Abstract
An influential causal theory attributes dyslexia to visual and/or auditory perceptual deficits. This theory derives from group differences between individuals with dyslexia and controls on a range of psychophysical tasks, but there is substantial variation, both between individuals within a group and from task to task. We addressed two questions. First, do psychophysical measures have sufficient reliability to assess perceptual deficits in individuals? Second, do different psychophysical tasks measure a common underlying construct? We studied 104 adults with a wide range of reading ability and two comparison groups of 49 dyslexic adults and 41 adults with normal reading, measuring performance on four auditory and two visual tasks. We observed moderate to high test–retest reliability for most tasks. While people with dyslexia were more likely to display poor task performance, we were unable to demonstrate either construct validity for any of the current theories of perceptual deficits or predictive validity for reading ability. We suggest that deficient perceptual task performance in dyslexia may be an associated (and inconsistent) marker of underlying neurological abnormality, rather than being causally implicated in reading difficulties.
Dyslexia involves an unexpected failure to develop literacy in otherwise intelligent children. Despite decades of research, there is little consensus about the underlying cause. One class of theory attributes reading failure to a low-level perceptual deficit affecting the visual magnocellar system and possible analogous pathways in the auditory system (see Stein, Citation2001, for a review). Studies investigating this hypothesis have compared people with dyslexia and normal readers on a range of visual and auditory tasks designed to tap into relevant constructs—for example, auditory temporal processing (Tallal, Citation1980), visual/auditory magnocellular functioning (Kronbichler, Hutzler, & Wimmer, Citation2002), visual magnocellular functioning (e.g., Amitay, Ben-Yehudah, Banai, & Ahissar, Citation2002b; Lovegrove, Martin, & Slaghuis, Citation1986; Talcott et al., Citation1998, Citation2000), dynamic sensory sensitivity (Talcott et al., Citation2000; Witton et al., Citation1998), and temporal processing (Laasonen, Service, & Virsu, Citation2001, Citation2002; Van Ingelghem et al., Citation2001). In addition, several studies have investigated associations between auditory frequency discrimination and dyslexia (e.g., Ahissar, Protopapas, Reid, & Merzenich, Citation2000; McAnally & Stein, Citation1996), although the extent to which poor frequency discrimination can be regarded as a consequence of magnocellular dysfunction is debatable.
Although the magnocellular hypothesis has been influential, the evidence for a replicable weakness in the visual or auditory perceptual processing of individuals with dyslexia is difficult to interpret. The existence of an auditory magnocellular system, analogous to that found in the visual system, remains highly speculative, and the most that can be confidently claimed in respect to auditory processing is that a subset of people with dyslexia shows auditory perceptual deficits compared to those with normal reading (Ramus, Citation2003). A large body of work exploring visual magnocellular functioning has demonstrated group differences between dyslexics and individuals with normal reading on a variety of tasks (e.g., motion processing and contrast sensitivity). However, these data have not been universally accepted (e.g., see Skottun, Citation2000), and in these studies, as with those showing auditory perceptual deficits, not all dyslexic individuals show deficient performance. Also, while studies in both auditory and visual perceptual processing do demonstrate between-group differences, they very often find but do not explain markedly greater variance within the dyslexic group.
There are two possible explanations for such findings. The first, which is most commonly assumed, is that the magnocellular theory (or a similar perceptual deficit account) is valid but applies only to a subset of those with dyslexia. However, another possibility that merits further examination is that the within-group variation reflects poor reliability and/or validity of measures derived from psychophysical tasks, which are seldom evaluated for their psychometric properties. For instance, as Roach, Edwards, and Hogben Citation(2004) have noted, the distributions of scores seen in dyslexic samples may show little consistency from one testing occasion to the next; they may also be influenced by nonperceptual factors. Thus, if this field is to advance, we need to pay attention to both the reliability and the validity of the measures used to assess perceptual functioning and to obtain normative standards against which to evaluate performance of dyslexic groups.
Reliability
A key question that has not been addressed until relatively recently in the perceptual processing literature is whether threshold estimates obtained for an individual on one testing occasion are reliable, in the sense that they would be replicated on retest. Heath and Hogben Citation(2004) demonstrated moderate reliability for a task of perception of rapid auditory sequences in 7- to 12-year-old children, and Talcott and colleagues (Talcott et al., Citation2003; Talcott et al., Citation2000) have reported high interrun correlations for coherent motion in children ranging from 9 to 12 years. McArthur and Bishop's Citation(2004) data in individuals aged from 12 to 21 years suggested that their frequency discrimination measures produced stable threshold estimates. Hulslander et al. Citation(2004) showed high interrun correlations on frequency modulation (FM) detection, amplitude modulation (AM) detection, and coherent motion tasks in a similar age range. However, other studies that have explicitly addressed this issue have methodological limitations. Fischer and Hartnegg Citation(2004) reported very respectable test–retest data for five auditory tasks in children and adults, but their data were biased because a large proportion of the participants had to be excluded because they could not do the tasks even at the easiest level. The only two studies in adults by Laasonen et al. (Citation2001, Citation2002) reported internal consistency for thresholds on a temporal order judgement, but the procedure they used, of calculating split-half reliability coefficients based on the first and last six reversals in a block, is questionable, given that the data were obtained from the same adaptive run and so were not independent.
Construct validity
Construct validity involves demonstrating that the task used taps the dimension we wish to examine and can be supported by (a) showing significant relationships within individuals on two or more tasks purported to tap that dimension, and (b) demonstrating that individuals with deficits on one task (i.e., those in the poor performance “tail” of the distribution) perform similarly weakly on at least one other task presumed to capture the same dimension. Some studies have examined relationships between a small number of tasks with promising results: Talcott et al. Citation(1998) showed that thresholds for flicker sensitivity and coherent motion, two tasks that putatively capture visual magnocellular functioning, were moderately correlated in adults with dyslexia and normal adult readers; Witton et al. Citation(1998) and Talcott et al. Citation(2000) found moderately strong relationships between FM detection and coherent motion in adults and children respectively; and Van Ingelghem et al. Citation(2001) reported a moderate but significant relationship between visual and auditory gap detection thresholds in children.
Other studies have taken a more systematic approach by creating a battery of auditory and/or visual tasks (e.g., Ahissar et al., Citation2000; Amitay, Ahissar, & Nelken, Citation2002a; Amitay et al., Citation2002b; Ramus et al., Citation2003) which are assumed to capture some broad underlying dimension, then attempting to demonstrate construct validity by showing that dyslexics have deficits on all those tasks. In general, these studies with more comprehensive batteries have not provided strong support for a deficit in any underlying perceptual processing dimension. These studies also do not report reliability data. Without data on task reliability, it is unclear whether failure to find a correlation between tasks may arise because some are psychometrically weak, or whether a lack of correlation might genuinely challenge the magnocellular account or any other suggested underlying perceptual dimension.
High intercorrelations between different measures of the same construct are a first step in demonstrating construct validity, but they are not always conclusive, because the commonality between tasks could arise for quite trivial reasons, such as ability to understand task demands or adopt efficient strategies for task performance. For this reason, it is far more impressive if one finds significant correlations between tasks that have different formats. The finding by Laasonen et al. Citation(2002) of correlations averaging around .4 to .5 between various tasks assessing temporal processing in a group of 16 adults with dyslexia is perhaps the strongest demonstration to date of cross-task similarity, but the study was restricted to cross-modal tasks, and the small sample size means that there were high standard errors around these estimates.
In the context of dyslexia, it is of particular interest to consider whether those with very poor scores on one measure are also impaired on another measure of the same construct. Even where two measures are reasonably correlated across the whole range of performance, there may be only weak concordance between impairments. For instance, Heath and Hogben Citation(2004) reported moderate correlations in children with dyslexia for a task based on the Tallal Citation(1980) repetition test and a backward recognition masking task. However, individuals with poor performance on the one task were not necessarily in the tail of the distribution on the other, indicating that these individuals were not consistently poor performers across tasks purporting to assess the same construct In a similar vein, Fischer and Hartnegg Citation(2004) noted that although there were significant correlations between auditory measures of time-order judgement and frequency discrimination in their sample, there were many participants who were severely impaired on one of these measures but quite unimpaired on the other.
