Abstract
Officiating bias is thought to contribute to home advantage. Recent research has shown that sports with subjective officiating tend to experience greater home advantage and that referees' decisions can be influenced by crowd noise, but little work has been done to examine whether individual referees vary in their home bias or whether biased decisions contribute to overall home advantage. We develop an ordinal regression model to determine whether various measures of home advantage are affected by the official for the match and by crowd size while controlling for team ability. We examine 5244 English Premier League (EPL) match results involving 50 referees and find that home bias differs between referees. Individual referees give significantly different levels of home advantage, measured as goal differential between the home and away teams, although the significance of this result depends on one referee with a particularly high home advantage (an outlier). Referees vary significantly and robustly in their yellow card and penalty differentials even excluding the outlier. These results confirm that referees are responsible for some of the observed home advantage in the EPL and suggest that home advantage is dependent on the subjective decisions of referees that vary between individuals. We hypothesize that individual referees respond differently to factors such as crowd noise and suggest further research looking at referees' psychological and behavioural responses to biased crowds.
Introduction
The existence of home advantage has been well documented for a variety of sports, including professional football (e.g. Clarke & Norman, Citation1995; Pollard, Citation1986; Pollard & Pollard, Citation2005), even though its causes are still debated. In general, home advantage can be explained by several non-exclusive factors, including rule advantages, travel factors, familiarity of home grounds, and crowd effects (Courneya & Carron, Citation1992). In professional football, rule advantages and familiarity have been shown to be insignificant factors (Nevill & Holder, Citation1999) and travel effects are negligible in leagues where teams are in close proximity and within a single time zone, such as the English Premier League (EPL) (Courneya & Carron, Citation1991; Pace and Carron, Citation1992; Pollard, Citation1986). In contrast, crowd effects have a significant influence on the outcome of many sports, including football (Agnew & Carron, Citation1994; Nevill, Newell, & Gale, Citation1996). Researchers are divided over whether the crowd's effect on home advantage is due primarily to an influence over player performance (e.g. Agnew & Carron, Citation1994; Bray & Widmeyer, Citation2000; Thirer & Rampey, Citation1979) or referee bias (e.g. Nevill & Holder, Citation1999; Sutter & Kochera, Citation2004).
Evidence from other sports suggests that either, or both, mechanisms are plausible. Summer and Winter Olympic sports that are judged, as opposed to objectively scored, have historically indicated a significantly greater home advantage (Balmer, Nevill, & Williams, Citation2001, Citation2003), but even strictly objective events like speedskating can exhibit home advantage (Koning, Citation2005). This effect can even be observed within a single sport: in boxing, for example, subjective points decisions result in more home advantage than knockouts (Balmer, Nevill, & Lane, Citation2005). For most sports, however, it is difficult to assess the extent to which matches are influenced by subjective officiating and, therefore, it is difficult to determine whether the demonstrated influence of crowd effects on match outcome is a consequence of player performance or referee bias.
Various researchers have identified player-centric effects. Behaviourally, Thirer and Rampey (Citation1979) demonstrated that normally cheering crowds led to better performance by home athletes. Psychologically, Jurkovac (Citation1985) found that college basketball players had higher motivation during home matches. Physiologically, researchers have demonstrated that male ice hockey, rugby, and football players all experience higher testosterone at home than away (Kerr & Vanschaik, Citation1995; McGuire, Courneya, Widmeyer, & Carron, Citation1992; Neave & Wolfson, Citation2003). These studies have been used to suggest that home players experience a territorial response, which increases their aggression and allows them to perform better (Neave & Wolfson, Citation2003). However, the research supporting crowd effects on players' performance is equivocal and a number of other researchers have shown no home or away effect on player psychology or performance (see Carron, Loughhead, & Bray, Citation2005).
