ABSTRACT
Using the data from Making a HIIT, this paper aimed to: 1) investigate the different heart rate (HR) quantification methods reported in school-based high-intensity interval training (HIIT) studies; and 2) assess the criterion validity of session rating of perceived exertion (RPE). During an 8-week HIIT intervention, 213 students (13.1 (0.6) years; 46% female) completed 10-minute HIIT workouts during physical education lessons. In total, 1057 HR and RPE measurements were collected across 68 HIIT workouts. For aim 1, the average and peak HR across all participants and workouts were 79% (8%) and 92% (6%) of HRmax, respectively. The average RPE was 6 (2) points on a 10-point scale. An average of 51% of students in a class had an average HR ≥ 80% for each workout. The between-person variation for peak and average HR were 19% and 30% , respectively. Both average and peak HR decreased by 0.5% each week (p < 0.001). To assess aim 2, a within-participant correlation was calculated for the internal training load produced using HR and RPE data. The correlation was 0.39 (p < 0.001), which suggests utility of using RPE when HR is not a viable option.
KEYWORDS:
Introduction
High-intensity interval training (HIIT) is gaining interest from researchers as a method for physical activity delivery and health promotion in the school setting (Duncombe et al., Citation2022). The popularity of HIIT for use with children and adolescents could be attributed to various factors, including research associating time spent in higher intensity physical activity with lower cardiometabolic risk in children and adolescents aged 4 – 18 years (Tarp et al., Citation2018), and the similarity to children’s intermittent patterns of physical activity (Sanders et al., Citation2014). However, there are limited data on the implementation of school-based HIIT interventions (Duncombe et al., Citation2022; Eather et al., Citation2023), which is integral to understanding the link between interventions and relevant outcomes (Naylor et al., Citation2015).
Successful implementation of physical activity interventions includes many determinants (Naylor et al., Citation2015). Some determinants are reported more often within school-based physical activity studies, such as the dosage delivered and received by students (Naylor et al., Citation2015). Meanwhile, fidelity, which is the extent to which an intervention has been implemented as intended, is one of the least examined determinants in school-based physical activity interventions (Naylor et al., Citation2015). Fidelity tends to encompass the frequency, duration, and intensity of exercise within exercise interventions and is crucial to the internal validity of a study (Horner et al., Citation2006; Naylor et al., Citation2015). Reporting intensity is especially important for HIIT interventions, as embedded within the prescription of HIIT, is the assumption that participants will be working within a specific intensity range (e.g., above 85% of maximum heart rate (HR)), which is necessary to experience benefits (Dencker et al., Citation2006; Tarp et al., Citation2018). However, a recent systematic review and meta-analysis on school-based HIIT interventions noted that the achieved intensity was only reported in 48% of the 42 studies (Duncombe et al., Citation2022). Due to the limited reporting of intensity data, the interpretation of the health benefits and the implications for practice cannot currently be ascertained. This paper aims to advocate for the reporting of intensity within school-based HIIT through the discussion of two methods for monitoring intensity and a variety of approaches for reporting these data.
HR is a valid and reliable method for monitoring intensity (Achten & Jeukendrup, Citation2003), and it is the most frequently used method in school-based research (Duncombe et al., Citation2022). However, there is no standardised procedure for reporting HR data to reflect the intensity of HIIT (Duncombe et al., Citation2022), making it difficult to compare between studies or develop a better understanding of the link between implementation and outcomes (Naylor et al., Citation2015). Comparisons among the various methods of reporting HR data used within the literature are warranted to further understand the implications of using different methods and enable more transparent reporting of the intensity of HIIT interventions. Another consideration when reporting intensity is how best to quantify more contemporary forms of HIIT, such as game-based HIIT, where some students might be resting while others work, which makes HR capture during only work intervals challenging for the number of participants involved. Additionally, the most frequently reported metrics in school-based HIIT studies at present, regardless of the HIIT protocol, are an average or peak HR for all students and sessions (Duncombe et al., Citation2022), which are not adequate for determining if the intervention was implemented as intended on an individual level. Two previous studies have examined fidelity in school-based HIIT in more detail (Kennedy et al., Citation2020; Taylor et al., Citation2015). Taylor et al. (Citation2015) examined variation within and between-participants and combined attendance to quantify the exposure variable (intervention). However, fidelity data were only collected from a small subsample of 17 participants, which could overestimate the fidelity of the intervention if the subsample were more actively engaged in the programme. Kennedy et al. (Citation2020) discussed fidelity as part of a larger process evaluation but did not aim to scrutinise the implications of the various HR quantification methods that they used.
While HR is a valid objective measure of exercise intensity during HIIT, there are some practical limitations to using HR monitors regularly in the school environment, including cost, the time required to put on the device, student comfort while wearing the devices, and data loss (Lagally, Citation2013; Pasadyn et al., Citation2019). An alternative measure of intensity that can be utilised in schools is self-reported rating of perceived exertion (RPE). RPE can be completed with ease in health and physical education (HPE) class as it is low cost, requires minimal class time, and is simple to use in group settings (Lagally, Citation2013).
