ABSTRACT
The present study cross-validated various cut-points to assess physical activity and sedentary behaviour in preschoolers, using hip- and wrist-worn accelerometers and both vertical axis and vector magnitude data. Secondly, we examined the influence of epoch length on time estimates of physical activity and sedentary behaviour. Sixty-four preschoolers (34 girls) wore two accelerometers, on their right hip and dominant wrist, during 1 hour of free play. Preschoolers’ activities were observed by two trained researchers. Area under the curve (AUC) was calculated for the receiving operating characteristic (ROC) curves as a measure of precision. AUC ranges were 0.603–0.723 for sedentary behaviour, 0.472–0.545 for light physical activity and 0.503–0.661 for moderate-to-vigorous physical activity (MVPA), indicating poor to fair precision. Percentage of time classified as sedentary behaviour, light or MVPA according to observation and accelerometer data varied largely between cut-points, accelerometer placements and axes. The influence of epoch length on time estimates was minimal across cut-points, except for one hip-based vector magnitude cut-point. Across all accelerometer placements and data axes, no set of cut-points demonstrated adequate precision for sedentary behaviour, light physical activity and MVPA. The highly variable and omnidirectional activity pattern of preschoolers may explain the lack of adequate cut-points.
Introduction
Accelerometers are widely used to estimate time spent in physical activity and sedentary behaviour in children. Such device-based measurement of physical activity and sedentary behaviour is particularly important in preschool children as their sporadic activity pattern is difficult to assess by proxy-report (Oliver et al., Citation2007). Previous studies have demonstrated the feasibility of using accelerometers in preschool children (Cliff et al., Citation2009; Van Cauwenberghe et al., Citation2011). To give biological meaning to accelerometer output, acceleration data is processed by a number of subjective decisions. Second-by-second, activity data is aggregated in specified epoch lengths and cut-points are applied to provide time estimates of physical activity and sedentary behaviour at different intensities. The use of cut-points is not without limitations and therefore new approaches to analyse accelerometer data are emerging. One alternative approach is pattern recognition such as machine learning models that predict the intensity of physical activity or activity types (e.g., walking, running) (Ahmadi, Chowdhury et al., Citation2020; Ahmadi, Pavey et al., Citation2020). Another approach is the application of alternative accelerometer metrics such as average acceleration (indicating the volume of physical activity), intensity gradient (indicating the intensity profile) and the minimum acceleration value above which a person’s most active X minutes are accumulated (indicating the volume and intensity of physical activity) (Rowlands, Citation2018; Rowlands et al., Citation2019). Although these new approaches may be an important step forward, they currently lack translatability to public health practice. Therefore, until more user-friendly tools are available for the application of above mentioned approaches, the application of cut-points will remain in use to classify time spent in sedentary behaviour, light-, moderate- and vigorous intensity physical activity (Chinapaw et al., Citation2019). Hence, calibration and cross-validation of age-specific cut-points remains important.
A number of studies have calibrated cut-points for preschool children based on hip-placed accelerometers, using the accelerometer data of the vertical axis (Costa et al., Citation2014; Johansson et al., Citation2016; Pate et al., Citation2006; Reilly et al., Citation2003; Sirard et al., Citation2005; Van Cauwenberghe et al., Citation2011), resulting in a wide range of cut-points (from 60 (Costa et al., Citation2014) to 1488 (Van Cauwenberghe et al., Citation2011) counts per minute for sedentary behaviour and 1680 (Pate et al., Citation2006) to 3564 (Sirard et al., Citation2005) counts per minute for moderate-to-vigorous physical activity (MVPA)). Studies cross-validating these established cut-points in a different sample of same-aged preschool children showed considerable differences in measures assessing precision (i.e., area under the curve (AUC), sensitivity and specificity) (Costa et al., Citation2014; Janssen et al., Citation2013; Kahan et al., Citation2013; Reilly et al., Citation2003). Moreover, observational studies demonstrated considerable differences in time estimates of free-living physical activity and sedentary behaviour in same-aged preschool children when applying different cut-points (Cliff & Okely, Citation2007; Cliff et al., Citation2009; Reilly et al., Citation2008; Van Cauwenberghe et al., Citation2011).