Researchers have also shown that referees' decisions can be influenced by crowd factors. Referees viewing videotaped potential fouls with crowd noise call significantly fewer fouls for the away team than those presented with just the video; the referees presented with audio also more closely reflect the judgements of the actual match official (Nevill, Balmer, & Williams, Citation1999, Citation2002). Furthermore, referees in the German Bundesliga allowed more stoppage time in matches the home team was losing rather than winning, offering the home team more opportunities to score the equalizing goal (Sutter & Kochera, Citation2004).
Although these studies are a useful means of discovering potential mechanisms by which crowds might influence players or referees, they do not prove that match outcomes are decided by such influences. Conversely, traditional statistical approaches have shown crowd effects do influence match outcomes, but do not address whether players or referees (or both) are the ones being influenced. In this paper, we present a novel statistical approach based on the following proposition: if referees or players influence match outcome on account of crowd effects, then it is extremely likely that individual referees or players will be more or less responsive to such effects than others. If several referees or players are each observed over multiple matches, it should be possible to test for individual differences in response to crowds that affect home advantage. Using this approach, we test for individual variation in referee bias in the English Premiership League.
Surprisingly few researchers have looked at referees on an individual rather than aggregate basis. Nevill et al. (Citation2002) looked at the responses of 40 qualified football referees in England who were asked to view 47 challenges on a television. Referee experience (up to 16 years) correlated positively with the number of fouls referees awarded for the away team, but that further experience actually led to a decrease in fouls awarded for the away team. This study expands on these findings by examining the effect of individual referees on home advantage using actual match results, including detailed analyses of the effect of individual referees, referee experience, and crowd size on home advantage in scoring, red and yellow cards, and penalties awarded.
Professional football is an excellent resource for studying home advantage for a variety of reasons. Researchers have discovered an unusually high home advantage in football matches at all levels (Clarke & Norman, Citation1995; Pollard, Citation2006; Pollard & Pollard, Citation2005). Meanwhile, pitch dimensions are similar and all professional matches are played on natural grass, thus reducing pitch familiarity factors (Nevill & Holder, Citation1999). Unlike baseball, hockey, and some other sports, there are no rule advantages based on a team's status as the home or away side (Courneya & Carron, Citation1992). Finally, nearly all the decisions made by officials in football, many of which are subjective, are made by a single referee.
Within the football world, the EPL is a particularly suitable league for study. England is small and fully contained in one time zone, so that any travel factors are minimized (Pollard, Citation1986). Teams are generally competitive and play a relatively large number of games in a balanced home-and-home season (each team plays each other twice, once at each team's home venue). Attendance at EPL matches is high, and well-trained referees officiate matches at numerous venues over the course of a season. For practical reasons, the EPL is a convenient sample as information about match outcomes, attendance, and referees are reliable and retrievable via the internet. In addition to, and no doubt because of, these advantages, the EPL has been heavily relied upon by scholars interested in confirming home advantage and exploring its causes (e.g. Barnett & Hilditch, Citation1993; Nevill et al., Citation1996; Pollard, Citation1986).
To assess the importance of referee bias in determining home advantage, our model examines the effect of home team ability, away team ability, crowd size, crowd density, between-season effects, and the particular referee for the match on several aspects of game outcome: the goal differential, match result (win/loss/draw), card differential, and penalty differential. If officiating bias is an important cause of home advantage, and each referee responds differently to crowd pressure, then the outcome should depend on the particular match referee. This is an extremely conservative test of referee bias, since if referees behave identically, or with equal favouritism, the referee factor will not be important. However, if referees differ in their systematic level of favouritism for the home team, this factor will show a significant effect.
Methods
We propose that the expected outcome of any particular match is a function of the abilities of the home and away team, home advantage, and luck (random error). Because we are interested in the home advantage associated with individual referees, we use regression analysis, controlling for team abilities, to determine whether the home advantage term systematically differs between referees. This model is conceptually similar to that of Clarke and Norman (Citation1995) except that we model home advantage as a function of referee and crowd effects rather than an attribute of each team. Their model allowed them to ignore team ability metrics since home and away team ability is equal over a complete and balanced season (as each team is counted equally home and away). However, individual referees' schedules are unlikely to “balance”, so we must control for team abilities and use regression analysis rather than aggregate information to assess home advantage (Clarke, Citation2005).