Normative performance
Psychophysical studies seldom adopt a standardized methodology, so where there is lack of replication across studies, it can be hard to know if this is due to differences in the participant characteristics or methodological factors. The wider psychophysical literature provides some general indication of values that can be expected in children and adults on typical tasks, and we do have some idea of how well these values can be generalized to the types of task that are used to examine deficits in dyslexics. For example Talcott et al. Citation(2002) reported normative data for both coherent motion sensitivity and frequency discrimination in 350 children unselected for reading ability between the ages of 7 and 12 years of age, and Hulslander et al. Citation(2004) examined FM and AM detection, coherent motion, and coherent form detection in a large sample ranging in age from 11 to 21 years.
Even so, although sample sizes of unimpaired individuals in most studies are typically large enough to give reasonable estimates of the mean, they are often far too small to give reliable estimates of variance within each age group, making it hard to quantify performance deficits at the individual level. An exception is the study by Fischer and Hartnegg Citation(2004), who set out explicitly to generate normative data across different age groups of normal reading and dyslexic children. However, as mentioned above, their tasks proved too difficult for a large proportion of children so their applicability is limited. Thus, we currently have limited standards against which we might sensibly compare the performance of either control or clinical groups on psychophysical measures of auditory and visual processing.
A related issue is the typical distribution patterns of psychophysical thresholds. Authors of standardized tests generally attempt to achieve normally distributed test values, but to date this has not been attempted in relation to perceptual processing tasks. McArthur and Bishop Citation(2001) and Roach et al. Citation(2004) delineated the typical distribution pattern seen in many between-group comparisons in this field: overlap across the range of threshold values between the control group and more than half the dyslexic group, but much greater variance in the dyslexic than the control group, with a subset of the clinical group spread out over a long tail at the poor performance end of the distribution. Indeed, as noted by Roach et al. Citation(2004), it is often this tail that accounts for between-group differences. This configuration is pervasive in our own results (Heath & Hogben, Citation2000; Heath, Hogben, & Clark, Citation1999; McArthur & Hogben, Citation2001) and in those of others (e.g., Ahissar et al., Citation2000; Amitay et al., Citation2002b; Talcott et al., Citation1998; Walther-Mueller, Citation1995; Witton, Stein, Stoodley, Rosner, & Talcott, Citation2002).
Criterion validity
The primary aim of research with perceptual tasks has been to examine relationships between performance on these tasks and reading. However, researchers in this area have found it notoriously difficult to demonstrate predictive or criterion validity with psychophysical tasks. As mentioned above, typically only a subset of dyslexics show deficits on perceptual tasks. Thus, in order to examine the relationships between perceptual processing and reading in those individuals with poor performance we need large samples of dyslexic people. Only a few studies have had large enough numbers to attempt this, and even Ahissar and colleagues (e.g., Amitay et al., Citation2002a, Citation2002b) have ended up with very small numbers of disabled readers who were categorized as weak across a range of perceptual tasks.
Aims of the current study
This study comprises two experiments that explored the psychometric properties of six perceptual processing tasks used in this field, each with strong claims to having revealed perceptual deficits in dyslexics. In both experiments we examined four auditory and two visual psychophysical tasks. Both visual tasks—global dot motion detection and flicker constrast sensitivity—have been used as measures of magnocellular functioning, with several studies suggesting reduced sensitivity in people with dyslexia (e.g., Amitay et al., Citation2002b; Cornelissen, Richardson, Mason, Fowler, & Stein, Citation1995; Lovegrove et al., Citation1986; Talcott et al., Citation1998; Witton et al., Citation1998). Three of the auditory tasks are designed to measure auditory temporal processing and have been regarded as indicators of integrity of analogous auditory pathways that are implicated in processing of rapid dynamic change. Frequency modulation detection has been extensively studied in relation to reading in both children and adults (e.g., Hulslander et al., Citation2004; Ramus et al., Citation2003; Talcott et al., Citation2000, Citation2003; Witton et al., Citation1998, Citation2002). Auditory backward detection masking and binaural masking level difference have been examined as tasks tapping auditory temporal processing in normal and disabled readers (e.g., Ahissar et al., Citation2000; Amitay et al., Citation2002a; McAnally & Stein, Citation1996; Protopapas, Ahissar, & Merzenich, Citation1997; Ramus et al., Citation2003; Rosen & Manganari, Citation2001). The fourth auditory task, frequency discrimination, measures spectral rather than temporal sensitivity and is not usually regarded as a magnocellular task, though McAnally and Stein Citation(1996) have suggested that it does involve temporal processing, insofar as sensitivity of a neural phase-locking mechanism might be implicated in poor frequency discrimination. Other studies have obtained variable results when studying deficits in frequency discrimination among poor readers (e.g., Ahissar et al., Citation2000; Amitay et al., Citation2002b; Cacace, McFarland, Ouimet, Schrieber, & Marro, Citation2000; France et al., Citation2002; Hill, Bailey, Griffiths, & Snowling, Citation1999; Walker, Shinn, Cranford, Givens, & Holbert, Citation2002).
In Experiement 1 we aimed to (a) describe normative performance on these six tasks by administering them to a large sample of adults with at least average nonverbal abilities unselected for reading ability; (b) examine the test–retest reliability of measurements on these tasks by comparing individual performance across blocks of trials; (c) discover on which of these tasks performance would covary within individuals when the tasks were all administered using a standardized psychophysical procedure; (d) explore relationships between these tasks and measures of component literacy skills (i.e., phonological decoding, which involves use of knowledge about letter–sound correspondences, and orthographic skills, which tap sight word knowledge) and of reading; and (e) profile these skills in any individuals who were weak in either auditory or visual perceptual processing or both.
Our aims for Experiment 2 were to (a) compare the performance of a large group of adults with dyslexia on these same tasks with that of a group of normal readers and with the normative data collected in Experiment 1; (b) examine the test–retest reliability of the tasks across blocks of trials; (c) explore relationships across tasks within the dyslexic and control groups; (d) examine the strength of relationships within the groups between performance on these tasks and measures of literacy; and (e) profile the expected proportion of individuals who were weak in either or both auditory and visual perceptual processing on the Experiment 1 literacy measures, spelling, and tests thought to capture phonological short-term memory, another area of functioning in which dyslexic individuals are said to have deficits (Hulme & Roodenrys, Citation1995; Jorm, Citation1983; Wagner & Torgesen, Citation1987).
Experiment 1
Method
Participants
A total of 104 adults aged 17 to 41 years (male = 50; female = 54) with widely varying reading ability were recruited by posters and personal contact from university and technical college campuses in Perth, Western Australia. Participants were required to have English as a first language, normal or corrected-to-normal visual acuity, a performance IQ (PIQ) on the Kaufman Brief Intelligence Test (Kaufman & Kaufman, Citation1990; KBIT) of at least 85, and bilateral pure-tone conduction thresholds of 20 dB HL or better for 1,000-Hz tones, in standard audiometric screening (Australian Standard No. 2586; Standards Association of Australia, Citation1983). Participants were paid an honorarium of A$30.
Psychometric tests
Reading accuracy and efficiency
The Test of Word Reading Efficiency (Torgesen, Wagner, & Rashotte, Citation1999; TOWRE) Sight Word Reading subtest measured speeded reading of a graded list of 104 regular and irregular words.
Component literacy skills
Phonological decoding was measured using three tests: (a) the Word Attack subtest from the Woodcock Reading Mastery Tests–Revised, Form G (Woodcock, Citation1987; WRMT-R), which requires the individual to read aloud a graded list of nonsense words in unspeeded conditions; (b) the TOWRE Phonemic Decoding subtest, which follows the same format as the Sight Word Reading subtest to measure speeded reading on a list of 63 nonsense words; and (c) The Phonological Choice Task adapted from Olson, Kliegl, Davidson, and Foltz Citation(1985). Participants were asked to indicate which of three written pseudowords (e.g., crisk/wosh/hesh) sounded like a real word. The 60 stimuli developed by Olson et al. were presented on an IBM-compatible computer run by a Matlab program, and both median latency and accuracy data were recorded for each trial.
Orthographic skills
The Orthographic Choice Task also adapted from Olson et al. Citation(1985) presented participants with adjacent pairs of letter strings. One of these was an orthographically correct real word but the other was a pseudohomophone of the word (e.g., goat/gote). The task was to identify the real word. A total of 80 orthographic choice trials were administered in a similar format to that used for phonological choice.