It should be noted that calibration, cross-validation and observational studies vary considerably in their design, potentially contributing to differences in measures of precision for a specific cut-point and differences in time estimates of physical activity and sedentary behaviour across different cut-points. In most calibration studies preschool children mainly performed structured activities whereas in cross-validation and observational studies free living activities were performed. Additionally, different comparison methods are used, including indirect calorimetry (Colley et al., Citation2013; Pate et al., Citation2006) and direct observation (Costa et al., Citation2014; Reilly et al., Citation2003; Sirard et al., Citation2005; Van Cauwenberghe et al., Citation2011). Another explanation for the large differences in measures of precision between cross-validation studies may be differences in the analysis of accelerometer data. When calibrating and cross-validating cut-points, two decisions are especially important. The first is the epoch length in which the data is aggregated. Because of the sporadic activity pattern on preschool children, a short epoch duration may best capture preschool children’s levels of physical activity and sedentary behaviour (Oliver et al., Citation2007). During 1 hour free play, Hislop et al. (Citation2014) demonstrated a smaller underestimation in preschool children’s MVPA with shorter epoch lengths (i.e. 15- and 30-s versus 60-s epochs; hip-based accelerometer data) compared to direct observation, using the adapted Children’s Activity Rating Scale (CARS) that allowed observations in 15-s epochs (Sirard et al., Citation2005). Additionally, observational studies examining the influence of epoch length demonstrated larger time estimates of habitual physical activity in preschool children when analysing hip-based accelerometer data in 5-s (Vale et al., Citation2009) and 15-s (Leeger-Aschmann et al., Citation2019; Vanderloo et al., Citation2016) versus 60-s epochs. However, Leeger-Aschmann et al. (Citation2019) demonstrated similar time estimates of sedentary behaviour based on 15-s versus 60-s hip-based accelerometer data. A second important decision in calibrating and cross-validating cut-points is the choice of using data from the vertical axis or data from all three axes (vertical, anterior-posterior and medio-lateral) combined in a vector magnitude score. Due to their rapid social and physical development, preschool children’s activity pattern is very different than that of older children, requiring less vertical movement and more omnidirectional movement (i.e. rolling and climbing) (Oliver et al., Citation2007). Previous studies showed that using each axis individually adds information over the use of the vector sum of the three axes in the association with metabolic health in 10-year-old children (Aadland et al., Citation2019), and using vertical axis data reveals strongest associations with fundamental motor skills in preschoolers (Nilsen et al., Citation2020). Nevertheless, vector magnitude data may better capture preschoolers’ activity pattern than vertical axis data. Despite this, the majority of studies have predominantly relied on data from the vertical axis, potentially leading to erroneous time estimates of physical activity. To date, only three studies calibrated accelerometer physical activity cut-points using both vertical axis data and the vector magnitude data in preschool children (Butte et al., Citation2014; Dobell et al., Citation2019; Johansson et al., Citation2016). Two studies demonstrated comparable precision for the classification of sedentary behaviour, light physical activity and MVPA using vector magnitude versus vertical axis data, for hip-placed accelerometers in 3-5-year-old pre-schoolers (Butte et al., Citation2014) and both hip- and wrist-placed accelerometers in 3-4-year-old pre-schoolers (Dobell et al., Citation2019). However, Johansson et al. (Citation2016) demonstrated a higher precision for classifying MVPA when using vector magnitude data versus vertical axis data, for both hip- and wrist-placed accelerometers in 4-year-old preschool children.
To the best of our knowledge, no previous studies have cross-validated cut-points for vertical axis and vector magnitude data in a sample of preschool children. The aim of this study was to cross-validate various cut-points for assessing physical activity and sedentary behaviour in preschool children during 1 hour of free play in their natural preschool setting, using vertical axis and vector magnitude data of both hip- and wrist-worn accelerometers. Accelerometer data were cross-validated against direct observations using the Observational System of Recording Physical Activity in Children-Preschool Version (OSRAC-P). Secondly, we examined the influence of epoch length (i.e., 5, 15, 30 s) on time estimates of physical activity and sedentary behaviour.
Materials and methods
Sample and study design
We recruited a convenience sample of preschool children from preschools in the Netherlands, located in both rural and urban areas. Preschools received written information about the study via email and were contacted again about one week later. Of all invited preschools (n = 9), seven preschools agreed to participate in this observational validation study. Due to practical reasons, one preschool could not participate, resulting in six participating preschools. All preschool children who were able to perform play activities that were common for a child of his/her age were eligible to participate in this study. In total, 64 preschool children (2–4 years old; 34 girls) were included in the present study after their parents had given written informed consent. The ethical committee of the VU Medical Centre approved this study and decided that the Medical Research Involving Human Subjects Act (WMO) did not apply to this study (2017.534).