Both HR and RPE can be used to determine the internal training load of a workout, which accounts for the duration and intensity (Impellizzeri et al., Citation2019). To quantify training load, RPE is often converted into a session RPE (sRPE) to reflect the entire exercise period, rather than at a specific moment during exercise. A review that examined the criterion validity of sRPE showcased a wide range of correlations (0.17 to 0.97) between sRPE and internal training load calculated with HR for intermittent sports (Haddad et al., Citation2017). However, the studies included in this review had sample sizes smaller than 20 participants, were completed with athletic populations, included adults, and were not undertaken in the school setting (Haddad et al., Citation2017). Due to these differences, the findings from the review cannot be extrapolated to a generalisable population of students. Currently, there is no evidence on the relationship between sRPE and HR for quantifying internal training load within HIIT workouts in the school setting. It is necessary to investigate the validity of sRPE during school-based HIIT workouts to understand the utility of this measure, especially when objective measures of exercise intensity, such as HR, are not feasible due to cost and time constraints due to curricular demands or in larger scale up studies.
Critiques of HIIT have recently questioned the operational definitions used to classify intensity within HIIT studies, noting that they are inconsistent and occasionally lead to the misclassification of the exercise intensity (Ekkekakis et al., Citation2023). At present, these critiques are valid and are a genuine concern for those advocating for the benefits of HIIT. It is necessary that studies monitor and report the intensity achieved within their studies and do so in a manner that enables variation to be understood. Therefore, the aims of this paper were: 1) to investigate the variation within and between students showcased through different intensity quantification methods that exist for HR in the current literature using the Making a HIIT study as an example; and 2) to assess the criterion validity of sRPE for quantifying the internal training load compared to HR within Making a HIIT.
Methods
This paper uses HR and RPE data from the Making a HIIT study, which involved co-designing HIIT workouts with students and teachers and using the workouts in a school-based intervention. The Making a HIIT study has been described in detail in a protocol paper (Trial Registration: ACTRN, ACTRN12622000534785) (Duncombe et al., Citation2022). This paper uses data from the group of classes who participated in the HIIT workouts and focuses only on the HPE lessons where both HR and RPE data were collected.
Participants
The Making a HIIT study was completed in three secondary schools (one co-educational school, one boys’ school, and one girls’ school) around Greater Brisbane, Australia. It was completed with grade 7 and 8 students and teachers as part of the HPE curriculum. Within each school, there were three groups: 1) the co-design group, which included classes that were involved in the creation of the HIIT workouts and used the HIIT workouts in HPE for a term; 2) the HIIT only group, which included classes that used the HIIT workouts in HPE (but were not involved in the co-design of the HIIT workouts); and 3) the control group, which included classes that continued with normal HPE lessons. In total, 10 classes completed the HIIT workouts in HPE across participating schools (i.e., groups one and two), and the students from these classes form the sample for this study. The control group were not used in this analysis as they did not perform HIIT. Making a HIIT was approved by The University of Queensland’s human research ethics committee (Project: 2020/HE002444) and relevant governing bodies and gatekeepers. Parents and teachers provided written informed consent for participation in the study, and students provided written informed assent.
Intervention
Prior to the intervention, classes in group one co-designed HIIT workouts with researchers and teachers in an iterative process across several HPE lessons. In this process, the class created criteria for the workouts based on identified barriers and facilitators to exercise. The class also established the parameters for the workouts, including the target HR, and maximum and minimum interval lengths. Then, groups of three to five students each designed a 10-minute HIIT workout. Students trialled the workouts and received peer feedback and HR data to modify their workouts in line with the criteria and parameters established in the previous lessons. Due to this co-design process, HIIT workouts varied in terms of theme (e.g., sport-specific, classroom-based workouts), percentage of time in work (average = 65%, range: 53–75%), and work and rest intervals (range 10–60 seconds), although running-based intervals were the most common. The workouts also included exercises and intervals that involved certain students working while others rested, making HR capture of only work intervals unfeasible. Several example workouts are provided in Supplement 1.
For the intervention, students in groups one and two used the co-designed HIIT workouts in an 8-week intervention. Teachers received the workouts in a laminated booklet prior to the term and reviewed them with a researcher. Workouts were delivered by teachers across practical and theory HPE lessons. However, both HR and RPE data were only collected during the practical lessons, due to logistics and time requirements associated with wearing the HR monitors. The HIIT workouts were used as a warmup prior to the rest of the HPE lesson. A researcher was present for all practical HIIT workouts to administer HR monitors. Students were verbally encouraged to provide maximal effort during the “work” periods by both the teacher and researcher throughout the workout. Students were instructed to aim for a HR equal or greater to 80% of their maximum HR (HRmax) while working.
Intensity measures collected
Heart rate
Students wore HR monitors (Polar H10, Polar Electro, Finland) that were fitted by researchers at the start of the intervention to ensure appropriate strap size and placement. Data were recorded using Polar GoFit software (https://polargofit.com/). Participants’ HRmax was determined using a 20-metre shuttle run test conducted in a HPE lesson one week prior to the intervention (The Cooper Institute: FitnessGram PACER test., Citation1982). For students who were absent during this lesson (n = 38), did not complete the test (n = 14), or for whom HR was not collected (1 class, n = 23), HRmax was calculated using the formula 208 – (0.7)*age (Mahon et al., Citation2010). For each HIIT workout, the following HR data were extracted from Polar GoFit for each student: peak HR, peak as a percentage of HRmax, average HR, average as a percentage of HRmax, and time spent with a HR between 1) 50 and 59%; 2) 60 and 69%; 3) 70% and 79%; 4) 80% and 89%; and 5) 90% and 100% of HRmax. The percentage of time spent in each of the above HR zones was calculated by dividing the time spent in each zone by the total length of the workout.