Measurement of auditory and visual thresholds
All tasks were presented on an IBM-compatible computer. Participants responded by pressing a keypad. A two-alternative forced-choice PEST procedure (Taylor & Creelman, Citation1967) was used to estimate the 75% correct performance level. Participants were given trial-by-trial feedback about response correctness. In the auditory tasks the words “correct” or “incorrect” appeared on the screen; in the visual tasks, a high or low tone indicated correct or incorrect, respectively. A total of 10 suprathreshold practice trials were provided for participants to learn each task. Two blocks of test trials were then administered.
The threshold estimate for each block was taken as the mean of all points after the fourth reversal, except where this value was clearly compromised by an extreme variation in stimulus level. This judgement was made by an experienced researcher blind to the participants' individual profile or group. Threshold was then calculated after a later reversal, provided at least 30 trials were available on which to calculate the threshold.
The auditory battery
Auditory testing was conducted in an acoustically shielded room. Stimuli were digitally generated on a TDT System 2 controlled by computer and presented binaurally through Sennheiser HD 265 headphones. Blocks of 70 trials were presented for all tasks except for estimation of masking level difference, where blocks consisted of 80 trials.
Frequency discrimination (FD)
A task based on the AXB design described by Mengler, Hogben, Michie, and Bishop Citation(2005) was used to measure difference thresholds. This task presents three 100-ms 85-dB SPL tones (10 ms rise/fall) separated by 300 ms. The first tone (A) was a 1,000-Hz standard, the third tone (B) was the same frequency plus the current frequency difference, and the middle tone (X) matched either the first or the third tone on a random basis. The initial stimulus difference was 30 Hz with a maximum step size of 8 Hz and a minimum step size of 0.1 Hz. The participant's task was to indicate whether the middle tone was the same as the first or last sound.
Frequency modulation (FM) detection
FM detection thresholds were measured in an adaptive version of the task described by Witton et al. Citation(1998). In our measure only the modulation depth was varied, while the rate of modulation was held constant. Participants were presented with pairs of 500-ms 81-dB SPL tones (10 ms rise/fall) separated by a 500-ms interval. One sound was a pure 500-Hz tone; the other target tone was 500 Hz, frequency modulated at a rate of 2 Hz. The order of the two tones was randomly varied from trial to trial. Initial depth of modulation in the target tone was 10 Hz, with a maximum step size of 4 Hz and a minimum step size of 0.1 Hz. The participant's task was to indicate whether the modulated tone came first or second.
Auditory backward masking (ABM)
This task was modelled on Wright et al. Citation(1997) and involved establishing threshold for detecting a tone that was immediately followed by a 300-ms 40-dB SPL burst of white noise. In each trial participants heard two 300-ms noise bursts, separated by a fixed 500-ms interval. One of these noises was preceded (interstimulus interval, ISI = 0 ms) by a 20-ms 1,000-Hz target tone initially presented at 60 dB SPL (10 ms rise/fall). The initial and maximum step size was 5 dB, with a minimum step size of 1 dB. The participant was required to indicate whether the target occurred before the first or the second noise.
Masking level difference (MLD)
In this task adapted from McAnally and Stein Citation(1996), participants' thresholds for detecting a 1,000-Hz target tone in Gaussian noise were measured. Two 500-ms bursts of 75-dB SPL white noise were presented, separated by a 500-ms interval. In each trial the target was presented either dichotically in phase or 180 deg antiphase. Participants had to indicate whether they heard the target tone during the first or second noise burst. The target was initially presented at 80 dB SPL but sound level was varied from trial to trial. Initial and maximum step size was 6 dB SPL, and the minimum was 0.1 dB SPL. Two interleaved sets of trials were used, one for in-phase and one for antiphase. The in-phase threshold was subtracted from the antiphase estimate to quantify the difference between the listening conditions. The higher the difference score, the greater the advantage the listener appears to have gained from antiphase presentation of the target tone.
Visual tasks
The stimuli for the two visual tasks were presented on a Sony Trinitron GDM-20SEI monitor, controlled by the framestore section of a Cambridge Research Systems VSG2/3. The screen was viewed binocularly in a darkened room. Blocks of 80 trials were presented for the Global Dot Motion Task, and for the Flicker Contrast Task each block consisted of 60 trials.
Global dot motion (GDM)
Stimuli similar to those described by Edwards and Badcock Citation(1996) consisted of 100 circular dots subtending 0.11 deg. The dots were randomly distributed across the screen with proximity restrictions imposed to ensure that the dots would not overlap. A total of 20 frames were each displayed for 30 ms, which resulted in a total duration of 600 ms for each trial. To eliminate local cues during detection, the coherently moving dots were chosen randomly on each frame instead of having the same dots moving coherently across all 20 frames. The remaining dots moved in a random direction, excluding the global motion direction.
The coherence of the first presentation was 20% (i.e., 20 dots were moving in the same direction). The spatial step size was 0.19 degrees, resulting in a stimulus velocity of 6.33 deg/s. After each trial the participant was asked to indicate the direction of movement (either up or down). Threshold was defined as the percentage of dots needed to detect the direction of global movement. Hence, a higher threshold on this task indicates low sensitivity.
Flicker contrast sensitivity (FCS)
This task was adapted from Evans, Drasdo, and Richards Citation(1994). In each trial two stimuli were presented. The target consisted of a Gaussian patch (SD = 3.15 deg) presented in the middle of the screen, with a temporal frequency of 10 Hz and a mean luminance of 20 cd/m2. The target was surrounded by a field of matched space-averaged luminance. In each trial, the target was presented randomly either before or after another stimulus, which had the same mean luminance but did not flicker. The temporal contrast (modulation depth) was varied from trial to trial to obtain the threshold.
Before the start of each trial, a fixation cross indicated where the centre of the target would appear. Two tones signalled separate 1,000-ms observational periods during which the target and the nonflickering stimulus appeared. Participants were required to indicate which observational period contained the target. Thresholds reflected the Michaelson constrast ratio needed to detect flicker. Thresholds for each participant were transformed to contrast sensitivity (1/threshold).
General procedure
Participants attended two sessions of approximately 90 min with appropriate breaks on separate days up to 2 weeks apart, or on the same day separated by a longer meal break. In Session 1, participants were screened for KBIT PIQ, and suitable participants then completed the visual tasks, the WRMT-R and TOWRE tests, and the audiometric screening. In Session 2, participants completed the Olson tasks and the auditory battery.
Results
presents descriptive data for the demographic and psychometric variables. As this table indicates, reading ability and educational level covered a wide range.
Table 1. Group descriptive data for Experiment 1 demographic variables, component literacy skills, and reading
Normative data for the psychophysical tasks
Where task performance is expressed as an absolute or differential threshold (i.e., for FD, FM, ABM, and GDM), distributions of individual differences were positively skewed. Therefore, data for these variables were subjected to a log transform prior to any parametric statistical analysis. The resulting distributions were approximately normal for all tasks. presents threshold values associated with a range of percentile rankings for each task. Given the large age range covered by this sample, we looked at the relationship between age and performance on these tasks. We found that there were low correlations (highest average r values ∼.2 for FD and FCS).
Table 2. Approximate raw threshold values for perceptual tasks corresponding to a range of percentiles for Blocks 1 and 2
Reliability of the psychophysical measures within individuals
shows the relationship between the two independent threshold estimates for each task. For all tasks except MLD we found statistically significant positive correlations between blocks (see ). As the use of psychophysical tasks in clinical settings is particularly concerned with identifying individuals with specific weaknesses, we also considered the consistency of poor performers between blocks. In line with the established psychometric convention of taking the normal range to extend from –1 to + 1 standard deviation, participants whose performance was ranked below the 16th percentile were deemed to be poor performers. The region of reliably poor performance (i.e., where an individual had a percentile rank of less than 16 on both blocks) is indicated by the shaded region in the plots in . Ideally, our measures would identify the same group of poor performers in each task, resulting in around 15% of the sample (between 15 and 16 individuals) occupying the shaded region for each task. At worst, with complete independence between estimates, we would expect around 2% (between 2 and 3 individuals) to perform poorly on both blocks. Again, MLD was the weak measure since only 2 individuals could be reliably identified as poor. The other five measures all show reasonable consistency, especially FD and FCS, where the number of individuals falling in the tail of the distribution on both blocks was 12 and 10, respectively.
