ABSTRACT
This study sought to assess the validity of contact involvement (CI) detection using microsensor technology (MST, Catapult Vector) within the context of a Tier One national rugby union (RU) squad, consisting of 44 players. Sensitivity of MST units to detect CI and scrums was assessed in eight test matches, by comparison with match data obtained by video analysis. This paper is the first to assess the sensitivity of MST to the full range of skilled CI which occur in RU, including evaluating “non-performance” collisions, such as incidental collisions or foul play. Sensitivity to tackles made (52.9–84.9%) and ruck hits (53.3–87.2%) was lower than previous research, although ball carries (71.9–93.5%) showed broadly similar sensitivity to established results. The sensitivity of the MST to detect scrums was substantially lower than previous findings, with large positional variation evident (51.4–91.5%). Further refinement of MST software should be considered in order to facilitate valid monitoring of RU performance and injury risk. An additional finding was that video analysis generally demonstrated satisfactory intrarater reliability. This result supports the use of video analysis as a reliable method of assessing RU performance, including CI.
Introduction
Rugby union (RU) performance is characterised by a combination of locomotive events and contact involvements (CI) (Quarrie et al., Citation2013). CI include dynamic actions such as ball carries, tackles, and rucks; and low velocity skills such as mauls and scrums (Coughlan et al., Citation2011; Cunniffe et al., Citation2009). Quantifying CI may benefit sports science and medicine practitioners in load monitoring and injury surveillance, given that ball carry and tackle events are the most frequent mechanisms of injury in RU (Williams et al., Citation2013). Assessment of CI has traditionally been conducted by video analysis (Austin et al., Citation2011; Quarrie et al., Citation2013; Reardon, Tobin, Tierney, Delahunt, & Maher, Citation2017; Roberts et al., Citation2008). Match analysis of rugby sevens has demonstrated typical error of measurement of video analysis to be < 8% (Ross et al., Citation2015). Similarly, our internal observations have shown that video analysis of RU skills can be conducted with excellent reliability, with intraclass correlation coefficient values ranging from 0.91 to 1.00, although these results remain unpublished. Despite this, the potential for human error within video analysis has been the subject of criticism (Chambers, Gabbett, Gupta, et al., Citation2019; Reardon, Tobin, Tierney, & Delahunt, Citation2017; Tierney et al., Citation2020). Furthermore, the labour-intensive nature of conducting video analysis has been noted as a barrier to its implementation (Till et al., Citation2020).
The use of micro-sensor technology (MST) in RU, most commonly housed in global positioning system (GPS) units, presents an opportunity for practitioners to quantify CI in real-time using accelerometers, gyroscopes and magnetometers (Gabbett et al., Citation2010). The detection of CI by MST is facilitated by a process of sensor fusion: incorporating accelerometer and magnetometer data with gyroscopic data to improve the accuracy of orientation estimates, whilst also accounting for gyroscopic drift (Bergamini et al., Citation2014).
PlayerLoad, a proprietary metric representing the sum of tri-axial accelerations (Catapult Innovations, Melbourne, Australia), has previously been studied to assess the efficacy of accelerometer-based CI measurement. Large to very large relationships have been observed between PlayerLoad and video-coded CI (ball carries, tackles, rucks and scrums) count (Roe et al., Citation2016). However, a possible confounding factor is the strong correlation observed between PlayerLoad and running volume, potentially limiting the utility of PlayerLoad as a proxy measure of CI (Roe et al., Citation2016).
Further study investigated the relationship between the count of specific video-coded CI and MST-identified CI based upon varying thresholds of g forces (Reardon, Tobin, Tierney, & Delahunt, Citation2017). Even when the g force threshold was optimised to minimise the smallest mean difference between video and MST methods, there was still a marked variation which resulted in as much as 98% underestimation or 74% overestimation of CI by MST. Furthermore, their study assessed the count of all CI, rather than the sensitivity of MST to identify individual video-coded CI. STATSports Viper (STATSports, Newry, Northern Ireland) devices demonstrated ability to identify tackle and carry events with a sensitivity of 93.9% (MacLeod et al., Citation2018). Collision Load (STATSports), a metric incorporating CI velocity, duration and peak force, was significantly greater during dominant tackle and carry events compared with passive events (as assessed by video). This finding has relevance for subsequent research, given that the sensitivity of MST may feasibly vary depending on CI dominance. However, rucks were not included in their analyses, limiting the application of their findings (MacLeod et al., Citation2018). Another study developed an MST algorithm to differentiate between tackle and ruck events (Chambers, Gabbett, Gupta, et al., Citation2019). Although this algorithm showed promising results, it was developed based upon one-on-one tackle events (i.e., not including assist tacklers), and covered only a partial range of the CI typical in RU.