Session rating of perceived exertion
Students reported their sRPE using the Children’s OMNI Scale of Perceived Exertion immediately after the completion of each HIIT workout (Robertson et al., Citation2000). The OMNI RPE has been validated against HR during ramp and continuous exercise, as well as resistance exercise (Robertson et al., Citation2000, Citation2003, Citation2006). Students were asked to circle one number between 0 and 10 on the pictorial scale that corresponded to their effort during the entire HIIT workout using the prompt “During this HIIT workout, I felt…”. Researchers explained to the students that a 10 would equate to an effort that was as hard as they could possibly work and where they felt “very, very tired”, while a zero was equivalent to “not tired at all”.
Data management
Aim one: Quantification of intensity with heart rate
To examine HR variability within and between students, we used various intensity quantification methods that have previously been used in school-based HIIT studies. These included: 1) the mean average HR (absolute and percentage of HRmax) for all students and workouts combined (Arariza, Citation2018; Baquet et al., Citation2002; Buchan et al., Citation2011; Camacho-Cardenosa et al., Citation2016; Costigan et al., Citation2016; Cvetković et al., Citation2018; Kennedy et al., Citation2020; Ketelhut et al., Citation2020; Lambrick et al., Citation2016; Larsen et al., Citation2017; Logan et al., Citation2016; Martin et al., Citation2015; McNarry et al., Citation2020; van Biljon et al., Citation2018; Williams et al., Citation2000); 2) the mean peak HR (absolute and percentage of HRmax) for all students and workouts combined (Arariza, Citation2018; Boddy et al., Citation2010; Camacho-Cardenosa et al., Citation2016; Kennedy et al., Citation2020; Ketelhut et al., Citation2020; Lambrick et al., Citation2016; Martin-Smith et al., Citation2019; McNarry et al., Citation2020); 3) the percentage of time students spent in various deciles (above 70%, 80%, and 90% of HRmax) (Larsen et al., Citation2017; McNarry et al., Citation2020); 4) the mean percentage of students in a class with an average HR above 80% and 90% of HRmax (Kennedy et al., Citation2020); 5) the percentage of students in a class who spent equivalent or more time above 80% and 90% of HRmax than the intended time in work for each workout; and 6) the variability within and between students (Taylor et al., Citation2015). We did not calculate the mean average HR for only time in work, which has been previously used in the literature (Baquet et al., Citation2002; Boddy et al., Citation2010; Camacho-Cardenosa et al., Citation2016; Logan et al., Citation2016; Martin-Smith et al., Citation2019; Williams et al., Citation2000), due to the contemporary HIIT workouts used that included partner exercises (one student exercises while one rests) and intervals of varying lengths, which limited the feasibility of this approach.
Aim two: Session-RPE criterion validity
To assess the criterion validity of sRPE, we calculated a training impulse (TRIMP) for HR using the Edwards method (Edwards, Citation1993). This method combines the volume of exercise with total intensity based on five intensity thresholds. The time spent (in seconds) in each HR zone as a percentage of HRmax was multiplied by a factor (50–59% = 1; 60–69% = 2, 70–79% = 3, 80–89% = 4, 90–100% = 5) and these were summated to generate a total internal training load (in arbitrary units). To calculate sRPE (in arbitrary units), we multiplied each student’s subjective RPE by the total duration (seconds) of the workout equivalent to the duration of the recorded HR.
Data analysis
Data analysis was conducted in R (Version 3.6.2; The R Foundation for Statistical Computing, Vienna, Austria). Alpha was set at 0.05.
Aim one: Quantification of intensity with heart rate
Normality was checked using a Shapiro-Wilk test. Means and standard deviations were reported for normally distributed variables and medians and inter-quartile ranges were reported for not normally distributed quantification methods. The variability within and between students was examined using linear mixed models for the outcome variables of peak and average HR as percentages of HRmax. Sex and intervention week were included as fixed effects. Each student was nested within a school. The assumptions of the model were satisfied, including linearity, homogeneity of variance, and normal distribution of the residuals.
Aim two: Session-RPE criterion validity
To assess the validity of using RPE, the within-participant correlation (r) between TRIMP and sRPE was calculated while accounting for repeated measures (Bland & Altman, Citation1995). This was completed using the RShiny application for repeated measures correlations (Marusich & Bakdash, Citation2021). The magnitude of the correlations was interpreted as follows: 0.1 to 0.3 = negligible; 0.3 to 0.5 = low; 0.5 to 0.7 = moderate; 0.7 to 0.9 = high; > 0.9 = very high (Mukaka, Citation2012).
Results
A total of 68 HIIT workouts included HR and RPE data, with 24 unique HIIT workouts completed. Class attendance varied between lessons and the average attendance for each class is reported in . Occasionally, HR data were not recorded for a participant during a workout due to students arriving late (n = 11); leaving the HIIT workout early (n = 3); removing the monitor (n = 5); leaving the Bluetooth range (n = 3); or equipment malfunctions (n = 39). In total, 1057 measurements were collected from 213 students across the 68 HIIT workouts.
Table 1. Classes involved in the high-intensity interval training (HIIT) intervention.
Aim one: Quantification of intensity with heart rate
The results showcasing variability between students from the various methods of quantifying intensity data in our study are presented in . Between student and across time variation is displayed in .
Figure 1. A) the average heart rate (percentage of heart rate maximum) across the intervention for students in a single class. B) the average heart rate (percentage of heart rate maximum) for each week of the intervention. It decreased on average 0.6% each week (p < 0.001). C) the peak heart rate (percentage of heart rate maximum) for each week of the intervention. It decreased on average 0.5% each week (p < 0.001).