Procedures
Two researchers conducted all measurements between February and April 2018 at the preschools. Before data collection, the two researchers were trained to use the OSRAC-P, consisting of studying OSRAC-P protocols, practicing video’s and discussing coding definitions (in total 16 hours of training per researcher). Additionally, the two researchers conducted a pilot study (see below) in which they further increased their competence of data collection using the OSRAC-P. The preschool staff indicated which moments and which preschool children were available for observations during preschool hours, thereby excluding napping time. An observation never started during snack- and mealtimes, but in a few cases such moments were included in the last 10–15 minutes of an observation period. Observation periods included both indoor and outdoor play hours. At each observation moment, first, informed consent forms and parent-reported data on preschool children’s height, weight, birthdate and sex were obtained. Next, preschool children were equipped with two ActiGraph GT3X+ accelerometers, one at the right hip and one at the dominant wrist, using elastic bands. Subsequently, the preschool children were explained that they could go back to their normal play activities. After 15 minutes, during which the preschool children could become familiar with the accelerometers and the presence of the researchers, the researchers observed the children during 1 hour of free play, using the OSRAC-P. In line with guidelines for administration, a maximum of two preschool children at a time were observed, i.e. one child by each researcher. Before starting the observation, researchers carefully noted the starting time using the computer at which the accelerometers were initialized, to allow alignment of the observation data with the accelerometer data. After completion of the measurement, the accelerometers were removed and the preschool children received a small incentive as a thank you for participation.
Measurement of physical activity and sedentary behaviour
The ActiGraph GT3X accelerometer (Pensacola, Florida, USA) was used in the present study, applying a 100-Hz sampling rate and both data from the vertical axis and vector magnitude. Subsequently, data were transferred into 5-, 15- and 30-s epochs. provides an overview of the cut-points for vertical axis data and vector magnitude data of wrist- and hip-worn accelerometers that were applied in the current study (Dobell et al., Citation2019; Johansson et al., Citation2016; Pate et al., Citation2006; Sirard et al., Citation2005). Cut-points were scaled to match the different epoch lengths.
Table 1. Hip- and wrist-based accelerometer cut-points for vertical axis and vector magnitude data, scaled to different epoch lengthsa.
The OSRAC-P is a direct observation method allowing assessment of intensity-level categories as introduced in the Children’s Activity Rating Scale (CARS; Puhl et al., Citation1990) as well as other behavioural, social and contextual information such as the type and location of the activity, who prompted the activity and the group composition (Brown et al., Citation2006). The OSRAC-P uses a 5-s observation/25-s recording interval, which was prompted by a custom-made audio fragment, indicating that the first 5 s of each recording interval was used for analysis. For the current study, researchers only classified preschool children’s intensity level in five levels, i.e. 1-stationary or motionless, 2-stationary with limb or trunk movement, 3-slow easy movements, 4-moderate movements and 5-fast movements (Brown et al., Citation2006). The first two categories were combined into a sedentary-category, while the latter two were combined into a MVPA-category, as recommended by others (Brown et al., Citation2006; Pate et al., Citation2008). The classification “can’t tell” was given when the researchers could not observe the preschool child because he/she was out of sight; these intervals were coded as missing. For example, when a child went to the lavatory or had to change clothes. For the epochs with missing observation data, the accompanying data from the accelerometers was also removed.
Before the start of this cross-validation study, a pilot study was conducted among slightly older children to assess the interobserver reliability of the OSRAC-P assessment. Eleven young children, recruited from the personal network of the researchers, aged 2–6 years (eight girls) participated in this pilot study. Observations took place during 1 hour of free play in the home setting of the young children. When two or more children participated at the same time, video-recordings were obtained to enable assessment afterwards. The intraclass correlation coefficient (ICC) was 0.83, indicating excellent interobserver reliability (Cicchetti, Citation1994; Landis & Koch, Citation1977).
Statistics
Descriptives of demographic information (means ± SD) were calculated. For the cross-validation of cut-points, accelerometer data in 30-s epochs were used to match the OSRAC-P observation interval. The area under the curve (AUC) was calculated using receiving operating characteristic (ROC) curve analysis (Jago et al., Citation2007), representing a measure of precision. An AUC of under 0.70 is considered as poor, 0.70–0.79 fair, 0.80–0.89 good and 0.90–1.0 excellent (Hanley & McNeil, Citation1982). To obtain an indication of the bias in time estimates, average time (in minutes; and 95% confidence intervals (95% CI)) classified as sedentary, light physical activity or MVPA was calculated for observational data and accelerometer data, representing a measure of accuracy. Analyses were performed in SPSS version 24 (SPSS Inc, Chicago, IL, USA).
