineering courses (also known as Integrating Content and Language (ICL) (Gustafsson and Jacobs Citation2013). All students were non-native speakers of English, and the context is what is sometimes referred to as an English-medium instruction (EMI) context. It is worth pointing out, as do Harwood and Petrić (Citation2017, 2), that language is only one characteristic that distinguishes the students in this type of educational context. We are aware that features such as language and educational background obviously affect students’ experience of their current education, but we have not divided our cohort into subgroups but focus on the perceptions represented in the context investigated.
In addition to the students, five supervisors were interviewed. These were all supervisors of at least one of the students participating in the workshop. The supervisors had varying degrees of experience, with one supervisor still being a PhD student while the four others were senior researchers. None of the supervisors interviewed had English as their first language.
Interview procedures
The study is based on interview data because interviews are useful for the collection of descriptions of people’s experience of particular situations or activities (Kvale Citation2007, 10–11). Two student interviews were carried out on the day of the workshop, one before and one after the workshop. The second interview primarily focused on what the students had worked on and changed during the workshop. A third student interview was carried out a few weeks after the workshop. The supervisor interviews were conducted after the students had completed the third interview and had handed in the final draft of their master’s thesis. In this paper, we use the supervisor interviews and two of the student interviews: the pre-workshop interview (referred to as interview 1) and the third student interview (referred to as interview 3). These interviews were used because they were exploratory and contained questions relevant for the research questions investigated here. All participants gave their consent to participating in the study.
All interviews were divided into two parts: a generic, semi-structured part and a semi-structured, discourse-based part (Appendix A Appendix B–C) (Moore and Morton Citation2017; Odell, Goswami, and Herrington Citation1983). The generic part was primarily used to collect the participants’ general perception of what is difficult and important about data commentaries. These questions therefore primarily addressed the first two research questions, but the information about what the students and supervisors find difficult and important can obviously also have consequences for the pedagogical strategies suggested. The discourse-based part was used to collect text-based examples of what the challenges of writing data commentaries may be. Discourse-based interviews are interviews based on talk around a written artefact. Odell, Goswami, and Herrington (Citation1983, 226) explain that one of the benefits of a discourse-based approach is that it can ‘tell us about the tacit knowledge that they [the writers, in our case also the supervisors] bring to writing tasks’. Since we wanted to understand students’ and supervisors’ perceptions of data commentaries and the challenges of writing data commentaries, the discourse-based parts were considered a good complement to the semi-structured parts, particularly when it comes to understanding what supervisors see as essential when writing data commentaries. As pointed out in the introduction, content supervisors may have problems explicating their knowledge about writing, and the discourse-based interviews therefore gave additional opportunities to find out how supervisors reason about text. In addition, the text-based part made it possible to compare how students and supervisors talked about the same piece of text.
For the discourse-based part of the student interviews, the students were asked to bring drafts of one or two figures or tables and their accompanying data commentaries from their ongoing thesis work. On the basis of a few questions, the students were then asked to comment on the drafts, for instance about what they wanted to convey with the figure, what the most important points were and who they considered to be their reader (see Appendix A and B). In the discourse-based supervisor interviews, the supervisors commented on one or two data commentaries written by a student they had supervised. These text excerpts were brought by the interviewer. The supervisors were asked to elaborate on matters such as whether or not the combination of figure and data commentary worked, and, if it did not, what they would like to change (Appendix C). The supervisor interviews were carried out after the students had handed in their final drafts and defended their thesis and did not affect the supervision or grading of the text. The text excerpts were however not taken from the final draft but from the draft that the students brought to the follow-up interview a few weeks after the data commentary workshop.
The first set of student interviews lasted between 9 and 16 min and the second set between 8 and 38 min. The reason for the vastly different lengths of the second interview was that some students had a lot more to say about data commentary than others, but also that there was significant variation in the level of detail with which they described their own commentaries.
The supervisor interviews were between 22 and 40 min long. The difference in length was partly due to one interview being considerably longer than the other interviews (the second longest supervisor interview was 31 min) since the supervisor in this interview had supervised two students and the discourse-based part therefore involved discussions of two data commentaries.
The great majority of the interviews were carried out by the authors (13 out of 18 student interviews and all the supervisor interviews), but in the 2015 run of the workshop, two senior researchers with extensive experience of performing interview studies were brought in to speed up the pre-workshop interview process and to avoid students having to wait for a very long time before the workshop could start. Three of the seven pre-workshop interviews (interview 1) were done by these two researchers. These researchers were not writing experts, and it is possible that this affected the results of the interviews, particularly the discourse-based parts, but our analyses have not indicated that the students gave different answers in these interviews. In addition, two of the post-workshop interviews (interview 3) in 2015 were conducted by another senior researcher with expertise in writing.