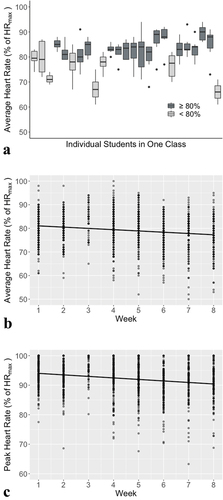
Table 2. Intensity of high-intensity interval training (HIIT) workouts using various heart rate quantifications and session rating of perceived exertion.
The mixed model for peak HR had a significant effect for week (p < 0.001), with an average decrease of 0.5% (95% CI: −0.6% to −0.4%) per week. The within-person variation was 19% points of HRmax. The variation between subjects was 19% points, which explained 51% of the total variance in peak HR (Intra-class coefficient (ICC) = 0.51). The mixed model for average HR also had a significant effect for week (p < 0.001), with a decrease of 0.6% (95% CI: −0.6% to −0.4%) per week. The within-person variation was 31% points of HRmax. The variation between subjects was 30% points, which explained 49% of the variance in average HR (ICC = 0.49). There was no significant effect for sex in any model for HR outcomes.
Aim two: Session-RPE criterion validity
The mean sRPE across all students and sessions was 6 (2). The within-person correlation between sRPE and TRIMP was r = 0.39 (95% CI: 0.33–0.45), p < 0.001, indicating a low correlation (). When stratified by sex, the correlation was r = 0.49 (95% CI: 0.40–0.57, p < 0.001) for girls and r = 0.31 (95% CI: 0.22–0.39, p < 0.001) for boys. The mixed model for sRPE had a significant temporal effect (p < 0,001), with an average decrease of 2.5 arbitrary units (95% CI: −3.0 to −2.0) per week.
Discussion
Making a HIIT is, to our knowledge, the first study to examine the reporting of different HR quantification methods in school-based HIIT. The results demonstrate that the different approaches for quantifying HR can showcase different levels of variability in the data, including within students, between students, and over time. As the intensity of HIIT is monitored on an individual basis, it is important that this variation is acknowledged and considered when evaluating the intervention and its effect on outcome variables. Recently, HIIT interventions have faced criticism (Ekkekakis & Biddle, Citation2023; Ekkekakis et al., Citation2023, Citation2023). One reason for this is that authors report that participants are completing HIIT when, in fact, they are exercising at a threshold that could also be considered moderate (Ekkekakis et al., Citation2023). Critiques argue that this falsely supports the claims that HIIT is enjoyable and beneficial for outcomes (Ekkekakis et al., Citation2023). Therefore, it is necessary that future studies are transparent in their reporting of intensity data and their definition of HIIT as depending on the threshold and quantification methods used, the fidelity of the intervention may be under- or overstated. Using Making a HIIT as an example, the average percentage of students in a class with an average HR above 80% of HRmax was 51%, indicating that half the students may not have achieved high intensity as it was defined, which is not evident when only an average or peak HR for all students and sessions is provided. Before we can appropriately assess the health benefits or enjoyment of these interventions through a per-protocol approach, it is imperative that we know what we are assessing and how much of the intervention was completed as per the stated protocol.
Making a HIIT is also the first study to examine the association between sRPE and HR during school-based HIIT to understand its validity in this setting and with this type of exercise. The low within-subject correlation of 0.39 will be important to consider moving forward if HR or other objective measurements of intensity are not feasible.
Aim one: Quantification of intensity and variation within and between students
The most frequently used approaches for quantifying HR in the school-based HIIT literature are mean average HR and peak HR for the entire HIIT workout (Duncombe et al., Citation2022), either in beats per minute or as a percentage of HRmax, which standardises the measurement based on age and sex. The average and peak HR of 161 (17) bpm and 188 (13) bpm, respectively, reported in Making a HIIT are within the ranges (143 to 179 and 168 to 207 bpm, respectively) reported in previous school-based HIIT studies (Supplement 2) (Kennedy et al., Citation2020; Martin et al., Citation2015). Variation between studies could be partly due to the inclusion of different workout components in the quantification of previous school-based literature as showcased in Supplement 2. For example, one study included warmup and cooldown in their quantification, while others included the full workout (rest and work intervals) or did not specify what was included. Several previous studies have specified that they only included work intervals for their calculations. As this doesn’t include rest, the reported HR data tend to be higher, and make it easier for readers to determine if the intended intensity was being achieved. However, compared with capturing the full workout, this approach also has limitations stemming from the HR lag at exercise onset, which could limit its ability to capture a portion of HR data that is above a threshold if rest is not included (Taylor et al., Citation2015), with short work bouts further limiting the capture of intensity with this approach. Additionally, this type of HR capture is not feasible in workouts where students are not working and resting at the same time as was sometimes the case in Making a HIIT. Overall, reporting the average or peak HR of a workout is a useful first step to quantifying intensity and making comparisons to previous literature. However, transparency on the included workout components (work; rest; warmup; cooldown) is necessary for these comparisons to be made. Additionally, this quantification method would be enhanced by including other approaches that provide further information on the variation within and between students.