We gathered data based on reported matches from SportsStats' database at www.premiersoccerstats.com for the 1992 – 2002 seasons and from The Independent Football Database at http://www.soccerfactsuk.co.uk/ for the 2002 – 2006 seasons. These data were double-checked with each other and other websites to ensure accuracy. For each match, we recorded the team names, referee, final score, crowd size (attendance), total number of cautions and dismissals for each team, and number of penalty kicks converted by each team (data on the number of penalties awarded to each team were not available for most games). We also estimated crowd density (crowd size divided by the maximum crowd size at that stadium during that season) for each match. Since estimating variation in officiating bias is impossible without sufficient observations for each referee, we only analysed games officiated by a referee with at least 25 matches in the dataset (the results are not dependent on the exact value of this cut-off); this left 50 referees in our sample. We further excluded three matches for which no definitive determination of the match referee could be established. In total, 5244 of the 5566 English Premiership matches played between August 1992 and June 2006 were included in the analysis.
For each match, we calculated the following four metrics to control for the teams' offensive and defensive strength: expected home goals for (xHGF), expected home goals against (xHGA), expected away goals for (xAGF), and expected away goals against (xAGA). These values were based on the average number of goals scored (or conceded) by the home (or away) team per match during the season, excluding the present match. Our model incorporated these four factors along with four independent variables of interest: referee, crowd size, crowd density, and season. Referee and season were treated as categorical variables, whereas the others were treated as continuous variables. We repeatedly dropped the least significant of the explanatory variables and re-ran the model until dropping further variables resulted in a significantly worse model fit (as measured by the likelihood ratio test). In this manner, we tested whether particular referees had a significant effect on particular dependent variables of interest and whether these effects changed between seasons or as a consequence of crowd size/density.
For our primary analysis, we constructed an ordinal (multinomial) regression model with goal differential (gD = home goals minus away goals) as the dependent variable. We also conducted similar analyses using yellow card (caution) differential (ycD), red card (dismissal) differential (rcD), and penalty kick differential (pkD) as dependent variables, since these are possible means by which referees can influence match outcomes. For these observations we controlled for a team's penalty propensity rather than their scoring ability using four metrics (xHCF, xHCA, xACF, xACA) calculated similarly to xHGF, xHGA, xAGF, and xAGA, but using card data (yellow plus red) instead of scoring data. Since both scoring ability and penalty propensity are likely to influence penalty data, we initially included all eight control metrics in the penalty model. This was done instead of calculating four new penalty kick metrics (xHPKF, xHPKA, xAPKF, xAPKA), since penalty kicks are rare enough that such metrics are not very informative (data not shown). While our penalty kick raw data and analysis of overall home advantage in penalties could be biased by differences in the rate home and away teams convert spot kicks, this should not lead to a spurious referee effect on penalties in our analysis unless home and away teams converted spot kicks at systematically different rates based on the match's referee. As this is implausible, converted penalty kicks should serve as a reasonable proxy for penalties awarded by the referee.
In addition to these analyses for home – away bias, we also analysed home and away values separately (i.e. gH = home goals, gA = away goals, and gT = gH + gA as dependent variables instead of just gD) to determine which factors were responsible for any observed home advantage. We also tested the effect of referee experience by adding to each best-fit model the term ref exp, which was equivalent to log (1 + number of previous games refereed in EPL since 1992). Because of our uncertainty of referee experience in the first two years of the dataset, we only used data for 1994 – 2006 in these models, throwing out games where the referee officiated fewer than 25 matches during this period (4400 matches involving 39 referees were retained). All analyses were performed using Proc Genmod (SAS Institute, Inc., Citation1999).