Workshop design
The four-hour workshop consisted of three main parts, of which the first part was the longest. Here, the students were introduced to the model of rhetorical moves developed for results figures and tables within applied chemistry and to the UAM corpus tool (O’Donnell Citation2009), which was used for text analysis in the workshop (for a more detailed account of the model and the corpus tool, see Nordrum and Eriksson Citation2015). As shown in , the three main moves of the model are background, presentation of visual and comment on result. These moves then contain several submoves or steps (Swales and Feak Citation2012, 331). It is not necessary to use all moves and submoves in single data commentary. For example, many data commentaries do not include a background move, and if they do, only one of the sub-moves procedure-method, disciplinary-knowledge or comment-on-choice-of-presentation is usually present. In the workshop, the students were given an overview of the moves model, and a few examples were analysed and colour-coded by means of the UAM corpus tool in order to show how moves can be distributed in a particular data commentary.
Figure 1. A moves model of data commentary on result-reporting visuals in chemical engineering. The moves should be read from left to right. An example of a data commentary from a master’s thesis in chemical engineering could be a background move realised by submove 1a) reminding the reader of how the data presented in the visual was obtained, followed by a presentation-of-visual move realised by submove 3a) providing a reference to the figure and the main result (e.g. Figure 1 shows that there is an increase in (…)), and last a comment-on-result move realised by submove 3d) giving a cross-reference to where in the master’s thesis the result in the visual is discussed (e.g. This increase will be further discussed in Section 4.2).
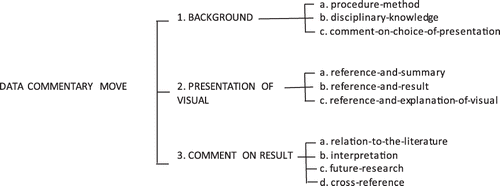
The second part of the workshop highlighted the move presentation of visual. The students analysed different ways of introducing a figure in the text (e.g. shows something vs. something is shown in ) and compared published journal articles and master’s theses.
The third and final part was relatively short. It involved students’ revisions of data commentaries from their own theses, which they had been asked to bring to the workshop.
Data analysis
The interviews were recorded and transcribed verbatim and then analysed by means of two main procedures.Footnote2 First, the responses from the generic part of the interviews (student interview 1 and 3 and the supervisor interview) about what is difficult and important were sorted into a construct table (Miles, Huberman, and Saldaña Citation2014, 171). This procedure was used to address the first two research questions. Construct tables are suitable for investigations of specific variables, in this case ‘difficult’ and ‘important’, and can be used to provide an overview of perceptions expressed by the students and the supervisors. Author 1 developed the initial codes of the construct table and then both authors refined the table iteratively and finalised the coding. The coding was performed manually directly in the transcribed text files. Data from the discourse-based parts of the interviews were later added to the construct tables provided that the comments were general comments rather than comments about specific passages of a text.
Second, the transcripts of the discourse-based interviews were annotated for topic themes by means of open coding as described by Miles, Huberman, and Saldaña (Citation2014). This analysis primarily aimed at addressing the third research question, and provisional topic themes and orientations around challenges of pedagogical concern were therefore developed. For example, passages in which the supervisors commented on content-related misunderstandings or mistakes were noted down as ‘disciplinary content mistakes’. These codes were then refined as both authors independently went through the transcriptions to find recurring themes. Finally, a process of moderation was used to arrive at a consensual analysis.
Results
The presentation of results is divided into two main parts. In the first part, we address the first two research questions: the students’ and the supervisors’ perceptions of what is difficult and what is important in data commentary. This account gives an overview of how the topics are perceived by the students and the supervisors. The second part presents pedagogical challenges of data commentary in the context investigated (research question 3). The results in this section are based on the discourse-based parts of the interviews. On the basis of analyses and comparisons of the student and supervisor interviews, themes of pedagogical challenges were developed from recurring challenges noticed in these conversations.
What is difficult and important
presents a thematic overview of the answers to what is difficult when commenting on result-reporting visuals in chemical engineering. The results have been collected from student interview 1 (pre-workshop), student interview 3 (post-workshop) and the supervisor interview. As can be seen, no themes were mentioned by all participants, which indicates a certain level of variability and complexity in terms of how students and supervisors experience the writing of data commentaries. In our qualitative analysis, two themes, selecting material and aspects of mechanics, emerged as salient both in terms of their distribution across students and supervisors and the comments made in connection with these themes.
Table 1. Students’ and supervisors’ perceptions of what is difficult about writing data commentaries (N students = 9, N supervisors = 5).
The comments on selecting material were of two types. The first type concerns selection of what and how much to write about a certain visual, as illustrated in the following student and supervisor comments:
Hmm it is a bit difficult. You are afraid that you write too much … so it becomes almost too clear. Don’t know if that is good. So it is probably knowledge … you, you think that someone else will understand directly, but you are afraid of writing too much.
yes it may be difficult to actually say everything I wanted to say because of course we don’t want the report to be extremely long, so I may have some difficulties keeping concise.
In my experience the writing is not difficult in itself, but selecting what to write. Too much and the wrong things. Beginning with describing what is already described in the visual rather than describing what is important.
The second type of selection difficulty concerns how to determine whether a result should be included among the results presented visually at all. This concern surfaced in one supervisor interview:
The thing about picking results is really difficult, really. Students have not yet developed the eye for knowing what a good result is.