Results
Complete observation data were obtained for 63 preschool children (53% girls) who were on average 2.7 ± 0.5 years old. According to the Cole criteria (Cole et al., Citation2000), 16% of the preschool children were overweight (n = 8) and 6% obese (n = 3). In total 7281 (out of 7560) epochs were included in the analysis.
presents the precision of assessing physical activity and sedentary behaviour using different hip- and wrist-based accelerometer cut-points for vertical axis and vector magnitude data, in 30-s epochs. Overall, the precision of classifying physical activity and sedentary behaviour was poor to fair across all cut-points, accelerometer placements and data axes. The precision of assessing sedentary behaviour was fair for almost all cut-points, accelerometer placements and data axes, with AUCs ranging from 0.603 (Sirard et al., Citation2005, hip-based VA data) to 0.723 (Dobell et al., Citation2019 and Butte et al., Citation2014, hip-based vector magnitude). The precision of assessing light physical activity was poor, with AUCs ranging from 0.472 (Johansson et al., Citation2016, hip-based vector magnitude) to 0.545 (Dobell et al., Citation2019, wrist-based vector magnitude). For MVPA, only the Dobell et al. (Citation2019) hip-based vector magnitude cut-point approached fair precision (AUC: 0.661).
Table 2. Precision of different hip- and wrist-based accelerometer cut-points for vertical axis and vector magnitude data in preschoolers (30-s epochs)
and Supplementary Table 1 show the time (in minutes) classified as sedentary, light or MVPA according to observation and accelerometer data, based on different accelerometer placements, data axes and cut-points. According to direct observation data, preschool children spend on average 77% of time sedentary, 16% of time in light physical activities and 5% of time in MVPA. Compared to direct observation, all hip- and wrist-based accelerometer data underestimated sedentary time and overestimated light physical activity, except for the hip-based Sirard et al. (Citation2005) cut-points (vertical axis). Time classified in MVPA was comparable to direct observation data for the Sirard et al. (Citation2005) cut-point (hip-based vertical axis), but was underestimated in all Johansson et al. (Citation2016) cut-points and overestimated in all other cut-points, irrespective of accelerometer placements and data axes.
Figure 1. Time (min) classified as sedentary, light or moderate-to-vigorous physical activity, according to different cut-points and based on hip vertical axis, hip vector magnitude and wrist vector magnitude data (30-s epochs) in preschoolers. SB, sedentary behaviour; min, minute; MVPA, moderate-to-vigorous physical activity; LPA, light-intensity physical activity.
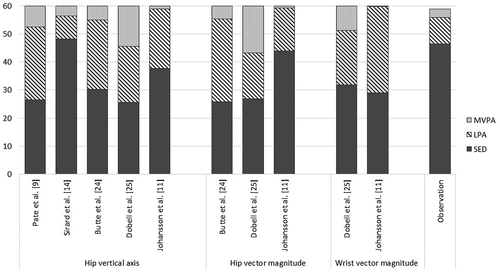
and Supplementary Table 1 show the time (in minutes) classified as sedentary, light or MVPA according to accelerometer data analysed in 5-, 15- and 30-s epochs, for all accelerometer placements, data axes and cut-points. Time estimates of sedentary behaviour and physical activity were comparable across epoch lengths and cut-points, except for the hip-based vector magnitude cut-point of Johansson et al. (Citation2016). Using this cut-point, time estimates of sedentary behaviour were lower using the 30-s epoch (43.9 minutes) compared to the 5- (32.4 minutes) and 15-s (33.1 minutes) epochs, and time estimates of light physical activity were higher using the 30-s epoch (19.4 minutes) compared to the 5-s (22.3 minutes) and 15-s (21.5 minutes) epochs. Time estimates for MPVA were comparable across epoch lenghts using the hip-based vector magnitude cut-point of Johansson et al.(Johansson et al., Citation2016).
Figure 2. Influence of epoch duration (5-, 15- and 30-s) on time (in min) classified as sedentary, light or moderate-to-vigorous physical activity, according to different cut-points and based on hip vertical axis (A), hip vector magnitude (B) and wrist vector magnitude (C) data of preschoolers.
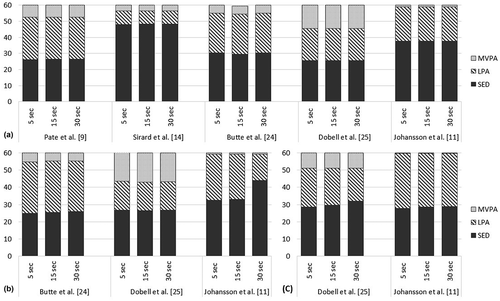
Discussion
This is the first study that cross-validated various cut-points to assess physical activity and sedentary behaviour in preschool children during 1 hour of free play in a natural setting, using both hip- and wrist-worn accelerometers and both vertical axis and vector magnitude data. The current study therefore provides a comprehensive overview of the utility of existing accelerometer cut-points to assess physical activity and sedentary behaviour in preschoolers. Across all accelerometer placements and data axes, the precision for assessing physical activity and sedentary behaviour was poor to fair. Additionally, we demonstrated that time estimates of physical activity and sedentary behaviour varied substantially across accelerometer placements and data axes. Taking into account both sedentary behaviour and physical activity at different intensities, the hip-based Sirard et al. (Citation2005) cut-points (vertical axis) resulted in the smallest deviations in time estimates compared to direct observations, i.e., indicating the highest accuracy. Time estimates of sedentary behaviour and physical activity varied hardly across epochs (i.e., 5, 15 and 30 s), independent of accelerometer placement and data axis.