Results
Home advantage in goal differential
As predicted, home teams showed a decisive advantage over visiting teams in the EPL (1.5 home goals vs. 1.1 away goals on average; see ). In the regression model, goal differential (gD) was significantly affected by team ability as well as two other factors: crowd size (χ 2 = 9.4, d.f. = 1, P = 0.002) and referee (χ 2 = 70.0, d.f. = 49, P = 0.03; see ). The attendance effect was in the direction predicted: for every 10,000-person increase in crowd size, home advantage increased by approximately 0.086 goals (95% confidence interval [CI]: 0.03 – 0.14). Crowd density did not have a significant effect on gD even when the correlated variable crowd size was excluded from the analysis (χ 2 = 1.1, d.f. = 1, P = 0.29). Notably, crowd size and referee affect gD by affecting both home scoring and away scoring; total goals scored is not significantly affected by either factor (see ).
Table I. Consistency of home advantage over time for several metrics of EPL games.
Table II. Ordinal regression analysis of goal differential for the EPL (1992 – 2006).
To control for the possibility of overly influential observations inflating our results, we re-ran the analysis using a truncated dataset where gD values below −3 (or above +3) were set to −3 (or +3). The results were nearly identical (crowd size: χ 2 = 9.2, d.f. = 1, P = 0.002; referee: χ 2 = 68.5, d.f. = 49, P = 0.03). However, when the analysis was run using match result (home win, draw or home loss) rather than gD as the dependent variable, the effect of referee was no longer significant (crowd size: χ 2 = 6.8, d.f. = 1, P = 0.001; referee: χ 2 = 52.4, d.f. = 47, P = 0.27).
A plot of the estimated home advantages for each referee in the data shows a skewed distribution of referee bias (). The modal home bias lies above the mean home advantage (gD = 0.41) with a long tail of referees with lower biases and a single referee with a substantially higher bias. Excluding this referee from the analysis pushes the referee effect on home advantage above the level of significance (χ 2 = 57.0, d.f. = 48, P = 0.18), although there is no obvious reason why this referee should be an outlier, particularly since he is well-sampled in the full dataset. Nevertheless, it is worth noting that in our subsequent analyses of penalties and referee experience, his inclusion/exclusion from the dataset does not affect the direction or significance of our results.
Home advantage in cards and penalties
As with goal differential, there was a significant home advantage in cards and penalties: home teams consistently received fewer cards and converted more penalty kicks than visiting teams (see ). Unlike goal scoring, however, yellow and red card averages were not consistent over time. In particular, significantly fewer cards were given in the 1992 and 1993 seasons before FIFA issued several directives aimed at promoting attacking football and enforcing the rules more stringently, particularly asking referees to give cards more liberally (The Independent, Citation1994). Since this could have led to spurious correlations with referees that primarily officiated during these years, we excluded these two seasons from the analysis.
As expected, yellow card differential and goal differential were negatively correlated (as were red card differential and goal differential; see ). After controlling for team penalty propensities, referee had an effect on yellow card differential (χ 2 = 68.6, d.f. = 38, P = 0.002; see ). Referee also had a significant effect on penalty differential (χ 2 = 65.2, d.f. = 38, P = 0.004; see ), although it had no effect on red card differential (see ). Various referees also gave significantly different total numbers of yellow cards, red cards, and penalties per game (see , , and ).
Table III. Ordinal regression analysis of yellow card cautions for the EPL (1994 – 2006).
Table IV. Ordinal regression analysis of red card dismissals for the EPL (1994 – 2006).
Table V. Ordinal regression analysis of penalties in the EPL (1994 – 2006).
Figure 2. The correlation between goal differential (gD) and penalty differential (ycD and rcD) in all EPL games, 1994 – 2006. Error bars represent standard errors. Extreme values for independent variables were collapsed into the appropriate endpoint. Red cards are represented by squares and yellow cards by triangles.