A separate study demonstrated an MST algorithm with a sensitivity of 90–98% and 94–91% for detection of scrums during RU match-play and training, respectively (Chambers, Gabbett, & Cole, Citation2019). Given that scrums are unlikely to be identified as CI by MST (due to the relatively low velocity and displacement at the point of contact (Preatoni et al., Citation2013)), the finding that MST algorithms can independently identify scrums with high sensitivity has the potential to provide RU practitioners with a more comprehensive understanding of the physical demands experienced by forwards.
More recently, efforts have been made to quantify a greater range of CI (carries, tackles, and rucks) (Tierney et al., Citation2020). The count of MST-identified CI demonstrated nearly perfect correlations with both Collision Load (STATSports) and the count of video-identified CI. Furthermore, greater Collision Load values were observed in backs than in forwards (Tierney et al., Citation2020), which supports previous suggestions (Reardon, Tobin, Tierney, & Delahunt, Citation2017) that backs tend to experience greater forces during CI than forwards, owing to greater running velocities prior to contact, although discrepancies in body mass will cause fluctuation in the force experienced during CI, given that momentum is the product of mass and velocity.
However, correlational research has limited application when assessing the utility of MST to identify CI, as a strong correlation between the count of MST-identified and video-coded CI does not necessarily indicate the ability of MST to accurately identify individual CI, and to distinguish these from noise signals of non-CI skills. Pearson correlation coefficient values consider the degree of association, rather than absolute agreement; therefore, exclusive reliance upon correlation analyses may be considered unsuitable in the validation of new measurement techniques (Altman & Bland, Citation1983).
To date, there is a lack of published research detailing the sensitivity of MST across the full range of CI known to occur in RU. Additionally, it is known that CI might occur under circumstances that would not be classified as skilled performance, such as incidental collisions or deliberate foul play. These events, termed in this paper as “non-performance collisions”, have been alluded to in previous research (Reardon, Tobin, Tierney, & Delahunt, Citation2017) but have yet to be appropriately quantified.
The validation of CI detection by MST has been praised as a time-efficient alternative to supposedly error-prone video analysis (Chambers, Gabbett, Gupta, et al., Citation2019; Reardon, Tobin, Tierney, & Delahunt, Citation2017; Tierney et al., Citation2020). However, studies to date have failed to cite any supporting evidence for poor reliability of video analysis. Furthermore, despite relying on video analysis as a reference comparator, studies have failed to assess the reliability of their own video analysis methods (Reardon, Tobin, Tierney, & Delahunt, Citation2017; Tierney et al., Citation2020).
The primary aim of this paper was to assess the validity of detection of skilled CI, non-performance collisions and scrums using Catapult Vector S7 (Catapult Innovations) devices. Furthermore, this study sought to determine the intrarater reliability of video analysis.
Methods
Participants
The study received institutional ethical approval prior to commencement. Players gave consent for their data to be used for research purposes as part of their standard playing contracts. Players were selected for inclusion if they played for the senior male national RU team in 2020. A total of 44 male players’ (age = 27.9 ± 2.9 years, body mass = 105.4 ± 12.2 kg, height = 187.3 ± 6.9 cm) data were pooled for analysis. Positional groups were coded as forward or back, with nine specific sub-groups (prop, hooker, second row, back row, scrum half, fly half, centre, wing, full back) (Reardon, Tobin, Tierney, & Delahunt, Citation2017).
Procedures
Eight matches in 2020 were selected for inclusion. Matches were coded by a performance analyst with four years of vocational experience in professional RU. Four video files (television feed, tight, wide, and end-on angles) were synchronised in Hudl Sportscode version 12.2.36 (Hudl, Lincoln, USA), to improve the accuracy of video analysis. The operational definitions for video analysis used in this study aligned to those routinely employed in the squad of interest, with the addition of non-performance collisions and collision events, to ensure the analyst was familiar with the coding protocol. These were in line with World Rugby’s “Laws of the Game”. For instance, this protocol used the World Rugby definition for a tackle (i.e., ball carrier must be brought to ground for the defender’s action to be considered a completed tackle). The operational definitions were developed in collaboration with a second experienced performance analyst with six years of vocational experience in professional RU (see ). CI were coded at the initial point of contact with the ground or another player. To assess intrarater reliability, one randomly selected match was recoded one month after initial coding, as per previous research (Ross et al., Citation2015).