Providing the percentage of time spent in different HR zones (e.g., 70–79%, 80–89%, 90–100%) presents readers with a clearer picture of students’ overall effort across the workout and is a valuable approach to showcase variability throughout a workout. On average, students in Making a HIIT spent more time in the 80% − 89% zone than they did in the 70% − 79% or 90% − 100% zones (). Only two previous studies have looked at percentage of time spent in HR zones during school-based HIIT (Larsen et al., Citation2017; McNarry et al., Citation2020). Larsen et al. (Citation2017) reported the percentage of time that students (aged 8 to 10 years) spent in the 70% − 79% and 90% − 100% HR zones, for two different HIIT protocols (interval running, small-sided games), with similar findings to Making a HIIT. McNarry et al. (Citation2020) reported a higher percentage of time spent above 90% for their HIIT intervention, which included circuits and games-based activities. This could be due to the specific activities performed, the trained professionals leading the workouts, or the participants, which included a group of students with asthma, who could have an altered HR response. Although this approach implies that there are specific cut-offs that are of significance, it does utilise a greater percentage of collected data and can showcase variability throughout a workout.
The aforementioned HR quantification methods group students and workouts to provide an overall average. However, they do not capture the substantial variation that exists between individual students. Examining the number of students that achieved a certain average can enhance understanding of the variation across individuals. In this study, 51% of students in a class achieved an average HR greater or equal to 80% of HRmax for a workout. This provides very different information to readers than the mean session average where the fidelity of the intervention appeared higher. The only other study that used this approach to report HR in school-based HIIT found that only 17% of participants achieved a HR average of 85% across the intervention (Kennedy et al., Citation2020). Approaches to reporting HR that examine data of individual participants enable readers to further understand how many students received the intervention as intended, beyond understanding fidelity at a group level. These approaches for reporting intensity are essential to consider when we investigate health outcomes that might stem from school-based interventions.
Further, using mixed models to understand variability both between and within individuals has only previously been completed in one other school-based HIIT study using peak HR (Taylor et al., Citation2015). The authors of the Fun Fast Activity Blast study reported a within-student variation of 15.1% points, which is similar to the results of this study (18.5% points) and indicates substantial variation within individual students throughout the intervention. The between student variation in this study was larger (18.9 to 7.8 points), which could stem from the larger and more generalisable sample included within the present study (Taylor et al., Citation2015). In addition to peak HR, average HR was examined as an outcome in mixed models in this paper, and a greater amount of variation was noted compared to peak HR. This is unsurprising as both rest and work time are counted in the second model, which increases within-participant variation. The increased monitoring time is also able to capture greater variability between participants.
Aim two: Session-RPE criterion validity
The 0.39 within-subject correlation coefficient between sRPE and TRIMP in Making a HIIT is within the range of coefficients (0.17 to 0.97) compiled in a review that assessed the validity of sRPE (Haddad et al., Citation2017). It is on the lower end of the range; however, most of the studies included in the review tended to use standard exercise protocols with adults or motivated athletic populations. For the 11 studies with participants <18 years, coefficients ranged from 0.17 in a group of 12 male soccer players to 0.88 in 13 male water polo players (Haddad et al., Citation2017). sRPE decreased throughout the Making a HIIT intervention, following a similar temporal trend for intensity to peak and average HR. The variance in sRPE that was accounted for by clustering students (ICC = 0.47) was also comparable to peak HR (ICC = 0.51) and average HR (ICC = 0.49). This, combined with the ease of completing sRPE and the low associated cost (Lagally, Citation2013), suggests that it could be a valuable method for monitoring intensity in large school-based programmes. However, further research on the validity of sRPE for monitoring HIIT in this population is necessary, especially if HIIT is being used in the classroom. Further work focused on prescribing HIIT using sRPE is also required.
RPE has not been used frequently in school-based HIIT interventions, with only two other studies reporting RPE results. One intervention that provided a range of workouts to students aged 16 years had an RPE similar to Making a HIIT (6.3 on an 11-point scale) but did not specify when these data were collected (Lubans et al., Citation2021). However, the RPE reported in the other sprint-based HIIT study (3.7 on a 10-point scale) (Camacho-Cardenosa et al., Citation2016) was far lower than the mean RPE in Making a HIIT (6 on a 10-point scale), even though both studies collected RPE immediately after the workout and had similarly aged participants (11 years old compared to 13 years old in Making a HIIT). This discrepancy warrants further exploration into the variation of RPE in this context. Additionally, in Making a HIIT, when stratified by sex, girls had a higher correlation coefficient than boys, with non-overlapping 95% confidence intervals. This could be partially attributed to the greater number of measurements (i.e., more practical lessons with HR) in the boy’s only school compared with the girl’s only school. It is most likely not due to students working at different intensities during HIIT as the HR responses between sexes were similar. While a previous validation study of the OMNI Pictorial Scale reported no difference between boys and girls (Robertson et al., Citation2006), there has been speculation that RPE could be effected by sex in addition to fitness level, age, and expertise (Haddad et al., Citation2017). However, further research is necessary to corroborate these findings.
