ABSTRACT
Background
Scientific thinking is an essential learning goal of science education and it can be fostered by inquiry learning. One important prerequisite for scientific thinking is procedural understanding. Procedural understanding is the knowledge about specific steps in scientific inquiry (e.g. formulating hypotheses, measuring dependent and varying independent variables, repeating measurements), and why they are essential (regarding objectivity, reliability, and validity). We present two studies exploring students’ ideas about procedural understanding in scientific inquiry using Concept Cartoons. Concept Cartoons are cartoon-like drawings of different characters who have different views about a concept. They are to activate students’ ideas about the specific concept and/or make them discuss them.
Purpose
The purpose of this paper is to survey students’ ideas of procedural understanding and identify core ideas of procedural understanding that are central for understanding scientific inquiry.
Design and methods
In the first study, we asked 47 students about reasons for different steps in inquiry work via an open–ended questionnaire using eight Concept Cartoons as triggers (e.g. about the question why one would need hypotheses). The qualitative analysis of answers revealed 42 ideas of procedural understanding (3-8 per Cartoon). We used these ideas to formulate a closed-ended questionnaire that contained the same Concept Cartoons, followed by statements with Likert-scales to measure agreement. In a second study, 64 students answered the second questionnaire as well as a multiple-choice test on procedural understanding.
Results
Using methods from educational data mining, we identified five central statements, all emphasizing the concept of confounding variables: (1) One needs alternative hypotheses, because there may be other variables worth considering as cause. (2) The planning helps to take into account confounding variables or external circumstances. (3) Confounding variables should be controlled since they influence the experiment/the dependent variable. (4) Confounding variables should be controlled since the omission may lead to inconclusive results. (5) Confounding variables should be controlled to ensure accurate measurement.
Conclusions
We discuss these ideas in terms of functioning as core ideas of procedural understanding. We hypothesize that these core-ideas could facilitate the teaching and learning of procedural understanding about experiments, which should be investigated in further studies.
Scientific thinking: The interplay of different knowledge-types
Scientific thinking is the competence to solve scientific problems (Mayer Citation2007). It can be divided into sub-competencies (e.g. formulating hypotheses or designing experiments), which in turn cover various competence aspects (). Scientific thinking is influenced by different types of knowledge on a procedural and declarative level (Chen and Klahr Citation1999; Gott and Duggan Citation1995; Roberts and Gott Citation2003; Mayer Citation2007). Before we look at these different types of knowledge in scientific thinking, it is useful to discuss procedural and declarative knowledge in general problem solving.
Figure 1. Scientific thinking and its sub-competences, competence-aspects as well as influencing factors (Arnold et al. Citation2018; Mayer Citation2007).
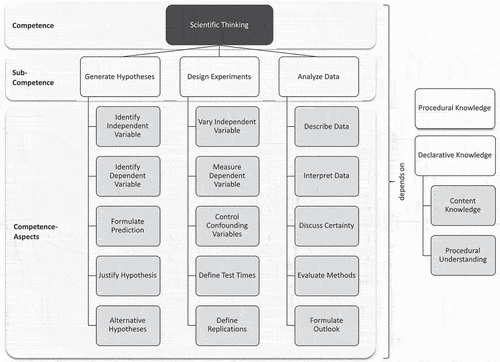
Procedural and declarative knowledge
Psychological models distinguish between non-declarative (procedural) and declarative content in long-term memory. To distinguish between procedural and declarative knowledge, different (partly overlapping) models exist, each using different pairs of terms. In this work, we use the terms procedural and declarative knowledge analogically to Arnold (Citation2015).
Non-declarative knowledge includes, among other things, implicit knowledge of rules, as well as procedural knowledge, whereby the latter relates to motor and cognitive skills. Procedural knowledge is knowledge of how to perform something. Accordingly, it is also called the ‘know how’ (e.g. Baroody Citation2003; Byrnes and Wasik Citation1991; Hiebert and Lefevre Citation1986). Hence, procedural knowledge is understood to be the implicit or explicit knowledge of rules and sequences of action (Star and Newton Citation2009), which forms the basis for the ability to act (action competence) and thus to solve problems. Here automation is seen as a quality feature rather than a characteristic of procedural knowledge (Anderson Citation1983; Rittle-Johnson and Schneider Citation2015).
Declarative knowledge, in turn, includes semantic knowledge, which deals with general, socially shared knowledge about the world, and episodic knowledge, which contains specific memories from one’s own life (Hasselhorn and Gold Citation2006; Winkel, Petermann, and Petermann Citation2006).
Declarative knowledge is the knowledge that something is the case (Woolfolk Citation2008). Hence it is also called ‘knowing that’ (Woolfolk Citation2008) or the ‘knowing why’ (Schneider Citation2006). Declarative knowledge is stored as propositions and propositional networks, imaginary images, and schemata (Woolfolk Citation2008). Declarative knowledge is the implicit or explicit knowledge (Goldin-Meadow, Alibali, and Church Citation1993) about facts, concepts, and connections of a domain. The interconnectedness of this knowledge is seen as a quality feature and not as a characteristic of declarative knowledge per se (Star Citation2005).
Procedural and declarative knowledge in scientific thinking
The literature on general problem solving provides only a limited basis for the analysis of problem-solving in scientific inquiry. Accordingly, there is a procedural level (knowledge of how to proceed) and a declarative level in problem-solving in scientific inquiry (knowledge of why this is done; procedural understanding; e.g. Roberts and Gott Citation2003; Mayer Citation2007). However, in scientific inquiry, declarative knowledge about the object under investigation (content knowledge) is added (Chen and Klahr Citation1999; Gott and Dugan 1995; Mayer Citation2007). Historically, content knowledge is even more important, since, for a long time, it was the sole learning objective of science lessons (Abd-El-Khalick et al. Citation2004; Anderson Citation2002). Inquiry learning was merely an instrument for illustrating and consolidating content knowledge, and pupils should be able to follow and implement recipe-like instructions for this purpose (Arnold, Kremer, and Mayer Citation2014). Only practical and manual skills were required. The cognitive part of procedural knowledge (knowing how to proceed) and the declarative level of scientific inquiry, i.e. knowing why something is being done (procedural unterstanding), were secondary (Mayer Citation2007). It was not until the educational reforms in the last decades and the introduction of educational standards in several countries (e.g. Germany, Switzerland, USA) that scientific thinking in the natural sciences became a learning goal on its own. Thus the importance of procedural understanding on the declarative level, beyond content knowledge, has become emphasized (Gott and Duggan Citation1995). The focus of this paper is on this type of knowledge: procedural understanding.