Our finding that, during 1 hour of free play, preschoolers spent on average 79% of their time sedentary, 16% in light physical activity and 5% in MVPA was in line with previous direct observation studies reporting that preschool children spent between 50% and 89% of time in sedentary bahaviour, between 8% and 36% in light physical activity and between 3% and 20% in MVPA (Bailey et al., Citation1995; Hnatiuk et al., Citation2014; Van Cauwenberghe et al., Citation2011).
Overall, the precision of cut-points for estimating sedentary behaviour was fair, across all accelerometer placements and data axes. Nevertheless, time estimates varied substantially compared to direct observation, underestimating the time spent in sedentary behaviour. Only the hip-based vertical axis cut-point of Sirard et al. (Citation2005) provided time estimates of sedentary behaviour that were similar to direct observation across epoch lengths, i.e., 48.2 and 46.4 minutes, respectively. For MVPA, only the hip-based vector magnitude cut-point of Dobell et al. (Citation2019) approached fair precision, yet time classified in MVPA was overestimated using this cut-point compared to direct observation (i.e., 16.9 versus 3 minutes MVPA, respectively). For light physical activity, in line with the poor precision across cut-points, time estimates varied substantionally with all cut-points overestimating time spent in light physical activity, except for the Sirard et al. (Citation2005) cut-points that slightly underestimated time spent in light physical activity. The minimal difference between time estimates of physical activity across epoch lengths is in contrast with previous studies in preschoolers (Leeger-Aschmann et al., Citation2019; Vanderloo et al., Citation2016). One explanation could be the limited observation time (1 hour) and as a result little variation in activities during the observation period of free play.
Similar to our findings, Janssen et al. (Citation2013) found higher precision for hip-based vertical axis cut-points classifying sedentary behaviour than for cut-points classifying light physical activity and MVPA. However, in the study of Janssen et al. (Citation2013) higher precision was demonstrated, with AUCs ranging from 0.64 (Sirard et al., Citation2005) to 0.80 (Evenson et al., Citation2008) for sedentary behaviour, from 0.50 (Sirard et al., Citation2005) to 0.65 (Evenson et al., Citation2008)) for light physical activity and from 0.62 (Sirard et al., Citation2005) to 0.72 (Pate et al. Citation2006) for MVPA. In contrast, Kahan et al. (Citation2013) demonstrated higher precision for cut-points classifying MVPA compared to cut-points classifying sedentary behaviour, with the highest precision using the MVPA cut-point of Sirard et al. (Citation2005) and the sedentary cut-point of Pate et al. (Citation2006).
Our findings indicate that there is currently no set of hip-based vertical axis cut-points that is adequate for assessing sedentary behaviour, light physical activity and MVPA. The present study additionally indicates that, despite slightly higher AUCs, cut-points using hip- or wrist-based vector magnitude data cut-points do not result in more precise classifications of sedentary behaviour, light physical activity and MVPA compared to classifications using hip-based vertical axis data. The lack of adequate cut-points may be explained by the highly variable and omnidirectional activity pattern of preschoolers, and emphazises the need for more advanced analysis approaches as well as accompanying user-friendly tools for the application of such approaches in practice. In the meantime, researchers could capture the various intensities of preschoolers’ physical activity using the whole intensity spectrum, e.g., by applying the multivariate physical activity signature (Nilsen et al., Citation2020). If researchers insist on classifying the intensity of physical activity in preschoolers, based on the present findings, we recommend using the Sirard et al. (Citation2005) vertical axis cut-points, as these cut-points provided the smallest deviations in time estimates (i.e., highest accuracy). Yet as these cut-points were not adequate in terms of precision, results should be carefully interpreted.