The two categories of comments on selection support Poe, Lerner, and Craig’s (Citation2010) claim that selection is an important theme in the teaching and learning of data commentary writing and suggest that selection should be addressed from two angles: (1) selection of what to comment on after the visual has been chosen, and (2) selection of which results should be displayed visually and commented on.
A second observation from is that both the supervisors and the students mentioned issues of mechanics as being problematic. The supervisor comments concerned appropriate layout and language as shown by the following comments:
The most common mistake is that they do not have proper units, axes are missing. They have not reflected on how long the axes are. Cosmetics.
They have difficulties achieving a proper layout of the text.
In terms of the influence of the workshops, the data collected is not large enough to establish changes in student behaviour and understanding, but we note that some students explicitly stated that the workshop made them think more carefully about the type of information to include in a data commentary and how to organise the information:
Well, you think a lot more about things like, perhaps you try to start with some background information, if that is what you want, and then you try to put that first in a nice way and then you move to making a reference to the figure and then you try to be a bit more detailed.
Yes what do you mean by detailed?
That it is more clear [indicates steps] ‘now it is background, now I explain what you see, now I discuss’.
[talking about what to put in a data commentary] what first you sort of make a reference in the literature eh background description of the figure and then to describe what’s in the figure and the most obvious results. Especially the ones that you want to show to the readers. And then some sort of implications of the results.
presents a thematic overview of the answers to what the students and the supervisors considered important in data commentaries in chemical engineering. Just as we saw for ‘what is difficult’, there is a wide range of themes and limited reoccurrence between themes, indicating variability and complexity in the perception of what is important in writing data commentary among both the students and the supervisors. In the qualitative analysis, the theme clarity of explanation emerged as the most salient theme in the student and supervisor comments, both in terms of comments and distribution across the participants ().
Table 2. Students’ and supervisors’ perceptions of what is important about writing data commentaries (N students = 9, N supervisors = 5).
The qualitative analysis of ‘clarity of explanation’ showed that in interview 1, the students had difficulties in elaborating what clarity means and comments were primarily of the following type:
Ah, that you, like, to explain what you have achieved, you need to use the visual to make it more clear.
Well you want the reader to understand the figure as well, so that all parts are included.
and then like it is very important that you spend time on trying to explain it [the visual] so that someone else can understand what it is that you see in the figure and what it is that is important and perhaps also what is strange or deviant.
that you explain very carefully what the reader should see in the graph, what you want to show with the figure.
after the workshop I have been thinking that one should try to explain … the figure to a blind person, for example things that are fairly obvious … so that one does not go too deep into things but say what can be seen in the graph and things like that – this bar is higher for this – well something like that … what is the most important result in a graph with a lot of information, kind of.
The supervisors elaborated on the topic of clarity in different ways. For example, one supervisor underlined the importance and value of understanding the figure without too much context:
All figures should be independent. Understand without having to go back to the text too much. The figure should be self-explanatory together with the figure caption.
This is the way it looks and it looks this way because … present results in a clear way. Trends in data, accuracy of measurements. A combination of data presentation, a combination of designing a figure and writing a text.
The discourse-based interviews: challenges of detail and focus, content learning and metalanguage
Through analyses of the discourse-based interviews, challenges related to teaching and learning were identified. Three themes based on recurring challenges emerged from the analysis: (1) challenges of detail and focus, (2) challenges of content learning and (3) challenges of using a shared metalanguage for data commentaries. As can be seen, the themes are quite different in character. The first one is a matter of being precise and detailed enough in the data commentary. We see that the supervisors easily question aspects of detail and focus, and although the material is fairly small, it is quite clear that this is something that students struggle with. It therefore seems important to familiarise students with negotiating detail and focus in their data commentaries. The second challenge reminds us that writing and disciplinary knowledge are closely intertwined. As data commentaries are central parts of master’s theses in chemical engineering, some of the challenges concern disciplinary knowledge. Challenges of data commentaries do therefore not only pertain to layout, design and discourse organisation, but also involve disciplinary understanding. The third challenge is a challenge that the students and the supervisors seem to face when trying to talk about data commentaries. The lack of a shared metalanguage generates uncertainty and sometimes also misunderstandings when the students and the supervisors negotiate the data commentaries. The presentation below primarily contains examples from the supervisor interviews, but the themes partly emerged through comparisons of how the students and their supervisors talked about the same student texts.
Challenges of detail and focus
The discourse-based parts of the interviews added significant detail to what is difficult for students when writing data commentaries, particularly with regard to the category ‘selection of material’. In the discussion of actual texts, the discourse shifted from difficulties in knowing what information to select to statements about appropriate levels of detail in the data commentary. Typically, the gist of the comments was that a particular commentary was not necessarily inaccurate, but that important aspects shown in the graph were not sufficiently highlighted. Two categories of comments emerged:
Details connected with formal aspects of the graph. Comments of this type concerned issues such as missing axes or missing information in figure captions.