Procedural understanding
Procedural understanding comprises knowledge about or understanding of scientific methods (e.g. experiments), their limits, and possibilities. The concept arose from opposition to recipe-like learning in science teaching, in which no thought is given to action, and which primarily serves to acquire or illustrate content knowledge. The concept goes back to the British school around Richard Gott and Sandra Duggan (Gott and Duggan Citation1995). It focuses on the declarative knowledge behind the action or the understanding of the actions taken:
Procedural understanding is the understanding of a set of ideas, which is complementary to conceptual understanding, but related to the “knowing how” of science and concerned with the understanding needed to put science into practice. It is the thinking behind the doing. (Gott and Duggan Citation1995), p. 26
The construct of procedural understanding is described by the authors as follows in distinction to procedural knowledge and other types of declarative knowledge:
The term ‘procedural knowledge’ is found in several areas (Star Citation2000), not just in science, and implies ‘knowing how to proceed’; in effect, in science, a synthesis of manual skills, ideas about evidence, tacit understanding from doing and the substantive content knowledge ideas relevant to the context. In science education in the UK and our work, the term procedural understanding has been used to describe the understanding of ideas about evidence, which underpin an understanding of how to proceed. The term procedural understanding has been used to distinguish ideas about evidence from other, more traditional substantive ideas. We have argued that a lack of ideas about evidence prevents students from exhibiting an understanding of how to proceed. (Glaesser et al. Citation2009a), p. 597
Procedural understanding hence is also called the ‘thinking behind the doing’ (Roberts Citation2001, 113). Accordingly, it involves an understanding of concepts like variables, measurements, and representative samples (Roberts and Johnson Citation2015). Hence, the construct is closely tied to the actual process of scientific inquiry. However, the notion of procedural understanding we use here is even closer to the individual process steps of scientific inquiry (Mayer Citation2007; Arnold, Kremer, and Mayer Citation2016). As procedural understanding can be seen as the answer to the question of ‘Why?’ in doing science, this question can always be answered with quality criteria of science (Gott, Duggan, and Roberts Citationn.d.; Roberts and Gott Citation2003). The main quality criteria in science are objectivity, reliability, and validity. For example, hypotheses are formulated in order to define the variables in focus and their relation to secure validity as well as objectivity (Glaesser et al. Citation2009a; Gott and Duggan Citation1996; Gott, Duggan, and Roberts Citationn.d.; Roberts and Gott Citation2003). In this work, procedural understanding refers specifically to the sub-competencies and competence aspects of scientific thinking (Arnold Citation2015). It is assumed that this knowledge is specific to each sub–competence or aspect (NRC/National Research Council Citation2012) and is based on knowledge of the quality criteria of scientific inquiry (Glaesser et al. Citation2009a; Gott and Duggan Citation1996). It is assumed that this is declarative knowledge, which contains specific concepts about the content, purpose, and function of individual aspects of scientific inquiry (‘knowing why’) and can, therefore, be explicitly promoted. Arnold (Arnold Citation2015, 35–39) identified essential aspects of procedural understanding from literature, which are summarized in the following:
Purpose of the experiment. An important goal of science is to explain the causes of phenomena, which can only be done via experiments. This is why the experiment is regarded as a central method of investigation in the natural sciences (Osborne et al. Citation2003). In order to explain the change of a variable (dependent variable), one manipulates explicitly the variable, which is the hypothesized cause of the change (independent variable; Roberts Citation2001). It is only through experiments (and the systematic variation of independent variables) that questions about causal connections can be answered validly (Roberts Citation2001).
Purpose of hypotheses. A hypothesis is a well-founded assumption about the (causal) connection between two variables (Mayer and Ziemek Citation2006). It predicts the outcome of an investigation (experiment) when the presumed cause (independent variable) is changed, and the changing variable (dependent variable) is measured and is, therefore, a testable or falsifiable statement (Mayer and Ziemek Citation2006). It is formulated before the actual investigation and structures the systematic planning of the investigation by the determination of the variables (validity) and the objective evaluation of data (Hammann Citation2006). Hypotheses and their testing form the basis of scientific research (Osborne et al. Citation2003). A hypothesis is used to identify possible causes by the systematic exclusion of unlikely hypotheses. Therefore, alternative hypotheses, which can be excluded by the hypothesis test (Mayer and Ziemek Citation2006), should be formulated.
Function of the dependent and independent variable. Dependent and independent variables play an important role both in hypothesis formulation and in planning. The independent variable is the factor that is assumed to be the cause and which is to be varied (Chen and Klahr Citation1999; Roberts Citation2001; Tamir, Doran, and Oon Chye Citation1992). The dependent variable is the factor in an experiment that is measured, and the independent variable is the hypothesized causes or condition, which is to be manipulated (Chen and Klahr Citation1999; Roberts Citation2001; Tamir, Doran, and Oon Chye Citation1992).
Purpose of planning. In planning an experiment, the procedure for its implementation is structured and documented. It is the most crucial section of empirical research work because its precision depends on whether the study results in measurable and meaningful results. Essential quality criteria here are, above all, objectivity and validity. The planning must be documented in a way that allows reproducing the experiment exactly and understanding it intersubjectively (Bortz and Döring Citation2006). Also, the planning of a study is based on the hypothesis and must be valid concerning it (Lederman et al. Citation2014). This means that it is necessary to ensure that changes in dependent variables are clearly due to the influence of independent variables (Roberts Citation2001).
Purpose of the operationalization of dependent variables. The dependent variable reflects the effects of the independent variables (causes, conditions). In an experiment, this must, therefore, be measured (Gott, Duggan, and Roberts Citationn.d.; Roberts Citation2001). How it is measured is determined in planning. The three criteria of validity, reliability, and objectivity again play an essential role here. An operationalization must be chosen, which makes it possible to measure what is to be measured (validity). Besides, this operationalization should allow an accurate measurement (reliability) and be intersubjectively comprehensible (objectivity) (Gott and Roberts Citation2003; Roberts and Gott Citation2003).