Players wore Catapult Vector S7 (Catapult Innovations) devices in a playing shirt pocket situated between the scapulae on the upper thoracic spine. Devices were equipped with an accelerometer sampling at 1000 Hz (±16 G in three dimensions), gyroscope (±2000º/s in three dimensions) and magnetometer (±4900µT), all providing data at 100 Hz. Devices were turned on during the warm-up and remained on for the duration of each match, with players using the same device on each occasion to minimise inter-device variability (Buchheit & Simpson, Citation2017). After each match, data were downloaded to Catapult OpenField software (versions 2.7.0 to 3.2.0, varying as updates were released; Catapult Innovations), and files were trimmed to the duration that player spent on the field during match-play, excluding half-time. Instances of “rugby union contact involvement” and “rugby union scrum”, with confidence levels ≥ 50% and ≥ 25%, respectively (derived from the decision tree classifier), were exported in “.csv” format. If a scrum occurred alongside a candidate CI, the scrum took precedence and would override any potential CI, as per the manufacturer’s design.
Statistical analysis
All data (video coding and MST) were exported to Microsoft Excel for Mac version 16.50, and the timelines were aligned using the start of the first half as a synchronisation point to facilitate analysis. Intrarater reliability for video coding was assessed in IBM SPSS Statistics for Macintosh version 25.0.0.1 using a two-way mixed model, absolute agreement, intraclass correlation coefficient (Koo & Li, Citation2016).
Formulae were written in Microsoft Excel to assess whether each video-coded event corresponded to an MST-identified CI or scrum. For MST-identified CI and scrum events, a time window starting at three seconds prior to the MST start time, and ending three seconds after the MST end time was used, to account for minor discrepancies in on-board clock time of the devices. These timeframes, developed using a pilot testing process, were deemed to represent an acceptable balance between capturing true positive CI, and minimising the risk of identifying erroneous false positive CI. If the timestamp on video-coded events corresponded to an MST-identified event, a positive result was returned.
As per previous research (MacLeod et al., Citation2018), MST sensitivity was calculated as the count of true positives (video-coded CI with corresponding MST CI), divided by the sum of true positives and false negatives (video-coded CI without corresponding MST CI). When determining the sensitivity of CI detection, only video-coded events which incorporated a collision event (as defined in ) were considered for inclusion. Without this distinction, events that did not incorporate a CI (such as scoring a try without being tackled or diving on the ground), and where MST would therefore not be expected to detect CI, may have led to apparent false negative results. MST data were subsequently synchronised with video footage in Catapult Vision version 3.7.1 (Catapult Innovations), enabling subjective analysis of skill types where MST demonstrated low sensitivity, to gain a richer understanding of the factors that may have contributed.
Results
The discrepancy between video-coded and MST-identified CI is shown in , presented as percentage difference from video-coded CI (Giavarina, Citation2015). The results in demonstrate that CI count obtained by MST is lower than the count of video-coded CI in the vast majority of instances. MST sensitivity for CI detection is displayed in . MST sensitivity for scrum detection within the forwards is displayed in .
Figure 3. Bland-Altman plot comparing CI count for forwards. CI = contact involvement; LoA = 95% limit of agreement; MST = microsensor technology.
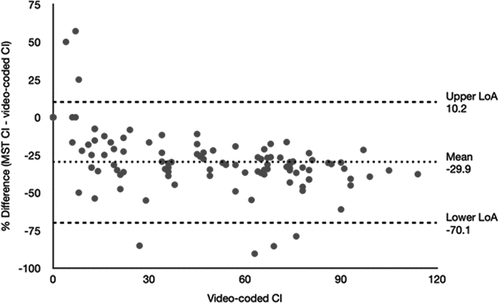
Figure 4. Bland-Altman plot comparing CI count for backs. CI = contact involvement; LoA = 95% limit of agreement; MST = microsensor technology.