The focus and clarity of the data commentary. These comments concerned what the author wanted to say in the text, what should be highlighted and how it was expressed. This category also involved comments indicating that something was missing in the data commentary.
The second subcategory of detail and focus concerned the focus and clarity of the data commentary. The main problem here was that the student data commentaries did not always highlight the most important point from the perspective of the research story of the entire thesis. The supervisors stated that students can be more or less precise in their selection and sometimes choose an unfortunate storyline, either in terms of highlighting a less interesting result or leaving out important results:
Here he could have discussed to what extent this adjustment is correct … when is it well-adjusted and when is it not.
but now that we have discussed it so I think I would want to have something about … like I said about concentration, a comment about these exchanges and concentrations being high enough … since that is not quite clear
Another problem of focus and clarity concerns the order in which information was presented rather than lacking information. In these cases, the supervisors argued that certain passages should be moved or deleted, as illustrated in the following supervisor statement:
well, no there are like no … there are many other explanations as well that have been combined with this data commentary … that do not really belong there … that could be put in a paragraph after this.
Data commentary and challenges in the learning of disciplinary content
The second specific challenge that emerged in the discourse-based interviews was disciplinary understanding. We indexed the following types of comments as problems of disciplinary learning: (1) comments that concern students’ use (or misuse) of particular concepts, and (2) comments about students’ contradicting disciplinary knowledge. In some cases, it was difficult to know, both for us and for the supervisors, whether a mistake was due to the student not fully understanding the concept or process described or not being able to express a particular idea or interpretation. In order to avoid exaggerating a category that is not within our field of specialisation, uncertain cases were categorised as issues of detail and focus (see the section Challenges of detail and focus) rather than disciplinary learning.
Some mistakes in the student texts can be attributed to the misunderstanding of a particular concept. For example, one of the supervisors commented on a student’s use of ‘absolute pressure’ in the following data commentary:
By plotting Equation (1.4) over an interval of moisture content in the bark at constant temperature together with the absolute pressure at the same temperature. Figure (1.2), it can be seen that the pressure for the isotherm will increase above the pressure for pure water at higher moisture contents
but absolute pressure I must say is a bit confusing (Supervisor 3)
‘absolute’ is not a good word here really …’absolute’, I am not sure why he is using that … it is a vapour pressure it is … that is given by the equation that he has there … but ‘absolute pressure’, I am not sure why he would call it that (Supervisor 3)
The second example comes from a passage that the student and the supervisor had discussed between the workshop and the supervisor interview, and it is a passage that the student had to revise:
It looks as if the fouling ratio is very low … and from her definition of fouling ratio, this is correct, but her thinking is completely wrong so she had to change this.
The third example of the connection between data commentaries and problems related to disciplinary understanding comes from a student who discusses the relationship between a model and a result. The supervisor expressed concern about the student’s way of reasoning about the term ‘model’:
[reading from the text, indicated by quotation marks] ‘shows a simplified model’, which it does not really do. It shows results from a model.
So one of the problems here is that she presents something as a model but in reality it is a result?
Yes
Why do you think that she has written that this is a model rather than a result?
It is quite possible that she thinks that these are the same thing …
Mediating master’s thesis supervision of data commentaries: the value of a shared metalanguage
Although this study does not include direct student–supervisor interaction, the data indicate that students and supervisors partly lack a shared metalanguage for data commentaries, which may be a problem for communication and thus potentially for learning. One example of this being an issue is the supervisors’ use of generic or metaphorical language when discussing the student texts. For instance, one of the supervisors talked about ‘additional information’ when referring to a sentence that both introduces the visual element in the text and includes a statement that summarises the content of the data commentary. Similarly, this supervisor talked about the need for a ‘bow’ to combine sentences or sections in a text, which we understood as a way of describing how a sentence or a section typically starts with information already known from the context (old-to-new information flow, Swales and Feak Citation2012, 31). A third example was a supervisor who talked about there being a need for ‘putting more emphasis on something’ without necessarily saying that this means that something should be highlighted in terms of where it is positioned in the rhetorical structure of the data commentary. Comments of this type can be more or less difficult for students to interpret and it is likely that students’ abilities to address such comments differ.
The above examples illustrate language that is potentially challenging for students, but there are also a couple of examples that illustrate how insufficient metalanguage made it difficult for the students and the supervisors to adjust aspects of the data commentary in their discussions. The first example is from a student who followed the advice from the supervisor that she ‘should start with what is most important’ (Student 6). This is a phrase that both the student and the supervisor used in their respective interviews. What the supervisor really meant was that the student should avoid rendering the chronological structure of the research process when presenting the study in the master’s thesis. The student, however, interpreted ‘start with what is most important’ as describing also how data commentaries should be written. The effect of this advice was that the student followed it literally and presented the main result of the figure before even introducing the figure in the text, as can be seen in the following excerpt from the thesis draft:
An experiment was performed with fresh alkali extraction. Initially, when the retentate flow pipe was below the surface of liquor in the feed tank, was the flux constant at 70 L/m2 h. When the pipe was lifted above the surface of the solution in the feed tank, flux increased with time, as shown in Figure 4.6.