Strengths of this study include the relatively large sample size and the excellent inter-observer reliability of the two researchers who conducted all observations. Moreover, the cross-validation of cut-points for sedentary behaviour and physical activity using both hip-based (vertical axis) and wrist-based (vertical axis and vector magnitude) accelerometer data strengthens this study. Finally, preschool children were observed during 1 hour of free play in their natural environment (i.e., daycare centre), thereby approaching their natural, everyday behaviour. A limitation of this study that the OSRAC-P is limited to a 5-s observation and 25-s recording interval, assuming that preschool children do not change their intensity of physical activity during the recording period. Though previous studies have demonstrated good validity and reliability of the OSRAC-P (Oliver et al., Citation2007), a shorter recording interval is necessary to cross-validate accelerometer data in shorter epochs, to capture the sporadic activity pattern of preschoolers. This indicates that the OSRAC-P might not be the most adequate criterion instrument when evaluating measurement instruments in young children. As a result, a 5-s epoch was the shortest duration we could include in our study. Finally, we only observed one hour of free play in the preschool setting, which may not have captured the wide range of activities that preschool children perform throughout the day, thereby reducing the generalizability of our findings.
We conclude that, across all accelerometer placements and data axes, there is currently no set of cut-points with fair validity to classify sedentary behaviour, light physical activity and MVPA in preschool children. Therefore, we recommend using cut-point free analyses methods of accelerometer data and for now refrain from categorizations into sedentary behaviour, light physical activity and MVPA.
Supplemental Material
Download MS Word (55.6 KB)Acknowledgments
We would like to thank all participating preschools and parents of participating children for enabling the current study.
Disclosure statement
No potential conflict of interest was reported by the authors.
Supplementary material
Supplemental data for this article can be accessed online https://doi.org/10.1080/02640414.2021.1994726.
Additional information
Funding
References
- Aadland, E., Kvalheim, O. M., Anderssen, S. A., Resaland, G. K., & Andersen, L. B. (2019). The triaxial physical activity signature associated with metabolic health in children. Medicine & Science in Sports & Exercise, 51(10), 2173–2179. https://doi.org/https://doi.org/10.1249/MSS.0000000000002021
- Ahmadi, M. N., Chowdhury, A., Pavey, T., Trost, S. G., & Schubert, M. M. (2020). Laboratory-based and free-living algorithms for energy expenditure estimation in preschool children: A free-living evaluation. PLoS One, 15(5), e0233229. https://doi.org/https://doi.org/10.1371/journal.pone.0233229
- Ahmadi, M. N., Pavey, T. G., & Trost, S. G. (2020). Machine learning models for classifying physical activity in free-living preschool children. Sensors (Basel), 20(16), 16. https://doi.org/https://doi.org/10.3390/s20164364
- Bailey, R. C., Olson, J., Pepper, S. L., Porszasz, J., Barstow, T. J., & Cooper, D. M. (1995). The level and tempo of children’s physical activities: An observational study. Medicine & Science in Sports & Exercise, 27(7), 1033–1041. https://doi.org/https://doi.org/10.1249/00005768-199507000-00012
- Brown, W. H., Pfeiffer, K. A., McLver, K. L., Dowda, M., Almeida, M. J., & Pate, R. R. (2006). Assessing preschool children’s physical activity: The observational system for recording physical activity in children-preschool version. Research Quarterly for Exercise and Sport, 77(2), 167–176.
- Butte, N. F., Wong, W. W., Lee, J. S., Adolph, A. L., Puyau, M. R., & Zakeri, I. F. (2014). Prediction of energy expenditure and physical activity in preschoolers. Medicine & Science in Sports & Exercise, 46(6), 1216–1226. https://doi.org/https://doi.org/10.1249/MSS.0000000000000209
- Chinapaw, M. J., Wang, X., Andersen, L. B., & Altenburg, T. M. (2019). From total volume to sequence maps: sophisticated accelerometer data analysis. Medicine & Science in Sports & Exercise, 51(4), 814–820. https://doi.org/https://doi.org/10.1249/MSS.0000000000001849
- Cicchetti, D. V. (1994). Guidelines, criteria and rules of thumb for evaluating normed and standardized assessment instruments in psychology. Psychological Assessment, 6(4), 282–290. https://doi.org/https://doi.org/10.1037/1040-3590.6.4.284
- Cliff, D. P., & Okely, A. D. (2007). Comparison of two sets of accelerometer cut-off points for calculating moderate-to-vigorous physical activity in young children. Journal of Physical Activity and Health, 4(4), 509–513. https://doi.org/https://doi.org/10.1123/jpah.4.4.510
- Cliff, D. P., Reilly, J. J., & Okely, A. D. (2009). Methodological considerations in using accelerometers to assess habitual physical activity in children aged 0-5 years. Journal of Science and Medicine in Sport, 12(5), 557–567. https://doi.org/https://doi.org/10.1016/j.jsams.2008.10.008
- Cole, T. J., Bellizzi, M. C., Flegal, K. M., & Dietz, W. H. (2000). Establishing a standard definition for child overweight and obesity worldwide: International survey. BMJ, 320(7244), 1240–1243. https://doi.org/https://doi.org/10.1136/bmj.320.7244.1240
- Colley, R. C., Garriguet, D., Adamo, K. B., Carson, V., Janssen, I., Timmons, B. W., & Tremblay, M. S. (2013). Physical activity and sedentary behavior during the early years in Canada: A cross-sectional study. International Journal of Behavioral Nutrition and Physical Activity, 10(1), 54. https://doi.org/https://doi.org/10.1186/1479-5868-10-54
- Costa, S., Barber, S. E., Cameron, N., & Clemes, S. A. (2014). Calibration and validation of the ActiGraph GT3X+ in 2-3 year olds. Journal Of Science And Medicine In Sport / Sports Medicine Australia, 17(6), 617–622.