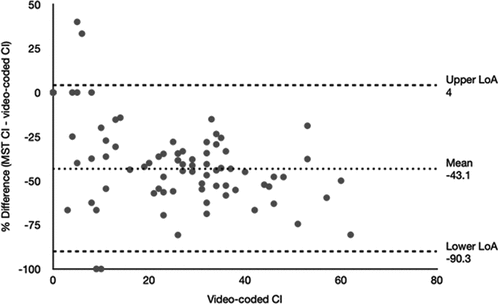
Table 1. Sensitivity of MST to identify CI.
Table 2. Sensitivity of MST to identify forwards’ scrums.
Intrarater reliability of video analysis is presented in , with 95% confidence intervals interpreted based on published recommendations (Koo & Li, Citation2016).
Discussion
This aims of this study were to assess the validity of Catapult Vector S7 (Catapult Innovations) MST devices in identifying CI and scrums, and to establish the reliability of RU video analysis. The sensitivity of MST to identify CI showed large variation depending on the skill type (highest for carries into contact, which were broadly in agreement with previous research (MacLeod et al., Citation2018), lowest for missed tackles) and positional group of interest (generally greater in forwards than backs). The findings of this study demonstrate that video analysis can generally be conducted with excellent intrarater reliability, although some variables such as latches and non-performance collisions had lower reliability.
The results obtained in this study show that relying upon the count of MST-identified CI is likely to lead to a mean underestimation of the true CI volume players experience in senior RU, by 30% for forwards and 43% for backs. One possible contributing factor is the design of the Catapult CI algorithm, which removes smaller peak accelerations if multiple peaks are identified within a rolling two second period. In practice, as observed during the subjective analysis, this is likely to lead to instances where multiple CI are erroneously grouped together as one MST-identified CI. For instance, a tackler who releases the ball carrier and immediately competes for possession at the defensive ruck may undergo two distinct CI (as identified by video analysis), which would be grouped together by MST. This methodology must be borne in mind as it is likely to cause substantial underestimation of the true CI volume experienced by players.
One factor that could feasibly influence MST sensitivity to tackles is the magnitude of acceleration detected by the accelerometer. MacLeod et al. (MacLeod et al., Citation2018) demonstrated that dominant tackles exhibited significantly greater velocity, but not impact force (g), compared with neutral and passive tackles. In the present study, forwards had the greatest sensitivity to passive tackles, compared with effective and dominant tackles. Conversely, backs had marginally greater sensitivity to dominant and effective tackles, compared with passive tackles. Given that video assessment of tackle dominance is guided by the tackle outcome (net territory gain or loss), a simplistic model might reasonably expect passive tackles to involve a greater magnitude of acceleration for the tackler. This can be explained due to the tackler advancing forwards towards the ball carrier before being accelerated back towards their own try line as they concede territory. This assumption is based on front-on tackles, although it is known that RU tackles may occur front-on, side-on (Hendricks et al., Citation2014), or from behind in the case of a cover tackle, increasing the complexity of analysing the biomechanics of the tackle event. The present study did not differentiate between front-on and side-on tackles, although this would be a valuable addition to future study of MST sensitivity. Furthermore, it should be noted that other factors (body mass, velocity, tackle technique) (Hendricks et al., Citation2014), as well as the inclusion of sequentially arriving players (assist tackler, or attacking player latching on to the ball carrier) (Hendricks et al., Citation2020) add greater complexity to analysis of the tackle event.
Despite the suggestions that backs experience greater forces during CI, owing to greater pre-contact velocities (Reardon, Tobin, Tierney, & Delahunt, Citation2017; Tierney et al., Citation2020), backs showed similar or marginally lower sensitivity for CI detection than forwards across most skill types. These findings suggest that force magnitude is unlikely to be the principal limiting factor during instances of CI not detected by MST (i.e., false negatives), and that the limiting factor may instead be attributed to force direction or body orientation.
To date, no published research has assessed the capability of MST to identify mauls. In the present study, sensitivity for attacking maul detection was greatest in props, centres and wingers. This finding may be explained by the roles typically played by these positions during lineouts (where most mauls originate). For instance, props are frequently involved in lifting jumping players in lineouts. Therefore, props would often be the first players to join the maul, leading to more rapid changes in posture (and therefore device pitch) than other forwards joining an attacking maul. Conversely, backs generally begin at least 10 metres behind a lineout, and are likely to join attacking mauls with a greater approach velocity than forwards, resulting in a greater deceleration and change in orientation at the point of collision, increasing the likelihood of CI detection by MST.