A final example comes from a student who in the interview and in the data commentary devoted a lot of attention to explaining unexpected results or results indicating that something in the experiment had gone wrong. This example suggests that the student had some problems understanding what to highlight in the data commentary. Comparisons of the student and the supervisor interviews and analyses of the student’s texts show that the student did in fact put too much focus on the unexpected results and did not comment on the main results of his study. In the third interview, the student asked the interviewer: ‘Do I have to comment on what is obvious, which is as expected?’ (Student 5). Here the point is that what is expected is also the student’s main result: the visual that the student comments on shows that the student’s method is acceptable, and this fact should be the first result commented. However, in the data commentary draft that the student brought to the interview, only the unexpected results were addressed. In a discussion of the same passage in the supervisor interview, the supervisor stated that the main result should be commented, and when asked about whether or not the student may think that the unexpected result is the most important result, he explained:
Well, he may think that it is [the most important thing] in the sense that this is not what it is usually like.
All in all, our analyses indicate that students struggle to find appropriate levels of detail in their data commentary, that students make content mistakes that can be identified through detailed reading, and that students and supervisors do not share a metalanguage to talk about data commentary at appropriate levels. To us these results give important information about what to consider when addressing data commentary in teaching and learning. We therefore synthesise these findings into measures in the discussion section.
Discussion
This study investigates what students and supervisors of master’s theses in chemical engineering find difficult and important when working with students’ data commentaries, and also what pedagogical challenges are pertinent to data commentaries. The study aligns with previous work on the teaching and learning of data commentaries (Poe, Lerner, and Craig Citation2010), and adds additional perspectives on data commentaries by looking primarily at student’s and supervisor’s perceptions of what happens or is supposed to happen in a data commentary rather than the textual aspects of the commentary (Sancho Guinda Citation2012; Stoller and Robinson Citation2013). The perceptions are important for understanding the teaching and learning context and for developing informed advice about important foci for the supervision of data commentary writing. We argue that such advice is of particular importance for students in disciplines in which data commentaries have a structuring role in the results section.
The results from the generic, semi-structured parts of the interviews show that the students and the supervisors bring up a wide range of themes as being difficult and important, which indicates that data commentary is not a topic that can be handled with a few simple guidelines. Many of the comments in this part of the interview concern selection of content and clarity. The students particularly seemed to struggle with what to comment on and how much to comment. The discourse-based parts of the interviews helped refine some of the themes mentioned in the semi-structured part. For instance, particularly the supervisor interviews stressed that the students did not express key points with necessary detail and focus and did not structure their commentaries in such a way that key results were appropriately highlighted.
In terms of understanding and responding to the challenges of detail and focus, there are different approaches. One of them is to see the challenges primarily as signs of the students being inexperienced researchers and scientific writers. This type of understanding was expressed by two supervisors, who emphasised that their students would probably develop a more precise writing style as they mature academically. The response may then be that students simply need more time and that they will eventually learn this if they pursue an academic career. Based on the findings of our study, however, we believe that there are at least a couple of dangers of such an approach. First of all, students’ writing processes may become unnecessarily complicated if they are not familiarised with central aspects of writing in their discipline. Secondly, disciplinary writing is closely connected with disciplinary understanding, and imprecise writing may therefore be due to the lack of understanding of disciplinary content rather than limited writing experience or insufficient language proficiency. We therefore believe that the challenges students face in their data commentary should be highlighted to unpack disciplinary conventions and disciplinary understanding, following Bernstein’s (Citation1990) advice of applying a ‘visible pedagogy’ (see also Johns et al. (Citation2006)).
In addition to challenges connected to detail and focus, the discourse-based interviews revealed that the teaching and learning of data commentary also involve challenges related to content learning and metalanguage. These findings give guidance to what type of support can be provided and how it can be organised. Specifically, we suggest three strategies to improve supervision of data commentary in the context investigated: developing a shared metalanguage, using a genre approach, and basing efforts on collaboration between content supervisors and academic writing experts. Working towards a shared metalanguage is a means to increase mutual understanding between supervisors and students. In our study, the problem of not having or not sharing a metalanguage is shown in the supervisors’ frequent use of generic or metaphorical language to describe student texts, and in the difficulties that some students faced when trying to interpret these metaphors. The problem is also indicated by the fact that the supervisors mean very different things when talking about what on the surface seems to be the same thing, for instance clarity.
The second measure, using a genre approach, has potential pedagogical value to master’s thesis writing in general and to data commentaries specifically. A first indication of this is that we saw that the students had problems elaborating on the issue of ‘clarity of explanation’ in the pre-workshop interview (interview 1), but that their comments were more explicit in the post-workshop interviews (interview 3). We also believe that the structured and focused analyses of data commentary provided in the genre approach can be useful in supervision, even if such correlations cannot be established in this study but would require further research. In our discussion with the supervisors, we found that genre analyses were partly new to the supervisors, but that the discourse-based interviews made them see perspectives of or details in the texts that they had not thought about before. The pedagogical usefulness of a genre approach has also been demonstrated by Stoller and Robinson (Citation2013) in their Write like a chemist project.