Strengths and limitations
This is the first paper to comprehensively examine various approaches for quantifying intensity using HR within a school-based HIIT intervention and to examine the relationship between sRPE and HR in this context. The data from Making a HIIT were not powered to assess the concurrent validity of RPE as the study was powered for the trial’s primary outcome (cardiorespiratory fitness). However, the sample size of this study (n = 213) is greater than other RPE validation studies in youth using the OMNI Scale of Perceived Exertion (Robertson et al., Citation2000, Citation2006). The Making a HIIT study employed a wide range of HIIT workouts as they were co-designed by the participants, which is a unique feature. However, within the workouts, there were rarely intervals longer than 30 seconds. Therefore, it will be necessary to examine the relationship between sRPE and HR in future work with varying HIIT protocols (e.g., intervals of various lengths and in HIIT games protocols). A researcher was present for all practical HPE lessons, which does not reflect “real-world” implementation; however, they did not facilitate the HIIT workouts or any of the subsequent HPE lesson. Lastly, the sample in Making a HIIT only included a specific age range and originated from a single region; therefore, further investigation is warranted to confirm our findings in different age groups and contexts where the HPE curriculum and allotted time differ. However, this age range was selected based on alignment with the Australian HPE curriculum to complement the units being conducted at each school and limit the burden to the teachers and curriculum time. The data in the present paper was restricted to practical lessons and it will be important for future work to examine if these findings are similar in theory lessons within the classroom.
Conclusions and recommendations
The findings from this study demonstrate that depending on the definition of “high-intensity” and the analysis of data, fidelity could range from poor to favourable. Therefore, it is important for studies to comprehensively investigate and report exercise intensity in school-based HIIT research to showcase the variability in HR data within and between students. Additionally, the variation over time suggests that future studies need to include intensity measurements across the entire programme along with reporting how programmes change and adapt across the intervention to account for students improving in fitness and becoming more familiar with the exercises. It is essential that future studies report which parts of the workout are captured by the HR data (e.g., only work intervals or the entire session) and document variation to enable readers to have a complete understanding of the extent to which an intervention was implemented as intended. We have showcased several options for documenting this variation beyond a session average, including, reporting the time spent in HR zones, the number of students who achieve a HR threshold, and mixed effect models for assessing within and between student variation. Further, our results suggest sRPE may be useful when HR is not a viable option, as it followed a similar temporal sequence to HR throughout the intervention. However, additional studies are necessary to corroborate our findings and to enhance our understanding of using RPE as a monitoring tool for high-intensity exercise in this setting.
Supplement 1.docx
Download MS Word (14.4 KB)Supplement 2.docx
Download MS Word (16.5 KB)Acknowledgments
We would like to acknowledge the participating teachers and students in the Making a HIIT study for their contribution to this research.
Disclosure statement
No potential conflict of interest was reported by the authors.
Supplementary material
Supplemental data for this article can be accessed online https://doi.org/10.1080/02640414.2023.2291736
Additional information
Funding
References
- Achten, J., & Jeukendrup, A. E. (2003). Heart rate monitoring. Sports Medicine, 33(7), 517–538. https://doi.org/10.2165/00007256-200333070-00004
- Arariza, A. (2018). 24 sessions of monitored cooperative high-intensity interval training improves attention-concentration and mathematical calculation in secondary school. Journal of Physical Education & Sport, 18(3), 1572–1582.
- Baquet, G., Berthoin, S., Dupont, G., Blondel, N., Fabre, C., & van Praagh, E. (2002). Effects of high intensity intermittent training on peak VO2 in prepubertal children. International Journal of Sports Medicine, 23(6), 439–444. https://doi.org/10.1055/s-2002-33742
- Bland, J. M., & Altman, D. G. (1995). Statistics notes: Calculating correlation coefficients with repeated observations: Part 1–correlation within subjects. BMJ, 310(6977), 446–446. https://doi.org/10.1136/bmj.310.6977.446
- Boddy, L., Stratton, G., Hackett, A., & George, K. (2010). The effectiveness of a ‘short, sharp, shock’ high intensity exercise intervention in 11- and 12-year-old Liverpool schoolgirls. Arch Exerc Health Dis, 1(1), 19–25.
- Buchan, D. S., Ollis, S., Young, J. D., Thomas, N. E., Cooper, S. M., Tong, T. K., Nie, J. L., Malina, R. M., & Baker, J. S. (2011). The effects of time and intensity of exercise on novel and established markers of CVD in adolescent youth. American Journal of Human Biology: The Official Journal of the Human Biology Council, 23(4), 517–526. https://doi.org/10.1002/ajhb.21166
- Camacho-Cardenosa, A., Brazo-Sayavera, J., Camacho-Cardenosa, M., Marcos-Serrano, M., Timon, R., & Olcina, G. (2016). Effects of high intensity interval training on fat mass parameters in adolescents. Revista espanola de salud publica, 90, e1–e9.