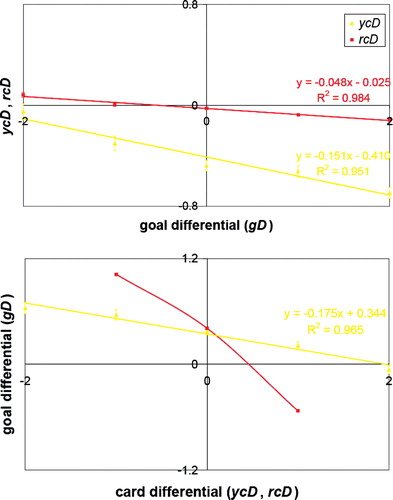
Crowd size also significantly affected yellow card differential (P = 0.03; see ), primarily because larger crowds reduced the number of yellow cards given to the home team (P = 0.009; see ). Crowd density significantly increased the number of yellow cards the away team received (P = 0.03). Similarly, crowd size was negatively correlated with the red card differential (P = 0.04; see ) and the number of red cards the home team received (P = 0.004). Crowd density had a significant positive correlation with the penalty differential (P = 0.01; see ) and a significant negative correlation with the number of away penalties (P = 0.04), although these results could be confounded if away players convert penalty kicks at a lower rate in front of larger crowds.
Referee experience
When ref exp was added to the best-fit model of gD, it was not significant (coefficient = −0.063: χ 2 = 3.4, d.f. = 1, P = 0.07; see ). Interestingly, ref exp was marginally significant when the dependent variable was match result (coefficient = −0.073: χ 2 = 3.7, d.f. = 1, P = 0.05). These results suggest a trend for decreasing home advantage with referee experience.
Table VI. The effect of including ref exp and referee in the best-fit models of the 14 home advantage metrics studied in this paper.
Adding ref exp to the best-fit models of cautions, dismissals, and penalties yielded mixed results (). For cautions, total cautions (ycT =ycH + ycA) and home team cautions were significantly and negatively affected by referee experience (P = 0.03 and P = 0.01 respectively) even though caution differential (ycD = ycH−ycA) was not (P = 0.3). In contrast, total dismissals (rcT) were not significantly affected by referee experience (P = 0.8), but dismissal differential (rcD) was (P = 0.009), with more experienced referees giving fewer dismissals to away teams (P = 0.05). No evidence for an effect of referee experience on penalties was observed.
Alternative models of home advantage
Our primary analysis sought to explain gD using referee, crowd size, and ability terms. A plausible alternative model would include a possible referee ×crowd size interaction term to detect whether referees differ in their response to crowd sizes rather than just in their baseline home advantage. Unfortunately, the multi-collinearity between the interaction term and the referee term result in both being non-significant (P = 0.8 and P = 0.9, respectively), making it impossible to determine whether the significant referee effect is due to differences in baseline home bias or crowd-size-influenced home bias.
We also considered the possibility that teams differ not just in ability but also in home advantage. Clarke and Norman (Citation1995) proposed such a scenario, although they proposed that home advantage varied between clubs across years (home team × season). This factor introduced more variables than could accurately be estimated using this dataset and was not justified on account of a non-significant season effect. Thus, we augmented our model with just the independent variable home team and found that it was significant (P = 0.004), while crowd size and crowd density were not (P = 0.9 and P = 0.8, respectively). The significance of the referee term was unchanged (P = 0.03 including all referees; P = 0.15 excluding the referee with the highest observed home advantage).