MST showed lower sensitivity to defensive mauls. Subjective comparison of video-coded and MST timelines identified two potential causes: the tactical approach of players delaying their engagement with the maul to maintain effective defensive positioning; and, the posture of jumping players joining the maul. If a player jumps to compete for possession at the defensive lineout, then upon landing they are often held upright in the maul. In this situation, it is possible that postural deviation of MST may be insufficient to be identified as a CI. Further study of MST maul detection would benefit from specific analysis considering the technical subtleties of positional roles (e.g., jumper, lifter, player with delayed engagement in the maul).
Given this was the first study to quantify the volume of non-performance collisions, the operational definition was intentionally broad. Sensitivity for non-performance collisions was lower than skilled CI, likely reflecting the fact that many of these CI were low-intensity interactions without substantial change in players’ posture that would not have satisfied the MST algorithm conditions. Whilst many of the non-performance collisions coded in this study were unlikely to contribute to muscle damage, there were high velocity CI, which would likely still be noteworthy for practitioners. Future studies of MST should include non-performance collisions in their analyses, and could be refined to only include situations involving a substantial change in torso orientation.
The exact parameters of video coding operational definitions should be clearly understood by members of the multi-disciplinary team applying video analysis data in their field. For instance, late, high or otherwise dangerous tackles are unlikely to be routinely coded by performance analysts, because doing so may be seen to “reward” a player by increasing their tackle count. However, from the perspective of accumulating muscle damage, the aforementioned CI are still likely to be relevant to practitioners. MST may offer a solution to this issue, by objectively quantifying CI volume in situations which would otherwise be (intentionally) overlooked by video analysts.
The sensitivity of MST to detect scrums in the present study was lower than that obtained previously (Chambers, Gabbett, & Cole, Citation2019), although sensitivity of scrum hits by props in this study was broadly similar to previous results (91.5% versus 96% respectively). The reduced sensitivity in the present study is surprising, considering a lower confidence cut-off (25%) was utilised compared with the 37% cut-off suggested previously (Chambers, Gabbett, & Cole, Citation2019). Large discrepancies in MST sensitivity to scrums were observed between positional groups. Unlike previous research (Chambers, Gabbett, & Cole, Citation2019), this study analysed props and hookers separately based on the distinct demands of each position (Reardon, Tobin, Tierney, & Delahunt, Citation2017). MST demonstrated substantially lower sensitivity to scrums in hookers than in props, which may be attributed to one of the primary requirements of the Catapult scrum algorithm: namely, that the MST device pitch drops below horizontal during candidate events, in order to progress to the second (random forest classifier) stage of analysis. Given that significant hip flexion is employed when hooking the ball in scrums, and both arms are bound around props’ shoulders, this may limit the extent to which hookers’ torsos drop towards, or below, the horizontal plane. An additional consideration is that different tactics (such as quickly securing the ball versus forcing an opposition infringement) are likely to influence scrummaging kinematics. If the front row drive their opposition upwards, then the likelihood of the MST device pitch dropping below horizontal may feasibly be reduced, leading to a false negative algorithm result.
The sensor fusion process (Bergamini et al., Citation2014) underpinning CI detection considers each MST unit as an isolated entity. That is, random forest-plot analysis considers only the accelerometer, gyroscope and magnetometer data of a given player for determining the likelihood of that player having experienced a CI. However, we propose that considering the actions of nearby players may improve future CI detection. RU scrums are typically contested by eight players per side, in a generally predictable formation (three front row, two second row, and three back row players). Therefore, if only some of the forward positions satisfy the requirements for scrum detection by MST, but other forwards are in close proximity (determined via GPS), then they are almost certain to have also been involved in the scrum. This proposed example of team-level sensor fusion could feasibly increase the sensitivity of MST scrum detection, particularly benefitting positions such as the back row which are prone to lower sensitivity due to spending less time in the necessary horizontal orientation (Chambers, Gabbett, & Cole, Citation2019).