- The Cooper Institute: FitnessGram PACER test. 1982. https://fitnessgram.net/pacertest/
- Costigan, S. A., Eather, N., Plotnikoff, R. C., Hillman, C. H., & Lubans, D. R. (2016). High-intensity interval training for cognitive and mental health in adolescents. Medicine & Science in Sports & Exercise, 48(10), 1985–1993. https://doi.org/10.1249/MSS.0000000000000993
- Cvetković, N., Stojanović, E., Stojiljković, N., Nikolić, D., Scanlan, A. T., & Milanović, Z. (2018). Exercise training in overweight and obese children: Recreational football and high‐intensity interval training provide similar benefits to physical fitness. Scandinavian Journal of Medicine & Science in Sports, 28(S1), 18–32. https://doi.org/10.1111/sms.13241
- Dencker, M., Thorsson, O., Karlsson, M. K., Lindén, C., Svensson, J., Wollmer, P., & Andersen, L. B. (2006). Daily physical activity and its relation to aerobic fitness in children aged 8–11 years. European Journal of Applied Physiology, 96(5), 587–592. https://doi.org/10.1007/s00421-005-0117-1
- Duncombe, S. L., Barker, A., Bond, B., Earle, R., Varley-Campbell, J., Vlachopoulos, D., Walker, J. L., Weston, K. L., Stylianou, M., & Harnish, C. (2022). School-based high-intensity interval training programs in children and adolescents: A systematic review and meta-analysis. PLOS ONE, 17(5), e0266427. https://doi.org/10.1371/journal.pone.0266427
- Duncombe, S. L., Barker, A. R., Price, L., Walker, J. L., Dux, P. E., Fox, A., Matthews, N., & Stylianou, M. (2022). Making a HIIT: Study protocol for assessing the feasibility and effects of co-designing high-intensity interval training workouts with students and teachers. BMC Pediatrics, 22(1). https://doi.org/10.1186/s12887-022-03440-w
- Eather, N., Babic, M., Riley, N., Costigan, S. A., & Lubans, D. R. (2023). Impact of embedding high-intensity interval training in schools and Sports training on children and adolescent’s cardiometabolic health and health-related fitness: Systematic review and meta-analysis. Journal of Teaching in Physical Education, 42(2), 243–255. https://doi.org/10.1123/jtpe.2021-0165
- Edwards, S. (1993). High performance training and racing. Feet Fleet Press.
- Ekkekakis, P., & Biddle, S. J. H. (2023). Extraordinary claims in the literature on high-intensity interval training (HIIT): IV. Is HIIT associated with higher long-term exercise adherence? Psychology of Sport and Exercise, 64, 102295. https://doi.org/10.1016/j.psychsport.2022.102295
- Ekkekakis, P., Hartman, M. E., & Ladwig, M. A. (2023). A methodological checklist for studies of pleasure and enjoyment responses to high-intensity interval training: Part II. Intensity, timing of assessments, data modeling, and interpretation. Journal of Sport & Exercise Psychology, 45(2), 92–109. https://doi.org/10.1123/jsep.2022-0029
- Ekkekakis, P., Vallance, J., Wilson, P. M., & Ewing Garber, C. (2023). Extraordinary claims in the literature on high-intensity interval training (HIIT): III. Critical analysis of four foundational arguments from an interdisciplinary lens. Psychology of Sport & Exercise, 66, 102399. https://doi.org/10.1016/j.psychsport.2023.102399
- Haddad, M., Stylianides, G., Djaoui, L., Dellal, A., & Chamari, K. (2017). Session-RPE method for training load monitoring: Validity, ecological usefulness, and influencing factors. Frontiers in Neuroscience, 11(612). https://doi.org/10.3389/fnins.2017.00612
- Horner, S., Rew, L., & Torres, R. (2006). Enhancing intervention fidelity: A means of strengthening study impact. Journal for Specialists in Pediatric Nursing, 11(2), 80–89. https://doi.org/10.1111/j.1744-6155.2006.00050.x
- Impellizzeri, F. M., Marcora, S. M., & Coutts, A. J. (2019). Internal and external training load: 15 years on. International Journal of Sports Physiology and Performance, 14(2), 270–273. https://doi.org/10.1123/ijspp.2018-0935
- Kennedy, S. G., Leahy, A. A., Smith, J. J., Eather, N., Hillman, C. H., Morgan, P. J., Plotnikoff, R. C., Boyer, J., & Lubans, D. R. (2020). Process evaluation of a school-based high-intensity interval training program for older adolescents: The burn 2 learn cluster randomised controlled trial. Children (Basel), 7(12), 16. https://doi.org/10.3390/children7120299
- Ketelhut, S., Kircher, E., Ketelhut, S. R., Wehlan, E., & Ketelhut, K. (2020). Effectiveness of multi-activity, high-intensity interval training in school-aged children. International Journal of Sports Medicine, 14(4), 227–232. https://doi.org/10.1055/a-1068-9331
- Lagally, K. M. (2013). Using ratings of perceived exertion in physical Education. The Journal of Physical Education, Recreation & Dance, 84(5), 35–39. https://doi.org/10.1080/07303084.2013.779533
- Lambrick, D., Westrupp, N., Kaufmann, S., Stoner, L., & Faulkner, J. (2016). The effectiveness of a high-intensity games intervention on improving indices of health in young children. Journal of Sports Sciences, 34(3), 190–198. https://doi.org/10.1080/02640414.2015.1048521
- Larsen, M. N., Nielsen, C. M., Orntoft, C., Randers, M. B., Helge, E. W., Madsen, M., Manniche, V., Hansen, L., Hansen, P. R., & Bangsbo, J. (2017). Fitness effects of 10-month frequent low-volume ball game training or interval running for 8-10-year-old school children. BioMed Research International, 2017, 2719752. https://doi.org/10.1155/2017/2719752