- Dobell, A. P., Eyre, E. L. J., Tallis, J., Chinapaw, M. J. M., Altenburg, T. M., & Duncan, M. J. (2019). Examining accelerometer validity for estimating physical activity in pre-schoolers during free-living activity. Scandinavian Journal of Medicine & Science in Sports, 29(10), 1618–1628. https://doi.org/https://doi.org/10.1111/sms.13496
- Evenson, K. R., Catellier, D. J., Gill, K., Ondrak, K. S., & McMurray, R. G. (2008). Calibration of two objective measures of physical activity for children. Journal of Sports Sciences, 26(14), 1557–1565. https://doi.org/https://doi.org/10.1080/02640410802334196
- Hanley, J. A., & McNeil, B. J. (1982). The meaning and use of the area under a receiver operating characteristic (ROC) curve. Radiology, 143(1), 29–36. https://doi.org/https://doi.org/10.1148/radiology.143.1.7063747
- Hislop, J., Law, J., Rush, R., Grainger, A., Bulley, C., Reilly, J. J., & Mercer, T. (2014). An investigation into the minimum accelerometry wear time for reliable estimates of habitual physical activity and definition of a standard measurement day in pre-school children. Physiological Measurement, 35(11), 2213–2228. https://doi.org/https://doi.org/10.1088/0967-3334/35/11/2213
- Hnatiuk, J. A., Salmon, J., Hinkley, T., Okely, A. D., & Trost, S. (2014). A review of preschool children’s physical activity and sedentary time using objective measures. American Journal of Preventive Medicine, 47(4), 487–497. https://doi.org/https://doi.org/10.1016/j.amepre.2014.05.042
- Jago, R., Zakeri, I., Baranowski, T., & Watson, K. (2007). Decision boundaries and receiver operating characteristic curves: New methods for determining accelerometer cutpoints. Journal of Sports Sciences, 25(8), 937–944. https://doi.org/https://doi.org/10.1080/02640410600908027
- Janssen, X., Cliff, D. P., Reilly, J. J., Hinkley, T., Jones, R. A., Batterham, M., Ekelund, U., Brage, S., & Okely, A. D. (2013). Predictive validity and classification accuracy of ActiGraph energy expenditure equations and cut-points in young children. PLoS One, 8(11), e79124. https://doi.org/https://doi.org/10.1371/journal.pone.0079124
- Johansson, E., Larisch, L. M., Marcus, C., Hagstromer, M., & Hind, K. (2016). Calibration and validation of a Wrist- and Hip-Worn Actigraph accelerometer in 4-year-old children. PLoS One, 11(9), e0162436. https://doi.org/https://doi.org/10.1371/journal.pone.0162436
- Kahan, D., Nicaise, V., & Reuben, K. (2013). Convergent validity of four accelerometer cutpoints with direct observation of preschool children’s outdoor physical activity. Research Quarterly for Exercise and Sport, 84(1), 59–67. https://doi.org/https://doi.org/10.1080/02701367.2013.762294
- Landis, J. R., & Koch, G. G. (1977). The measurement of observer agreement for categorical data. Biometrics, 33(1), 159–174. https://doi.org/https://doi.org/10.2307/2529310
- Leeger-Aschmann, C. S., Schmutz, E. A., Zysset, A. E., Kakebeeke, T. H., Messerli-Burgy, N., Stulb, K., Arhab, A., Meyer, A. H., Munsch, S., Jenni, O. G., Puder, J. J., & Kriemler, S. (2019). Accelerometer-derived physical activity estimation in preschoolers - comparison of cut-point sets incorporating the vector magnitude vs the vertical axis. BMC Public Health, 19(1), 513. https://doi.org/https://doi.org/10.1186/s12889-019-6837-7
- Nilsen, A. K. O., Anderssen, S. A., Loftesnes, J. M., Johannessen, K., Ylvisaaker, E., & Aadland, E. (2020). The multivariate physical activity signature associated with fundamental motor skills in preschoolers. Journal of Sports Sciences, 38(3), 264–272. https://doi.org/https://doi.org/10.1080/02640414.2019.1694128
- Oliver, M., Schofield, G. M., & Kolt, G. S. (2007). Physical activity in preschoolers: Understanding prevalence and measurement issues. Sports Medicine, 37(12), 1045–1070. https://doi.org/https://doi.org/10.2165/00007256-200737120-00004