Purpose of the variation of independent variables. The independent variable is the factor whose influence or effect on the dependent variable (factor to be measured) is examined (Gott, Duggan, and Roberts Citationn.d.; Roberts Citation2001). It is, therefore, systematically varied in an experiment. How it is to be changed is defined in planning. This relates to the number of characteristics and measurement intervals. The quality criteria of scientific inquiry also play a role here. The specification and ranges of the independent variable must be chosen so that they can be reproduced by others (objectivity and reliability). This leads to the fact that what is to be investigated is actually being investigated (validity), (Gott, Duggan, and Roberts Citationn.d.; Roberts and Gott Citation2003).
Purpose of control of confounding variables. Confounding variables are variables that can affect the dependent variable in addition to the independent variable. If these are controlled (by keeping them equal or by measuring them), they are called control variables. The control of confounding variables ensures the precise measurement of the factor of interest. Besides, the reproducibility of the results increases (Gott, Duggan, and Roberts Citationn.d.; Roberts Citation2001).
Purpose of repetitions. Individual measurements/experiments can always be erroneous and uncertain (Gott, Duggan, and Roberts Citationn.d.). To increase the reliability of a measurement/experiment, it has to be carried out multiple times using different measuring methods (Buffler et al. Citation2001; Lubben et al. Citation2001; Lubben and Millar Citation1996). To be able to make meaningful statements, measurements/experiments have to be carried out multiple times, which increases the reliability and validity (Gott and Duggan Citation1995; Osborne et al. Citation2003). Besides, the reproducibility of the results can be checked by repetition. Depending on the investigator, the repetition of the measurement is accompanied by an increase in the sample (Gott, Duggan, and Roberts Citationn.d.).
Purpose of the separation between description and interpretation of data. Data are collected in the process of scientific knowledge acquisition. The description includes the neutral comparison of the obtained data (in an experiment, the experimental and control approach, or in a series of measurements the individual trial approaches are compared). All data must be included (Osborne et al. Citation2003). This is the basis for the interpretation (Lederman et al. Citation2002; Osborne et al. Citation2003). However, data does not speak for itself but has to be interpreted regarding the question or hypothesis. This subjectively colored step has to anticipate an objective description of the data (Lederman et al. Citation2014, Citation2002; Osborne et al. Citation2003). The interpretation summarizes the data and assesses it with regard to the hypothesis. Only the interpretation gives meaning to data. The independent variable is identified as a probable cause, or the hypothesis is considered to be rejected. Since the interpretation differs from a purely descriptive, objective description, it is to be separated from it (Osborne et al. Citation2003). The interpretation is a subjectively expressed step, which nevertheless has to be closely related to the existing data (Lederman et al. Citation2014, Citation2002).
Purpose of critical reflection. Investigations always represent only part of reality and must, therefore, always be evaluated with regard to their meaningfulness. This concerns questions of the validity of the experimental setup and the reliability of the measurement. Within the scope of the data evaluation, the limits of the meaningfulness of the data and the investigation are pointed out (Mayer and Ziemek Citation2006; Osborne et al. Citation2003). Additionally, the design is critically discussed with regard to its validity (concerning the hypothesis) and its reliability. Approaches to the generalizability and optimization of the procedure are followed and, if necessary, lead to new investigations.
Addressing the concepts of procedural understanding (or the described similar constructs) in science classrooms takes into account that doing science is more than carrying out memorized procedures (Roberts and Gott Citation2003). Furthermore, procedural understanding can improve scientific thinking, because procedural understanding and conceptual understanding, as well as procedural knowledge, were found to be positively correlated (Kremer and Mayer Citation2013; Roberts and Gott Citation2004). Even more, procedural understanding is necessary for conducting scientific experiments (Glaesser et al. Citation2009b). Additionally, it was shown that students often lack the procedural understanding necessary for successfully conducting experiments (Arnold, Kremer, and Mayer Citation2014) and that activating students’ procedural understanding during scientific inquiry can improve students’ scientific thinking (Arnold Citation2015; Arnold, Kremer, and Mayer Citation2017).
In this paper, we argue that procedural understanding in terms of understanding why the different steps are essential with respect to the quality criteria is essential for scientific inquiry and scientific literacy. Applying the concepts of objectivity, reliability, and validity gives a universal understanding of scientific inquiry because that is what all scientific inquiry is about and what differentiates it from non-scientific actions. In this paper, we present two studies that deal with surveying students’ ideas of procedural understanding.
Although there are instruments for surveying procedural understanding, for example, the evidence test (Gott and Roberts Citation2008), or test for procedural understanding (Arnold Citation2015). They ask students to identify dependent and independent variables, to find adequate controls for experimental settings, to choose appropriate measuring techniques, or to discuss scientific activities or the influences of scientists’ work, for example. But none of these instruments ask students directly about what they think, why the different steps of scientific inquiry are essential. Hence, the goal of this research is to 1) identify students’ ideas about why different steps of scientific inquiry are important and 2) to give quantitative information about ideas related to quality criteria in science. These goals are addressed in two studies.
Study 1: Identifying ideas about the purpose of scientific inquiry
The first study aimed at answering the question, which ideas students hold about the purpose of the different aspects of scientific inquiry (RQ1).
Materials and methods
The sample of this study consisted of forty-seven students (mean age 16.9; 68% girls; 11th grade) of a German Gymnasium. They were part of a study where they had to work on two inquiry projects guided by research booklets during their normal lessons (Arnold Citation2015; Arnold, Kremer, and Mayer Citation2017). Students worked in groups of two to three and before each step of inquiry they had to comment on Concept Cartoons (Arnold, Kremer, and Mayer Citation2014) that were included in the research booklets ().
Figure 2. Exemplary concept cartoon ‘Why do we need a hypothesis?’ (Arnold, Kremer, and Mayer Citation2017; translated).