Contrary to previous criticism of video analysis as a potentially inaccurate assessment method (Chambers, Gabbett, Gupta, et al., Citation2019; Reardon, Tobin, Tierney, & Delahunt, Citation2017; Tierney et al., Citation2020), this study demonstrated that video analysis can generally be conducted with satisfactory intrarater reliability. Scrum assessment showed perfect intrarater reliability, most likely due to scrums being a discrete skill. Lower reliability was observed for ball carry and tackle effectiveness, despite the count of all ball carry and tackle events both showing excellent reliability. This was most likely due to subtle distinctions between the gradings of these skills requiring a greater degree of subjective judgement. No tries were scored by the team of interest during the re-coded match. However, given the binary nature of try scoring, it is strongly expected that tries would show perfect reliability. Due to their relative infrequency, minor inaccuracies in coding clean breaks had a substantial detrimental impact on the observed reliability. Latch events had poor to good reliability, potentially due to the subtle temporal distinction between a latch (binding prior to tackle completion) and attacking ruck hit (arriving after tackle completion).
The large variation observed in intrarater reliability of non-performance collisions is most likely attributable to the novel inclusion of this variable during video analysis. Despite analysing four angles of video footage, non-performance collisions may have been overlooked. It is speculated that with further coding of a larger number of matches, non-performance collisions would show greater reliability.
Although video analysis was used as a reference comparator, and broadly demonstrated acceptable reliability, it is important to acknowledge the limitations of this method in RU. In the present study, all instances of skill performance were additionally coded as either “collision event” or “non-collision event”. This was deemed crucial to ensure that MST devices were being validated against actual CI. However, this distinction would not routinely be made in the context of the squad used. Our results demonstrated that approximately 7% of attacking ruck involvements did not incorporate a video-coded CI. These instances may represent so-called “caterpillar” rucks, in which players bind on to increase the length of the ruck, protecting the scrum half as they execute a box kick. Players joining caterpillar rucks are unlikely to experience significant, if any, CI, but these would still be coded as attacking ruck hits.
The adoption of instrumented mouthguard (iMG) technology could offer a further alternative to video/MST assessment of CI (Waldron et al., Citation2021). In principle, the tight fit of iMGs to players’ upper dentition should limit erroneous movement and may therefore provide superior surveillance of impacts at the level of the head or neck, with implications for sports medicine practitioners. In both laboratory and field-based assessments of validity, iMGs have demonstrated promising (albeit highly variable between manufacturers) sensitivity to head acceleration events (Jones et al., Citation2022). However, given iMG are – by design – optimised to measure head acceleration events, it could be speculated that they may not detect all instances of contact exposure (contact occurring in the absence of a head acceleration event). Replication of the present study’s methods in order to determine the validity of iMG to detect contact exposure across a full range of RU skills is warranted.
Considering the relative novelty of iMG, it has been noted that product technical specifications and classification algorithms are likely to continue to evolve over the coming years (Jones et al., Citation2022). Critical to this will be ensuring that classification algorithms are tailored to, and validated in, the population they are deployed in. For instance, a recent study addressed the issue of anatomical (reduced cervical spine stability) differences of female RU players by developing a population-specific classifier algorithm for female RU players (Powell et al., Citation2023). These considerations are equally relevant for MST, given that both hardware and software are likely to be refined further as the adoption of MST increases. In this regard, it is possible that the MST devices used in this study (Catapult Vector S7; Catapult Innovations) may have updated their algorithms since the analysis phase of this study, and therefore may demonstrate improved sensitivity in the future. It is hoped that the recommendations presented in this study may inform the refinement of MST algorithms to further improve their accuracy within RU. As the proliferation of technology in sport (e.g., MST, iMG) evolves, rigorous and ongoing validation is recommended as a priority for future research.
This study is the first to demonstrate the potential utility of MST in identifying lower-velocity CI such as mauls, although further study is warranted to improve understanding of positional discrepancies in maul identification. Replication of this study in a female squad should be considered, given that anatomical differences may alter skill kinematics, and therefore affect MST algorithm sensitivity. The advantages of video analysis (ability to detect multiple events in close temporal proximity, with rich contextual information) and MST (objective quantification of whether a force threshold has been met in a candidate CI) should be considered when implementing these methods in RU. For RU practitioners, a holistic approach that integrates MST and video analysis data sources is recommended for understanding performance demands and injury risk. Exclusive reliance on video analysis might lead to an overestimation of CI volume.