- Pate, R. R., Almeida, M. J., McIver, K. L., Pfeiffer, K. A., & Dowda, M. (2006). Validation and calibration of an accelerometer in preschool children. Obesity (Silver Spring), 14(11), 2000–2006. https://doi.org/https://doi.org/10.1038/oby.2006.234
- Pate, R. R., McIver, K., Dowda, M., Brown, W. H., & Addy, C. (2008). Directly observed physical activity levels in preschool children. Journal of School Health, 78(8), 438–444. https://doi.org/https://doi.org/10.1111/j.1746-1561.2008.00327.x
- Pfeiffer, K. A., Dowda, M., McIver, K. L., & Pate, R. R. (2009). Factors related to objectively measured physical activity in preschool children. Pediatric Exercise Science, 21(2), 196–208. https://doi.org/https://doi.org/10.1123/pes.21.2.196
- Puhl, J., Greaves, K., Hoyt, M., & Baranowski, T. (1990). Children’s Activity Rating Scale (CARS): Description and calibration. Research Quarterly for Exercise and Sport, 61(1), 26–36. https://doi.org/https://doi.org/10.1080/02701367.1990.10607475
- Reilly, J. J., Coyle, J., Kelly, L., Burke, G., Grant, S., & Paton, J. Y. (2003). An objective method for measurement of sedentary behavior in 3- to 4-year olds. Obesity Research, 11(10), 1155–1158. https://doi.org/https://doi.org/10.1038/oby.2003.158
- Reilly, J. J., Penpraze, V., Hislop, J., Davies, G., Grant, S., & Paton, J. Y. (2008). Objective measurement of physical activity and sedentary behaviour: Review with new data. Archives of Disease in Childhood, 93(7), 614–619. https://doi.org/https://doi.org/10.1136/adc.2007.133272
- Rowlands, A. V. (2018). Moving forward with accelerometer-assessed physical activity: two strategies to ensure meaningful, interpretable, and comparable measures. Pediatric Exercise Science, 30(4), 450–456. https://doi.org/https://doi.org/10.1123/pes.2018-0201
- Rowlands, A. V., Sherar, L. B., Fairclough, S. J., Yates, T., Edwardson, C. L., Harrington, D. M., Davies, M. J., Munir, F., Khunti, K., & Stiles, V. H. (2019). A data-driven, meaningful, easy to interpret, standardised accelerometer outcome variable for global surveillance. Journal of Science and Medicine in Sport, 22(10), 1132–1138. https://doi.org/https://doi.org/10.1016/j.jsams.2019.06.016
- Sirard, J. R., Trost, S. G., Pfeiffer, K. A., Dowda, M., & Pate, R. R. (2005). Calibration and evaluation of an objective measure of physical activity in preschool children. Journal of Physical Activity & Health, 2(3), 345–357. https://doi.org/https://doi.org/10.1123/jpah.2.3.345
- Vale, S., Santos, R., Silva, P., Soares-Miranda, L., & Mota, J. (2009). Preschool children physical activity measurement: Importance of epoch length choice. Pediatric Exercise Science, 21(4), 413–420. https://doi.org/https://doi.org/10.1123/pes.21.4.413
- Van Cauwenberghe, E., Gubbels, J., De Bourdeaudhuij, I., & Cardon, G. (2011). Feasibility and validity of accelerometer measurements to assess physical activity in toddlers. International Journal of Behavioral Nutrition and Physical Activity, 8(1), 67. https://doi.org/https://doi.org/10.1186/1479-5868-8-67
- van Cauwenberghe, E., Labarque, V., Trost, S. G., De Bourdeaudhuij, I., & Cardon, G. (2011). Calibration and comparison of accelerometer cut points in preschool children. International Journal of Pediatric Obesity, 6(2–2), e582–589. https://doi.org/https://doi.org/10.3109/17477166.2010.526223
- Vanderloo, L. M., Di Cristofaro, N. A., Proudfoot, N. A., Tucker, P., & Timmons, B. W. (2016). Comparing the actical and ActiGraph approach to measuring young children’s physical activity levels and sedentary time. Pediatric Exercise Science, 28(1), 133–142. https://doi.org/https://doi.org/10.1123/pes.2014-0218