Regarding the third measure, basing pedagogical effort on collaboration between content supervisors and academic writing experts, our study gives clear examples of the potential contribution of content supervisors and writing experts in such a collaboration. The writing expert can support in the identification of functions of the genre via genre conventions and negotiate useful metalanguage with supervisors. Supervisors have the necessary content knowledge to make sure that the discipline-specific challenges are not missed, which may easily happen in stand-alone, general academic writing courses. Here, we particularly saw one example of a data commentary that looked perfectly acceptable from the perspective of language, structure and genre conventions but which included misunderstandings of vital disciplinary knowledge. This misunderstanding was not discovered until a carefully scaffolded reading of the data commentary was made in collaboration between the writing and the content experts. The collaboration therefore has potential to unpack aspects of writing and learning and make them accessible to students in ways which they would not have been unpacked if the writing and content experts had worked independently (Jacobs Citation2010). In addition, the collaboration can offer a space in which participants can share supervisory experience and develop supervisory practices. Harwood and Petrić (Citation2017, 197) stress the importance of signalling the importance of supervision within a department to avoid the practice merely becoming an act of fulfilling prescriptive duties.
The forms of a content supervisor and writing expert collaboration need to be adapted to meet local conditions and resources available. It may for example take the form of faculty training as described by Anson et al. (Citation2012) or involve joint contributions from content supervisors and writing experts through collaborative interaction or collaborative teaching, as discussed for instance by Jacobs (Citation2007) and Gustafsson and Jacobs (Citation2013). Another approach that has proven to be effective for both students and faculty is the use of assessment protocols to train students in how to engage in scientific review processes (Dowd et al. Citation2015; Reynolds and Thompson Citation2011).
Finally, although our results are not immediately generalisable or transferable to other contexts, we see clear overlaps between our observations and similar types of studies. For example, Poe, Lerner, and Craig (Citation2010), in a different context (L1, undergraduate), argue that not enough attention is given to the selection of content in writing pedagogy and several studies discuss the difficulty for content specialists to unpack or articulate aspects of disciplinary discourse, which makes it difficult for students to learn these aspects (Geisler Citation1994; Jacobs Citation2007; Pelger and Sigrell Citation2016). We therefore believe that our findings are relevant for other scholars working in highly specialised, disciplinary contexts. We particularly hope that our study can promote more studies that approach disciplinary writing both from the perspective of rhetorical functions in disciplinary language as well as how writing development is connected to disciplinary ways of thinking.
Disclosure statement
No potential conflict of interest was reported by the authors.
Acknowledgements
We are very grateful to senior lecturers Anders Ahlberg and Torgny Roxå for their help in interviewing students, to lecturer Jennifer Löfgreen for assistance in material development for the workshop and for interviewing students, and to associate professor Magnus Gustafsson for being involved in the preparation of interview questions. We are also very grateful to the two anonymous reviewers for their helpful comments on an earlier version of this article.
Notes
1. Two students who attended the workshops could not participate in the third interview and were therefore not included in this study (see the section Interview procedures).
2. The extracts from interviews in the paper are generally verbatim transcriptions but false starts and hesitations such as ‘erm’ have been removed in order to increase readability. For the same reason, fillers like ‘mm’ and ‘yeah’ from the interviewer have been deleted in a few of the extracts. Pauses are indicated by ‘…’.
References
- Anson, C. M., D. P. Dannels, P. Flash, and A. L. Housley Gaffney. 2012. “Big Rubrics and Weird Genres: The Futility of Using Generic Assessment Tools across Diverse Instructional Contexts.” The Journal of Writing Assessment 5 (1).
- Bernstein, B. 1990. Vol. 4 of Class, Codes and Control. London: Routledge.10.4324/9780203011263
- Blåsjö, M. 2011. “From Percentage to Prediction: University Students Meeting a Parallel Language of Visuals and Numerals.” Ibérica 22: 123–140.
- Curry, M. J. 2014. “Graphics and Invention in Academic Engineers’ Writing for Publication.” In Language, Literacy, and Learning in STEM Education: Research Methods and Perspectives from Applied Linguistics, edited by M. J. Curry and D. I. Hanauer, 87–106. Amsterdam/Philadelphia: John Benjamins.10.1075/lsse
- Dowd, J. E., C. P. Roy, R. J. Thompson Jr, and J. A. Reynolds. 2015. “‘On Course’ for Supporting Expanded Participation and Improving Scientific Reasoning in Undergraduate Thesis Writing.” Journal of Chemical Education 92 (1): 39–45.10.1021/ed500298r
- Dysthe, O. 2002. “Professors as Mediators of Academic Text Cultures: An Interview Study with Advisors and Master’s Degree Students in Three Disciplines in a Norwegian University.” Written Communication 19 (4): 493–544.10.1177/074108802238010
- Dysthe, O., A. Samara, and K. Westrheim. 2006. “Multivoiced Supervision of Master’s Students: A Case Study of Alternative Supervision Practices in Higher Education.” Studies in Higher Education 31 (3): 299–318.10.1080/03075070600680562
- Geisler, C. 1994. “Literacy and Expertise in the Academy.” Language and Learning across the Disciplines 1: 35–57.