Figure 1. Relationships between threshold estimates obtained on Blocks 1 and 2 for each task in Experiment 1 (N = 104). FD = frequency discrimination (Hz); MLD = masking level difference (dB SPL); FM = frequency modulation (Hz); ABM = auditory backward masking (dB SPL); GDM = global dot motion (percent); FCS = flicker contrast sensitivity (1/threshold). Dotted lines represent the cut-offs for poor performance (16th percentile) on each task. Shaded quadrant shows the region of reliably poor performance, with number of individuals in each quadrant shown within shaded area. Pearson correlation coefficients are shown in frames (all were significant with p < .008, except MLD).
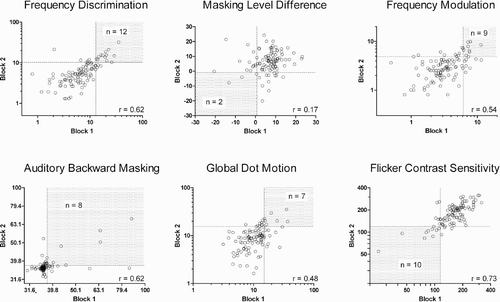
Relationships between the psychophysical tasks
Correlations between tasks were mostly quite weak, and after applying a Bonferroni correction (αFW = .05) only the relationship between FD and FM remained significant (r = .40 for both blocks). To address the question of whether poor performance covaries between tasks, we calculated the number of tasks that each participant performed poorly. As some overlap between tasks would be expected purely by chance, the resulting frequency distribution was compared against predictions assuming complete statistical independence between tasks. As the likelihood of being a poor performer on a single block of any task is fixed at 0.15, the probability of an individual being poor at n tasks out of 6 is given by:
Figure 2. Frequency distributions of the number of psychophysical tasks performed poorly (a) on each testing block and (b) reliably across both blocks. The solid lines are not fits to the data. Rather, they show chance predictions assuming complete statistical independence between tasks.
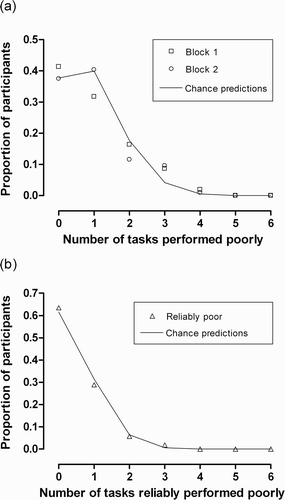
Given that a certain amount of error is associated with any single threshold estimate, it seemed plausible that the noise associated with classifying poor performance based on a single block of trials might have masked any systematic relationships between the tasks. To limit this potential problem, we repeated the analysis for individuals who were reliably poor performers on each task. Restricting the analysis to individuals who repeatedly fall in the tail of the distribution should act to increase the robustness of the poor performance classification and increase the sensitivity to pick up any patterns of covariation between tasks. As the probability of an individual being reliably poor varied between tasks, chance predictions were obtained by a Monte Carlo simulation incorporating the separate base rates for each task. Results for this analysis are shown in the lower panel of . Again, the distribution of reliably poor performance closely adheres to expectations based on complete independence between tasks.
These results suggest an absence of any systematic covariation of poor performance across psychophysical tasks. Additional support for this conclusion was found when we looked more closely at the profiles of individuals who happened to be poor at multiple tasks, either in particular blocks or reliably across blocks. In each case there was little consistency in the combinations of tasks performed poorly by participants. Even for the FD–FM pairing, of the 12 participants who were reliably poor at FD and the 9 who were reliably poor at FM, only 3 participants had conjoint deficits.
Relationships between the psychophysical measures and reading ability
Scores for the component literacy skills and reading measures were highly intercorrelated. Although we selected measures that aimed to separately index phonological and orthographic literacy skills, a principal components analysis revealed one dominant component loading on all seven measures that accounted for nearly half of the variance in scores. The ability of psychophysical task performance to predict reading skills was assessed by multiple regression on this extracted reading factor. In order to control for general performance abilities we first entered KBIT PIQ to the regression model, then observed the additional variance in reading ability that was explained when the psychophysical measures were added as a second stage. Separate regressions were run for each of the two blocks of threshold estimates. Block 1 psychophysical measures accounted for an additional 10% of variance in reading ability, whereas Block 2 measures explained an additional 12% of variance. Squared semipartial correlations revealed that of the six psychophysical measures, only FD uniquely explained significant amounts of variance in reading (5.01% for Block 1 and 6.35% for Block 2).
Discussion
We have demonstrated moderate reliability across different testing occasions for all tasks except MLD, with FD, ABM, and FCS being the most reliable. Our coefficient for the GDM task is lower than that reported in children by Talcott et al. Citation(2000) and Talcott et al. Citation(2003). However, we point out that the coherent motion task used in those studies is a very different task from the present one. The poor reliability of MLD is most likely related to the use of a difference score to quantify the effect. When the components of a difference score are positively correlated, the reliability of the difference is often less than the average reliability of the two components (Cronbach & Furby, Citation1970). The correlations for the antiphase and in-phase thresholds were of similar strength to those for the other psychophysical tasks, and the intercorrelation between these components was moderate (r = .46 and r = .44 for the two blocks).
Regarding construct validity, the assumption that these tasks may be tapping some pervasive underlying perceptual processing dimension (e.g., magnocellular processing), or even a more limited domain (e.g., auditory or visual processing) was not supported. The only two tasks that were significantly correlated within this sample were FD and FM, which were moderately related across both blocks. On the surface, this might suggest that in this sample of adults unselected for reading ability, both these tasks are capturing some sort of auditory domain, possibly related to the perception of small differences in frequency (cf. Ahissar et al., Citation2000). However, when attention was focused on participants with unusually poor performance, results on even these two tasks were not consistent. Overall, when we examined the proportion of participants who performed reliably poorly on any given number of tasks, this was at chance level, suggesting that there was little systematic effect within the participants leading to poor task performance.
We were not able to demonstrate predictive or criterion validity for any of the tasks. There was little evidence that the psychophysical tasks were meaningfully related to individual differences in reading ability. Indeed, our brief PIQ measure accounted for almost as much variance in reading ability as the combined total of our six psychophysical tasks. Despite the reasonable statistical power afforded to us by the size of the sample, only FD uniquely accounted for significantly more than zero variance.
In sum, we found that in a large sample of adults unselected for reading ability, at least some of our measures were tolerably reliable, but they did not appear to be capturing the constructs that previous research has argued for, and poor individual performance was not related to reading and literacy skills. However, the possibility remains that deficiencies in reading and psychophysical tasks will be more closely related in people with dyslexia. This was the principal focus of Experiment 2.
Experiment 2
Method
Participants
A total of 49 adults (male = 24; female = 25) who reported a childhood history of literacy problems and/or a previous diagnosis of dyslexia were recruited to the dyslexic group in a similar way and with identical selection criteria to those for Experiment 1. A total of 41 adults with normal reading (male = 10; female = 31) were also recruited in this manner. Participants in the two groups were further required to rank on the TOWRE Phonemic Decoding subtest below the 16th percentile for the dyslexic group and above the 40th percentile for the control group. Because the age bands for this test extend only to 24 years 11 months, cut-offs (dyslexic group: raw score ≤ 44; control group: raw score ≥ 53) were based on percentiles generated from the Experiment 1 sample.
Psychometric tests
Reading and component literacy skills
Tests of reading accuracy and efficiency and component literacy skills were all administered as described in Experiment 1.
Spelling
The Spelling subtest from the Wide Range Achievement Tests–3rd Edition, Tan Form (Wilkinson, Citation1993; WRAT-3) was administered according to the standardized directions.
Phonological short-term memory (PSTM)
Three aspects of PSTM were assessed using the Memory for Digits and Nonword Repetition subtests from the Comprehensive Test of Phonological Processing (Wagner, Torgesen, & Rashotte, Citation1999; CTOPP) and the Memory for Sentences subtest from the Stanford Binet Intelligence Scale–4th Edition, Australian Adaptation (Thorndike, Hagen, & Sattler, Citation1986), all administered according to the test instructions.
Psychophysical measures
The six psychophysical tasks were administered according to the procedures reported for Experiment 1. As for the first experiment, raw thresholds are reported as descriptive data, but these values were log transformed for any inferential analyses.