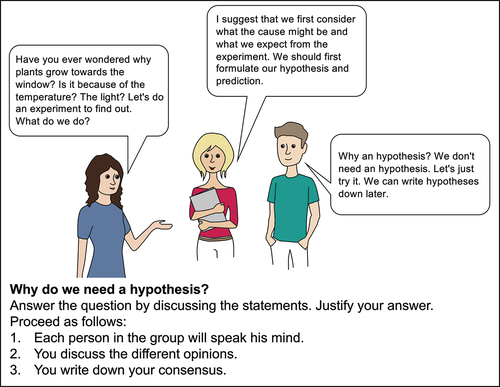
Concept Cartoons are cartoon-like drawings of different characters who have different views about a concept. They are to activate students’ ideas about the specific concept and/or make them discuss them (Keogh and Naylor Citation1998; Naylor and Keogh Citation1999). Concept Cartoons can be used for assessment as well as for teaching and learning (Keogh and Naylor Citation1998). In the context of procedural understanding, they have previously been used as assessment method (e.g. Allie and Buffler Citation1998; Allie et al. Citation1998; Buffler et al. Citation2001; Lubben et al. Citation2001; Lubben and Millar Citation1996; Millar et al. Citation1994) as well as a teaching method (Kuhn et al. Citation2000). For this study, they are also used as an assessment method. In our cartoons, three to four characters are discussing steps of scientific inquiry, and they raise the question about why one would need that specific step (e.g. ). In total, students had to answer eight different Concept Cartoons that were distributed among the two booklets. They asked students to discuss e.g. the reasons for formulating hypotheses, planning experiments, operationalizing dependent variables, or repeating experiments.
Students in the study had to discuss these questions and write down reasons. The written answers were then divided into single statements, this means that one answer could include different statements. These statements were categorized inductively into subcategories thus creating a coding manual, which included exemplary answers. An exemplary coding manual is given in . These subcategories were subsequently summarized to the quality criteria objectivity, reliability, and validity as broader categories. If sub-categories or statements did not (indirectly) deal with quality criteria, they were assigned to the category ‘other’ (see example in ), which will not be reported in this paper, because they were not used for study 2 due to economic factors. Subsequently, a second independent rater got the statements and the coding manual and had to assign it to the scheme of sub-categories and categories. Interrater-agreement was measured using Cohen’s κ and was found to be .55 < κ < .86. In case of disagreement, an agreement was reached in discussion. An overview of all Concept Cartoons and the Interrater-agreement is given in .
Table 1. Coding manual for concept cartoon ‘Why do we need a hypothesis?’.
Table 2. Concept cartoon-questions (Arnold Citation2015; Arnold, Kremer, and Mayer Citation2016).
Results
summarizes all the sub-categories (ideas of procedural understanding) concerning the quality criteria. Because these statements occur throughout the paper, we introduce abbreviations in this Table. One has to notice that there are no completely wrong answers to the posed questions, so there instead is a continuum of more or less importance.
Table 3. Ideas of procedural understanding, abbreviations, and matching quality criteria.
It can be seen that about half (N = 428) of a total of 830 statements for the cartoons refer to the quality criteria (validity, reliability and objectivity). The proportion of statements relating to quality criteria is highest in the cartoon on confounding variables (CV) (89.19%), followed by alternative hypotheses (AH; 68.18%) and variation of the independent variable (IV; 67.78%). The measurement of the dependent variable (DV) and the repetition of experiments (RE) are justified with quality criteria to 62.07%. Planning experiments (PL; 35.92%), describing data (DE; 33.04%) and hypotheses (HY; 22.31%) are least frequently associated with quality criteria. Here, mainly practical reasons are given in the category ‘other’. Over all, the majority of the statements refer to validity (N = 234), followed by aspects of objectivity (N = 117) and statements relating to reliability are the rarest (N = 77).
Study 2: Educational data mining for core ideas
Study 2 aimed at quantifying the data from study 1. After knowing a variety of different ideas about why different steps in inquiry are necessary in terms of scientific quality criteria in the students’ eyes (RQ 1), the goal of the second study was to find out in how far students agree to these ideas (RQ2.1). Furthermore, we wanted to find out if there are core ideas that seem to be particularly important to the concept of procedural understanding for students (RQ2.2).
Materials and methods
For quantification, a questionnaire was developed that showed a) the Concept Cartoons and b) the respective sub-categories from study 1 (see ). Students had to agree or disagree with these statements on a five-point Likert scale. Additionally, students had to answer a 12-question multiple-choice-test on procedural understanding (Arnold Citation2015; Cronbach’s α = .59). The test included one item each for the purpose or function of (1) experiments, (2) hypotheses, (3) the independent variable, (4) the dependent variable, (5) the planning of experiments, (6) the operationalization of dependent variables, (7) the variation of independent variables, (8) the control of confounding variables, (9) repetitions, (10) separation between description and interpretation of data, as well as to (11) validity and (12) objectivity. The questionnaire and the test were administered as a paper-pencil-test and the students had 25 minutes to answer it without help. The sample consisted of sixty-four students (mean age 16.13; 54.7% girls; 11th and 12th grade) of a German Gymnasium. Four of the students had biology as an advanced course. Regarding recruitment, it should be noted that the teachers decided to take part in the study, but the students all filled in the questionnaire voluntarily. Furthermore, the questionnaire was filled out anonymously and neither participation nor completion had any consequences for the students.
To answer RQ2.1, the mean agreement to each statement was calculated (‘strongly agree’ and ‘agree’). To answer RQ2.2, we used an approach based on educational data mining (EDM; Baker Citation2010). EDM applies computational analyses on (often large) datasets to identify patterns and structures, typically in an exploratory setting (Baker and Yacef Citation2009). Notably, this also includes the distillation and visualization of data for human judgment, which we predominantly rely on in this study. Since the research question is a somewhat open one, an exploratory approach seems best to identify how to proceed further.
The methods that we used are: Visualizing the interdependencies between the statements by visualizing the magnitude of all significant correlations. This was done by applying the multi-dimensional scaling (MDS) algorithm on the cross-correlation matrix (Cox and Cox Citation2001). The result is a two-dimensional layout of a graph representing the interconnected structure between the students’ agreements with the respective statements. We then applied several methods of graph analyses to support our qualitative analysis of the structure quantitatively. Most notably, we used the page-rank algorithm by Brin and Page (Citation1998) that is also used to identify the importance of search results in the search engine Google and the identification of maximally large cliques in the graph (Balakrishnan and Ranganathan Citation2012). The analyses were done with GNU-R (R Core Team Citation2013) and the package ‘igraph’.
Additionally, a t-test was done with median-splitted test-scores on procedural understanding to look for statements that differentiate high (N = 25) from low (N = 39) performers on the test.
Results
In , we present the percentage of the agreement to the statements.