- Gustafsson, M., and C. Jacobs. 2013. “Editorial: Student Learning and ICLHE–Frameworks and Contexts.” Journal of Academic Writing 3 (1).
- Harwood, N., and B. Petrić. 2017. Vol. 25 of Experiencing Master’s Supervision: Perspectives of International Students and Their Supervisors. London/New York: Routledge.
- Jacobs, C. 2007. “Towards a Critical Understanding of the Teaching of Discipline-Specific Academic Literacies: Making the Tacit Explicit.” (Kenton Special Issue). Journal of Education 41: 59–81.
- Jacobs, C. 2010. “Collaboration as Pedagogy: Consequences and Implications for Partnerships between Communication and Disciplinary Specialists.” Southern African Linguistics and Applied Language Studies 28 (3): 227–237.10.2989/16073614.2010.545025
- Johns, A. M., A. Bawarshi, R. M. Coe, K. Hyland, B. Paltridge, M. J. Reiff, and C. Tardy. 2006. “Crossing the Boundaries of Genre Studies: Commentaries by Experts.” Journal of Second Language Writing 15 (3): 234–249.10.1016/j.jslw.2006.09.001
- Kanoksilapatham, B. 2005. “Rhetorical Structure of Biochemistry Research Articles.” English for Specific Purposes 24 (3): 269–292.10.1016/j.esp.2004.08.003
- de Kleijn, R. A., M. T. Mainhard, P. C. Meijer, M. Brekelmans, and A. Pilot. 2013. “Master’s Thesis Projects: Student Perceptions of Supervisor Feedback.” Assessment & Evaluation in Higher Education 38 (8): 1012–1026.10.1080/02602938.2013.777690
- de Kleijn, R. A., P. C. Meijer, A. Pilot, and M. Brekelmans. 2014. “The Relation between Feedback Perceptions and the Supervisor–Student Relationship in Master’s Thesis Projects.” Teaching in Higher Education 19 (4): 336–349.10.1080/13562517.2013.860109
- Kuteeva, M., and L. McGrath. 2015. “The Theoretical Research Article as a Reflection of Disciplinary Practices: The Case of Pure Mathematics.” Applied Linguistics 36 (2): 215–235.10.1093/applin/amt042
- Kvale, S. 2007. Doing Interviews. London: Sage.10.4135/9781849208963
- Miles, M. B., A. M. Huberman, and J. Saldaña. 2014. Qualitative Data Analysis: A Methods Sourcebook. 3rd ed. Thousand Oaks, CA: Sage.
- Moore, T., and J. Morton. 2017. “The Myth of Job Readiness? Written Communication, Employability, and the ‘Skills Gap’ in Higher Education.” Studies in Higher Education 42 (3): 591–609.10.1080/03075079.2015.1067602
- Nixon, R. S., T. J. Godfrey, N. T. Mayhew, and C. C. Wiegert. 2016. “Undergraduate Student Construction and Interpretation of Graphs in Physics Lab Activities.” Physical Review Physics Education Research 12 (1): 010104.10.1103/PhysRevPhysEducRes.12.010104
- Nordrum, L., and A. Eriksson. 2015. “Data Commentary in Science Writing: Using a Small, Specialized Corpus for Formative Self-Assessment Practices.” In Learner Corpora in Language Testing and Assessment, edited by M. Callies and S. Götz, 59–83. Amsterdam: John Benjamins.
- O’Donnell, M. 2009. “The UAM Corpus Tool: Software for Corpus Annotation and Exploration.” In Applied Linguistics Now: Understanding Language and Mind [La lingüística aplicada hoy: Comprendiendo el lenguaje y la mente], edited by C. M Bretones Callejas, J. F. Fernández Sánchez, J. R. Ibáñez Ibáñez, M. E. García Sánchez, M. E. Cortés de los Ríos, M. S. Salaberri Ramiro, M. S. Cruz Marines, N. Perdu Honeyman, and B. Cantizano Márquez, 1433–1447. Almería: Universidad de Almería.
- Odell, L., D. Goswami, and A. Herrington. 1983. “The Discourse-Based Interview: A Procedure for Exploring the Tacit Knowledge of Writers in Non-Academic Settings.” In Research on Writing: Principles and Methods, edited by P. Mosenthal, L. Tamor and S. Walmsley, 221–236. New York: Longman.
- Pelger, S., and A. Sigrell. 2016. “Rhetorical Meta-Language to Promote the Development of Students’ Writing Skills and Subject Matter Understanding.” Research in Science & Technological Education 34 (1): 15–42.
- Poe, M., N. Lerner, and J. Craig. 2010. Learning to Communicate in Science and Engineering: Case Studies from MIT. Cambridge, MA: MIT Press.