Results
Demographic and psychometric data are presented in . On average, both groups in this experiment were slightly older than the group in Experiment 1. The control group was a little younger than the dyslexic group, stronger on the KBIT matrices and somewhat better educated. Group differences in PIQ and years of education were statistically significant. Since the KBIT matrices mean for the dyslexic group was at the 63rd percentile, and the range of scores overlapped almost completely, the difference in PIQ was not thought to be an issue. Notwithstanding, the effect size was relatively large (Cohen's d = 0.7), so we controlled for PIQ in any between-group analyses of the dependent variables. As would be expected, the dyslexic group had spent fewer years on average in education. The mean number of years of education for the dyslexic group was 12 years, which suggests that fewer of this group had gone on to extended tertiary education. Between-group differences were as would be expected in these groups for component literacy skills, reading, spelling, and phonological short-term memory.
Table 3. Group mean values for control and dyslexic groups in Experiment 2 on demographic, psychometric and component reading skills
Reliability of the psychophysical measures
As shown in , Block 1 to Block 2 correlations for the two groups combined were fairly consistent with those for the Experiment 1 sample. Reliability of the FD and ABM tasks was slightly stronger in this sample, whereas GDM measurements were slightly less reliable. Once again, the MLD task did not appear to deliver consistent threshold estimates from one block to the next. All correlation coefficients except for MLD and GDM were significant (α = .05). shows that similar patterns were reflected within the groups as well. The only task where this was not the case was GDM: There was no relationship between performance on Blocks 1 and 2 in the control group.
Table 4. Pearson correlation coefficients between blocks on the psychophysical tasks in Experiment 2
Differences between dyslexic and normal readers
shows summary statistics for each of the two groups on the psychophysical tasks. Mean performance levels for the dyslexic group were consistently poorer than that for the normal readers on all tasks. Analysis of variance performed on the log transformed thresholds with PIQ as a covariate revealed significant differences between the group means on both blocks for two of the tasks—FD and GDM—and on Block 2 for FM. However, as can be seen in , there was substantial overlap between performance levels of individuals in each group. Accordingly, the differences in mean performance seen between the groups cannot be thought of as characteristic of all individuals within the sample.
Figure 3. Relationships between threshold estimates obtained on Blocks 1 and 2 for each task in Experiment 2, both groups combined (N = 90). FD = frequency discrimination (Hz); MLD = masking level difference (dB SPL); FM = frequency modulation (Hz); ABM = auditory backward masking (dB SPL); GDM = global dot motion (percent); FCS = flicker contrast sensitivity (1/threshold). Dotted lines represent the cut-offs for poor performance (16th percentile) on each task, based on Experiment 1. Shaded quadrant shows the region of reliably poor performance, with number of individuals in each quadrant shown within shaded area. Pearson correlation coefficients for each group are shown in .
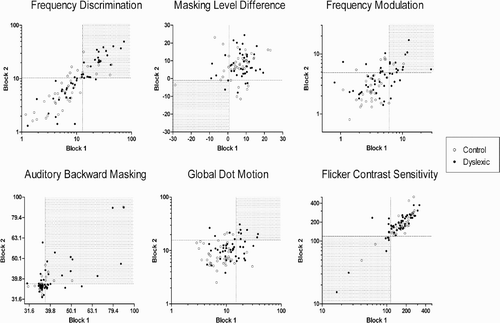
Table 5. Descriptive data for Blocks 1 and 2 on psychophysical tasks in Experiment 2
An alternative analytical approach is to consider the frequency of individuals within each group who perform poorly on a given task. The region of reliably poor performance is shaded for each task in (i.e., at or below the 15th percentile compared to normative data from Experiment 1 on both blocks). In assessing the evidence for deficient performance in dyslexia, we need to consider both the sensitivity and the specificity of the tasks. Ideally, a task would identify a large proportion of dyslexic individuals as poor performers (good sensitivity), while identifying a relatively low proportion of normal readers (good specificity). shows the frequency of reliably poor performers for each of the tasks. For all tasks the proportion of poor performers is greater in the dyslexic group than in the normal reader group. In particular, GDM, FM, and FD show reasonable utility in discriminating between individuals in each group with credible effect sizes in each case ().
Table 6. Participants performing at or below the 15th percentile on the psychophysical tasks in Experiment 2
Relationships between the psychophysical tasks
Mirroring the results in the unselected sample, correlations computed between the psychophysical tasks suggested that only the FD and FM measures are substantially related. After a Bonferroni correction, performance on these tasks was significantly related across the whole sample: Block 1, r = .57, p < .003; Block 2, r = .51, p < .003. This pattern was preserved within the groups, although not all coefficients reached this stringent level of significance: control group: Block 1, r = .45, p < .003; Block 2, r = .54, p < .003; dyslexic group: Block 1, r = .61, p < .003; Block 2, r = .42, p > .003.
To assess the possibility that poor performance might covary between tasks, we again calculated the proportion of participants in each group who were poor at a given number of tasks. In line with findings in unselected readers (see Experiment 1), frequency distributions for the group of normal readers closely adhered to chance predictions assuming complete independence between tasks (see , left). Results for the dyslexic group are shown in the right-hand panels of . Compared with normal readers, the centre of mass of the frequency distributions for the dyslexic group is shifted rightward, reflecting a greater proportion of individuals performing poorly at multiple tasks. This is to be expected, as the frequency of poor performance was higher in the dyslexic group for all six tasks. When these higher base rates were taken into consideration, chance predictions provided a reasonable fit to the data, though not as tight as that seen previously.
Figure 4. Frequency distributions of poor performance on multiple psychophysical tasks for control and dyslexic participants. The upper panels show the results when each testing block is treated separately; the lower panels show the results restricted to consistently poor performance across both blocks. Predictions assuming complete independence between tasks are shown in each case, taking into account the rates of poor performance on each task.
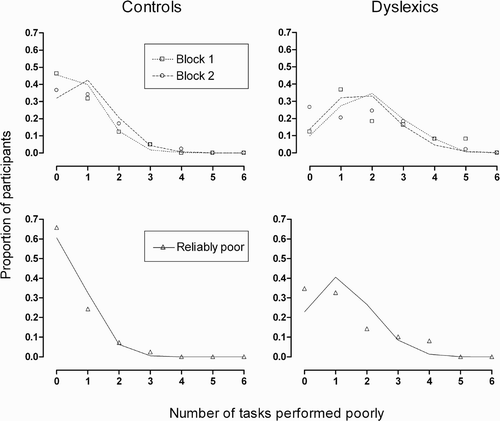
As the poor performance data for the dyslexic group showed some evidence of departing from statistical independence, we investigated the patterns of covariation in more detail. To do this, we calculated the number of times that reliably poor performance co-occurred for each pairwise combination of tasks. The resulting frequencies could then be compared to chance predictions obtained by multiplying the proportion of dyslexic individuals who were reliably poor at each of the two tasks. As shown in , results reveal a tendency for poor performance to co-occur slightly more than would be expected by chance in most instances. There is no evidence of a particularly strong association between any set of tasks. Rather, there is a tendency for poor performers on any given task to be more likely to perform poorly on most other tasks.
Table 7. Pairwise analysis of reliably poor performance by dyslexic participants on multiple tasks in Experiment 2
Relationships between the psychophysical and psychometric measures
To explore associations between psychophysical task performance and the psychometric profiles of the dyslexic sample, we first conducted a principal components analysis on the reading, spelling, and phonological short term memory test results. shows the resulting component matrix following a varimax rotation. Three factors were extracted, accounting for a total of 69.49% of variance in the test measures. The first loaded most heavily on measures of reading and spelling accuracy, contributing 31% of the total variance. A further 21.81% of variance was explained by the second factor, which isolated the three memory measures. The third factor loaded primarily on the response latency measures for the Olson phonological and orthographic choice tasks, as well as on the speeded TOWRE sight word efficiency. We termed these factors Reading/Spelling Accuracy, Phonological STM, and Reading Speed, respectively.
Table 8. Factor loadings from principal components analysis on literacy tasks
Correlations between these extracted psychometric factors and performance on psychophysical tasks were generally small. Following a Bonferroni correction for multiple correlations, only the association between FD and the Reading/Spelling Accuracy factor remained significant. Coefficient values were remarkably stable across testing blocks (r = –.50 for Block 1, r = –.45 for Block 2, p < .004, in both cases). However, examination of the scatter plots for Block 1 and 2 FD thresholds versus the Reading/Spelling factor suggested that these relationships were not particularly robust.