Figure 4. Percentage of the agreement to concept-cartoon-items (the bars represent the combined ‘strongly agree’ and ‘agree’ answers).
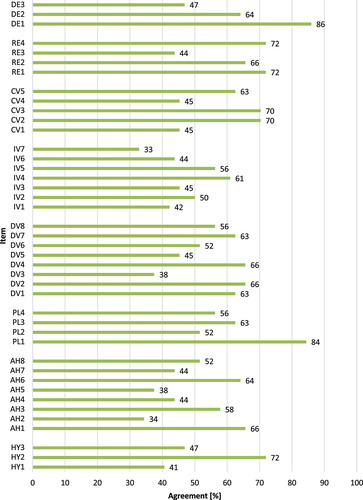
As can be seen from , the agreement to the statements varies from 33% (‘The type of variation of the independent variable should be carefully considered, to select sufficient appropriate intervals’) to 86% (‘The description of the data serves the traceability’). When the students are asked to assess the importance of the reasons for the different steps, the majority of them agree to their importance: The reasons for the considered variation of the independent variable are rated with an average of 47.32% agreement, those for an alternative hypothesis with 49.80% agreement and those for making a hypothesis with 53.13% agreement. The reasons for the considered operationalization of the dependent variable are approved with 55.86% and those for the control of confounding variables with 58.75%. The reasons for repetitions, the planning of an experiment and the description of the results were most agreed with 63.28%, 63.37% and 65.63%. Overall, the level of agreement with the statements also varies greatly within an aspect. For example, more than 80% agree with the statements that one should describe the results to ensure traceability and that an experiment should be planned to investigate what should be investigated. In contrast to the open questions, objectivity as the reason for the data description and validity as the reason for the planning seem to be given high priority here. With regard to the quality criteria, the justifications relating to validity receive on average 56.73% support, those relating to reliability 55.13% and those relating to objectivity are the least supported at 53.65%.
In , the significant correlations (p < .05) between the 42 ideas of procedural understanding are displayed. Two statements are connected if there exists a (positive) significant correlation between the students’ answers for this pair. The layout has then been derived algorithmically using MDS such that the magnitude of each correlation is inversely reflected by the distance of the corresponding statements (the higher the magnitude, the closer together). As it is, in general, not possible to reduce the high-dimensional space of distances on the two-dimensional plane accurately, the MDS-algorithm minimizes the inevitable inaccuracies.
Figure 5. Significant correlations (p < .05) between the ideas of procedural understanding (Arnold, Kremer & Mühling, Citation2017).

One can see that there are three concepts that are not related to other concepts (DV4, PL1, and AH5), i.e. there is no significant correlation to any of the other statements. Also, certain concepts do appear to be very central in the overall structure. While there are many candidates that do appear centrally, the statements concerning the confounding variable (CVx) stick out as 4 out of 5 statements are located very prominently in the structure.
When calculating the mean correlation of each statement to all other statements, the five highest-ranking statements are CV2, CV3, CV5, PL2, and AH1 (marked green and red in ). Again, indicating central importance for the statements regarding confounding variables.
The page rank algorithm identifies how ‘relevant’ one node in a graph is by calculating iteratively its page rank value that is dependent on how many other nodes are connected with this node and how high the page ranks of the connected nodes are. The more connections of a high-ranking node, the higher a node will be ranked itself. Similar reasoning is applied in deriving citation graphs. When calculating the page ranks for all statements in the structure derived from the correlations, the top 5 statements are CV2, CV3, CV4, AH1, PL2. Again, the concept of confounding variables appears saliently in these results.
Next, we investigated the maximally larges cliques of the structure. A clique is a subset of nodes (i.e. statements in this case) for which every possible connection within the subset is present in the graph structure. So, in our case, a subset of statements that all show significant correlations among each other. Since every pair of two connected nodes forms a clique of size 2, and there are usually many cliques of size 3, one is typically interested in the largest subsets that can be identified in a structure. For the structure in , the largest appearing cliques have a size of 4 statements. There are three such cliques made up of the statements:
HY3, IV1, IV7, CV4
AH1, DV2, CV2, CV3
DV2, CV2, CV3, CV5
Again, the statements concerned with confounding variables appear prominently, as at least one appears in each of the three cliques, which is not valid for any of the other concepts.
The t-test revealed that there are six concepts that differentiate good from bad performers on the procedural-understanding-test (p < .05; ) with little to medium effect sizes (.27 < r < .47).
Table 4. Concepts that differentiate good from bad performers on the procedural-understanding-test (p < .05).
One can see that students that perform better on the test (> 5 points) also have a significantly higher agreement with statements AH1, AH2, PL2, CV3, and CV5. Four of these statements are also included in the top-five relations to all other statements. But there is one item where students that perform better on the test agree significantly less to (AH4). This is also one of the items that have no significant correlations to any other.
Discussion
Procedural understanding is essential to inquiry learning and a valuable learning goal that has to be addressed explicitly. In this paper, we understand procedural understanding as the knowledge that underlies scientific inquiry and goes beyond mere manual skills. Procedural understanding is the ‘knowledge behind doing’ and refers explicitly to the sub–competencies and competence aspects of scientific thinking (Arnold Citation2015; ). We differentiate eight central topics of procedural understanding: (1) purpose of hypotheses, (2) purpose of planning experiments, (3) purpose of the operationalization of dependent variables, (4) purpose of the variation of independent variables, (5) purpose of the separation between description and interpretation of data, (6) purpose of alternative hypotheses, (7) purpose of control of confounding variables, (8) purpose of repetitions.
We collected students’ ideas of procedural understanding in regard to the purpose of these topics and were able to identify 42 ideas that related to quality criteria of science. It should be noted that even if the ideas of the learners in the study were counted without weighting, they are not all equally valuable or equally appropriate. Even within one category more or less appropriate ideas can be found. For example, one could compare statements that explicitly state the quality criteria (e.g. ‘B must know whether he is investigating what he wants to investigate (validity)’) with those that paraphrase the concept (e.g. ‘You cannot start without a hypothesis, because you need it to know what you want to check’; see ).