Using MST to monitor CI volume may lead to an underestimation of the true load players experience, particularly if body orientation does not deviate substantially during candidate CI. As MST continues to develop, consideration should be given to ensuring that multiple CI occurring in quick succession are quantified as distinct events, to ensure that CI volume is a valid representation of the demands players experience, and to facilitate comparison with data obtained by other methods (such as video analysis).
Acknowledgments
The authors wish to thank Cameron Walker, performance analyst at the Scottish Rugby Union, for his contribution to the design of the video coding protocol developed for this study.
Disclosure statement
No potential conflict of interest was reported by the author(s).
Additional information
Funding
References
- Altman, D. G., & Bland, J. M. (1983). Measurement in medicine: The analysis of method comparison studies. The Statistician, 32(3), 307–317. https://doi.org/10.2307/2987937
- Austin, D., Gabbett, T., & Jenkins, D. (2011). Repeated high-intensity exercise in professional rugby union. Journal of Sports Sciences, 29(10), 1105–1112. https://doi.org/10.1080/02640414.2011.582508
- Bergamini, E., Ligorio, G., Summa, A., Vannozzi, G., Cappozzo, A., & Sabatini, A. (2014). Estimating orientation using magnetic and inertial sensors and different sensor fusion approaches: Accuracy assessment in manual and locomotion tasks. Sensors, 14(10), 18625–18649. https://doi.org/10.3390/s141018625
- Buchheit, M., & Simpson, B. M. (2017). Player-tracking technology: Half-full or half-empty glass? International Journal of Sports Physiology & Performance, 12(s2), S2-35-S2–41. https://doi.org/10.1123/ijspp.2016-0499
- Chambers, R. M., Gabbett, T. J., & Cole, M. H. (2019). Validity of a microsensor-based algorithm for detecting scrum events in rugby union. International Journal of Sports Physiology & Performance, 14(2), 176–182. https://doi.org/10.1123/ijspp.2018-0222
- Chambers, R. M., Gabbett, T. J., Gupta, R., Josman, C., Bown, R., Stridgeon, P., & Cole, M. H. (2019). Automatic detection of one-on-one tackles and ruck events using microtechnology in rugby union. Journal of Science & Medicine in Sport / Sports Medicine Australia, 22(7), 827–832. https://doi.org/10.1016/j.jsams.2019.01.001
- Coughlan, G. F., Green, B. S., Pook, P. T., Toolan, E., & O’Connor, S. P. (2011). Physical game demands in elite rugby union: A global positioning system analysis and possible implications for rehabilitation. The Journal of Orthopaedic and Sports Physical Therapy, 41(8), 600–605. https://doi.org/10.2519/jospt.2011.3508
- Cunniffe, B., Proctor, W., Baker, J. S., & Davies, B. (2009). An evaluation of the physiological demands of elite rugby union using global positioning system tracking software. Journal of Strength & Conditioning Research, 23(4), 1195–1203. https://doi.org/10.1519/JSC.0b013e3181a3928b
- Gabbett, T., Jenkins, D., & Abernethy, B. (2010). Physical collisions and injury during professional rugby league skills training. Journal of Science & Medicine in Sport / Sports Medicine Australia, 13(6), 578–583. https://doi.org/10.1016/j.jsams.2010.03.007
- Giavarina, D. (2015). Understanding bland altman analysis. Biochemia Medica, 25(2), 141–151. https://doi.org/10.11613/BM.2015.015
- Hendricks, S., Karpul, D., & Lambert, M. (2014). Momentum and kinetic energy before the tackle in rugby union. Journal of Sports Science & Medicine, 13(3), 557–563.