A similar result was found when we looked at relationships between the psychometric factors and reliably poor performance on each psychophysical task. The dyslexic group was split at the median for each of the three psychometric factors, and the frequency of reliably poor task performance in each subgroup was compared against chance predictions. shows a cross tabulation of reliably poor performance on each task and low factor scores. Reliably poor FD and low reading/spelling accuracy stands out as the only combination producing a substantially higher conjoint frequency than would be expected by chance.
Table 9. Pairwise analysis of reliably poor task performance and low scores on three psychometric factors for dyslexic participants in Experiment 2
Profiles of reliably poor and good FD performers in the dyslexic group
Finally, we examined the psychometric profiles of the dyslexics who were reliably poor at FD (poor FD subgroup; N = 21) with those who were not (good FD subgroup; N = 28). The good FD subgroup was significantly stronger nonverbally (mean KBIT PIQ = 109.0, SD = 9.2) than the poor FD subgroup (mean = 100.1, SD = 6.7); t(47) = 3.7, p = .001. We also found that the profiles of these two groups reflected the factors described above: That is, the groups differed significantly, or almost so, on four of the five measures that loaded heavily on the Reading/Spelling factor. These were Woodcock Word Attack (poor FD, mean scaled score = 83.8, SD = 7.1; good FD, mean scaled score = 90.7, SD = 10.4); t(47) = 2.6, p = .013, TOWRE Phonemic Decoding (poor FD, mean scaled score = 70.0, SD = 8.4; good FD, mean scaled score = 75.0, SD = 8.6); t(47) = 2.0, p = .050, WRAT-3 Spelling (poor FD, mean scaled score = 80.1, SD = 14.9; good FD, mean scaled score = 91.6, SD = 13.1); t(47) = 2.7, p = .010, and Olson Phonological Task Accuracy (poor FD, mean correct/60 = 33.0, SD = 10.3; good FD, mean correct/60 = 43, SD = 9.4); t(47) = 3.4, p = .001. However, these subgroups also differed significantly on one of the measures that loaded on Factor 3 in the principal components analysis, Olson Phonological Task Latency (poor FD, mean = 3,650.4 ms, SD = 945.9; good FD, mean = 2,931.4 ms, SD = 876.1); t(47) = 2.7, p = .009. The subgroups did not differ significantly on any of the memory measures that loaded on Factor 2, or the efficiency measures that loaded on Factor 3 (i.e., TOWRE Sight Word, and Olson Orthographic Task Latency). However, they also showed no significant differences on Olson Orthographic Task Accuracy. In summary, we found that the poor FD and good FD subgroups differed on measures of phonologically based literacy skills (largely loading onto the Reading/Spelling factor), and did not differ on phonological memory, orthographic skills or Reading Speed. It is noteworthy that when we examined the subgroup of 7 control participants who were reliably poor in FD, the means and standard deviations for the five literacy tasks on which the two dyslexic subgroups differed were almost identical to those of the rest of the control group. This suggests that the apparent relationship between poor FD and weaker literacy is in some way specific to dyslexics.
Discussion
The distributions on the psychophysical tasks for our two comparison groups mapped very closely onto the normative distributions obtained in Experiment 1. We found almost identical patterns of test–retest reliability to those in the normative sample, both when the two groups were combined and when we looked within the groups. That is, FD, ABM, and FCS were strongly reliable measures, FM was moderately reliable, and the MLD task was not reliable. We found GDM in this experiment to be still less reliable than that in Experiment 1: There was a very weak block-to-block relationship within the control group and overall a weaker relationship between performance levels across blocks. We were not able to explain this apparently anomalous result other than possible sampling variation.
When we compared the groups on the psychophysical tasks, our results followed the patterns commonly seen in this field. There were significant between-group differences in at least some of the variables (i.e., FD, FM, and GDM), but these differences were due to some very large threshold values in the dyslexic group, with overlap in scores between the groups, as usually observed in this field. Nevertheless, when we examined the sensitivity and specificity of the tasks, these three did discriminate tolerably well between individuals in the groups. Thus, only FD and FM showed reasonable sensitivity, specificity, and reliability.
However, when we attempted to identify coherent patterns of weaknesses across individuals, we were unable to demonstrate validity for any specific perceptual construct that might explain poor performance on these tasks. Although the number of dyslexic individuals showing conjoint deficits on FD and FM was slightly higher than would be expected by chance, this was also true of most other pairwise combinations of tasks. Indeed, compared to other dyslexic individuals, poor performers on FD were also more likely to perform poorly on ABM, GDM, and FCS. In this situation it is very difficult to infer support for a deficiency in any particular perceptual ability based on poor performance on a particular combination of tasks. Given the generality of the patterns of conjoint poor performance observed, it seems likely that the only common processes or abilities that are being captured relate to generic difficulties with performing tasks.
Although the psychophysical tasks employed here do not seem to converge on a set of underlying perceptual constructs, this does not preclude the possibility that some may be valid measures of specific aspects of perceptual functioning. In principle the differences between normal and dyslexic readers on FD, FM, and GDM could reflect the existence of independent perceptual weaknesses in the dyslexic group. One way of validating this interpretation would be to demonstrate that failure on each of the tasks is related to a separate aspect of the psychometric profiles of dyslexic individuals. Analysis of our psychometric battery revealed three independent components providing a reasonable delineation of three areas of weakness in dyslexia: reading/spelling accuracy, phonological short-term memory, and reading speed. Within the dyslexic group, FD exhibited a robust correlation with the reading/spelling accuracy component. However, none of the other tasks was related to any of the three components.
When we examined the profiles of poor FD and good FD performers, we found that the patterns were consistent to some extent with the proposal by Ben-Yehudah, Banai, and Ahissar Citation(2004) and Banai and Ahissar Citation(2004) that there are two subgroups of dyslexics, distinguished by their performance on FD. However, the difference here appears to be primarily in the phonological processing that directly underpins literacy, rather than working memory as these authors have suggested.
General discussion
Our two studies provide a unique opportunity to evaluate perceptual deficit accounts of dyslexia because they incorporate several important design features that have not hitherto been combined in a single investigation. First, the sample size of nondyslexic individuals was large enough to allow us to quantify perceptual deficits in relation to a normative standard; second, the sample of people with dyslexia was large enough to make it possible to look at correlates of perceptual deficits within the dyslexic group; third, we tested all participants on two occasions, so could establish test–retest reliability for our tasks and identify people with consistently poor performance; and finally, we tested participants on two visual and four auditory tasks, making it possible to test the construct validity of perceptual impairment accounts of dyslexia.
Three main findings emerged from our study. First, we confirmed that dyslexia in adults is associated with a deficient performance on certain psychophysical tasks, most notably auditory frequency discrimination. Second, like previous researchers, we found that these deficits affected only a minority of participants. Previous studies have reported similar results but have left open the question of whether poor reliability of measures might be involved in these inconsistent findings. We were able to show that the reliability of most of the psychophysical tasks was good, and most people with dyslexia who showed poor performance on FD, FM, ABM, or FCS on an initial testing block continued to do poorly on a second session. This suggests that these tasks are capturing some stable dimension within a minority of dyslexic individuals, and therefore these tasks are useful for quantifying individual differences. Furthermore, performance on the FD task related systematically to performance on literacy measures, and this relationship was evident only in measures tapping phonological processing, which many researchers would now accept as the core deficit for the majority of dyslexic people. Third, the present data do not add to the extant support for a construct of a magnocellular deficit in dyslexia. Granted we have only two measures of magno processing; we were also only able to demonstrate moderate reliability for one of these measures (i.e., GDM) in our unselected sample and a lower level of reliability in dyslexics but not in our control group of normal readers. Even so, there seemed to be little support here for this construct—or indeed for any other overarching explanatory factor that loaded on the different perceptual tasks. Correlations between the different auditory and visual measures were weak, and, with few exceptions, deficits in the different tasks were not associated at above-chance levels. In addition, frequency discrimination, for which the data are least supportive of a magnocellular explanation, was the task that seemed most strongly related to reading ability.
Two related questions are raised by this pattern of findings. First, what is the underlying basis of the link between poor frequency discrimination and low literacy, and second, how can we explain the fact that on the one hand there are significant associations between perceptual deficits and dyslexia, and on the other hand there is no consistency in the pattern of deficits within individuals across different perceptual tasks?