Nevertheless, the 42 ideas identified in Study 1 cover the core of what learners need to know about scientific inquiry as derived from literature (see the points 1–10 above). The results of Study 1 suggest that students rarely associate the individual steps of scientific inquiry with quality criteria of scientific work. If they do, they predominantly cite aspects of validity. Although validity is an important and overarching quality criterion (Roberts and Johnson Citation2015), it is not the only one. Reasons of objectivity and reliability seem to be less considered here. Furthermore, essential parts of scientific inquiry, such as planning experiments, formulating hypotheses, and objectively describing results, seem to have little to do with quality criteria of scientific inquiry for students. Furthermore, it is noticeable that the concept of falsifiability as a validity criterion hardly seems to play a role, e.g. in (alternative) hypotheses. This may be due to the fact that it is a less intuitive concept that is also rarely actively named by scientists themselves (Harwood, Reiff, and Phillipson Citation2002) Besides the intersubjective comprehensibility and repeatability as concepts of objectivity are only little considered during the planning and the definition of the variables as well as the repetition of investigations.
With regard to RQ2.1, it can be seen that students are more likely to agree with the reasons for the individual aspects as compared to Study 1, where they had to actively name them. In contrast to Study 1, the planning of an experiment and the description of the results, for example, seem to do better in the assessment of existing statements than in open formulation, and the difference between the quality criteria suggested in Study 1 also seems to dissolve here. Nevertheless, an understanding of the purpose of planning experiments and describing data in scientific inquiry could be supported and a promotion of the understanding of the quality criteria objectivity and reliability could be useful to enable students to actively consider them earlier. Regarding RQ2.2, we applied novel techniques of educational data mining and showed how to identify statements that appear to be central for students’ understanding. The ideas derived from qualitatively analyzing the algorithmic visualization of significant correlations are backed by the analysis of using page rank and cliques and hence seem to be important for understanding scientific inquiry. The top-five core-ideas are
One needs alternative hypotheses, because there may be other variables worth considering as cause. (AH1)
The planning helps to take into account confounding variables or external circumstances. (PL2)
Confounding variables should be controlled since they influence the experiment, the dependent variable. (CV2)
Confounding variables should be controlled since the omission may lead to inconclusive results. (CV3)
Confounding variables should be controlled to ensure accurate measurement. (CV5)
It turned out that these statements are all related to the same concept, namely the concept of confounding variables and therefore, can function as core-ideas. The concept of confounding variables is closely related to the principle of variable control strategy (CVS), which is regarded as central to scientific inquiry (Schalk et al. Citation2019). Accordingly, the CVS is promoted in class in a focused manner (Schwichow et al. Citation2016), which can be a reason why these ideas appear to be central in this study. But, four out of the five statements turned out to be differentiating between good and bad performers in a procedural understanding-test, which supports the importance of these ideas. Nevertheless, what learners have learned in class in terms of experiments and scientific inquiry should be controlled in future studies.
Concerning limitations of the two studies, we have to mention that the connection between these items made in the analyses is based on correlations in a broader sense. This refers mainly to MDS. These correlations could of course also have methodological reasons, e.g. because the items are formulated in a similar way. However, further analyses, such as page rank and those for identifying cliques and the comparison with the probability of solution in the test on procedural understanding, support the hypothesis that the concepts are of special importance for the understanding of scientific inquiry since they are not only closely connected to each other, but also strongly connected to the other concepts. Above that, we found one item acting oddly (AH4), which turned out to be an idea that did not correlate to any other idea. This may indicate that the item is malfunctioning, the item or the respective concept should be looked at in further studies. Furthermore, it should also be noted that the samples for both studies are relatively small and the reliability of the procedural understanding test is limited, as well as is the test itself is only a short and economic test to assess procedural understanding. Its informative value is accordingly also limited. The results therefore only provide clues for hypotheses that need to be analyzed in further studies.
Finally, we have to note that we view this study as explorative to get to the core ideas of procedural understanding. From these findings, we can now deduce hypotheses that can be tested in instruction studies. Here, we argue that fostering the core ideas of procedural understanding within an intervention could result in more significant learning gain than promoting some other ideas of procedural understanding. We assume this should be the case because the core ideas are closely tied to other ideas, and hence a deeper understanding of them could lead to a more elaborate understanding of related ideas. Accordingly, we hope to contribute to the promotion of scientific thinking by encouraging ‘thinking behind the doing’.
Disclosure statement
Parts of this research have been published in German language in Arnold et al. (Citation2017).
References
- Abd-El-Khalick, F., S. BouJaoude, R. Duschl, N. G. Lederman, R. Mamlok-Naaman, A. Hofstein, M. Niaz, D. Treagust, and H.-L. Tuan. 2004. “Inquiry in Science Education: International Perspectives.” Science Education 88 (3): 397–419. doi:10.1002/sce.10118.
- Allie, S., and A. Buffler. 1998. “A Course in Tools and Procedures for Physics I.” American Journal of Physics 66 (7): 613–624. doi:10.1119/1.18915.
- Allie, S., A. Buffler, B. Campbell, and F. Lubben. 1998. “First-year Physics Students’ Perceptions of the Quality of Experimental Measurements.” International Journal of Science Education 20 (4): 447–459. doi:10.1080/0950069980200405.
- Anderson, J. R. 1983. The Architecture of Cognition. Cambridge, MA: Harvard Univ. Press.
- Anderson, R. D. 2002. “Reforming Science Teaching: What Research Says About Inquiry.” Journal of Science Teacher Education 13 (1): 1–12. doi:10.1023/A:1015171124982.
- Arnold, J. 2015. Die Wirksamkeit von Lernunterstützungen beim Forschenden Lernen: Eine Interventionsstudie zur Förderung des Wissenschaftlichen Denkens in der gymnasialen Oberstufe. Berlin: Logos.
- Arnold, J., K. Kremer, and A. Mühling. 2017. “Denn sie wissen nicht, was sie tun« Educational Data Mining zu Schülervorstellungen im Bereich Methodenwissen.” Mathematisch und naturwissenschaftlicher Unterricht (MNU) 70 (5): 334–340.
- Arnold, J., K. Kremer, and J. Mayer. 2016. “Concept Cartoons als diskursiv-reflexive Szenarien zur Aktivierung des Methodenwissens beim Forschenden Lernen.” Biologie Lehren und Lernen– Zeitschrift für Didaktik der Biologie 20 (1): 33–43. doi:10.4119/UNIBI/zdb-v1-i20-324.