Discussion
The results demonstrate that individual referees influence the outcome of English Premier League matches. Since different referees have significantly different levels of home advantage, referees are likely responsible for at the least some of the observed home advantage in EPL football. However, given that one referee had extremely high home advantage, other leagues should be tested to verify the robustness of this result. More generally, though, referee psychology seems to affect the amount of home advantage given as more experienced referees give less home advantage. That result confirms Nevill and co-workers' (Citation2002) result inasmuch as Premiership referees likely have been refereeing at least 16 years and thus fall into their declining home advantage period. Referees may be able to adapt to game conditions such as crowd noise and pressure over many seasons, suggesting that home advantage is caused by a psychological mechanism in referees. It also fits with Nevill and colleagues' (1996) finding that home advantage is highest in the English First Division, where referees face smaller crowds than in the EPL, but have had less time to adjust to high-intensity games played in front of many supporters. It would be interesting to examine referees' hormone and stress levels, as has been done with players, to see if there are general characteristics of referees with high and low home advantage and to see how hostile crowds, different outcomes, and difficult decisions influence referees physiologically. This factor could be used to aid officials in identifying their own potential biases and correcting for them. From a competition standpoint, our results validate the use of more experienced officials for more well-attended games, particularly important games that are not played as a home-and-home series and which therefore present a greater opportunity for biased results.
Our results indicate that referee and crowd size both significantly influence home advantage, but the data are insufficient to infer the precise dependencies between these two variables. The findings are consistent with several scenarios: (1) referees vary in their response to a partisan crowd, but not in their marginal response to crowd size; (2) referees vary in their marginal response to crowd size, but not in their baseline response to the presence of a partisan crowd; or (3) referees differ in both their baseline and marginal response to crowd size. Furthermore, the identity of the home team is correlated with crowd size and thus confounds the effect of crowd size. After controlling for home team, crowd size was no longer significant, suggesting the relationship between crowd size and home advantage is not as straightforward as is often assumed.
The distribution of referee home advantages fits with a model assuming a relatively high baseline home advantage shared by most referees from which a few deviate by giving lower home advantage. The fact that some referees can systematically give less home advantage, as seen by the long tail on the low end of the distribution, is evidence that referees can control the amount of home advantage they give, and that most referees who deviate from the mode do so in the direction of giving less home advantage. To achieve the observed distribution, either home teams perform better and most referees are unbiased with a few referees biasing their calls for the away team, or home teams do not perform much better but most referees give them the edge. Certainly, the latter seems more reasonable, especially given the observed floor near gD = 0 (no home advantage). The referee represented by the outlier is also a cause for concern: referees with much higher home advantages than the league average may cause the public to doubt the fairness of officials in general and allow league administrators to systematically favour particular teams. Although this outlier drives the significance of the referee effect on goal differential, he does not affect the findings that more experienced referees give significantly less home advantage and that different referees give significantly different amounts of home advantage in yellow cards and penalties. Since these are more sensitive measures of home advantage, we can be confident that referees do systematically differ in the home advantage they give and that most referees cluster around a high level of home advantage with a few giving less and one giving much more.
These results also suggest mechanisms by which referees influence the outcome. Referees exhibit significantly different home advantages in penalties and yellow cards, two potentially game-changing factors. Since different referees also give significantly different total numbers of yellow cards, red cards, and penalties, our intuition that these are subjective decisions is confirmed. This finding suggests one way of assessing officiating bias as a cause of home advantage across different sports: by assessing the extent to which certain calls differ by official, and the importance of each kind of call to game outcome, researchers could determine the relative subjectivity of officiating different sports and how much the measure correlates with the observed home advantage. Examining home advantage through specific subjective decisions would provide a more sensitive measure of home advantage, since referees have a greater ability to bias these decisions than the actual match result.
Although officiating bias is recognized as an important component of the home advantage in many sports, it is not included in Carron and colleagues' (2005) conceptual framework for home advantage research in part because officials do not have “home” or “away” status and thus it is difficult to test for relationships between officials and home advantage. Our novel statistical approach addresses this concern, and the presence of individual variation in officiating bias suggests that officials should be reinstated into Courneya and Carron's (Citation1992) conceptual model.
An alternative application of our individual-variation regression model of home advantage would be to look for variation in home advantage between competitors instead of between officials by including player terms instead of a referee term. A significant player effect would indicate that competitors are also responsible for some of the observed home advantage. Such studies could also allow for explicit comparison of the variability of player and referee home advantages by including both referee and player terms.