Considering first the FD task, we may ask why are deficits on this measure more closely linked to dyslexia than are deficits on other tasks. Although most of the literature on perceptual impairments in dyslexia has focused on temporal processing, our findings join a growing body of work suggesting that poor FD is a correlate (albeit very imperfect) both of dyslexia and of the related disorder of specific language impairment (e.g., McAnally & Stein, Citation1996; McArthur & Bishop, Citation2004; Mengler et al., Citation2005). Roach et al. Citation(2004) suggested that the typical patterns seen in dyslexic groups could simply result from their having general difficulties with performing tasks. That is, they are more prone to give errant responses and so are generally less reliable at task performance. However, Roach et al.'s simulation data demonstrated that an individual's measured threshold may at one time approximate the true threshold and at another constitute a gross overestimate of the true value. Therefore, this type of explanation cannot explain the findings of consistent poor performance on the FD task. Our results suggest instead that the FD task is capturing some other stable processing variable. A strong test of this interpretation would be to retest impaired individuals: If poor performance does reflect some enduring deficiency, data from Heath and Hogben Citation(2004) with a version of the original Tallal Citation(1980) auditory repetition task suggest that those individuals would remain within the tail of the distribution, even after extended practice on this task. An alternative explanation of poor FD was proposed by Amitay et al. Citation(2002b), who suggested that people with dyslexia who had difficulty discriminating both auditory and visual spatial frequencies did so because the “retain and compare” paradigm that they used loads heavily on perceptual memory. We do not think that this explains the patterns in our data: The suggestions of France et al. Citation(2002) lead us to believe that our 3-interval AXB paradigm might minimize demands on memory, although this is difficult to verify without directly assessing these demands. Furthermore, if weak perceptual memory were implicated, we might expect to see correlated patterns of impairment on a range of perceptual tasks, and we did not. For instance, in our study, only 4 of the 21 dyslexic participants who were poor performers in FD were also reliably poor in FCS (see ). An alternative line of explanation regards poor FD as evidence of genuine limitations of frequency resolution. Such a deficit could plausibly impact on speech discrimination, by compromising perception of voice pitch or formant frequencies in speech. Such an explanation could be contradicted by the fact that—at least when we used a threshold of 1 SD to determine the presence of a deficit or otherwise—there is a subset of people who are reliably poor at FD and yet have no reading deficits (see ). In future studies with FD, a more conservative threshold of 1.5 SD might constitute a clearer boundary, which is not crossed by control participants. Even so, profiling of these control participants on the literacy and literacy-related tasks that differentiated dyslexics who were reliably poor at FD from those who were not suggested that these readers may simply be well compensated for earlier reading problems that may have been associated with FD originally.
Our second question, of why perceptual deficits are so inconsistent in dyslexia, has been considered by Ramus and colleagues (Ramus, Citation2004; Ramus et al., Citation2003), who obtained data similar to ours in a sample of 16 adult dyslexics compared with 16 controls. They too found that on a range of perceptual and motor tasks, a minority of people with dyslexia were impaired, but there was no consistent pattern in the profile of impairments. Ramus Citation(2004) suggested that under certain hormonal conditions, people with dyslexia may have disrupted neural functioning secondary to focal cortical anomalies. He has speculated on the effects of these anomalies on sensory pathways in the thalamus, posterior parietal cortex, and cerebellum, producing a sensorimotor syndrome in some dyslexics. Where disruption is more widespread, one might find people with dyslexia being generally less reliable at tasks that make significant neurological demands. The subgroup of poor FD dyslexic individuals in this study do certainly appear to show this pattern of marked weaknesses in tasks that arguably demand significant cognitive resources. It may be that affected individuals appear to have specific perceptual deficits on tasks that make the greatest demands neurologically (e.g., FD). This type of account is reminiscent of early suggestions by Denckla Citation(1977) that at least some people with dyslexia suffer a more generalized neurological debility. Although the precise mechanism remains highly speculative, the key feature of this line of explanation is that the perceptual deficits observed in dyslexia are not causally implicated in the reading and phonological deficits, but rather are variable correlates, which are themselves caused by the same factors that compromise the brain's ability to acquire literacy.
It has been the practice in earlier studies to generate a plausible perceptual explanation of whatever deficit is found in a particular data set. We believe that the apparent absence in such studies of any consistent perceptual deficit is the pattern to which we need to attend. Our results urge a change in direction in this field. In future our efforts should be directed to discovering how the tendency to greater variability and poorer task performance is related to literacy failure, and to do this we need to consider both the stability of perceptual deficits in individuals over time and the interrelationship between deficits on different tasks.
This research was supported by an Australian Research Council grant to Hogben and Bishop.
References
- Ahissar , M. , Protopapas , A. , Reid , M. and Merzenich , M. M. Auditory processing parallels reading abilities in adults . Proceedings of the National Academy of Sciences . Vol. 97 , pp. 6832 – 6837 .
- Amitay , S. , Ahissar , M. and Nelken , I. 2002a . Auditory processing deficits in reading disabled adults . Journal of the Association for Research in Otolaryngology , 3 : 302 – 320 .
- Amitay , S. , Ben-Yehudah , G. , Banai , K. and Ahissar , M. 2002b . Disabled readers suffer from visual and auditory impairments but not from a specific magnocellular deficit . Brain , 125 : 2272 – 2285 .
- Banai , K. and Ahissar , M. 2004 . Poor frequency discrimination probes dyslexics with particularly impaired working memory . Audiology and Neuro-otology , 9 : 328 – 340 .
- Ben-Yehudah , G. , Banai , K. and Ahissar , M. 2004 . Patterns of deficit in auditory temporal processing among dyslexic adults . Neuroreport , 15 : 627 – 631 .
- Cacace , A. T. , McFarland , D. J. , Ouimet , J. R. , Schrieber , E. J. and Marro , P. 2000 . Temporal processing deficits in remediation-resistant reading-impaired children . Audiology and Neuro-Otology , 5 : 83 – 97 .
- Cornelissen , P. , Richardson , A. , Mason , A. , Fowler , S. and Stein , J. 1995 . Contrast sensitivity and coherent motion detection measured at photopic luminance levels in dyslexics and controls . Vision Research , 35 : 1483 – 1495 .
- Cronbach , L. J. and Furby , L. 1970 . How we should measure “change”—or should we? . Psychological Bulletin , 74 : 68 – 80 .
- Denckla , M. B. 1977 . “ Minimal brain dysfunction and dyslexia: Beyond diagnosis by exclusion ” . In Topics in child neuropsychology , Edited by: Blaw , M. E. , Rapin , I. and Kinsbourne , M. New York : Spectrum Publications Inc .
- Edwards , M. and Badcock , D. R. 1996 . Global-motion perception: Interaction of chromatic and luminance signals . Vision Research , 36 : 2423 – 2431 .
- Evans , B. J. W. , Drasdo , N. and Richards , I. L. 1994 . An investigation of some sensory and refractive visual factors in dyslexia . Vision Research , 34 : 1913 – 1926 .
- Fischer , B. and Hartnegg , K. 2004 . On the development of low-level auditory discrimination and deficits in dyslexia . Dyslexia , 10 : 105 – 118 .
- France , S. J. , Rosner , B. S. , Hansen , P. C. , Calvin , C , Talcott , J. B. Richardson , A. J. 2002 . Auditory frequency discrimination in adult developmental dyslexics . Perception & Psychophysics , 64 : 169 – 179 .
- Heath , S. M. and Hogben , J. H. 2000 . Auditory temporal processing, phonological awareness and oral language ability in prereaders: Can we identify children at risk for reading disability more accurately? . The Australian Educational and Developmental Psychologist , 17 : 63 – 75 .
- Heath , S. M. and Hogben , J. H. 2004 . The reliability and validity of tasks measuring perception of rapid sequences in children with dyslexia . Journal of Child Psychology and Psychiatry , 45 : 1275 – 1287 .
- Heath , S. M. , Hogben , J. H. and Clark , C. D. 1999 . Auditory temporal processing in disabled readers with and without oral language delay . Journal of Child Psychology and Psychiatry , 40 : 637 – 647 .
- Hill , N. I. , Bailey , P. J. , Griffiths , Y. M. and Snowling , M. J. 1999 . Frequency acuity and binaural masking release in dyslexic listeners . Journal of the Acoustical Society of America , 106 : 53 – 58 .
- Hulme , C. and Roodenrys , S. 1995 . Practitioner review: Verbal working memory development and its disorders . Journal of Child Psychology and Psychiatry , 36 : 373 – 398 .