- Arnold, J., K. Kremer, and J. Mayer. 2017. “Scaffolding beim Forschenden Lernen - Eine empirische Untersuchung zur Wirkung von Lernunterstützungen.” Zeitschrift für Didaktik der Naturwissenschaften 23 (1): 21–37. doi:10.1007/s40573-016-0053-0.
- Arnold, J. C., K. Kremer, and J. Mayer. 2014. “Understanding Students‘ Experiments—What Kind of Support Do They Need in Inquiry Tasks?” International Journal of Science Education 36 (16): 2719–2749. doi:10.1080/09500693.2014.930209.
- Arnold, J. C., W. J. Boone, K. Kremer, and J. Mayer. 2018. “Assessment of Competencies in Scientific Inquiry through the Application of Rasch Measurement Techniques.” Education Sciences 8 (4): 184. doi:10.3390/educsci8040184.
- Baker, R. S. J. D. 2010. “Data Mining.” In International Encyclopedia of Education, edited by B. McGaw, P. Peterson, and E. Baker, 112–118. Oxford: Elsevier.
- Baker, R. S. J. D., and K. Yacef. 2009. “The State of Educational Data Mining in 2009: A Review and Future Visions.” Journal of Educational Data Mining 1 (1): 3–16.
- Balakrishnan, R., and K. Ranganathan. 2012. A Textbook of Graph Theory. Heidelberg: Springer.
- Baroody, A. J. 2003. “The Development of Adaptive Expertise and Flexibility: The Integration of Conceptual and Procedural Knowledge.” In The Development of Arithmetic Concepts and Skills: Constructing Adaptive Expertise, edited by A. J. Baroody and A. Dowker, 1–33. Mahwah, NJ: Erlbaum.
- Bortz, J., and N. Döring. 2006. Forschungsmethoden Und Evaluation Für Human- Und Sozialwissenschaftler [Research Methods and Evaluation for Human and Social Scientists]. Heidelberg: Springer.
- Brin, S., and L. Page. 1998. “The Anatomy of a Large-scale Hypertextual Web Search Engine.” Computer Networks and ISDN Systems 30 (1–7): 107–117. doi:10.1016/S0169-7552(98)00110-X.
- Buffler, A., S. Allie, F. Lubben, and B. Campbell. 2001. “The Development of First Year Physics Students’ Ideas about Measurement in Terms of Point and Set Paradigms.” International Journal of Science Education 23 (11): 1137–1156. doi:10.1080/09500690110039567.
- Byrnes, J. P., and B. A. Wasik. 1991. “Role of Conceptual Knowledge in Mathematical Procedural Learning.” Developmental Psychology 27 (5): 777–786. doi:10.1037/0012-1649.27.5.777.
- Chen, Z., and D. Klahr. 1999. “All Other Things Being Equal: Acquisition and Transfer of the Control of Variables Strategy.” Child Development 70 (5): 1098–1120. doi:10.1111/1467-8624.00081.
- Cox, T. F., and M. A. A. Cox. 2001. Multidimensional Scaling. Boca Raton, FL: Chapman and Hall/CRC.
- Glaesser, J., R. Gott, R. Roberts, and B. Cooper. 2009a. “The Roles of Substantive and Procedural Understanding in Open-Ended Science Investigations: Using Fuzzy Set Qualitative Comparative Analysis to Compare Two Different Tasks.” Research in Science Education 39 (4): 595–624. doi:10.1007/s11165-008-9108-7.
- Glaesser, J., R. Gott, R. Roberts, and B. Cooper. 2009b. “Underlying Success in Open-Ended Investigations in Science: Using Qualitative Comparative Analysis to Identify Necessary and Sufficient Conditions.” Research in Science and Technological Education 27 (1): 5–30. doi:10.1080/02635140802658784.
- Goldin-Meadow, S., M. W. Alibali, and R. B. Church. 1993. “Transitions in Concept Acquisition: Using the Hand to Read the Mind.” Psychological Review 100 (2): 279–297. doi:10.1037/0033-295X.100.2.279.
- Gott, R., and R. Roberts. 2003. “Assessment of Performance in Practical Science and Pupils` Attributes.” British Educational Research Association Annual Conference, Heriot-Watt University, Edinburgh.
- Gott, R., and R. Roberts. 2008. “Concepts of Evidence and Their Role in Open-ended Practical Investigations and Scientific Literacy; Background to Published Papers.” https://www.dur.ac.uk/resources/education/research/res_rep_short_master_final.pdf
- Gott, R., and S. Duggan. 1995. Investigative Work in the Science Curriculum. Buckingham: Open University Press.
- Gott, R., and S. Duggan. 1996. “Practical Work: Its Role in the Understanding of Evidence in Science.” International Journal of Science Education 18 (7): 791–806. doi:10.1080/0950069960180705.
- Gott, R., S. Duggan, and R. Roberts. n.d. “Concepts of Evidence.” http://www.dur.ac.uk/rosalyn.roberts/Evidence/CofEv_Gott_et_al.pdf
- Hammann, M. 2006. “Fehlerfrei Experimentieren [Error-free experimentation].” Der Mathematische und Naturwissenschaftliche Unterricht 5 (59): 292–299.
- Harwood, W. S., R. Reiff, and T. Phillipson. 2002. “Scientists´ Conceptions of Scientific Inquiry: Voices from the Front.” Paper presented at the Proceedings of the Annual International Conference of the Association for the Education of Teachers in Science, Charlotte, NC.
- Hasselhorn, M., and A. Gold. 2006. Pädagogische Psychologie: Erfolgreiches Lernen und Lehren [Educational psychology: Successful learning and teaching]. Stuttgart: Kohlhammer.
- Hiebert, J., and P. Lefevre. 1986. “Conceptual and Procedural Knowledge in Mathematics: An Introductory Analysis.” In Conceptual and Procedural Knowledge: The Case of Mathematics, edited by J. Hiebert, 1–27. Hillsdale, NJ: Lawrence Erlbaum Associates.
- Keogh, B., and S. Naylor. 1998. “Teaching and Learning in Science Using Concept Cartoons.” Primary Science Review.
- Kremer, K., and J. Mayer. 2013. “Entwicklung und Stabilität von Vorstellungen über die Natur der Naturwissenschaften.” Zeitschrift für Didaktik der Naturwissenschaften (ZfDN) 19, 77–101.