One potential complication of our method is the need to control for team ability. We chose to use season scoring and card averages, although more complex methods may ultimately prove to be better predictors of game outcome. However, our results are robust to how we controlled for team ability. In fact, excluding the team ability metrics from the models leads to nearly identical results (data not shown), suggesting that referees were assigned games nearly randomly with regard to team abilities and that over 25+ games, differences in ability largely cancelled out for each referee. Nevertheless, our team ability metrics do demonstrate some interesting trends. As expected, scoring increased when a team's xGF (expected goals for) or the opposition's xGA (expected goals against) was high, but it also increased when xGA or the opposition's xGF was low. This indicates offence and defence are not two separate entities, but are both correlated to some fundamental team ability factor. Yellow cards were not just correlated to expected cards for, but also to the opposition's expected cards against, suggesting that cards are not just a consequence of how “dirty” a team plays, but also how well a team can spur the opposition into drawing a card. In fact, our model of red cards suggests the latter is a stronger influence than the former.
Most commentators accept home advantage as a legitimate and fair part of the game, presumably because most people assume that the crowd's main effect is to inspire their players to perform better. While subconscious referee bias does not necessarily make home advantage unfair, our finding of significant variation in home advantage by referee is hard to accept as fair. It has not escaped our notice that league administrators could influence games through referee assignments, although it should be noted that inter-referee variation has only a slight influence on game outcome and requires large datasets to detect. Leagues could also systematically increase or decrease their overall home advantage by choosing which referees remain active each year. Thus, one might predict that leagues might preferentially assign referees with relatively high home advantages if home wins increased attendance and thus revenue; however, the English Premier League, at least, does not appear to be doing so, as their referees with the longest tenures have the lowest home advantages. Leagues could minimize variability in referee-mediated home advantage in two ways: increasing their training of officials and decreasing the reliance on subjective decisions wherever possible. Home advantage decreases with referee experience and may decrease faster if referees are made aware of their role in providing the home advantage. Nevill and co-workers' (Citation1999, Citation2002) results suggest that neutral assessors watching games on muted videotape may also help identify biased decisions. While certain sports, such as football, will always have many subjective officiating decisions, employing technology such as goal line cameras may help a league reduce home advantage. Such technologies could play an important role in promoting fairness, especially in important international competitions such as the World Cup Finals, where there are no home-and-home series.
Home advantage is a commonly accepted phenomenon in most sports. While most researchers have focused on factors influencing players, referee bias has been shown by a number of recent experiments to be a plausible cause of the home advantage. Our results demonstrate that different referees provide significantly different levels of home advantage and show that variation in home bias of subjective officiating decisions is likely responsible. Research examining the psychological effects of biased crowds on referees is needed to understand the mechanisms underlying home advantage and how biased environmental pressures influence decision makers generally.
Acknowledgements
We appreciate the helpful comments of M.E. Boyko, F.A. Cushman, D.R. Feinberg, J.R. Lucas, and two anonymous reviewers on earlier drafts of the manuscript.
References
- Agnew , G. A. and Carron , A. V. 1994 . Crowd effects and the home advantage . International Journal of Sport Psychology , 25 : 53 – 62 .
- Balmer , N. J. , Nevill , A. M. and Lane , A. 2005 . Do judges enhance home advantage in European championship boxing? . Journal of Sport Sciences , 23 : 409 – 416 .
- Balmer , N. J. , Nevill , A. M. and Williams , A. M. 2001 . Home advantage in the Winter Olympics (1908 – 1998) . Journal of Sports Sciences , 19 : 129 – 139 .
- Balmer , N. J. , Nevill , A. M. and Williams , A. M. 2003 . Modelling home advantage in the Summer Olympic Games . Journal of Sports Sciences , 21 : 469 – 478 .
- Barnett , V. and Hilditch , S. 1993 . The effect of an artificial pitch surface on home performance in football (soccer) . Journal of the Royal Statistical Society , 156 : 39 – 50 .