- Logan, G. R. M., Harris, N., Duncan, S., Plank, L. D., Merien, F., & Schofield, G. (2016). Low-active male adolescents: A dose response to high-intensity interval training. Medicine & Science in Sports & Exercise, 48(3), 481–490. https://doi.org/10.1249/MSS.0000000000000799
- Lubans, D. R., Smith, J. J., Eather, N., Leahy, A. A., Morgan, P. J., Lonsdale, C., Plotnikoff, R. C., Nilsson, M., Kennedy, S. G., & Holliday, E. G. (2021). Time-efficient intervention to improve older adolescents’ cardiorespiratory fitness: Findings from the ‘Burn 2 Learn’ cluster randomised controlled trial. British Journal of Sports Medicine, 55(13), 751–758. https://doi.org/10.1136/bjsports-2020-103277
- Mahon, A. D., Marjerrison, A. D., Lee, J. D., Woodruff, M. E., & Hanna, L. E. (2010). Evaluating the prediction of maximal heart rate in children and adolescents. Research Quarterly for Exercise and Sport, 81(4), 466–471. https://doi.org/10.1080/02701367.2010.10599707
- Martin, R., Buchan, D. S., Baker, J. S., Young, J., Sculthorpe, N., & Grace, F. M. (2015). Sprint interval training (SIT) is an effective method to maintain cardiorespiratory fitness (CRF) and glucose homeostasis in Scottish adolescents. Biology of Sport, 32(4), 307–313. https://doi.org/10.5604/20831862.1173644
- Martin-Smith, R., Buchan, D. S., Baker, J. S., Macdonald, M. J., Sculthorpe, N. F., Easton, C., Knox, A., & Grace, F. M. (2019). Sprint interval training and the school curriculum: Benefits upon cardiorespiratory fitness, physical activity profiles, and cardiometabolic risk profiles of healthy adolescents. Pediatric Exercise Science, 31(3), 296–305. https://doi.org/10.1123/pes.2018-0155
- Marusich, L. R., & Bakdash, J. Z. (2021). rmcorrShiny: A web and standalone application for repeated measures correlation. F1000research, 10, 697. https://doi.org/10.12688/f1000research.55027.1
- McNarry, M. A., Winn, C. O. N., Davies, G. A., Eddolls, W. T. B., & Mackintosh, K. A. (2020). Effect of high-intensity training and asthma on the V˙O2 kinetics of adolescents. Medicine & Science in Sports & Exercise, 16(6), 1322–1329. https://doi.org/10.1249/MSS.0000000000002270
- Mukaka, M. M. (2012). Statistics corner: A guide to appropriate use of correlation coefficient in medical research. Malawi Medical Journal: The Journal of Medical Association of Malawi, 24(3), 69–71.
- Naylor, P.-J., Nettlefold, L., Race, D., Hoy, C., Ashe, M. C., Wharf Higgins, J., & McKay, H. A. (2015). Implementation of school based physical activity interventions: A systematic review. Preventive Medicine, 72, 95–115. https://doi.org/10.1016/j.ypmed.2014.12.034
- Pasadyn, S. R., Soudan, M., Gillinov, M., Houghtaling, P., Phelan, D., Gillinov, N., Bittel, B., & Desai, M. Y. (2019). Accuracy of commercially available heart rate monitors in athletes: A prospective study. Cardiovascular Diagnosis and Therapy, 9(4), 379–385. https://doi.org/10.21037/cdt.2019.06.05
- Robertson, R. J., Goss, F. L., Aaron, D. J., Tessmer, K. A., Gairola, A., Ghigiarelli, J. J., Kowallis, R. A., Thekkada, S., Liu, Y., & Randall, C. R. (2006). Observation of perceived exertion in children using the OMNI pictorial scale. Psychobiology and Behavioral Strategies, 38(1), 158–166. https://doi.org/10.1249/01.mss.0000190595.03402.66
- Robertson, R. J., Goss, F. L., Boer, N. F., Peoples, J. A., Foreman, A. J., Dabayebeh, I. M., Millich, N. B., Balasekaran, G., Riechman, S. E., & Gallagher, J. D. (2000). Children’s OMNI scale of perceived exertion: Mixed gender and race validation. Medicine & Science in Sports & Exercise, 32(2), 452. https://doi.org/10.1097/00005768-200002000-00029
- Robertson, R. J., Goss, F. L., Rutkowski, J., Lenz, B., Dixon, C., Timmer, J., Frazee, K., Dube, J., & Andreacci, J. (2003). Concurrent validation of the OMNI perceived exertion scale for resistance exercise. Medicine & Science in Sports & Exercise, 35(2), 333–341. https://doi.org/10.1249/01.MSS.0000048831.15016.2A
- Sanders, T., Cliff, D. P., & Lonsdale, C. (2014). Measuring adolescent boys’ physical activity: Bout length and the influence of accelerometer epoch length. PLoS One, 9(3), e92040. https://doi.org/10.1371/journal.pone.0092040
- Tarp, J., Child, A., White, T., Westgate, K., Bugge, A., Grøntved, A., Wedderkopp, N., Andersen, L. B., Cardon, G., & Davey, R. (2018). Physical activity intensity, bout-duration, and cardiometabolic risk markers in children and adolescents. International Journal of Obesity, 42(9), 1639–1650. https://doi.org/10.1038/s41366-018-0152-8
- Taylor, K. L., Weston, M., Batterham, A. M., & Piacentini, M. F. (2015). Evaluating intervention fidelity: An example from a high-intensity interval training study. PLOS ONE, 10(4), e0125166. https://doi.org/10.1371/journal.pone.0125166
- van Biljon, A., McKune, A. J., DuBose, K. D., Kolanisi, U., & Semple, S. J. (2018). Do short-term exercise interventions improve cardiometabolic risk factors in children? The Journal of Pediatrics, 203, 325–329. https://doi.org/10.1016/j.jpeds.2018.07.067
- Williams, C. A., Armstrong, N., & Powell, J. (2000). Aerobic responses of prepubertal boys to two modes of training. British Journal of Sports Medicine, 34(3), 168–173. https://doi.org/10.1136/bjsm.34.3.168