- Kuhn, D., J. Black, A. Keselman, and D. Kaplan. 2000. “The Development of Cognitive Skills to Support Inquiry Learning.” Cognition and Instruction 18 (4): 495–523. doi:10.1207/S1532690XCI1804_3.
- Lederman, J. S., N. G. Lederman, S. A. Bartos, S. L. Bartels, A. A. Meyer, and R. S. Schwartz. 2014. “Meaningful Assessment of Learners‘ Understandings about Scientific inquiry-The Views about Scientific Inquiry (VASI) Questionnaire.” Journal of Research in Science Teaching 51 (1): 65–83. doi:10.1002/tea.21125.
- Lederman, N. G., F. Abd-El-Khalick, R. L. Bell, and R. S. Schwartz. 2002. “Views of Nature of Science Questionnaire: Toward Valid and Meaningful Assessment of Learners‘ Conceptions of Nature of Science.” Journal of Research in Science Teaching 39 (6): 497–521. doi:10.1002/tea.10034.
- Lubben, F., B. Campbell, A. Buffler, and S. Allie. 2001. “Point and Set Reasoning in Practical Science Measurement by Entering University Freshmen.” Science Education 85 (4): 311–327. doi:10.1002/sce.1012.
- Lubben, F., and R. Millar. 1996. “Children’s Ideas about the Reliability of Experimental Data.” International Journal of Science Education 18 (8): 955–968. doi:10.1080/0950069960180807.
- Mayer, J. 2007. “Erkenntnisgewinnung als wissenschaftliches Problemlösen [Knowledge acquisition as scientific problem solving].” In Theorien in Der Biologiedidaktischen Forschung [Theories in Biology Education Research], edited by D. Krüger and H. Vogt, 177–186. Berlin: Springer.
- Mayer, J., and H.-P. Ziemek. 2006. “Offenes Experimentieren - Forschendes Lernen Im Biologieunterricht [Open Experimentation– Inquiry Learning in Biology Education].” Unterricht Biologie 317: 4–12.
- Millar, R., F. Lubben, R. Gott, and S. Duggan. 1994. “Investigating in the School Science Laboratory: Conceptual and Procedural Knowledge and Their Influence on Performance.” Research Papers in Education 9 (2): 207–248. doi:10.1080/0267152940090205.
- Naylor, S., and B. Keogh. 1999. “Constructivism in Classroom: Theory into Practice.” Journal of Science Teacher Education 10 (2): 93–106. doi:10.1023/A:1009419914289.
- NRC/National Research Council. 2012. A Framework for K-12 Science Education: Practices, Crosscutting Concepts, and Core Ideas. Washington, DC: National Academies Press.
- Osborne, J., S. Collins, M. Ratcliffe, R. Millar, and R. Duschl. 2003. “What “Ideas-about-science” Should Be Taught in School Science? A Delphi Study of the Expert Community.” Journal of Research in Science Teaching 40 (7): 692–720. doi:10.1002/tea.10105.
- R Core Team. 2013. “R: A Language and Environment for Statistical Computing.” Retrieved from http://www.R-project.org
- Rittle-Johnson, B., and M. Schneider. 2015. “Developing Conceptual and Procedural Knowledge of Mathematics.” In Oxford Handbook of Numerical Cognition, edited by R. Cohen Kadosh and A. Dowker, 1112–1118. Oxford: University Press.
- Roberts, R. 2001. “Procedural Understanding in Biology: The ‘Thinking behind the Doing’.” Journal of Biological Education 35 (3): 113–117. doi:10.1080/00219266.2001.9655758.
- Roberts, R., and P. Johnson. 2015. “Understanding the Quality of Data: A Concept Map for ‘The Thinking behind the Doing’ in Scientific Practice.” The Curriculum Journal 26 (3): 345–369. doi:10.1080/09585176.2015.1044459.
- Roberts, R., and R. Gott. 2003. “Assessment of Biology Investigations.” Journal of Biological Education 37 (3): 114–121. doi:10.1080/00219266.2003.9655865.
- Roberts, R., and R. Gott. 2004. “A Written Test for Procedural Understanding: A Way Forward for Assessment in the UK Science Curriculum?” Research in Science and Technological Education 22 (1): 5–21. doi:10.1080/0263514042000187511.
- Schalk, L., P. A. Edelsbrunner, A. Deiglmayr, R. Schumacher, and E. Stern. 2019. “Improved Application of the Control-of-variables Strategy as a Collateral Benefit of Inquiry-based Physics Education in Elementary School.” Learning and Instruction 59: 34–45. doi:10.1016/j.learninstruc.2018.09.006.
- Schneider, M. 2006. Konzeptuelles Und Prozedurales Wissen Als Latente Variablen: Ihre Interaktion Beim Lernen Mit Dezimalbrüchen [Conceptual and Procedural Knowledge as Latent Variables: Their Interaction in Learning with Decimal Fractions]. Berlin: Technische Universität Berlin.
- Schwichow, M., S. Croker, C. Zimmerman, T. Höffler, and H. Härtig. 2016. “Teaching the Control-of-variables Strategy: A Meta-analysis.” Developmental Review 39: 37–63. doi:10.1016/j.dr.2015.12.001.
- Star, J. R. 2005. “Reconceptualizing Procedural Knowledge.” Journal for Research in Mathematics Education 36 (5): 404–411.
- Star, J. R., and K. J. Newton. 2009. “The Nature and Development of Experts’ Strategy Flexibility for Solving Equations.” ZDM - the International Journal on Mathematics Education 41 (5): 557–567. doi:10.1007/s11858-009-0185-5.
- Star, J.R. 2000. “On the relationship between knowing and doing in procedural learning.” In Fourth international conference of the learning sciences, edited by B. Fishman and S. O’Connor-Divelbiss, 80–86. Mahwah, NJ: Erlbaum.
- Tamir, P., R. L. Doran, and Y. Oon Chye. 1992. “Practical Skills Testing in Science.” Studies in Educational Evaluation 18 (3): 263–275. doi:10.1016/0191-491X(92)90001-T.
- Winkel, S., F. Petermann, and U. Petermann. 2006. Lernpsychologie [Psychology of Learning]. Paderborn: Verlag Ferdinand Schöningh.
- Woolfolk, A. 2008. Pädagogische Psychologie [Educational Psychology]. München: Pearson.