- Hulslander , J. , Talcott , J. , Witton , C. , DeFries , J. , Pennington , B. Wadsworth , S. 2004 . Sensory processing, reading, IQ, and attention . Journal of Experimental Child Psychology , 88 : 274 – 295 .
- Jorm , A. F. 1983 . Specific reading retardation and working memory: A review . British Journal of Psychology , 74 : 311 – 342 .
- Kaufman , A. S. and Kaufman , N. L. 1990 . Kaufman Brief Intelligence Test: Manual , Circle Pines, MN : American Guidance Service .
- Kronbichler , M. , Hutzler , F. and Wimmer , H. 2002 . Dyslexia: Verbal impairments in the absence of magnocellular impairments . NeuroReport , 13 : 617 – 620 .
- Laasonen , M. , Service , E. and Virsu , V. 2001 . Temporal order and processing acuity of visual, auditory, and tactile perception in developmentally dyslexic young adults . Cognitive, Affective and Behavioural Neuroscience , 1 : 394 – 410 .
- Laasonen , M. , Service , E. and Virsu , V. 2002 . Crossmodal temporal order and processing acuity in developmentally dyslexic young adults . Brain and Language , 80 : 340 – 354 .
- Lovegrove , W. , Martin , F. and Slaghuis , W. 1986 . A theoretical and experimental case for a visual deficit in specific reading disability . Cognitive Neuropsychology , 3 : 225 – 267 .
- McAnally , K. and Stein , J. F. Auditory temporal coding in dyslexia . Proceedings of the Royal Society of London, Series . Vol. 263 , pp. 961 – 965 .
- McArthur , G. M. and Bishop , D. V. M. 2001 . Auditory perceptual processing in people with reading and oral language impairments: Current issues and recommendations . Dyslexia , 7 : 150 – 170 .
- McArthur , G. M. and Bishop , D. V. M. 2004 . Frequency discrimination deficits in people with specific language impairment: Reliability, validity, and linguistic correlations . Journal of Speech Language and Hearing Research , 47 : 527 – 541 .
- McArthur , G. M. and Hogben , J. H. 2001 . Auditory backward recognition masking in children with a specific language impairment and children with a specific reading disability . Journal of the Acoustical Society of America , 109 : 1092 – 1100 .
- Mengler , E. D. , Hogben , J. H. , Michie , P. and Bishop , D. V. M. 2005 . Poor frequency discrimination is related to oral language disorder in children: A psychoacoustic study . Dyslexia , 11 : 1 – 19 .
- Olson , R. K. , Kliegl , R. , Davidson , B. J. and Foltz , G. 1985 . “ Individual and developmental differences in reading disability ” . In Reading research: Advances in theory and practice , Edited by: Waller , G. E. MacKinnon T. G. Vol. 4 , 1 – 64 . New York : Academic Press .
- Protopapas , A. , Ahissar , M. and Merzenich , M. M. 1997 . Auditory processing is related to reading ability . Journal of the Acoustical Society of America , 102 : 3188
- Ramus , F. 2003 . Developmental dyslexia: A specific phonological or general sensorimotor dysfunction . Current Opinion in Neurobiology , 13 : 212 – 218 .
- Ramus , F. 2004 . Neurobiology of dyslexia: A reinterpretation of the data . Trends in Neurosciences , 27 : 720 – 726 .
- Ramus , F. , Rosen , S. , Dakin , S. C. , Day , B. L. , Castellote , J. M. White , S. 2003 . Theories of developmental dyslexia: Insights from a multiple case study of dyslexic adults . Brain , 126 : 841 – 865 .
- Roach , N. W. , Edwards , V. T. and Hogben , J. H. 2004 . The tale is in the tail: An alternative hypothesis for psychophysical performance variability in dyslexia . Perception , 33 : 817 – 830 .
- Rosen , S. and Manganari , E. 2001 . Is there a relationship between speech and nonspeech auditory processing in children with dyslexia? . Journal of Speech, Language and Hearing Research , 44 : 720 – 736 .
- Skottun , B. C. 2000 . The magnocellular deficit theory of dyslexia: The evidence from contrast sensitivity . Vision Research , 40 : 111 – 127 .
- Standards Association of Australia . 1983 . Australian standards on audiometers , Sydney, , Australia : Author . (No. 2586)
- Stein , J. 2001 . The magnocellular theory of developmental dyslexia . Dyslexia , 7 : 12 – 36 .
- Talcott , J. B. , Gram , A. , Van Ingelghem , M. , Witton , C. , Stein , J. F. and Toennessen , F. E. 2003 . Impaired sensitivity to dynamic stimuli in poor readers of a regular orthography . Brain and Language , 87 : 259 – 266 .
- Talcott , J. B. , Hansen , P. C. , Willis-Owen , C. , McKinnell , I. W. , Richardson , A. J. and Stein , J. F. 1998 . Visual magnocellular impairment in adult developmental dyslexics . Neuro-ophthalmology , 20 : 187 – 201 .
- Talcott , J. B. , Witton , C. , Hebb , G. S. , Stoodley , C. J. , Westwood , E. A. France , S. J. 2002 . On the relationship between dynamic visual and auditory processing and literacy skills; results from a large primary-school study . Dyslexia , 8 : 204 – 225 .
- Talcott , J. B. , Witton , C. , McLean , M. F. , Hansen , P. C. , Rees , A. Green , G. G. R. Dynamic sensory sensitivity and children's word decoding skills . Proceedings of the National Academy of Sciences . Vol. 97 , pp. 2952 – 2957 .
- Tallal , P. 1980 . Auditory temporal perception, phonics, and reading disabilities in children . Brain and Language , 9 : 182 – 198 .
- Taylor , M. M. and Creelman , C. D. 1967 . PEST: Efficient estimates on probability functions . Journal of the Acoustical Society of America , 41 : 782 – 787 .
- Thorndike , R. L. , Hagen , E. P. and Sattler , J. M. 1986 . Stanford–Binet Intelligence Scale–4th Edition: Manual , Chicago, IL : Riverside Publishing Company .
- Torgesen , J. K. , Wagner , T. K. and Rashotte , C. A. 1999 . Test of Word Reading Efficiency: Manual , Austin, TX : Pro-Ed .
- Van Ingelghem , M. , van Wieringen , A. , Wouters , J. , Vandenbussche , E. , Onghena , P. and Ghesquiere , P. 2001 . Psychophysical evidence for a general temporal processing deficit in children with dyslexia . NeuroReport , 12 : 3604 – 3607 .
- Wagner , R. K. and Torgesen , J. K. 1987 . The nature of phonological processing and its causal role in the acquisition of reading skills . Psychological Bulletin , 101 : 192 – 212 .
- Wagner , R. K. , Torgesen , J. K. and Rashotte , C. A. 1999 . Comprehensive Test of Phonological Processing: Manual , Austin, TX : Pro-Ed .
- Walker , M. M. , Shinn , J. B. , Cranford , J. L. , Givens , G. D. and Holbert , D. 2002 . Auditory temporal processing performance of young adults with reading disorders . Journal of Speech, Language and Hearing Research , 45 : 598 – 605 .
- Walther-Mueller , P. U. 1995 . Is there a deficit of early vision in dyslexia? . Perception , 24 : 919 – 936 .
- Wilkinson , G. S. 1993 . Wide Range Achievement Tests–3rd Edition: Manual , Wilmington, DE : Wide Range Inc .
- Witton , C. , Stein , J. F. , Stoodley , C. J. , Rosner , B. S. and Talcott , J. B. 2002 . Separate influences of acoustic AM and FM sensitivity on the phonological decoding skills of impaired and normal readers . Journal of Cognitive Neuroscience , 14 : 866 – 874 .
- Witton , C. , Talcott , J. B. , Hansen , P. C. , Richardson , A. J. , Griffiths , T. D. Rees , A. 1998 . Sensitivity to dynamic auditory and visual stimuli predicts nonword reading ability in both dyslexic and normal readers . Current Biology , 8 : 791 – 797 .
- Woodcock , R. N. 1987 . Woodcock Reading Mastery Tests–Revised: Examiner's Manual , Circle Pines, MN : American Guidance Service .
- Wright , B. A. , Lombardino , L. J. , King , W. M. , Puranik , C. S. , Leonard , C. M. and Merzenich , M. M. 1997 . Deficits in auditory temporal and spectral resolution in language-impaired children . Nature , 387 : 176 – 178 .