- Hendricks, S., Till, K., Den Hollander, S., Savage, T. N., Roberts, S. P., Tierney, G., Burger, N., Kerr, H., Kemp, S., Cross, M., Patricios, J., McKune, A. J., Bennet, A., Rock, A., Stokes, K. A., Ross, A., Readhead, C., Quarrie, K. L., Tucker, R., & Jones, B. (2020). Consensus on a video analysis framework of descriptors and definitions by the Rugby Union video analysis consensus group. British Journal of Sports Medicine, 54(10), 566–572. https://doi.org/10.1136/bjsports-2019-101293
- Jones, B., Tooby, J., Weaving, D., Till, K., Owen, C., Begonia, M., Stokes, K. A., Rowson, S., Phillips, G., Hendricks, S., Falvey, E. C., Al-Dawoud, M., & Tierney, G. (2022). Ready for impact? A validity and feasibility study of instrumented mouthguards (iMgs). British Journal of Sports Medicine, 56(20), 1171–1179. https://doi.org/10.1136/bjsports-2022-105523
- Koo, T. K., & Li, M. Y. (2016). A guideline of selecting and reporting intraclass correlation coefficients for reliability research. Journal of Chiropractic Medicine, 15(2), 155–163. https://doi.org/10.1016/j.jcm.2016.02.012
- MacLeod, S. J., Hagan, C., Egaña, M., Davis, J., & Drake, D. (2018). The use of microtechnology to monitor collision performance in professional rugby union. International Journal of Sports Physiology & Performance, 13(8), 1075–1082. https://doi.org/10.1123/ijspp.2017-0124
- Powell, D. R. L., Petrie, F. J., Docherty, P. D., Arora, H., & Williams, E. M. P. (2023). Development of a head acceleration event classification algorithm for female Rugby Union. Annals of Biomedical Engineering, 51(6), 1322–1330. https://doi.org/10.1007/s10439-023-03138-9
- Preatoni, E., Stokes, K. A., England, M. E., & Trewartha, G. (2013). The influence of playing level on the biomechanical demands experienced by rugby union forwards during machine scrummaging. Scandinavian Journal of Medicine & Science in Sports, 23(3), e178–84. https://doi.org/10.1111/sms.12048
- Quarrie, K. L., Hopkins, W. G., Anthony, M. J., & Gill, N. D. (2013). Positional demands of international rugby union: Evaluation of player actions and movements. Journal of Science & Medicine in Sport / Sports Medicine Australia, 16(4), 353–359. https://doi.org/10.1016/j.jsams.2012.08.005
- Reardon, C., Tobin, D. P., Tierney, P., & Delahunt, E. (2017). Collision count in rugby union: A comparison of micro-technology and video analysis methods. Journal of Sports Sciences, 35(20), 2028–2034. https://doi.org/10.1080/02640414.2016.1252051
- Reardon, C., Tobin, D. P., Tierney, P., Delahunt, E., & Maher, B. (2017). The worst case scenario: Locomotor and collision demands of the longest periods of gameplay in professional rugby union. PLOS ONE, 12(5), e0177072. https://doi.org/10.1371/journal.pone.0177072
- Roberts, S. P., Trewartha, G., Higgitt, R. J., El-Abd, J., & Stokes, K. A. (2008). The physical demands of elite English rugby union. Journal of Sports Sciences, 26(8), 825–833. https://doi.org/10.1080/02640410801942122
- Roe, G., Halkier, M., Beggs, C., Till, K., & Jones, B. (2016). The use of accelerometers to quantify collisions and running demands of rugby union match-play. International Journal of Performance Analysis in Sport, 16(2), 590–601. https://doi.org/10.1080/24748668.2016.11868911
- Ross, A., Gill, N., Cronin, J., & Malcata, R. (2015). The relationship between physical characteristics and match performance in rugby sevens. European Journal of Sport Science, 15(6), 565–571. https://doi.org/10.1080/17461391.2015.1029983
- Tierney, P., Blake, C., & Delahunt, E. (2020). The relationship between collision metrics from micro‐sensor technology and video‐coded events in rugby union. Scandinavian Journal of Medicine & Science in Sports, 30(11), 2193–2204. https://doi.org/10.1111/sms.13779
- Till, K., Weakley, J., Read, D. B., Phibbs, P., Darrall-Jones, J., Roe, G., Chantler, S., Mellalieu, S., Hislop, M., Stokes, K., Rock, A., & Jones, B. (2020). Applied sport science for male age-grade rugby union in England. Sports Medicine – Open, 6(1), 14. https://doi.org/10.1186/s40798-020-0236-6
- Waldron, M., Jones, C., Melotti, L., Brown, R., & Kilduff, L. P. (2021). Collision monitoring in elite male rugby union using a new instrumented mouth-guard. Journal of Sport and Exercise Science, 5(3), 179–187.
- Williams, S., Trewartha, G., Kemp, S., & Stokes, K. (2013). A meta-analysis of injuries in senior men’s professional rugby union. Sports Medicine, 43(10), 1043–1055. https://doi.org/10.1007/s40279-013-0078-1