- Posteguillo, S. 1999. “The Schematic Structure of Computer Science Research Articles.” English for Specific Purposes 18 (2): 139–160.10.1016/S0889-4906(98)00001-5
- Reynolds, J. A., C. Thaiss, W. Katkin, and R. J. Thompson. 2012. “Writing-to-Learn in Undergraduate Science Education: A Community-Based, Conceptually Driven Approach.” CBE-Life Sciences Education 11 (1): 17–25.10.1187/cbe.11-08-0064
- Reynolds, J. A., and R. J. Thompson. 2011. “Want to Improve Undergraduate Thesis Writing? Engage Students and Their Faculty Readers in Scientific Peer Review.” CBE-Life Sciences Education 10 (2): 209–215.10.1187/cbe.10-10-0127
- Robinson, M. S., and F. L. Stoller. 2007. “A Read–Analyze–Write Approach to Research-Related Literacy Skills for Upper-Division Chemistry Majors.” In Designing, Implementing, and Sustaining a Research-Supportive Undergraduate Curriculum: A Compendium of Successful Curricular Practices from Faculty and Institutions Engaged in Undergraduate Research, edited by K. K. Katukstis and T. Elgren, 175–190. Washington, DC: Council on Undergraduate Research.
- Robinson, M. S., F. L. Stoller, M. S. Costanza-Robinson, and J. K. Jones. 2008. Write like a Chemist. A Guide and Resource. New York/Oxford: Oxford University Press.
- Robinson, M. S., F. L. Stoller, and J. K. Jones. 2008. “Using the ACS Journals Search to Validate Assumptions about Writing in Chemistry and Improve Chemistry Writing Instruction.” Journal of Chemical Education 85 (5): 650–654.10.1021/ed085p650
- Sancho Guinda, C. 2012. “Variation in Students’ Accounts of Graphic Data: Context and Context Factors in a Polytechnic Setting.” In Insights into Academic Genres, edited by C. Berkenkotter, V. K. Bhatia and M. Gotti, 355–376. Bern: Peter Lang.
- Stoller, F. L., B. Horn, W. Grabe, and M. S. Robinson. 2005. “Creating and Validating Assessment Instruments for a Discipline-Specific Writing Course: An Interdisciplinary Approach.” Journal of Applied Linguistics 2 (1): 75–104.
- Stoller, F. L., B. Horn, W. Grabe, and M. S. Robinson. 2006. “Evaluative Review in Materials Development.” Journal of English for Academic Purposes 5 (3): 174–192.10.1016/j.jeap.2006.07.003
- Stoller, F. L., and M. S. Robinson. 2013. “Chemistry Journal Articles: An Interdisciplinary Approach to Move Analysis with Pedagogical Aims.” English for Specific Purposes 32 (1): 45–57.10.1016/j.esp.2012.09.001
- Swales, J. M. 1990. Genre Analysis. English in Academic and Research Settings. Cambridge: Cambridge University Press.
- Swales, J. M., and C. B, Feak. 2012. Academic Writing for Graduate Students. Essential Tasks and Skill. 3rd ed. Ann Arbor: University of Michigan Press.10.3998/mpub.2173936
- Wolfe, J. 2015. “Teaching Students to Focus on the Data in Data Visualization.” Journal of Business and Technical Communication 29 (3): 344–359.10.1177/1050651915573944
Appendix A.
Interview questions used in student interview 1
General questions
1. Can you briefly describe your master’s thesis topic?
2. What do you think is important when commenting on figures that report on results in master’s theses in your field of study?
3. What might be difficult about commenting on figures?
Discourse-based part
4. Why did you choose this figure and text today?
5. What did you think about when you wrote the text?
6. Who do you consider to be your reader (in the master’s thesis)?
7. What do you think the person you consider to be your reader sees in the figure you brought with you today; and what do you think he or she needs to know to understand the figure?
Appendix B.
Interview questions used in student interview 2
General questions
1. What do you think is important when commenting on data in figures that report on results in your field of study?
2. Have you worked on the result part of your thesis since we last met?
3. Have you worked with result-reporting visuals (figures and tables) in the result part? What did you try to think about when you commented on the visuals?
Follow-up question: Have you approached the commenting in the same way for all figures?
4. Was something difficult when you commented on your figures?
Discourse-based part
5. Why did you choose this figure and text today?
6. What did you think about when you wrote the text?
7. Who did you consider to be your reader?
8. What do you think the person you considered to be your reader sees; and what do you think he or she needs to know?
Appendix C.
Interview questions used in supervisor interviews
General questions
1. What do you think is important when commenting on data in figures that report on results in your field of study?
Follow-up: Is there a difference between research articles and master’s theses in this respect?
2. What might be difficult about commenting on figures?
Follow up: For you? For students?
Discourse-based part
3. In this figure (which is taken from your student’s master’s thesis): What do you see (and what might a master student see?), and what do you think is necessary to know?
4. Do you think that the text that accompanies this figure works?
Follow up: Why/Why not?
5. Would you like to see some changes? If so, what type of changes would you like to see?
Follow up/specification: Should anything be taken out or added to the commentary? Would you like to see something phrased differently?