- Bray , S. R. and Widmeyer , W. N. 2000 . Athletes' perceptions of the home advantage: An investigation of perceived causal factors . Journal of Sport Behavior , 23 : 1 – 10 .
- Carron , A. V. , Loughhead , T. M. and Bray , S. R. 2005 . The home advantage in sport competitions: Courneya and Carron's (1992) conceptual framework a decade later . Journal of Sports Sciences , 23 : 395 – 407 .
- Clarke , S. R. 2005 . Home advantage in the Australian football league . Journal of Sports Sciences , 23 : 375 – 385 .
- Clarke , S. R. and Norman , J. M. 1995 . Home ground advantage of individual clubs in English soccer . The Statistician , 44 : 509 – 521 .
- Courneya , K. S. and Carron , A. V. 1991 . Effects of travel and length of home stand/road trip on the home advantage . Journal of Sport and Exercise Psychology , 13 : 42 – 49 .
- Courneya , K. S. and Carron , A. V. 1992 . The home advantage in sport competitions: A literature review . Journal of Sport and Exercise Psychology , 14 : 13 – 27 .
- Jurkovac , T. 1985 . Collegiate basketball players' perceptions of the home advantage , Bowling Green, OH : Bowling Green State University . Unpublished master's thesis
- Kerr , J. H. and Vanschaik , P. 1995 . Effects of game venue and outcome on psychological mood states in rugby . Personality and Individual Differences , 19 : 407 – 410 .
- Koning , R. 2005 . Home advantage in speed skating: Evidence from individual data . Journal of Sports Sciences , 23 : 417 – 427 .
- The Independent . 1994 . “ Managers fear flood of red cards after rule changes ” . In The Independent 31 London 9 August 1994, Sports
- McGuire , E. J. , Courneya , K. S. , Widmeyer , W. N. and Carron , A. V. 1992 . Aggression as a potential mediator of the home advantage in professional ice hockey . Journal of Sport and Exercise Psychology , 14 : 148 – 158 .
- Neave , N. and Wolfson , S. 2003 . Testosterone, territoriality, and the “home advantage” . Physiology and Behavior , 78 : 269 – 275 .
- Nevill , A. , Balmer , N. and Williams , M. 1999 . Crowd influence on decisions in association football . Lancet , 353 : 1416
- Nevill , A. M. , Balmer , N. J. and Williams , A. M. 2002 . The influence of crowd noise and experience upon refereeing decision in football . Psychology of Sport and Exercise , 3 : 261 – 272 .
- Nevill , A. M. and Holder , R. 1999 . Home advantage in sport: An overview of studies on the advantage of playing at home . Sports Medicine , 28 : 221 – 236 .
- Nevill , A. M. , Newell , S. M. and Gale , S. 1996 . Factors associated with home advantage in English and Scottish football . Journal of Sports Sciences , 14 : 181 – 186 .
- Pace , A. and Carron , A. V. 1992 . Travel and the home advantage . Journal of Sports Sciences , 17 : 60 – 64 .
- Pollard , R. 1986 . Home advantage in football: A retrospective analysis . Journal of Sports Sciences , 4 : 237 – 248 .
- Pollard , R. 2006 . Worldwide regional variations in home advantage in association football . Journal of Sports Sciences , 24 : 231 – 240 .
- Pollard , R. and Pollard , G. 2005 . Long-term trends in home advantage in professional sports in North America and England (1876 – 2003) . Journal of Sports Sciences , 23 : 337 – 350 .
- SAS Institute, Inc. 1999 . SAS/STAT user's guide, Version 7 , Cary, NC : SAS Institute Inc. .
- Sutter , M. and Kochera , M. G. 2004 . Favoritism of agents – the case of referees' home bias . Journal of Economic Psychology , 25 : 461 – 469 .
- Thirer , J. and Rampey , M. 1979 . Effects of abusive spectator behaviour on the performance of home and visiting intercollegiate basketball teams . Perceptual and Motor Skills , 48 : 1047 – 1053 .